Dear friends,
I am writing to you from Colombia today, and am excited to announce the opening of our office in Medellín. The office will serve as the Latin American headquarters for three of the companies in our AI ecosystem: Landing AI, deeplearning.ai, and AI Fund.
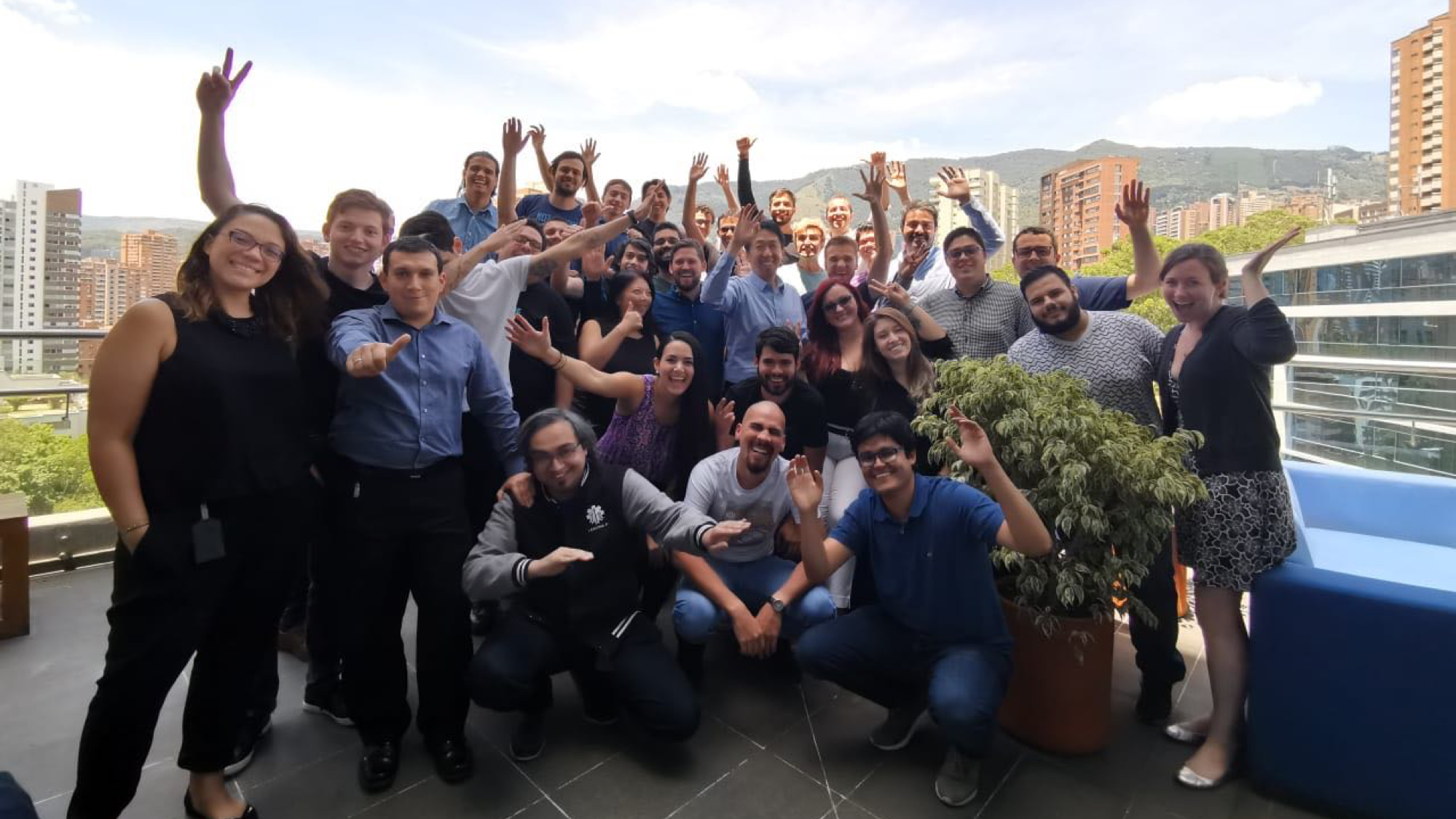
AI is still in its infancy. Although Silicon Valley and Beijing are currently leading the way in AI, with the UK and Canada also emerging as innovation hubs, there are still opportunities for every major country. Colombia is on a trajectory to become a hub of AI in Latin America.
