A Career in AI Felt Impossible. Then He Took the Course That Changed Everything
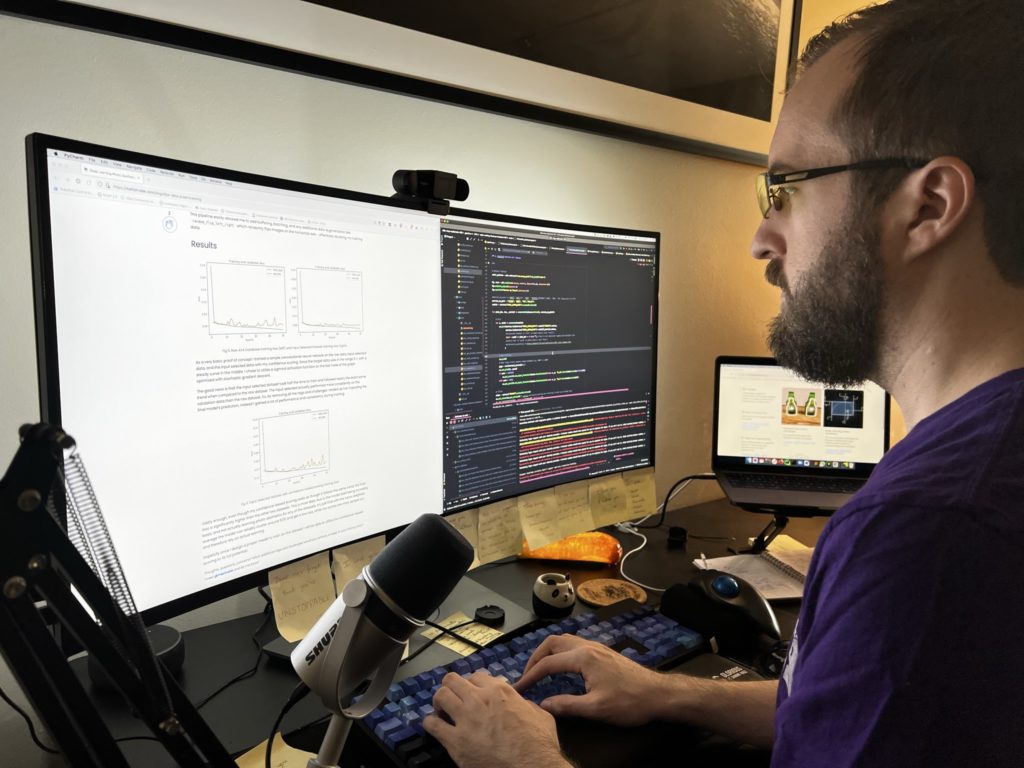
“I had an interest in AI and had dabbled in it a little bit, but always felt like an outsider looking in. Then I was able to accomplish what previously seemed impossible, like understanding academic papers and building my own models from scratch.”
Name: Matt Struble
Title: Senior Machine Learning Engineer
Location: Boston, Massachusetts, U.S.
Education: Bachelor of Science, Game programming from Champlain College; Master of Science, Computer Technology from Georgia Tech
Favorite ML area: Computer vision and Fintech
Favorite video game: The Legend of Zelda series
Matt Struble is an engineer at a sportswear company, where he currently leads a team that’s developing a deep learning system for predicting shoe trends a year or two into the future. Before taking the Deep Learning Specialization, he was a computer programmer who watched data scientists from afar. He spoke with us about how the course helped him overcome his Imposter syndrome and land a job he loves.
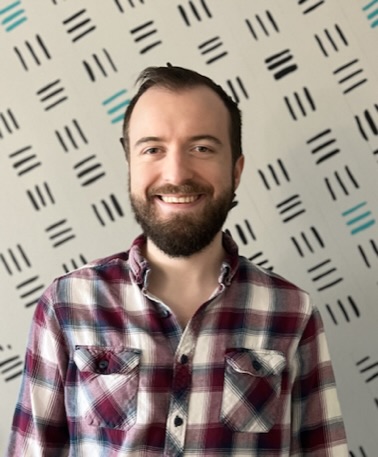
What were you doing before you became a deep learning engineer?
MS: Before the Deep Learning Specialization, I was a contractor at Draper, an engineering company in Boston. My responsibilities included managing flight data and developing guidance navigation control algorithms for various rocket propellant systems. I was essentially creating a data warehouse that enabled other scientists to go through and perform analysis.
That sounds like a really cool job. Why did you decide to leave?
MS: Due to the pandemic, Draper was unable to continue with contractors on board, and gave me a choice to become a full-time software engineer. Instead, I chose to use the time to pivot my career into machine learning and truly get out of my comfort zone.
Why did you decide to enroll in the Deep Learning Specialization?
MS: I always knew that I wanted to do more than just basic software engineering. At Draper, I would see what the data scientists were doing and always had the desire to be more hands-on and not just a supporting software engineer. I had an interest in AI and dabbled a bit, but I always felt like an outsider. I felt like a hobbyist, poking my head in where I didn’t belong, making assumptions but not really contributing anything to the field. The Specialization helped me understand what was under the hood of machine learning and provided me that extra reassurance that I did understand things. From there, I achieved things that previously seemed impossible, like understanding academic papers and building my own projects from scratch.
You’re working as a machine learning engineer at a major tech company. How does deep learning fit into your daily work now?
MS: I’m the lead engineer of a team that does digital demand forecasting. A lot of my day-to-day work is analyzing data streams of past shoe demand and developing models that produce predictions of what style is going to be the most sought-after during, say, the 2023 holiday season. I work closely with our data scientists, project managers, and other software engineers to see what the marketplace will look like.
I’m also currently working on a side project where I’m developing a deep learning model to rate and gauge the aesthetics of a photo. So this involves training a computer vision system to do something similar to what people do when they are trying to pick the best image out of 30 similar photos. We plan to release that as a phone app in the future.
How would you describe your job satisfaction now instead of how you felt before becoming a deep learning engineer?
MS: I feel like I’m more involved in AI, and that in itself is super rewarding. I’m a part of this cutting-edge ecosystem. I’m involved in conversations. I can read, understand, and recreate research papers. Before, all of this stuff was a mumbo jumbo mystery!
What did you find most impactful about the Deep Learning Specialization?
MS: Overall, it was very helpful to understand things like model workflow, data pre-processing and post-processing, and other prep work that goes into creating a model. Creating a model is only a small part of being an engineer; understanding what’s going on beneath the hood lets you know what to do if your model starts exhibiting biases or making weird predictions. So you know what’s going wrong and how to pivot in the right direction.
Ready to #BeADeepLearner like Matt? Enroll in the Deep Learning Specialization
You can find Matt Struble on LinkedIn.