Dear friends,
I’ve devoted several recent letters to building a career in AI. In this one, I’d like to discuss some fine points of finding a job.
The typical job search follows a fairly predictable path.
- Research roles and companies online or by talking to friends.
- Optionally, arrange informal informational interviews with people in companies that appeal to you.
- Either apply directly or, if you can, get a referral from someone on the inside.
- Interview with companies that give you an invitation.
- Receive one or more offers and pick one. Or, if you don’t receive an offer, ask for feedback from the interviewers, the human resources staff, online discussion boards, or anyone in your network who can help you plot your next move.
Although the process may be familiar, every job search is different. Here are some tips to increase the odds you’ll find a position that supports your thriving and enables you to keep growing.
Pay attention to the fundamentals. A compelling resume, portfolio of technical projects, and a strong interview performance will unlock doors. Even if you have a referral from someone in a company, a resume and portfolio will be your first contact with many people who don’t already know about you. Update your resume and make sure it clearly presents your education and experience relevant to the role you want. Customize your communications with each company to explain why you’re a good fit. Before an interview, ask the recruiter what to expect. Take time to review and practice answers to common interview questions, brush up key skills, and study technical materials to make sure they are fresh in your mind. Afterward, take notes to help you remember what was said.
Proceed respectfully and responsibly. Approach interviews and offer negotiations with a win-win mindset. Outrage spreads faster than reasonableness on social media, so a story about how an employer underpaid someone gets amplified, whereas stories about how an employer treated someone fairly do not. The vast majority of employers are ethical and fair, so don’t let stories about the small fraction of mistreated individuals sway your approach. If you’re leaving a job, exit gracefully. Give your employer ample notice, give your full effort through your last hour on the job, transition unfinished business as best you can, and leave in a way that honors the responsibilities you were entrusted with.
Choose who to work with. It’s tempting to take a position because of the projects you’ll work on. But the teammates you’ll work with are at least equally important. We’re influenced by people around us, so your colleagues will make a big difference. For example, if your friends smoke, the odds rise that you, too, will smoke. I don’t know of a study that shows this, but I’m pretty sure that if most of your colleagues work hard, learn continuously, and build AI to benefit all people, you’re likely to do the same. (By the way, some large companies won’t tell you who your teammates will be until you’ve accepted an offer. In this case, be persistent and keep pushing to identify and speak with potential teammates. Strict policies may make it impossible to accommodate you, but in my mind, that increases the risk of accepting the offer, as it increases the odds you’ll end up with a manager or teammates who aren’t a good fit.)
Get help from your community. Most of us go job hunting only a small number of times in our careers, so few of us get much practice at doing it well. Collectively, though, people in your immediate community probably have a lot of experience. Don’t be shy about calling on them. Friends and associates can provide advice, share inside knowledge, and refer you to others who may help. I got a lot of help from supportive friends and mentors when I applied for my first faculty position, and many of the tips they gave me were very helpful.
I know that the job search process can be intimidating. Instead of viewing it as a great leap, consider an incremental approach. Start by identifying possible roles and conducting a handful of informational interviews. If these conversations tell you that you have more learning to do before you’re ready to apply, that’s great! At least you have a clear path forward. The most important part of any journey is to take the first step, and that step can be a small one.
Keep learning!
