My Journey into AI – learning resources recommended by the speakers
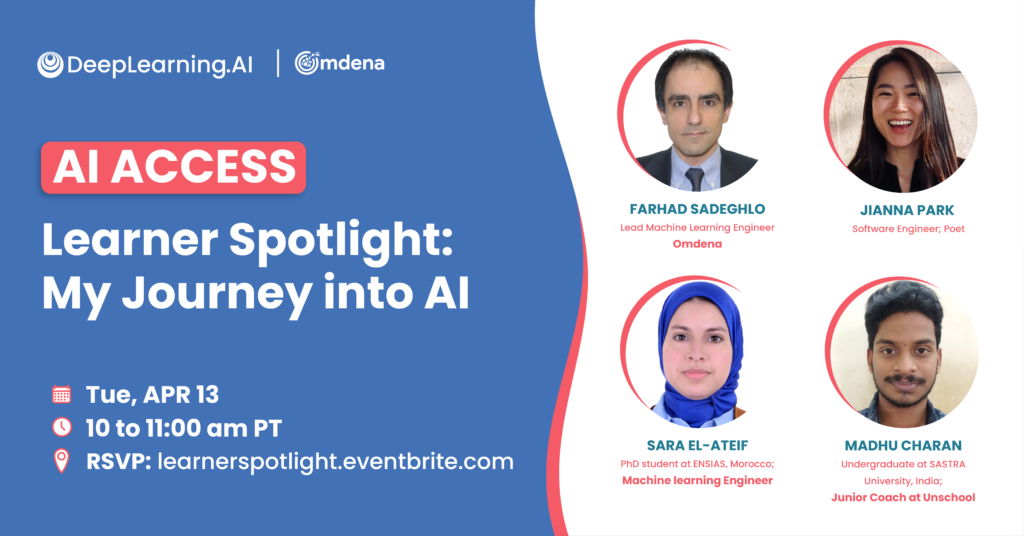
On April 13, DeepLearning.AI and Omdena assembled a panel of machine learning practitioners who shared their first-hand experience of going from non-traditional starting points to building a career in AI. For any aspiring machine learning engineers who missed the event, here’s a few of the speakers’ tips that we hope you’ll find helpful:
- The most important part of the job is cleaning data, so data science courses will be a good place to start.
- Try a bit of everything when you’re starting, especially when you’re not sure what to dedicate your time on
- Read a lot of articles on various topics like statistics, data science and NLP on Medium publications.
- It’s great to be a part of Slack workspaces where you can get and provide help
- If you can’t find mentors, look for co-learners
- Mentoring helps you better your own skills and knowledge base.
- Match your capabilities with the companies you are looking for
- Keep learning and evolving with the projects you pick up
- Good communication habits are key – learning to communicate well is just as important as technical skills
- I learn the most in the shortest time by being around people who are already doing the work and finding opportunities to collaborate with them
Additionally, the speakers shared a list of learning resources that they found useful:
- DeepLearning.AI online courses
- Aggregators
- Towards Data Science
- Medium
- DeepLearning.AIs newsletter The Batch
- Companies to follow
- Google AI
- Facebook AI
- Github
- Stack Overflow
- Kaggle Notebooks – The best way to learn implementations from top kagglers and competition winners
- Blogs/Newsletters/Research paper/Books:
- Blogs like Omdena to understand interesting implementations and approaches to solve a problem
- Hands on ML book by Aurélien
- Two Minute Papers on Youtube summarizes research papers
- Deep AI , Arxiv – websites and Twitter handle for latest research papers.
- Papers With Code for the latest datasets and implementations.
- Future Is Intersectional: talks and amazing resources presented by Spelman College, on topics of intersectionality, race, and ethics in AI/tech
- Jay Alammar’s illustrated series like this one helps visualize difficult concepts
- YouTube and Podcast channels:
- AIEngineering for End to End ML implementations.
- 3blue1brown for mathematics intuition.
- What’s AI for the latest advancements in AI to stay up to date with them
- Computerphile channel on Youtube also helped me understand some parts of NLP
- Practical AI
- Communities/Events
- PIE & AI meetups by DeepLearning.AI (get to hear other people’s stories and projects, network)
- Montréal.AI
- Women Who Code – Data Science: their community has an archive of recordings on topics of ML/NLP
- ML Explained – Aggregate Intellect
- Caleekal AI
- Influencers to follow
Click here to watch the full event recording.
Ready to break into AI?
Get started with the Deep Learning Specialization today!