I am proud to bet on Colombia and support the growth of the Colombian AI community and the broader Latin American AI community. You can find additional details here or in Frederic Lardinois’ TechCrunch article.
Keep learning!
Andrew
News
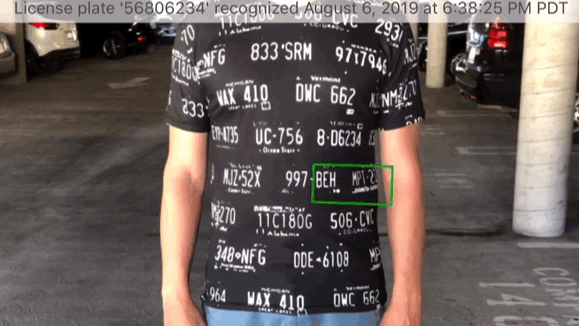
This Shirt Hates Surveillance
Automatic license plate readers capture thousands of vehicle IDs each minute, allowing law enforcement and private businesses to track drivers with or without their explicit consent. Fashion-forward freedom fighters are countering the algorithms with a line of shirts, dresses, and tops covered with images of license plates.
What’s new: Security researcher and clothing designer Kate Rose unveiled her Adversarial Fashion line at the Defcon hacker convention. The garments are meant to foul automatic license plate readers by diluting their databases with noise.
How it works: Such readers typically use optical character recognition to capture lettering found in rectangular shapes they identify as license plates. But they aren’t picky about whether those rectangles are attached to a car.
- Rose used an open source reader to optimize her designs until they had shapes, sizes, and lettering that fooled the software.
- Each time a reader captures a plate from Rose’s clothes, it takes in a line of meaningless data.
- With enough noise, such systems become less precise, require more human oversight, and cost more money to operate.
- Rose’s Defcon deck provides a fun overview.
Behind the news: Use of automatic license plate readers grew by 3,000 percent over the past two years, according to a February article in Quartz. Companies like OpenALPR and PlateSmart Technologies have spurred the trend by marketing their systems to casinos, hospitals, and schools.
Why it matters: ALPR technology isn’t useful only for catching scofflaws who blow through red lights. Bad actors with access to license plate tracking data can stalk an individual’s movements, according to the Electronic Frontier Foundation. They can create databases of people who regularly visit sensitive locations like women’s health clinics, immigration centers, and union halls.
We’re thinking: Rose’s designs aren’t likely to have a practical impact unless they become a widespread geek craze (and in that case, makers of license plate readers will respond by building in an adversarial clothing detector). Their real effect may be to spur a public conversation about the worrying proliferation of automated surveillance technology.
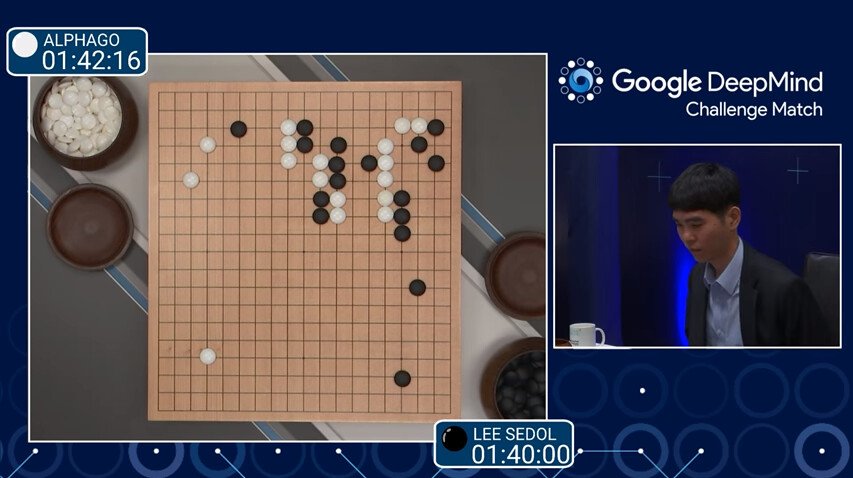
DeepMind Results Raise Questions
Alphabet subsidiary DeepMind lost $572 million in the past year, and its losses over the last three years amounted to more than $1 billion. AI contrarian Gary Marcus used the news as an opportunity to question the direction of AI as an industry.
