Dear friends,
Bias in AI is a serious problem. For example, if a judge who’s deciding how to sentence a defendant relies on an AI system that routinely estimates a higher risk that offenders of a particular race will reoffend, that’s a terrible thing. As we work to reduce bias in AI models, though, it’s also worth exploring a different issue: inconsistency. Specifically, let’s consider how inconsistent human decisions are, and how AI can reduce that inconsistency.
If a human judge, given two defendants who committed the same crime under identical circumstances, sentences one to three years in prison and the other to 30 days, we would consider this inconsistency blatantly unfair. Yet, as Daniel Kahneman and his co-authors document in their book, Noise: A Flaw in Human Judgment, human decision-making is extremely inconsistent (or noisy).
One study found that judges systematically sentenced defendants more harshly if the local football team had suffered an upset loss (which presumably made the judge cranky). Judges are only human, and if they’re swayed by football outcomes, imagine how many other irrelevant factors may influence their decisions!
Many human decisions rest on complex criteria, and humans don’t always define their criteria before weighing them. For example:
- In medicine, I’ve seen individual doctors make highly inconsistent diagnoses given the same input. Working on a project with a doctor whom I’ll call Alice, we measured the “inter-Alice agreement score,” which was loosely a measure of how much her diagnoses differed between morning and afternoon. (For the record, Alice is a brilliant doctor and wonderful collaborator. This score measured the inherent ambiguity of the task more than it measured her competence.)
- In manufacturing, I’ve seen skilled inspectors make very different decisions about whether or not parts with similar flaws were defective.
- In online retailing, I’ve seen human annotators make inconsistent decisions about how to tag or categorize products. (Should a fun gadget go under electronics or entertainment?)
In contrast, given the same input, a trained neural network will produce the same output every time. Given similar inputs, a trained model will also typically output similar results. Automated software tends to be highly consistent. This is one of automation’s huge advantages: Algorithms make decisions much more consistently than humans. To my mind, they offer a way to give patients more consistent and fair treatment options, make manufacturing more efficient, make retail product catalogs less confusing to shoppers, and so on.
In conversations about whether and how to build an AI system, it’s important to address how to ensure that the system doesn’t have significant bias as well as how to benchmark its bias against human bias. If you’re trying to get an AI project approved, you may find it useful raise the issue of consistency as well. Measuring the consistency of your algorithm relative to humans who make the same decision can add weight to arguments in favor of investing in an automated system.
Keep learning!
Andrew
News
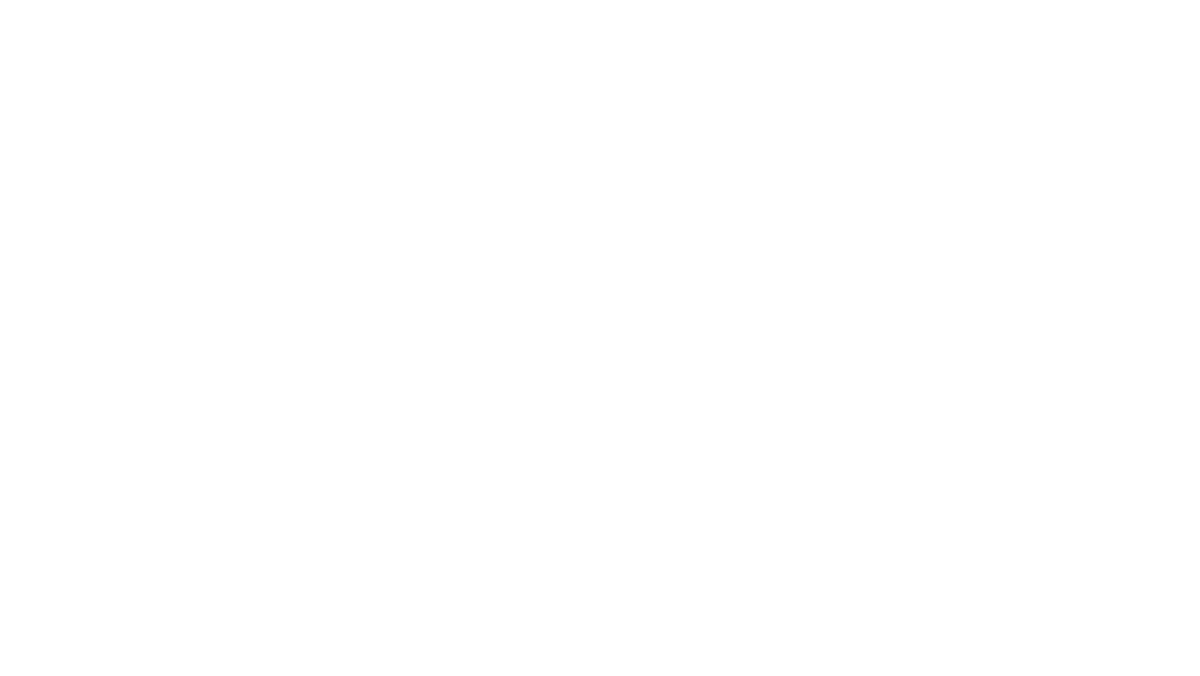
AI Jobs Grow in Pharma
New data suggests the drug industry is hooked on AI.
