Dear friends,
I invite you to be part of Pie & AI, a series of meetups that bring together members of the global AI community for education and conversation. Pie & AI is a place where you can network with peers, learn best practices from industry leaders, get hands-on practice from mentors, and engage in thought-provoking discussions.
Since our first Pie & AI shortly after March 14 — Pi Day — 2019, we’ve hosted over 500 events in 110 cities across 52 countries. More than 65,000 attendees have participated.
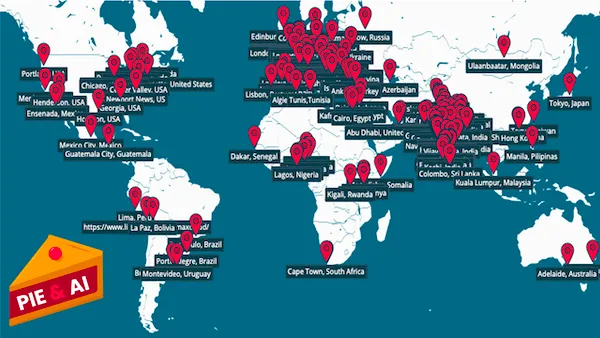
I’d like to thank our 200-plus event ambassadors. These extraordinary individuals organize gatherings that connect learners, practitioners, researchers, and special guests. In fact, this week marks the second anniversary of the Pie & AI Ambassador Program, which enables AI practitioners and enthusiasts to host Pie & AI events for their local community. To celebrate this anniversary, DeepLearning.AI is highlighting 10 event ambassadors. You can read their stories on our website. If you're interested in becoming an event ambassador yourself, please apply here.
All of us are stronger when we come together in a community and support each other. Please join us to share ideas and learn together!
Keep learning!
Andrew
News
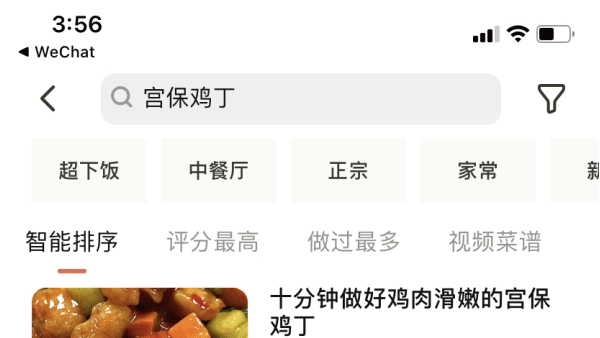
Rules for Recommenders
China moved toward a clamp down on recommendation algorithms.
What’s new: China’s internet regulatory agency proposed rules that include banning algorithms that spread disinformation, threaten national security, or encourage addictive behavior.
What it says: The plan by the Cyberspace Administration of China (CAC) broadly calls for recommendation engines to uphold China’s social order and “promote socialist core values.” The public has until September 26, 2021, to offer feedback. It’s not clear when the rules would take effect. Under the plan:
- Recommendation algorithms would not be allowed to encourage binges or exploit users’ behavior by, say, raising prices of goods they buy often.
- Content platforms would be required to tell users about their algorithms’ operating principles and audit them regularly to make sure they comply with CAC regulations. They would also have to allow users to disable automated recommendations easily.
- Algorithms that make false user accounts, generate disinformation, or violate an individual’s rights would be banned.
- Platforms would have to obtain approval before deploying recommendation algorithms capable of swaying public sentiment.
Behind the news: China is not alone in its national effort to rein in the influence of AI.
- The European Union released draft regulations that would ban or tightly restrict social scoring systems, real-time face recognition, and algorithms engineered to manipulate behavior.
- The Algorithmic Accountability Act, which is stalled in the U.S. Congress, would require companies to perform risk assessments before deploying systems that could spread disinformation or perpetuate social biases.
Why it matters: Recommendation algorithms can enable social media addiction, spread disinformation, and amplify extreme views.
We’re thinking: There’s a delicate balance between protecting the rights of consumers and limiting the freedoms of content providers who rely on platforms to get their message out. The AI community can help with the challenge of formulating thoughtful regulations.