What’s new: In an essay published by Wired, Marcus extrapolates DeepMind’s finances into an indictment of AI trends in recent years.
The blow-by-blow: He begins with a seeming defense of DeepMind, saying that the losses can be viewed as investments in cutting-edge research.
- But he quickly doubles back, suggesting the lack of a payoff indicates that DeepMind’s research focus, deep reinforcement learning, is a dead end.
- He calls out DRL’s failure to make progress towards artificial general intelligence and practical goals like self-driving vehicles.
- DeepMind’s expenditures aren’t just an expensive mistake, he claims. They rob research funding for worthier AI techniques, such as approaches based on cognitive science.
Behind the news: Marcus is a longtime critic of deep learning. He published a 10-point critique of deep learning’s shortcomings last year. He is currently promoting a book, Rebooting AI, arguing that the AI community should reorder its priorities to accommodate approaches that mimic human intelligence. In June, he announced a new venture, robust.ai, with roboticist Rodney Brooks.
Yes, but: As a tech company, Alphabet does well to invest in nascent technologies or risk being disrupted by them. As a public company, it has a fiduciary responsibility to do so. Moreover, DeepMind has achieved phenomenal successes at solving Go and StarCraft II and helped make Google’s data centers and Android devices run more efficiently.
What they’re saying: The essay created a stir on social media.
- Some voiced agreement with Marcus conclusions: “DeepMind struggles to achieve breakthrough results in transfer learning for at least two years. I believe part of them must see this as the key to AGI. I think deep nets are but one ingredient.” — @donbenham
- Others found Google’s investment well justified: “DeepMind may be over-invested in snake oil (DRL is lazy, brittle & struggles to scale past toy problems) but Google has 120B in cash sitting in the bank, w/ positive cash flow. DeepMind costs like 1% of profit, provides positive coverage, attracts talent, is a long odds bet, etc.” — @nicidob
- And many called out Marcus for ignoring DeepMind’s achievements: “What about @DeepMindAI’s protein folding success, using RL for data center cooling, WaveNet, etc, and their great neuroscience division?” — @blackHC
