Dear friends,
In my experience, the most sophisticated decision makers tend to be hypothesis-driven thinkers. They may be engineers solving a technical problem, product designers fulfilling a customer need, or entrepreneurs growing a business. They form a hypothesis about how to reach their goal and then work systematically to either validate or falsify it.
Say you’re tuning a learning algorithm that estimates the health of corn stalks based on input from a tractor-mounted camera. (Many companies are developing products like this to help farmers make decisions about planting, weeding, or harvesting.) If your algorithm is doing poorly, how should you go about improving it?
Some engineers tend to apply a one-size-fits-all rule. Someone who has experience improving algorithms by collecting more data may tend to gather more photos of corn stalks. When that doesn’t work, they may end up trying things more or less at random until they stumble on something that works.
Hypothesis-driven thinkers, on the other hand, have seen learning algorithms perform poorly for many different reasons. Based on that experience, they can make a list of hypotheses about what could be going wrong. Perhaps the algorithm does well in sunlight but performs poorly on overcast days, in which case the best solution indeed may be to collect — or synthesize — more images under cloudy skies. Or perhaps the camera’s lens is obscured by dust, or the hyperparameters are poorly tuned.
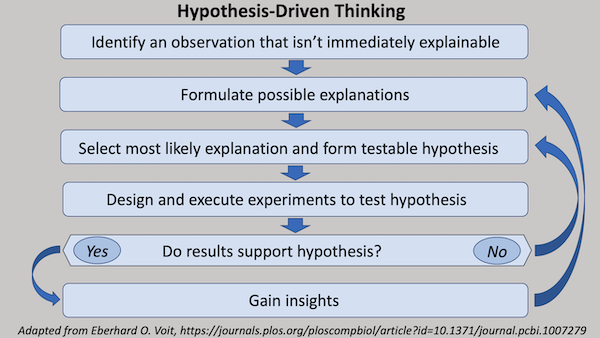
Hypothesis-driven thinkers see a variety of possibilities. They pick the most likely one and carry out error analysis or other tests to falsify or validate it. Then they apply the insights they've gained to devise a solution, choose a new hypothesis, or re-evaluate the range of hypotheses. In this way, they find a good solution efficiently.
How can you gain skill in building hypotheses?
- Seek out stories of how others have built machine learning systems. Learning from friends and colleagues about not only what worked but also what path led there — including wrong turns and ideas considered and rejected — can hone your intuition.
- If you work with other engineers and they advocate a course of action, ask why. Conversely, if you favor a particular approach, share your reasoning and invite them to challenge you. This helps you to (i) gain exposure to more tactics and (ii) understand when various tactics apply.
- Keep taking courses. They can expose you quickly to a wide range of examples.
Hypothesis-driven thinking is helpful not only in developing AI systems, but also in building products and businesses. Perhaps you’ve identified a market need, a concept to fulfill that need, and a sales strategy to get the product into the customers’ hands. Rather than rushing ahead and assuming that everything will work out, you might question key assumptions behind the hypothesis systematically and pinpoint those that are unproven or incorrect. If you discover early on that the concept is flawed (say, because it requires AI technology that hasn’t been invented yet), you’ll have more time to pivot and find an alternative.
Keep learning!