Andrew
DeepLearning.AI Exclusive
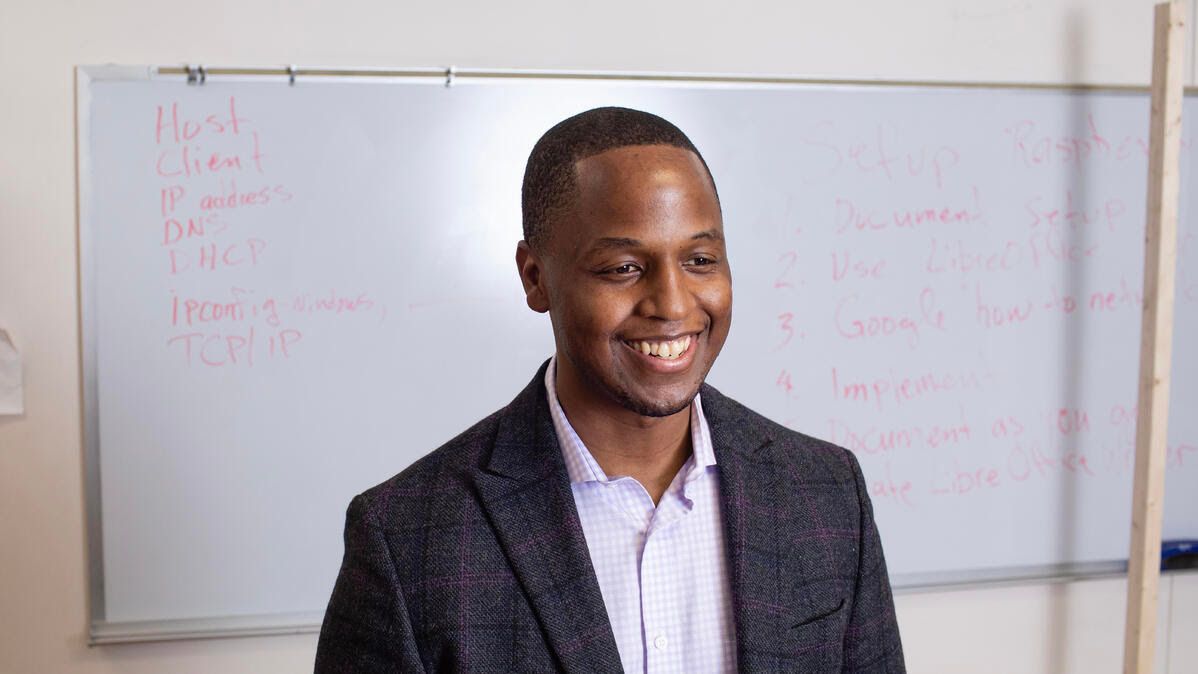
Working AI: From Spy craft to Startups
Dr. Benjamin Harvey recently raised $6 million for his company. An engineer at heart, he has used AI to measure pollution, predict Covid risk, and analyze the Edward Snowden leaks for the NSA. He spoke to us about turning every job into a passion project. Read his story here.
News
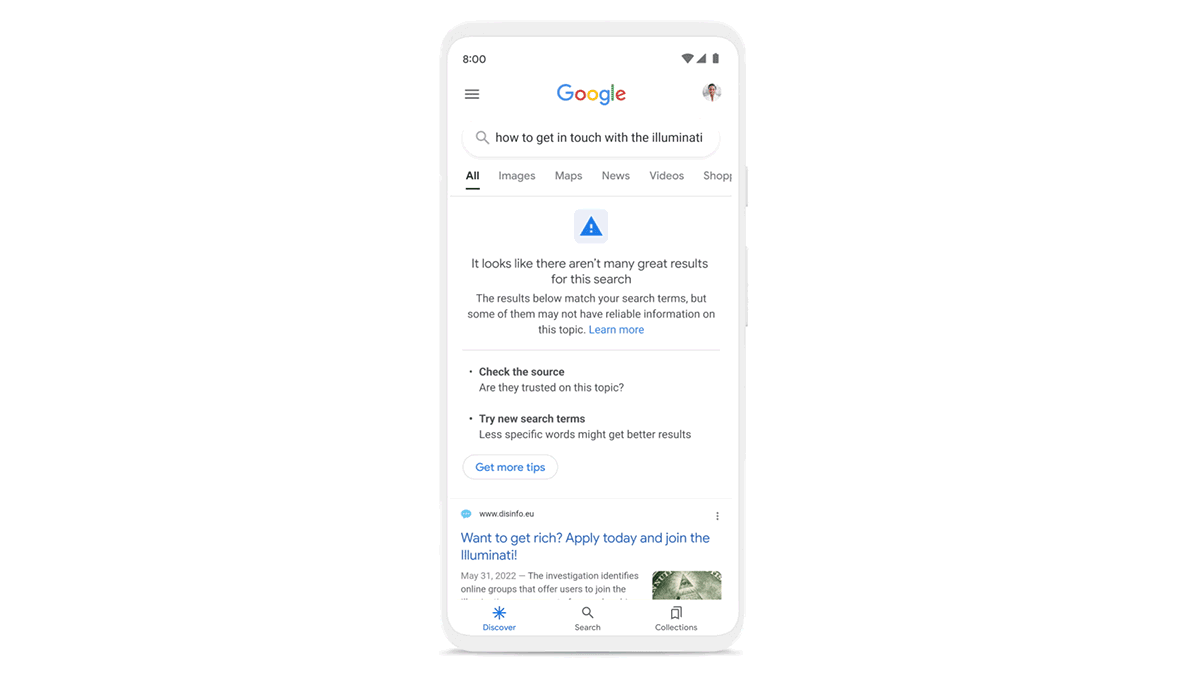
Misinformation Recognition
Google updated a key model behind the algorithm that ranks its search results to respond to the flood of misinformation on the web.