We’re thinking: Marcus warns that investors may abandon AI if big investments like DeepMind don’t start providing returns. But some AI approaches already are having a huge economic impact, and emerging techniques like DRL new enough that it makes little sense to predict doom for all approaches based on slow progress in one. Better to save such double-barreled criticism for AI that is malicious or inept. We disagree with Marcus’ views on deep learning, but cheer him on as he codes, tests, and iterates his own way forward.
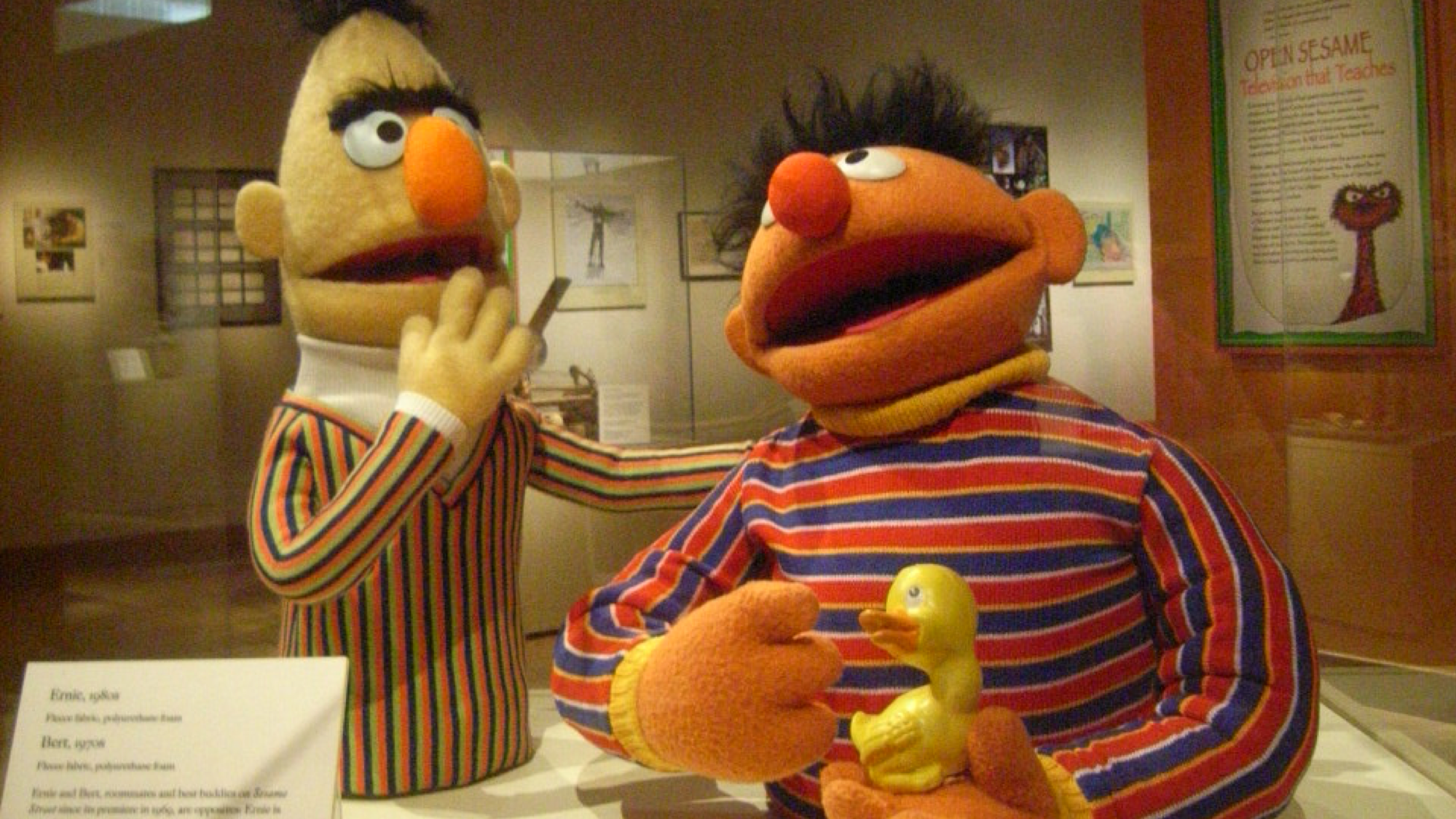
BERT Is Back
Less than a month after XLNet overtook BERT, the pole position in natural language understanding changed hands again. RoBERTa is an improved BERT pretraining recipe that beats its forbear, becoming the new state-of-the-art language model — for the moment.
What’s new: Researchers at Facebook AI and from the University of Washington modified BERT to beat the best published results on three popular benchmarks.
Key insight: Since BERT’s debut late last year, success in language modeling has been fueled not only by bigger models but also by an order of magnitude more data, more passes through the training set, and larger batch sizes. RoBERTa shows that these training choices can have a greater impact on performance than advances in model architecture.
How it works: RoBERTa uses the BERT LARGE configuration (355 million parameters) with an altered pretraining pipeline. Yinhan Liu and her colleagues made the following changes:
- Increased training data size from 16Gb to 160Gb by including three additional datasets.
- Boosted batch size from 256 sequences to 8,000 sequences per batch.
- Raised the number of pretraining steps from 31,000 to 500,000.
- Removed the next sentence prediction (NSP) loss term from the training objective and used full-sentence sequences as input instead of segment pairs.
- Fine-tuned for two of the nine tasks in the GLUE natural language understanding benchmark as well as for SQuAD (question answering) and RACE (reading comprehension).
Results: RoBERTa achieves state-of-the-art performance on GLUE without multi-task fine tuning, on SQuAD without additional data (unlike BERT and XLNet), and on RACE.
Yes, but: As the authors point out, the comparison would be fairer if XLNet and other language models were fine-tuned as rigorously as RoBERTa. The success of intensive fine-tuning raises the question whether researchers with limited resources can obtain state-of-the-art results in the problems they care about.
