Dear friends,
Recently I attended an online celebration of my late grandfather’s life. He had passed away quietly in his sleep in March. Two days later, Coursera was publicly listed on the New York Stock Exchange. And two days after that, my son Neo Atlas Ng was born.
The sequence of events reminds me that every day is precious. Had my grandfather lived just a few more days, he would have shared in the joy of his first great grandson’s birth and the celebration of Coursera’s listing.
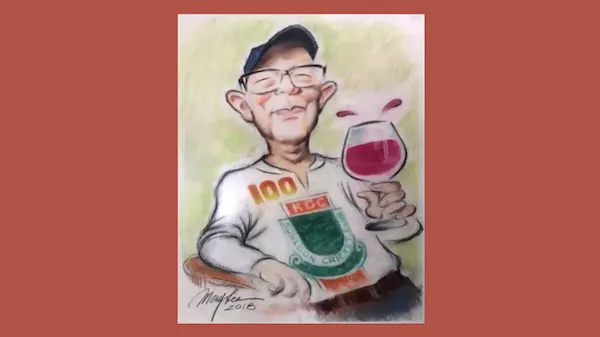
My grandfather lived a remarkable life. He was born in Jamaica in 1918 during one pandemic, and he passed away 102 years later during another. His father was an indentured laborer who had moved from China to Jamaica, and his mother was half Jamaican. (Thus I’m 1/16 Jamaican.) As a young man, he sailed from the Caribbean through the Panama canal to settle in Hong Kong, where he had a fruitful career as an accountant and spent his last few years holding court at his beloved Kowloon Cricket Club.
If you’ve lost a loved one, you probably miss them as much as I do my grandfather. It goes to show that even if someone close to you lives to 102, likely it will feel like it’s not enough. If only he had lived four more days — or four more years — he could have shared in even more joy.
I’m grateful for the time I had with my grandfather. I hope you’ll take care of yourself so that you, too, can live a long life. Let’s squeeze every drop of joy out of life in the time we have.
Love,
Andrew
News
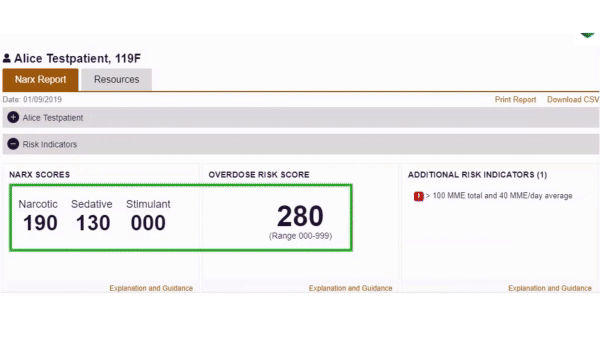
Fighting Addiction or Denying Care?
An epidemic of opioid abuse in the U.S. killed 93,000 people in 2020 alone. An algorithm intended to help doctors prescribe the drugs responsibly may be barring worthy patients from pain relief.
What’s new: A widely used system assesses whether individual patients are at risk of abusing opioids. In some cases, it has recommended denying painkillers to people who suffer from severe pain and have no history of drug abuse, Wired reported.
How it happened: Most U.S. states have developed databases that track drug prescriptions. NarxCare, a system developed by medical technology company Appriss Health, analyzes such data for at least eight states.
- Given a patient’s name, NarxCare considers drugs and doses prescribed, numbers of doctors and pharmacies involved, and whether any prescriptions overlap. It produces scores that evaluate the risk that the patient will abuse opioids and other drugs, and a score that evaluates the risk that they will overdose. Appriss says the scores are meant to assist, not to overrule or replace, a doctor’s decision.
- Several patients interviewed by Wired said they were denied care or were dropped by their doctors after receiving mistakenly elevated scores. In one case, veterinary drugs purchased for pets contributed to a high score.
- Some behaviors used by such algorithms to generate risk scores — such as visiting multiple doctors or traveling long distances for care — may artificially inflate scores for people who have multiple conditions or live in rural areas, according to a recent study.
Behind the news: Flawed algorithms unexpectedly have cut healthcare benefits to many U.S. citizens, leaving them without care or a way to appeal the decision.
Why it matters: Most people who have opioid prescriptions are not addicts. Cutting them off from painkillers not only leaves them to suffer, it also could drive them to obtain the drugs illegally or harm themselves with illicit substitutes.