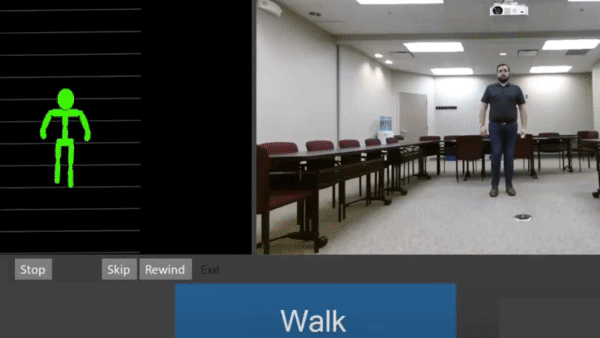
Risk Reduction for Elders
Deep learning is helping to protect elderly people from potentially catastrophic tumbles.
What’s happening: More than 2,000 senior living facilities across the U.S. use a diagnostic system called VirtuSense Balance to keep residents on their feet.
How it works: The system helps a specialist spot postures and motions that could contribute to a fall. It scans patients with infrared light as they perform a series of motions. A pose detection model analyzes their positions, a company spokesperson told The Batch.
- A balance test measures how much a person sways while standing still.
- A gait test assesses walking speed, the angles of the knees, and length of each step.
- In function tests, the system analyzes various sitting, standing, and walking activities.
- The system compares input from a given patient with norms for their age group, then assigns a fall risk score. It also provides to caregivers recommendations for improving the patient’s mobility.
Behind the news: Automated systems are helping to improve elder care in various ways.
- CarePredict is a wearable device that tracks patient behavior and alerts caregivers if they aren’t eating or sleeping well.
- The People Power Family system uses sensors to monitor seniors living at home for falls, late-night activity, and unexpected comings and goings. A model learns each patient’s habits and sends out warnings when they diverge in alarming ways.
Why it matters: Falls kill thousands of elderly adults each year and injure millions more. Highlighting risk factors could save lives, reduce insurance premiums, and help caregivers use their time more efficiently.
We’re thinking: AI has a clear role to play in caring for a surging elderly population. However, a recent study found that many older people resented and resisted being monitored by electronic systems. Technologists and health care practitioners alike must build such systems with compassion and respect for the people who will use them.
A MESSAGE FROM DEEPLEARNING.AI