What’s new: Pharmaceutical companies in several countries are hiring machine learning engineers at increasing rates, industry news publication Pharmaceutical Technology reported. Most job openings are posted in the United States, though some countries in Europe and Asia are gaining ground.
How it works: The publication analyzed data from GlobalData’s paywalled database, which tracks job listings in a variety of industries and analyzes the text to group them into categories.
- 26.4 percent of pharmaceutical companies in the database posted at least one machine learning opening in June 2022, an increase of 2.3 percent over the previous year. Of all the pharma industry jobs posted in June, 1.2 percent were related to machine learning.
- 61 percent of machine learning jobs advertised by pharma companies globally in the three months ending in May were located in the U.S. The Boston, Massachusetts, metropolitan area saw the largest cluster of such jobs followed by the San Francisco Bay Area and San Diego, California.
- The top three European countries — Belgium, France, and the United Kingdom — each represented less than 6 percent of machine learning jobs advertised during the three months ending in May.
- The Asia-Pacific region’s total share decreased 1.9 points in the same time period. Job losses were not consistent across the region, however, China’s share declined from 5 percent to 2 percent, while India’s rose from 5 to 6 percent.
Behind the news: In a recent report, GlobalData estimated that the pharmaceutical industry will spend over $3 billion on AI by 2025, driven largely by applications in drug discovery. The trend has also prompted major pharma companies including Astra-Zeneca, Pfizer, and Sanofi to acquire, invest in, or partner with startups. GlobalData counted 67 such partnerships in 2021, up from 23 in 2018.
Why it matters: Bringing a new drug to market can take decades and cost billions of dollars. AI can cut time and costs in myriad ways, for instance by recognizing viable molecules without lab experimentation, identifying patients who might benefit from a drug, and predicting how patients might respond to them.
We’re thinking: Given the economic value of online advertising and product recommendations, many machine learning engineers — and an entire genre of machine learning approaches — are devoted to optimizing their results. Given the value of pharmaceuticals, we have no doubt that machine learning has immense potential in that domain as well. Similarly, a large body of specialized machine learning techniques is waiting to be developed for many industries.
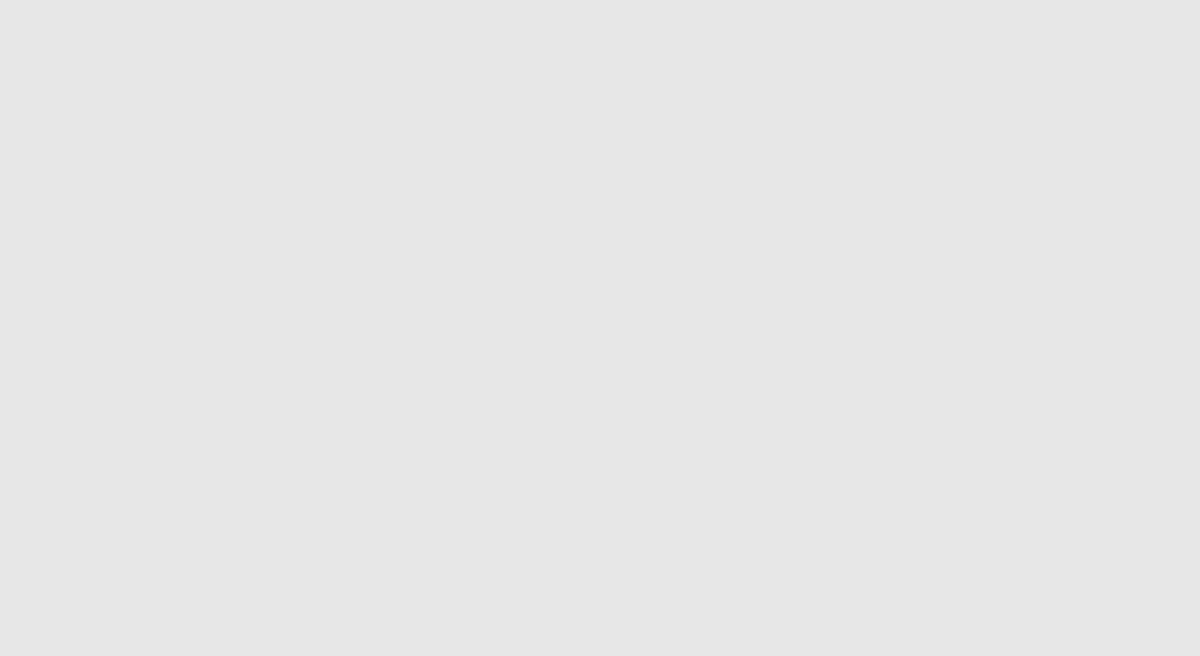
Self-Driving Safety Check
Data from vehicle makers sheds light — though not much — on the safety of current autonomous and semi-autonomous vehicles.