We’re thinking: Efforts to limit prescriptions of opioids could save countless people from addiction, and AI can play an important role. Stories of patients who have been denied care highlight the pressing need to improve and audit AI systems, even as they help us avoid fueling the opioid epidemic.
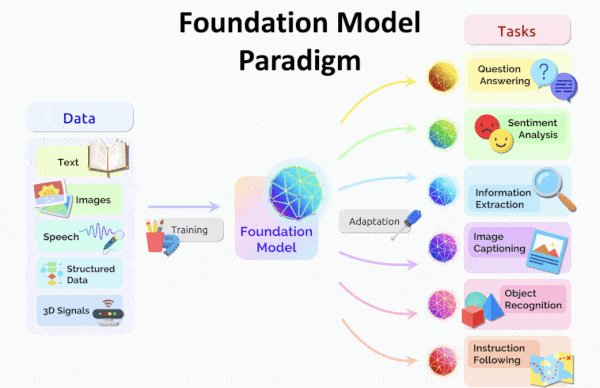
Weak Foundations Make Weak Models
A new study examines a major strain of recent research: huge models pretrained on immense quantities of uncurated, unlabeled data and then fine-tuned on a smaller, curated corpus. The sprawling 200-page document evaluates the benefits and risks.
What’s new: Researchers at Stanford’s Human AI Institute proposed ways to prevent large language models like BERT, CLIP, and GPT-3 — which they call foundation models for their ability to support a plethora of high-performance, fine-tuned variations — from manifesting hidden flaws after fine-tuning.
Key insight: The very factors that make large language models so valuable — unsupervised training followed by adaptation to a wide variety of tasks (indeed, some outside the domain of natural language) — make them potential vectors for harm. Defects in the foundation, such as biases learned from uncurated training data, can emerge in fine-tuned versions as challenges to fairness, ethical use, and legal compliance. Moreover, this approach encourages a technological monoculture in which a limited number of architectures, despite their strengths, proliferate their weaknesses across various domains.
Toward solid foundations: The authors recommend ways to minimize unwelcome surprises such as unwitting contributions to social or economic inequality, unemployment, or disinformation:
- Develop metrics that predict ways in which a model may instill harmful behavior in its fine-tuned offspring and standardized ways to document these metrics, for instance data sheets.
- Create incentives for companies that develop large-scale, unsupervised models to publicly test and audit their work. Warn developers of follow-on systems to vet them thoroughly for undesired behaviors prior to deployment.
- Counterbalance the power of deep-pocketed companies by making it easier for academic institutions and independent researchers to develop such models, for instance through a National Research Cloud and crowdsourced efforts to recreate GPT-style language models.
Behind the news: The advent of BERT in 2018 accelerated adoption of unsupervised pretraining in natural language models and spawned ever-larger networks as researchers scaled up the concept and experimented with architectures. The approach has spun off fine-tuned models not only for language tasks like conversation, image captioning, and internet search but also far-flung applications including modeling proteins, testing mathematical theorems, generating computer code, image recognition, image generation, and reinforcement learning.
Why it matters: Such models can cause harm due to intrinsic flaws by, say, propagating data-driven biases against members of particular religions or other groups) and extrinsic flaws, such as energy-intensive training that leaves a large carbon footprint and misuse such as propagating disinformation. Deep learning systems developed without foresight run the risk of becoming a burden rather than a boon.
We’re thinking: The future of AI may well be built on a limited variety of foundation models. In any case, the painstaking work of checking models for flaws beats cleaning up messes caused by neglecting to do so.