Why it matters: The authors show that rigorous tuning of hyperparameters and dataset size can play a decisive role in performance. The study highlights the importance of proper evaluation procedures for all new machine learning techniques.
We’re thinking: Researchers are just beginning to assess the impact of hyperparameter tuning and data set size on complex neural network architectures at scale of 100 to 1,000 million parameters. BERT is an early beneficiary, and there’s much more exploration to be done.
A MESSAGE FROM DEEPLEARNING.AI
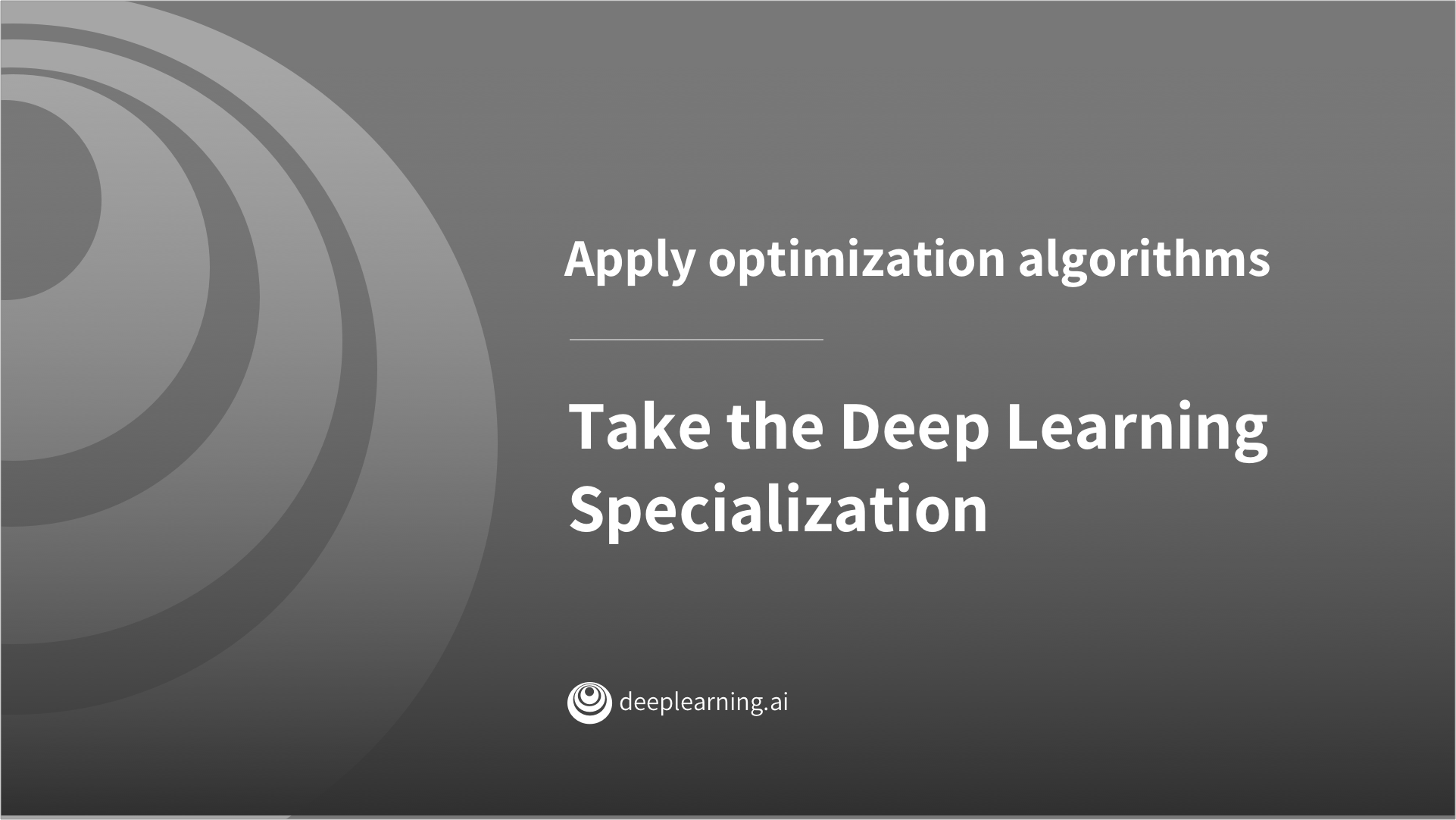
How do you check the convergence of optimization algorithms like momentum, RMSprop, and mini-batch gradient descent? Learn how in the Deep Learning Specialization.