What’s new: The United States National Highway Traffic Safety Administration (NHTSA) detailed collisions over a 12-month period that involved cars that drive themselves or automate some driving tasks. This is the first edition of what promises to be an annual report.
Going driverless: Fully automated driving systems (often called ADS) that operate without a driver behind the wheel aren’t yet widely available. For the most part, they're being tested in a small number of designated areas. Manufacturers must report incidents that occurred within 30 seconds of engaging an ADS or resulted in property damage or personal injury.
- Fully autonomous vehicles were involved in 130 reported crashes.
- Collisions resulted in minor, moderate, or serious injuries in 12 percent of incidents. No fatalities were reported.
- Most incidents involved other vehicles. A few involved non-motorists or fixed objects.
- Waymo’s autonomous taxis reported the most incidents (62). Transdev Alternative Services, which makes autonomous buses, trolleys, and other mass-transit vehicles, reported the second-highest number (34). Cruise, which makes autonomous driving systems for Chevrolet, came in third (23).
Extra hands on the wheel: Semi-autonomous vehicles equipped with automated driving assistance systems (known as ADAS) require a flesh-and-blood driver but can steer, accelerate, and decelerate on their own. Manufacturers must report crashes that caused an airbag to inflate, required a car to be towed, or sent someone to a hospital.
- Semi-autonomous vehicles were involved in 392 reported collisions.
- Whether injuries occurred and their severity is unknown in 75 percent of cases. Of the remaining 25 percent, injuries were reported in more than half, including six fatalities.
- The object struck is unknown in 37 percent of cases. The remaining cases divided roughly evenly between other vehicles and poles, trees, and other fixed objects.
- Tesla vehicles accounted for the most crashes (273). Honda’s semiautonomous vehicles accounted for the second highest number (90). No other manufacturer reported more than 10 incidents.
Yes, but: The report doesn’t tally miles driven by fully autonomous, semi-autonomous, and conventional vehicles, nor at what speeds they traveled. Without that information, there's no way to derive a collision rate per mile or evaluate the severity of injuries at various speeds. Moreover, the report includes only crashes known to manufacturers. It may have missed those that weren’t reported to law enforcement or through consumer complaints. (This may explain the high numbers for Tesla, which harvests data directly from its vehicles.)
Why it matters: Vehicle safety is a life-and-death matter. Fully autonomous cars may not reach the market for years, but a degree of automated driving is commonplace: Vehicles that can steer, accelerate, and decelerate temporarily with a human present accounted for 30 percent of new car sales in the U.S. during the fourth quarter of 2020.
We’re thinking: Initial efforts to collect data, however incomplete, often lead to better data in the future. We hope that NHTSA improves these reports in the coming years by adding the total miles, as well as subdivisions according to in-town and on-highway speed ranges, driven by each of the two automation classes as well as unassisted humans.
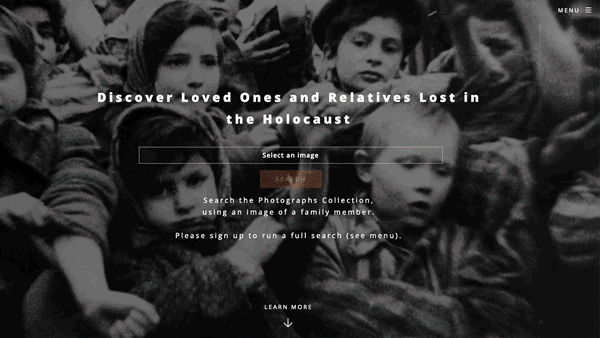
Identifying Faces of History
A face recognition system is helping identify victims of the Holocaust.
What’s new: From Numbers to Names matches individuals to faces in publicly available images related to the genocide of European Jews between 1941 and 1945.