A MESSAGE FROM DEEPLEARNING.AI
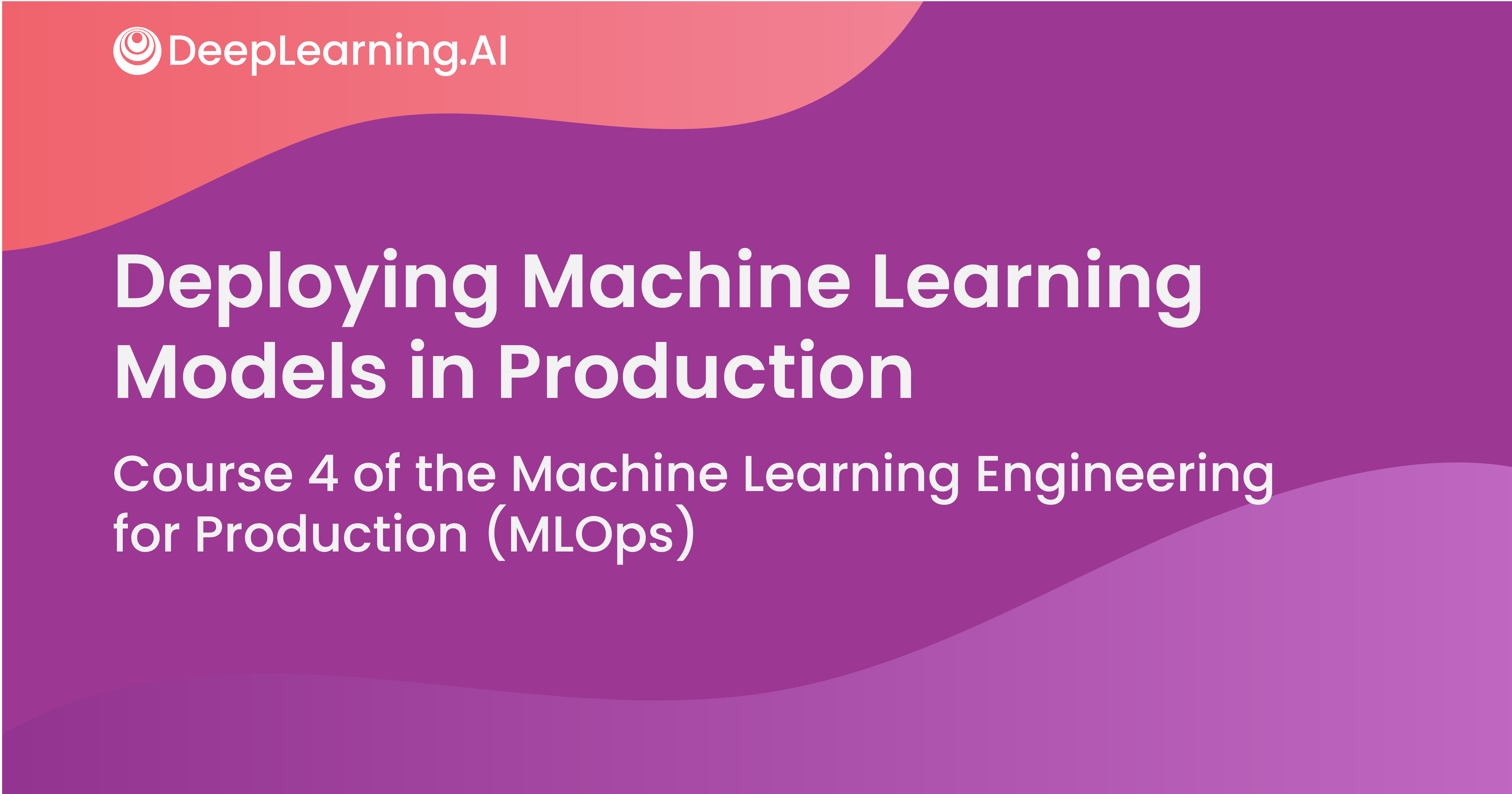
Mark your calendar: On September 8, 2021, we’re launching “Deploying Machine Learning Models in Production,” Course 4 of the Machine Learning Engineering for Production (MLOps) Specialization! Pre-enroll now
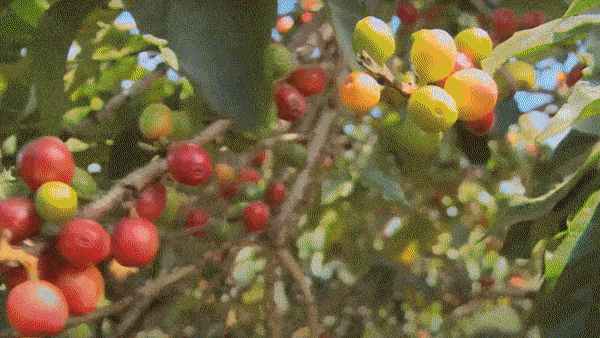
Wake Up and Smell the AI
Coffee producers are using machine learning to grow better beans.
What’s new: Beverage giant Nespresso is rolling out a system to assess the quality of hybrid coffee seedlings using technology from Israeli-Colombian startup Demetria.