Andrew
News
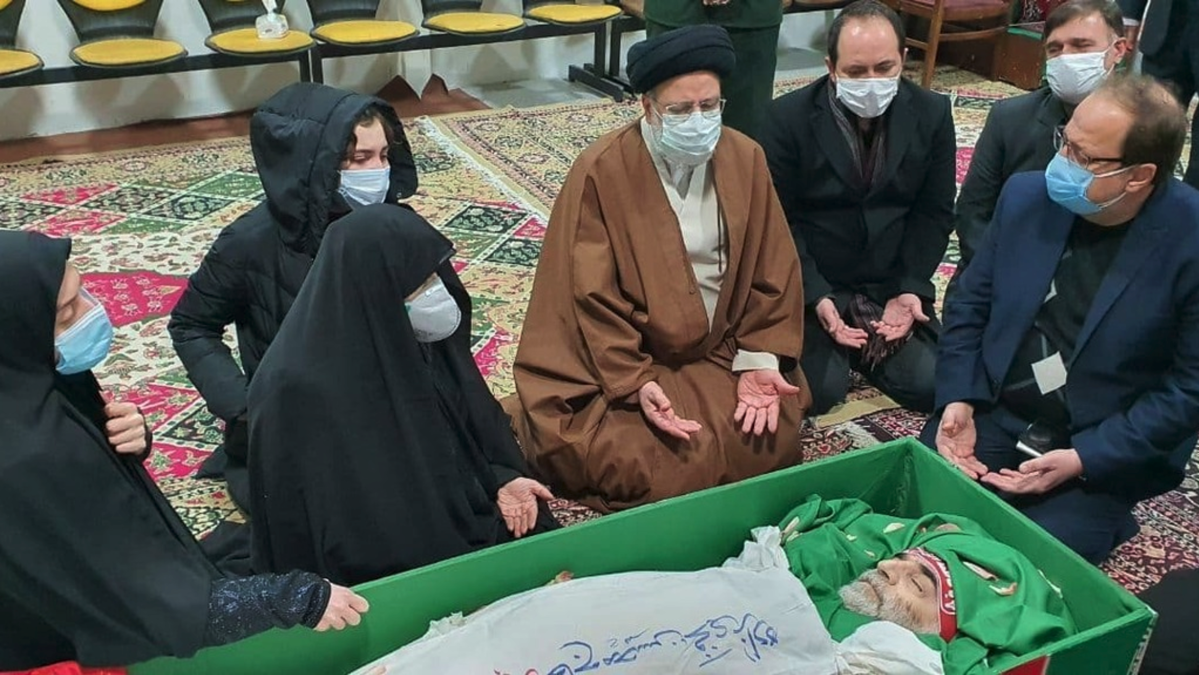
Distance Killing
A remote sniper used an automated system to take out a human target located thousands of miles away.
What happened: The Israeli intelligence agency Mossad used an AI-assisted rifle in the November killing of Iran’s chief nuclear scientist, according to The New York Times.
How it worked: The agency killed Mohsen Fakhrizadeh, whom it considered a key player in Iran’s covert nuclear weapons program, as he was driving near Tehran.
- The Israelis smuggled a machine gun and robotic control system into Iran piece by piece. Agents inside the country assembled the weapon in secret and mounted it on a camera-equipped pickup truck parked near Fakhrizadeh’s home. A separate truck, also outfitted with cameras, alerted the sniper when his car was nearby. The gun’s operator watched the images from an undisclosed location in a different country.
- The agency estimated that it would take 1.6 seconds for video to travel via satellite from the gun truck’s cameras to the sniper and for the sniper's aim and fire orders to reach the gun. It had programmed the system to compensate for the delay as well as the target car’s speed and weapon’s recoil while firing.
- The sniper fired 15 auto-targeted bullets in less than a minute, killing Fakhrizadeh while sparing his wife in the passenger seat.
Behind the news: Scores of military weapon systems around the world use AI to assist in targeting and other functions.
- A Libyan military faction last year deployed autonomous aerial vehicles to attack enemy troops.
- The South Korean company DoDaam developed automated sentry towers that identify patterns of heat and motion caused by people using cameras, thermal imagers, and laser range-finders.
- Milrem Robotics of Estonia sells a ground-based robot that can be outfitted with various remote-controlled weapons including machine guns, missiles, and drones.
Why it matters: Automated weapons have a long history. This AI-targeted shooting, however, opens a new, low-risk avenue for well funded intelligence agencies to kill opponents.
We’re thinking: We find AI-assisted killing deeply disturbing even as we acknowledge that countries need ways to protect themselves. We believe that AI can be a tool for advancing democracy and human rights, and that the AI community should take part in drawing clear boundaries for acceptable machine behavior.