How it works: Built by Google software engineer Daniel Patt and financier Jed Limmer, the site matches images uploaded by users with faces from the United States Holocaust Memorial Museum’s photo collection.
- Users with a free account can upload an image to the website. It works best with pre-1960s grayscale photographs that feature a single face, Patt told the Times of Israel.
- Patt trained the system to calculate the similarity between uploaded photographs and the museum’s database of roughly 177,000 faces in around 35,000 photos. The system returns the 10 faces that have the highest similarity.
- Patt, who is the descendant of Holocaust survivors, is working to add more photos from the Holocaust and pre-Holocaust eras. The project is also analyzing footage from the museum's 1,265-hour film and video archive.
Behind the news: Deep learning plays a growing role in understanding history.
- DeepMind researchers recently built a neural network called Ithaca that’s designed to help historians read ancient Greek inscriptions by enhancing photos of them, dating them, and identifying where they were produced.
- Anthropologists at Northern Arizona University trained neural networks to classify images of centuries-old pottery fragments according to the Native American cultures that archaeologists believe created them.
- Transkribus offers several tools to recognize and transcribe historical handwriting. Users can train their own model using 50 to 100 pages of transcribed writing.
- Image analysis guided by AI revealed a 2,000-year-old picture dug into the Peruvian desert. Researchers analyzing aerial imagery shot over Peru found a pattern that looks like a three-horned humanoid holding a staff.
Why it matters: Roughly 11 million people were systematically murdered by the government of Nazi Germany for their ethnicity, religion, political beliefs, or sexual orientation. Identifying the victims doesn’t erase the crime of their deaths, but it can help bring closure to their relatives and strengthen our resolve to make sure nothing similar ever happens again.
We’re thinking: While lives lost to war have decreased significantly over the decades, humanity has yet to progress beyond senseless killing. Learning about the atrocities of the past helps us view current events — such as the Russia-Ukraine war — with a critical eye and stand firm for human rights.
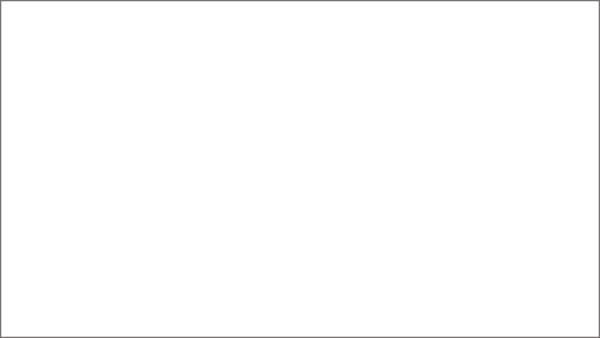
Protein Families Deciphered
Models like AlphaFold have made great strides in finding protein shapes, which determine their biological functions. New work separated proteins into functional families without considering their shapes.
What’s new: A team led by Maxwell L. Bileschi classified protein families using a model (called ProtCNN) and a process (called ProtREP) that used that model’s representations to address families that included fewer than 10 annotated examples. The project was a collaboration between Google, BigHat Biosciences, Cambridge University, European Molecular Biology Laboratory, Francis Crick Institute, and MIT.
Key insight: A neural network that has been trained on an existing database of proteins and their families can learn to assign a protein to a family directly. However, some families offer too few labeled examples to learn from. In such cases, an average representation of a given family’s members can provide a standard of comparison to determine whether other proteins fall into that family.
How it works: The authors trained a ResNet on a database of nearly 137 million proteins and nearly 18,000 family classifications.
- The authors trained the model to classify proteins in roughly 13,000 families that each contained 10 or more examples.
- Taking representations from the second-to-last layer, they averaged the representations of proteins in each family.
- At inference, they compared an input protein’s representation with each family’s average representation. They chose the family whose average matched most closely according to cosine similarity.
- In addition, they built an ensemble of 19 trained ResNets that determined classifications by majority vote.
Results: The ensemble model achieved accuracy of 99.8 percent, higher than both comparing representations (99.2 percent) and the popular method known as BLASTp (98.3 percent). When classifying members of low-resource families, the representation-comparison method achieved 85.1 percent accuracy. Applying the ensemble to unlabeled proteins increased the number of labeled proteins in the database by nearly 10 percent — more than the number of annotations added to the database over the past decade.
Why it matters: New problems don’t always require new methods. Many unsolved problems — in biology and beyond — may yield to well established machine learning approaches such as few-shot learning techniques.
We’re thinking: Young people, especially, ought to appreciate this work. After all, it’s pro-teen.