Working AI: How an Accomplished Data Scientist Found Job Satisfaction
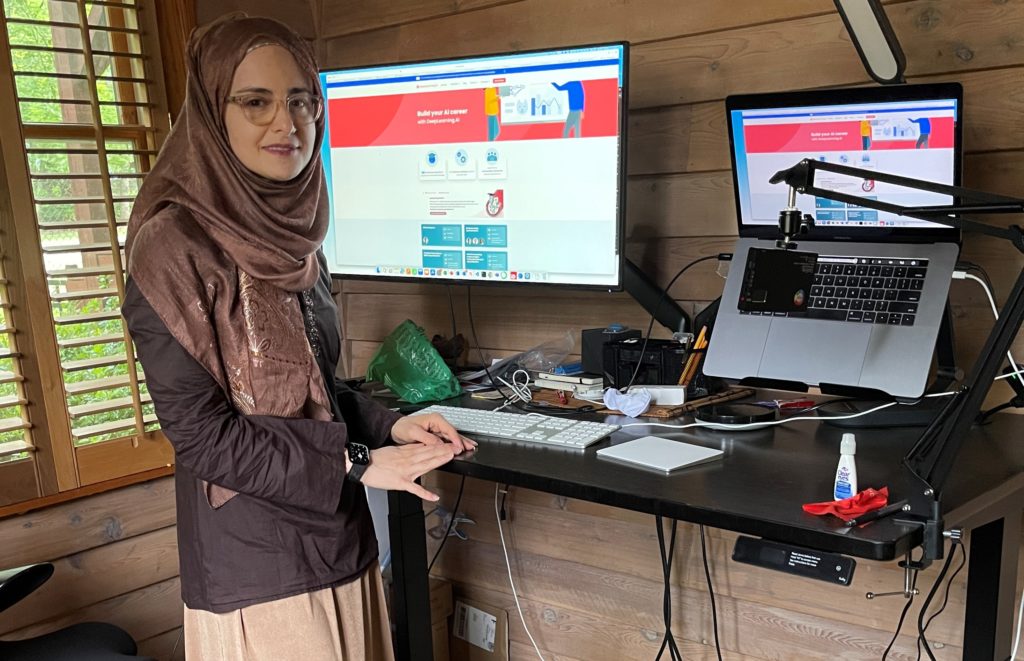
Name: Kulsoom Abdullah
Title: Principal Data Scientist, Anthem, Inc.
Location: Atlanta, Georgia, United States
Education: BS, Computer Engineering, University of Central Florida; MS, Electrical and Computer Engineering, Georgia Tech; PhD, Electrical and Computer Engineering, Georgia Tech
Most Memorable Travel Destination: South Korea
Kulsoom Abdullah pivoted from network security into a data science role after taking Andrew Ng’s original Introduction to Machine Learning course from Stanford in 2013. A self-described learning addict, she has taken more than 10 online courses, most recently the Deep Learning Specialization. Outside of work, she’s a fitness fanatic, and was the first competitive weightlifter to compete professionally for Pakistan — after winning a fight to change the dress code to allow religious head coverings. She spoke with us about overcoming bias, finding a global community of AI friends, and why she always challenges herself with a personal project after taking an online course.
To start, can you tell us about your current role?
KA: I am a senior data scientist at Anthem, a health insurance company. I work in a group called program integrity, where our goal is detecting overpayments, misuse, fraud, and other anomalies. We work with auditors, who are subject matter experts, to validate whether the patterns we detect are legit.
What data science projects are you currently working on?
One of the biggest challenges we’re focused on is making the data more uniform. When you’re dealing with health insurance in the U.S. you have data coming from government providers, like Medicare and Medicaid, as well as private plans. Then there are different facilities, different practitioners, and so on. It’s all disjointed, so we want to get all that data into formats where it can all be easily used when modeling. This would let us build models that don’t just look at claims retroactively, but could capture claims as they come in.
Have you always been into computers and technology?
I didn’t have much access to tech growing up in a small town, and there was very little exposure to engineering. But I remember being interested in math, and not so much in English, literature, and other subjects. When I was preparing to go to undergraduate, I knew I wanted to get into something that was math-oriented. I looked at the choices of majors and did a process of elimination, and it seemed like engineering was the best fit for my interests.