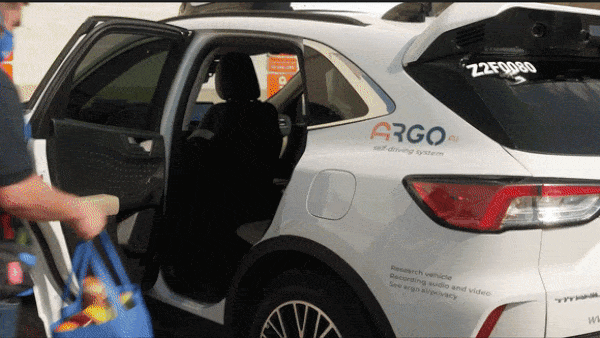
Driverless Delivery in High Gear
Walmart aims to deliver goods via self-driving vehicles this year.
What’s new: The retail giant will test autonomous delivery in three U.S. cities. Cars built by Ford and piloted by Argo AI will ferry merchandise directly to the customer’s front steps.
How it works: The service initially will be limited to parts of Austin, Miami, and Washington, D.C.
- Argo is integrating its cloud-based vehicle routing system with Walmart’s online ordering platform. When a customer places an order, the system will schedule a vehicle to make the delivery.
- The Pittsburgh startup’s self-driving technology relies on radar, cameras, and a proprietary lidar sensor.
Behind the news: Walmart has been testing automated delivery services using technology from Cruise, Gatik, and Waymo. Nuro, which also has partnered with Walmart, focuses on autonomous delivery on the grounds that it lowers requirements for riding comfort and permits slower, and thus safer, driving.
Why it matters: Although self-driving vehicles aren’t ready for widespread use, the partnership between one of the world’s largest retailers and one of the world’s biggest auto makers signals potential for near-term commercial applications.
We’re thinking: Fully self-driving cars likely will reach the market through a vertical niche, which is easier than building vehicles that can handle all circumstances. Some companies focus on trucking, others on local shuttles, still others on transportation within constrained environments such as ports or campuses. Although self-driving has taken longer than expected to come to fruition, we remain optimistic that experiments like these will bear fruit.
A MESSAGE FROM DEEPLEARNING.AI
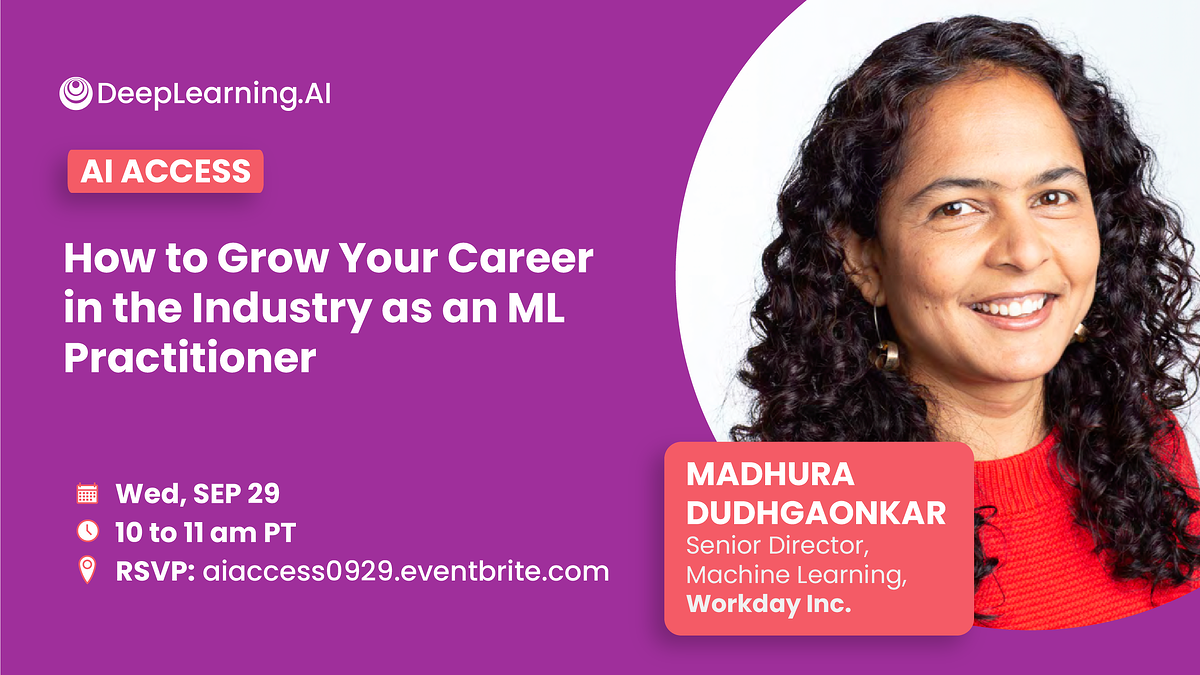
Do you have days when you feel like you’re stalled on the road to becoming a machine learning professional? Join us for a live event on September 29, 2021, as Workday senior director of machine learning Madhura Dudhgaonkar shares real-world insights for crafting your career!
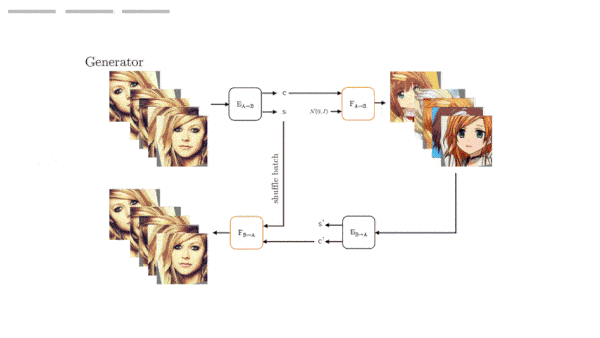
AI With a Sense of Style