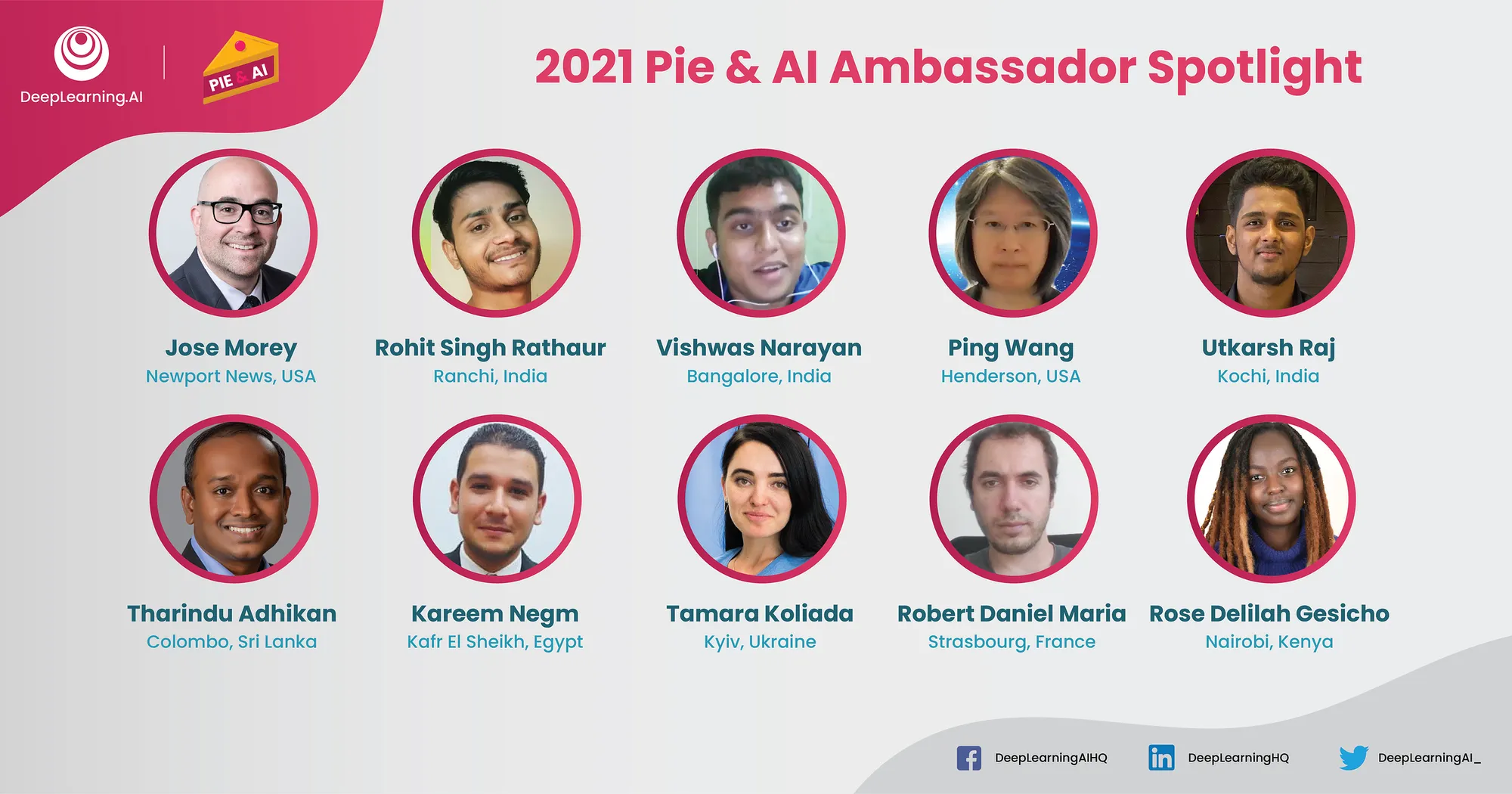
This week, we’re celebrating the achievements of our Pie & AI Event Ambassadors in 2021. Over the last two years, 200+ ambassadors have hosted over 500 Pie & AI meetups in 110 cities across 52 countries. Read their inspiring stories and see what makes them stand out.
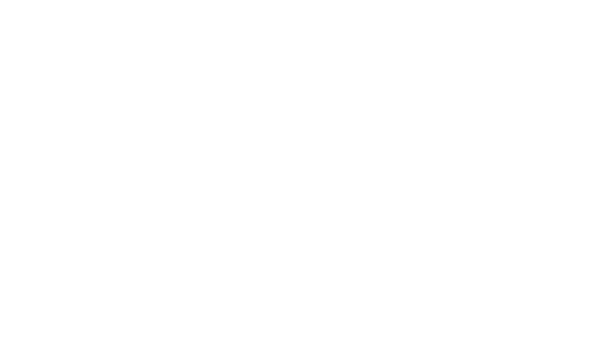
Team Players
Playing a team sport involves a fluid blend of individual and group skills. Researchers integrated both types of action into realistic humanoid agents that play football (known as soccer in the U.S.).
What's new: Siqi Liu, Guy Lever, Zhe Wang, and colleagues at DeepMind developed a method for training simulated football teams that learned to run, pass, defend, and score goals on a physically accurate virtual field. You can see the output here.
Key insight: Football players must control their own muscle motions over time spans measured in milliseconds while collaborating with teammates over greater intervals. By training in stages — starting with lower-level controllers that operate on short time scales for things like running and moving on higher-level controllers that operate on longer time scales for, say, teamwork — agents can learn to move both independently and cooperatively.
How it works: The authors trained 16 agents to compete in two-member teams. An agent could apply torques to its 56 joints; track its own joint angles, positions, and velocities; and observe the positions and velocities of other players and objects on the field. All model architectures were vanilla neural networks.
- In the first stage of training, a model learned motions like running and turning. The authors trained an encoder and decoder via supervised learning to predict an agent's motion, given 105 minutes of motion-capture data from real players in scripted scenes. The encoder learned to convert the agent’s physical state into a representation, while the decoder learned to convert the representation into torques on joints. The same decoder was used in subsequent steps.