What’s new: The search giant aims to minimize the prominence of falsehoods in the information it presents near the top of search results, which it calls snippets.
How it works: Google revised its Multitask Unified Model to verify the accuracy of snippets.
- The model evaluates how well the top results agree. It can compare pages on a given topic even if they use different phrases or examples.
- If the model doesn’t have high confidence in available sources, instead of a snippet, it generates an advisory such as, “It looks like there aren’t many great results for this search.”
- The model also recognizes misleading questions such as, “When did Snoopy assassinate Abraham Lincoln?” The update cuts inappropriate snippets in response to such queries by 40 percent.
Behind the news: Google isn’t the only major website to task AI with filtering the torrent of disinformation.
- Facebook uses multimodal learning to detect misinformation related to COVID-19.
- In 2020, YouTube deployed a classifier that downgraded recommendations for videos that contain conspiracy theories and anti-scientific misinformation.
Why it matters: Human fact-checkers can’t keep up with the rising tide of misinformation. AI has an imperfect record of moderating online content. For instance, Facebook faces allegations that its algorithms suppress ads aimed at people with disabilities and overlook incitements to violence. But even incremental improvements are worthwhile in the face of anti-vaccine panics, denial of climate change, and calls for genocide.
We’re thinking: AI is an important tool in keeping web searches honest, but it’s not yet ready to do the job alone. Just as autonomous taxi companies often employ human safety drivers to oversee their vehicles, automated content moderation systems benefit from humans in the loop.
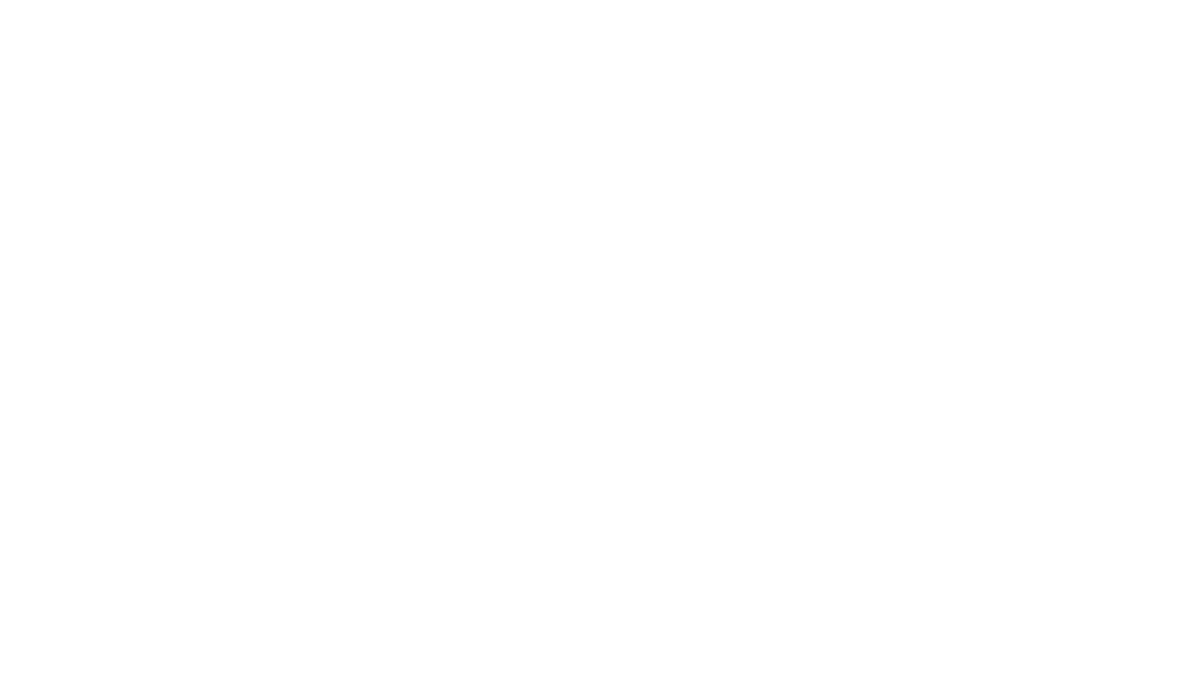
China’s AI ROI
China’s investment in AI business, infrastructure, and research could pay off big over the next decade.