The process known as image-to-image style transfer — mapping, say, the character of a painting’s brushstrokes onto a photo — can render inconsistent results. When they apply the styles of different artists to the same target content, they may produce similar-looking pictures. Conversely, when they apply the same style to different targets, such as successive video frames, they may produce images with unrelated shapes and colors. A new approach aims to address these issues.
What’s new: Min Jin Chong and David Forsyth at University of Illinois at Urbana-Champaign proposed GANs N’ Roses, a style transfer system designed to maintain the distinctive qualities of input styles and contents.
Key insight: Earlier style transfer systems falter because they don't clearly differentiate style from content. Style can be defined as whatever doesn’t change when an image undergoes common data-augmentation techniques such as scaling and rotation. Content can be defined as whatever is changed by such operations. A loss function that reflects these principles should produce more consistent results.
How it works: Like other generative adversarial networks, GANs N’ Roses includes a discriminator that tries to distinguish synthetic anime images from actual artworks and a generator that aims to fool the discriminator. The architecture is a StyleGAN2 with a modified version of CycleGAN’s loss function. The authors trained it to transfer anime styles to portrait photos using selfie2anime, a collection of unmatched selfies and anime faces. The authors created batches of seven anime faces and seven augmented versions of a single selfie (flipped, rotated, scaled, and the like).
- The generator used separate encoder-decoder pairs to translate selfies to animes (we’ll call this the selfie-to-anime encoder and decoder) and, during training only, animes to selfies (the anime-to-selfie encoder and decoder).
- For each image in a batch, the selfie-to-anime encoder extracted a style representation (saved for the next step) and a content representation. The selfie-to-anime decoder received the content representation and a random style representation, enabling it to produce a synthetic anime image with the selfie’s content in a random style.