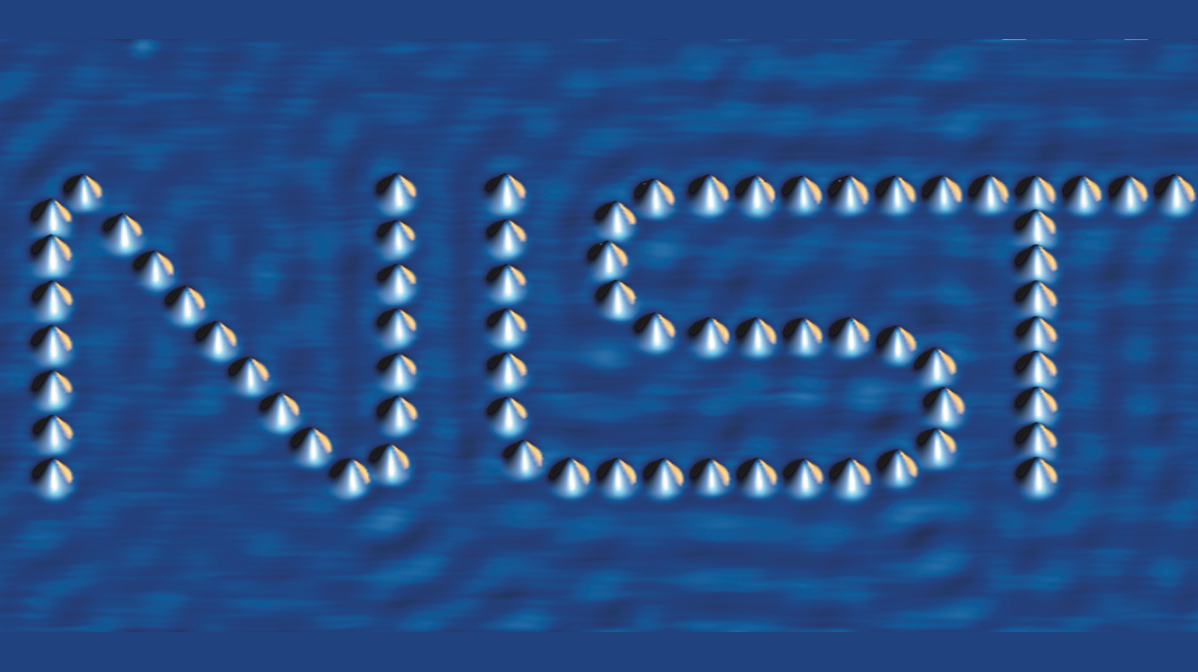
Standards in the Making
The U.S. federal government released a plan to develop technical standards for artificial intelligence, seeking to balance its aim to maintain the nation’s tech leadership and economic power with a priority on AI safety and trustworthiness.
What’s new: Responding to a February executive order, the National Institute of Standards and Technology issued its roadmap for developing AI standards that would guide federal policy and applications. The 46-page document seeks to foster standards strict enough to prevent harm but flexible enough to drive innovation.
What it says: The plan describes a broad effort to standardize in areas as disparate as terminology and user interfaces, benchmarking and risk management. It calls for coordination among public agencies, institutions, businesses, and foreign countries, emphasizing the need to develop trustworthy AI systems that are accurate, reliable, secure, and transparent.
Yes, but: The authors acknowledge the risk of trying to corral such a dynamic enterprise. In fact, they admit that aren’t entirely sure how to go about it. “While there is broad agreement that these issues must factor into US standards,” they write, “it is not clear how that should be done and whether there is yet sufficient scientific and technical basis to develop those standards provisions.
We’re thinking: NIST’s plan drives a stake in the ground for equity, inclusivity, and cooperation. Here’s hoping it can bake those values — which is not to say specific implementations — into the national tech infrastructure and spread them abroad.