What’s new: AI is expected to add $600 billion to the Chinese economy by 2030, according to an analysis by consulting firm McKinsey.
Market-ready technologies: The researchers interviewed more than 50 experts and analyzed market data collected between October 2021 and November 2021. They looked for market-ready AI technologies (as opposed to those that are either early-stage or mature) with the highest potential for impact in the coming decade. Conclusion: Transportation, manufacturing, enterprise software development, and healthcare stand to add huge value to the nation’s economy.
- Transportation, which encompasses the automotive and logistics applications, is expected to constitute 64 percent of the total contribution. Autonomous vehicles alone would contribute $335 billion including sales and savings. For instance, autonomous vehicles are expected to cause fewer accidents, which will lead to savings on ambulance rides and hospital stays. AI is also expected to help fleet managers stay on top of maintenance and plan more efficient paths through China’s network of roads, rails, waterways, and air routes.
- Manufacturing is expected to make up 19 percent of the total. The majority ($100 billion) would come from innovations in efficiency and productivity like assembly-line robots or wearables that monitor workers. The rest ($15 billion) would reflect AI-enabled improvements in design processes.
- The report forecasts that around 13 percent would come from enterprise software. Just over half of that ($45 billion) would be generated by cloud and AI tools. The remainder ($35 billion) would arise from software as a service.
- AI’s potential to aid with drug discovery, clinical trials, patient care, and other medical applications is expected to comprise the final four percent, amounting to around $25 billion.
The big picture: In 2017, China announced its aim to become “the world's primary AI innovation center” by 2030. Since then, the country has pushed to accelerate AI research, development, and product development. The latest AI Index estimated that China accounted for one-fifth of all private investment in AI.
Yes, but: Not everything is rosy in China’s tech sector. Venture capital investment in the first four months of 2022 was 43.5 percent lower than the same period in 2021 — a decline nearly four times more severe than the global average.
Why it matters: China’s contribution to AI has been impressive, but the outcomes outlined in the report are not foregone. To cash in on AI’s potential, China must put more effort into acquiring and using high-quality data, collaborating with other nations, protecting intellectual property, and training skilled workers, the report says.
We’re thinking: Many commentators view AI development as a competition among global superpowers. Rising tensions between the U.S. and China tend to reinforce this view. Yet every nation’s AI effort relies on numerous individual developers. This global community can play an important role in steering AI in directions that benefit all people as well as their home countries.