- In the second stage, separate encoders learned via reinforcement learning to perform four drills: following a point, following a point while dribbling, kicking a ball to a point on the field, and shooting a goal. Each encoder learned representations of not only the agent’s physical state but also the drill, such as the point to be followed. The decoder determined how the agent should move its joints.
- Four additional encoders learned via supervised learning to re-create the drill model’s representations without access to information about where to run or kick the ball.
- Finally, the agents learned via reinforcement to compete in teams. An encoder learned to combine the drill representations and passed the result to the decoder to determine the agent’s motion. The model received +1 when its team scored a goal and -1 when its team was scored upon. Further rewards encouraged the player closest to the ball to advance it toward the opponents’ goal.
Results: The agents’ skills increased with the number of training episodes. For example, at initialization, when an agent fell, it got up 30 percent of the time. After 375 million training steps in competition, it righted itself 80 percent of the time. Likewise, at initialization, when an agent touched the ball, it executed a pass 0 percent of time. After 80 billion training steps in competition, it passed the ball in 6 percent of touches.
Why it matters: It may take more than one training mode to teach all the skills required to perform a complex task. In this case, the authors combined supervised learning, reinforcement learning, and training in teams.
We’re thinking: How to build agents that operate at both short and long time scales is a longstanding problem in reinforcement learning. The authors solved it by specifying the skills at each time scale manually. The next step is to design agents that can learn that abstraction on their own.
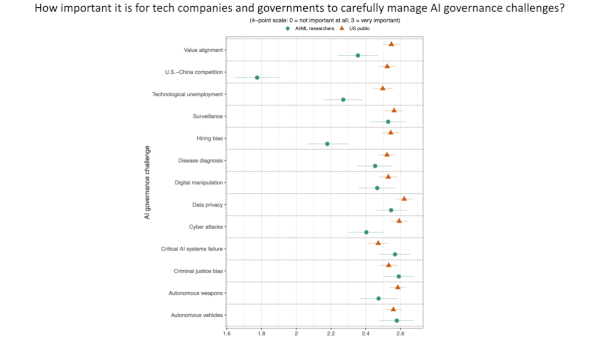
AI Engineers Weigh In on AI Ethics
Machine learning researchers tend to trust international organizations, distrust military forces, and disagree on how much disclosure is necessary when describing new models, a new study found.
What’s new: A survey of accomplished machine learning researchers by Cornell University, University of Oxford, and University of Pennsylvania probed their stances on key ethical issues and compared them with those of the U.S. public.
What they found: The study drew on responses from 534 researchers whose work had been accepted by NeurIPS or ICML. The respondents were 89 percent male and came mostly from Europe, Asia, and North America. The findings include:
- Safety: 68 percent of respondents said the AI community should place a higher priority on safety, defined as systems that are “more robust, more trustworthy, and better at behaving in accordance with the operator’s intentions.”
- Openness: The respondents valued openness in basic descriptions of AI research — to a point. 84 percent believed that new research should include a high-level description of methods, and 74 percent said it should include results. Only 22 percent believed that published research should include a trained model.
- Trust in militaries: Respondents generally supported uses of AI in military logistics. One in five strongly opposed AI for military surveillance. 58 percent were strongly opposed to the development of AI-driven weapons, and 31 percent said they would resign if their job required them to work on such projects.
- Trust in corporations: Among top AI companies, respondents deemed Open AI the most trustworthy followed by Microsoft, DeepMind, and Google. Respondents showed the least trust in Facebook, Alibaba, and Baidu.
Behind the news: Technologists have been nudging the industry towards safe, open, and ethical technology. For example, the Institute for Electrical and Electronics Engineers introduced standards to help its members protect data privacy and address ethical issues. Sometimes engineers take a more direct approach, as when 3,000 Google employees signed a petition that censured their company’s work for the U.S. military, causing it to withdraw from a Defense Department computer vision project.
Why it matters: AI raises a plethora of ethical quandaries, and machine learning engineers are critical stakeholders for addressing them. Machine learning engineers should play a big role in understanding the hazards, developing remedies, and pushing institutions to follow ethical guidelines.
We’re thinking: The machine learning researchers surveyed were markedly more concerned than the U.S. public about competition between the U.S. and China, surveillance, technological unemployment, and bias in hiring. These disagreements suggest an active role for the AI community in navigating the myriad challenges posed by AI.