- The anime-to-selfie encoder received the synthetic anime image and extracted a content representation. The anime-to-selfie decoder took the content representation and the selfie style representation generated in the previous step, and synthesized a selfie. In this step, a cycle consistency loss minimized the difference between original selfies and those synthesized from the anime versions; this encouraged the model to maintain the selfie’s content in synthesized anime pictures. A style consistency loss minimized the variance of selfie style representations within a batch; this minimized the effect of the augmentations on style.
- The discriminator received synthetic and actual anime images and classified them as real or not. A diversity loss encouraged a similar standard deviation among all synthetic and all actual images; thus, different style representations would tend to produce distinct styles.
Results: Qualitatively, the system translated different selfies into corresponding anime poses and face sizes, and different styles into a variety of colors, hair styles, and eye sizes. Moreover, without training the networks on video, the authors rendered a series of consecutive video frames. Subjectively, those videos were smooth, while those produced by CouncilGAN’s frames showed inconsistent colors and hairstyles. In quantitative evaluations comparing Frechet Inception Distance (FID), a measure of similarity between real and generated images in which lower is better, GANs N’ Roses achieved 34.4 FID while CouncilGAN achieved 38.1 FID. Comparing Learned Perceptual Image Patch Similarity (LPIPS), a measure of diversity across styles in which higher is better, GANs N’ Roses scored .505 LPIPS while CouncilGAN scored .430 LPIPS.
Why it matters: If style transfer is cool, better style transfer is cooler. The ability to isolate style and content — and thus to change content while keeping style consistent — is a precondition for extending style transfer to video.
We’re thinking: The next frontier: Neural networks that not only know the difference between style and content but also have good taste.
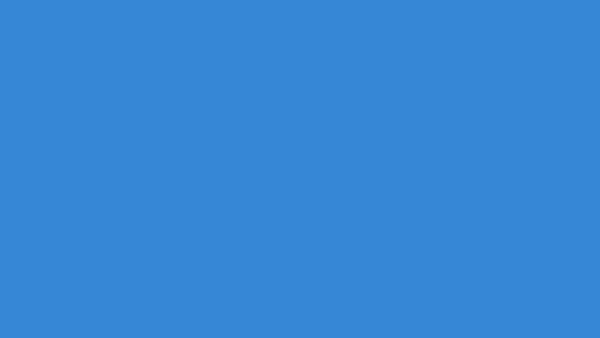
UN Calls Out AI
Human rights officials called for limits on some uses of AI.
What’s new: Michelle Bachelet, the UN High Commissioner for Human Rights, appealed to the organization’s member states to suspend certain systems until safety protocols are established and to ban those she believes may infringe on human rights. Her call coincided with a report from the UN Human Rights Council warning that automated systems for policing, healthcare, and content recommendation threaten rights like privacy, free expression, and freedom of movement and could limit access to health care and education.
Clear and present dangers: The report highlights documented hazards such as algorithmic bias, intrusive surveillance, and lack of transparency in AI. It argues that governments and businesses often deploy AI products without determining whether they pose risks to human rights. The authors call for:
- A ban on systems that pose acute risks to human rights such as real-time biometric identification.
- A moratorium on algorithms that determine a person’s eligibility for health care until regulations are in place.
- Guidelines, independent oversight, and laws that protect data privacy.
- Mechanisms such as explainable AI that would help rectify AI-enabled abuses of human rights.
- Ongoing monitoring of AI systems for potential threats to human rights.
Behind the news: The UN report is the latest high-level call for rules that rein in AI’s potential for harm.
- In April, the European Union proposed a similar system of bans and moratoriums.
- Another recent report highlights how algorithmic recommendation systems spread disinformation on social media platforms.
Why it matters: The UN can’t force anyone to heed its recommendations. But strong statements like Bachelet’s backed by well reported data can bring attention and public pressure to bear on the intersection of AI and human rights.
We’re thinking: Voluntary restrictions and finger-wagging reports are no substitute for concrete legal limits. Meanwhile, the AI community — each and every one of us — can push toward more beneficial uses.