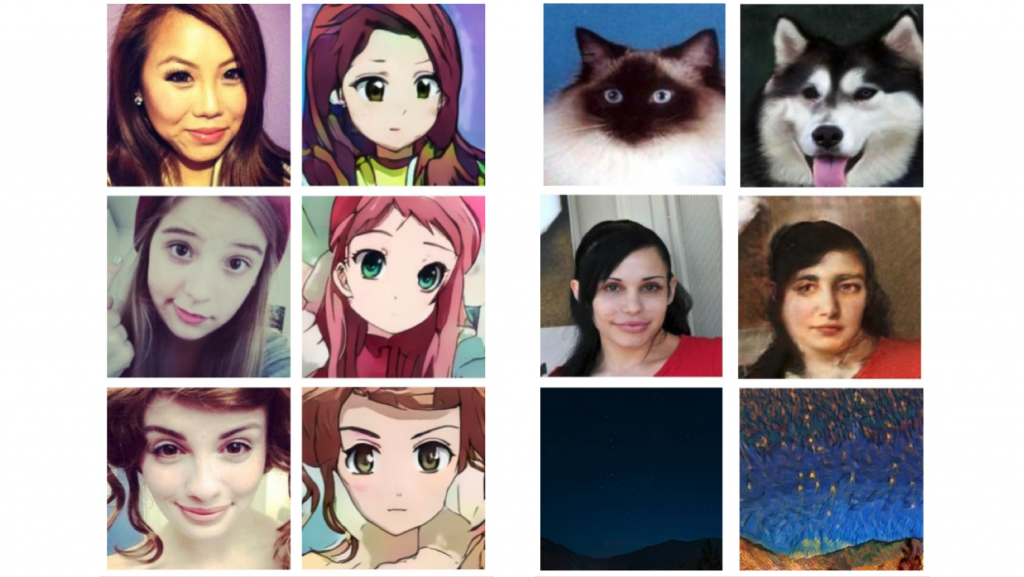
Style Upgrade
Image-to-image translation, in which stylistic features from one image are imposed on the content of another to create a new picture, traditionally has been limited to translating either shapes or textures. A new network translates both, allowing more flexible image combinations and creating more visually satisfying output.
What’s new: A team from Boeing’s South Korea lab created U-GAT-IT, a network that produces superior translations between images.
Key insights: Where earlier image-to-image translation networks work best with particular image styles, U-GAT-IT adds layers that make it useful across a variety of styles.
- Such networks typically represent shapes and textures in hidden feature maps. U-GAT-IT adds a layer that weights the importance of each feature map based on each image’s style.
- The researchers also introduce a layer that learns which normalization method works best.
How it works: U-GAT-IT uses a typical GAN architecture: A discriminator classifies images as either real or generated and a generator tries to fool the discriminator. It accepts two image inputs.
- The generator takes the images and uses a CNN to extract feature maps that encode shapes and textures.
- In earlier models, feature maps are passed directly to an attention layer that models the correspondence between pixels in each image. In U-GAT-IT, an intermediate weighting layer learns the importance of each feature map. The weights allow the system to distinguish the importance of different textures and shapes in each style.
- The weighted feature maps are passed to the attention layer to assess pixel correspondences, and the generator produces an image from there.
- The discriminator takes the first image as a real-world style example and the second as a candidate in the same style that’s either real or generated.
- Like the generator, it encodes both images to feature maps via a CNN and uses a weighting layer to guide an attention layer.
- The discriminator classifies the candidate image based on the attention layer’s output.
Results: Test subjects chose their favorite images from a selection of translations by U-GAT-IT and four earlier methods. The subjects preferred U-GAT-IT’s output by up to 73% in four out of five data sets.
Why it matters: Image-to-image translation is a hot topic with many practical applications. Professional image editors use it to boost image resolution and colorize black-and-white photos. Consumers enjoy the technology in apps like FaceApp.
We’re thinking: The best-performing deepfake networks lean heavily on image-translation techniques. A new generation that takes advantage of U-GAT-IT’s simultaneous shape-and-texture modeling may produce even more convincing fake pictures.