Did you encounter prejudice growing up?
I did encounter biases based on my race or religion, but not because of my gender. When I decided to pursue an engineering degree in college I was not yet aware of anything like gender bias. I do sometimes wonder if I would have gotten into engineering if I knew what the struggles were going to be.
In undergrad there were classes where I was the only female, and those tended to happen when I was in my third or fourth year. I think I really became aware of it, however, in graduate school, where I encountered sexist professors. And in the work environment my ideas would be ignored or outright denied, then acknowledged when a male colleague brought up the same thing in a slightly different way. When this happened it made me feel like I was hallucinating.
I was also treated poorly if I didn’t know every single thing there was to know about my job. I remember having a co-worker raise his voice in anger because I wasn’t typing fast enough in a programming language that I didn’t know. At the time, I internalized those experiences as though something was wrong with me. I’ve since learned that this is called imposter’s syndrome, and it was a product of being in toxic environments. This is partly what led me to seek out a new career and to discover AI through Dr. Ng’s Machine Learning course.
What can the machine learning community do to be more inviting to people from diverse religious or cultural backgrounds?
In the machine learning field, there are no clearly written rules barring anybody from participating, but there are obvious disparities in terms of who is involved in the field. There are very small populations of women and certain minority groups. There are various reasons for that. One reason is because there aren’t enough women or minorities getting an education in computer science. This problem has many roots, but it may be because people from certain backgrounds don’t feel as welcome. For example, a person like me may have a hard time getting a job because of what I’m wearing. But because people want to be politically correct, you can never be totally sure when and where these biases actually exist.
But I think this is beginning to change, and the field of machine learning is becoming more accepting. I think that many people in machine learning are realizing that we aren’t going to have successful models if we don’t have diverse perspectives. It’s not even a social justice issue, this lack of diversity results in models that are bad for business.
In addition to your Ph.D, you have taken nearly a dozen online courses. What is the value of online courses compared to a traditional education?
In college, if professors described a concept I didn’t understand or was more curious about, I would go online and dig in deeper. Looking back, if MOOCs were available when I was going through college I definitely would have used them. They would have been a great way to supplement a traditional course. I take them now because I think I am addicted to learning. If I could be a student for life, I definitely would.
Do you use outside projects to supplement your learning?
I like to practice what I’ve learned on real-life problems. You can use your new knowledge within the constraints of a project, experiment with different options, and figure out how to continue. For instance, when I completed the Deep Learning Specialization I wanted to do something in the domain of medicine, and I had never done a deep learning computer vision project before. With help from a mentor, I created a multi-label classification model for x-rays working with a pre-existing dataset.
Are there any other projects you are proud of working on?
I have done some volunteer work with an organization called Omdena, an organization that uses AI to solve health, social, and environmental challenges. Each project typically lasts around one to two months. One project I’m really proud of was helping a South African startup develop an AI system that allowed them to detect breast tumors using low-resolution sonograms.
Do you have any advice for people who are entering the field?
It can be tough just being yourself. You may doubt yourself and feel like you need to change. If you are experiencing challenges with being accepted, try to seek out others who are supportive so you don’t feel so alone. It isn’t always possible, because everybody needs to pay the bills, but you can try to be vocal and change your environment if it is not letting you be true to yourself.
How do you keep learning?
I am part of a women’s group called AI Wonder Girls. Most of us met doing volunteer work with Omdena. We are all over the world, so we communicate online and sometimes collaborate on projects or hackathons. It’s been really nice having this group to socialize with, especially while everything has been remote during the pandemic. When things get better we hope to meet in person.
Besides that, I try to use social media as a filter, because there is so much new research coming out all the time. Whenever I’m considering what to read, I try to evaluate how it will help me with what I’m trying to work on at that moment. Keeping my current goal in mind helps me remember that I can’t do too many things at once, because there are so many things I want to learn.
You can find Kulsoom Abdullah on LinkedIn.
Ready to #BeADeepLearner like Kulsoom?