How it works: Nespresso develops new coffee varieties by grafting plant seedlings. Previously it relied on human experts to assess whether these grafts were viable. Demetria’s algorithm uses readings from a handheld near-infrared optical scanner to automate the evaluation.
- The scanner measures light frequencies reflected by the plants, which the algorithm interprets as markers of plant health.
- In a three-month pilot program, Nespresso used the system to analyze over 240,000 plants. It sent the top-graded plants to farmers in Colombia.
- An earlier Demetria model lets farmers match the near-infrared signature of raw beans to established flavor categories. The company trained that model on taste and smell data recorded by human tasters.
- The company also offers a smartphone app for commercial coffee buyers that measures the size of individual coffee beans. Larger beans tend to produce better coffee.
Behind the news: The food and beverage industry has a growing appetite for AI.
- Tuna Scope is a computer vision-powered smartphone app that scans slices of fish to determine whether they are suitable for sushi.
- Indian startup Intello Labs has developed computer vision tools that assess the quality of various types of fruits and vegetables.
- Frito-Lay patented a machine learning system that analyzes laser readings of individual chips to grade their texture.
Why it matters: Nespresso believes that Demetria’s technology will save time and money. This may be bad for traditional plant assessors, whose skills may become obsolete. On the other hand, it may help struggling Colombian coffee farmers grow more profitable beans.
We’re thinking: The thought of better coffee through AI perked us right up.
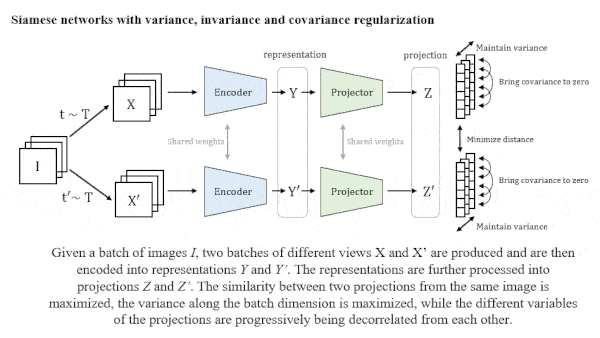
More Reliable Pretraining
Pretraining methods generate basic representations for later fine-tuning, but they’re prone to certain issues that can throw them off-kilter. New work proposes a solution.
What’s new: Researchers at Facebook, PSL Research University, and New York University led by Adrien Bardes devised an unsupervised pretraining method they call Variance-Invariance-Covariance Regularization (VICReg). VICReg helps a model learn useful representations based on well understood statistical principles.
Key insight: Pretraining methods can suffer from three common failings: Generating an identical representation for different input examples (which leads to predicting the mean consistently in linear regression), generating dissimilar representations for examples that humans find similar (for instance, the same object viewed from two angles), and generating redundant parts of a representation (say, multiple vectors that represent two eyes in a photo of a face). Statistically speaking, these problems boil down to issues of variance, invariance, and covariance respectively.
How it works: VICReg manages variance, invariance, and covariance via different terms in a loss function. The authors used it to pretrain a ResNet-50 on ImageNet without labels.
- To discourage similar representations of every example, the variance term of VICReg’s loss function computes the variance within an input batch’s representations; that is, the average amount by which each value differs from the mean. This term penalizes the model if this variance falls below a threshold.
- The covariance term computes correlations between elements of each representation. It sums the correlations and penalizes the model for extracting correlated features within a given representation.
- To prevent dissimilar representations of similar examples, VICReg borrows an idea from contrastive learning: It uses data augmentation. Two different, random augmentations are applied to each example, and the model processes them separately to generate two different, but related, representations. The invariance term computes the distance between them. The greater the distance, the greater the penalty.
Results: The authors transferred the VICReg-trained ResNet-50’s representations to a linear classifier and trained it on ImageNet with labels. That model achieved a 73.2 percent accuracy, just shy of the 76.5 percent achieved by a supervised ResNet-50. A linear classifier using representations from a ResNet-50 pretrained using the contrastive learning method SimCLR achieved 69.3 percent accuracy.
Why it matters: Contrastive learning, a successful pretraining technique, requires a large number of comparisons between dissimilar inputs to ensure that not all representations are identical. VICReg avoids that issue by computing the variance within a batch, a much less memory-intensive operation.
We’re thinking: Comparing different augmentations of the same example has proven to be a powerful way to learn. This technique extends that approach, and we expect to see more.