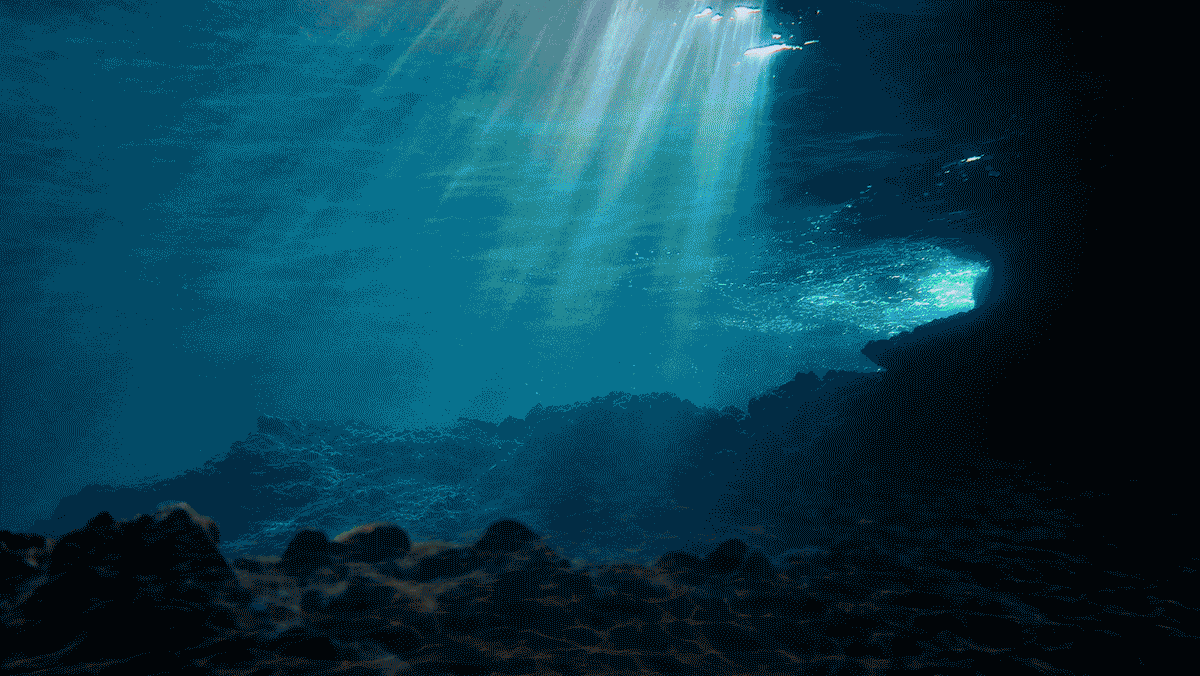
Neural Nets Catch Fresher Fish
A robot deckhand aims to help fishing boats keep their haul fresh all the way to your table.
What’s new: Shinkei Systems developed a machine that uses computer vision to slaughter fish in a way that maximizes their shelf life and flavor, TechCrunch reported.
How it works: The refrigerator-sized system, which is designed to withstand heavy seas, attaches to a boat’s deck. Fishermen empty their nets into a hopper that passes individual fish through the machine one by one. Inside, computer vision guides tools to pierce the animal’s brain, sever its spine, drain its blood, and deposit it into an ice bath. The process takes between 10 to 15 seconds per fish.
- The system identifies each fish’s species and shape, then uses this data to pinpoint where its vital organs are located. Currently it recognizes a limited number of Northern Atlantic species including striped bass, steelhead trout, and black sea bass.
- The company developed the system in partnership with fishermen and leases it to boats in New England on a profit-sharing basis. It has also partnered with several New York restaurants.
Behind the news: The process is modeled on a manual technique called ike jime, which typically requires a skilled practitioner, making it difficult to industrialize. Ike jime is increasingly popular among upscale seafood restaurants both within and outside Japan, where it was developed.
Why it matters: The fast pace aboard fishing boats leaves little time for processing the catch, so most fish are left to suffocate to death, which can take minutes to hours. This isn’t just inhumane, it results in meat that’s bruised by flopping and tainted by stress-induced hormones, leading to shorter shelf life and less appetizing flavor. This system could give fishing operations an efficient way to sell their catches more profitably while dispatching fish more humanely.
We’re thinking: Giving such a delicate task to a robot may seem fishy, but this application seems sure to scale.
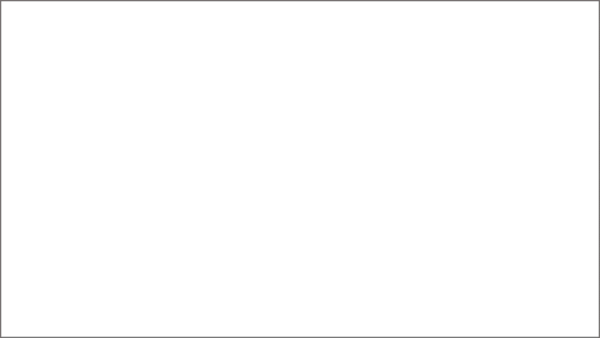
Object-Detection Transformers Simplified
Vision transformers need architecture modifications and retraining from scratch to be used for object detection — or so most researchers thought. New work used vision transformers for object detection without the usual redesign and training.
What’s new: Yanghao Li and colleagues at Facebook proposed ViTDet, which adds an object detector to a plain pretrained transformer.
Key insight: Vision transformers (ViTs) have rivaled convolutional neural nets (CNNs) in many vision tasks — but not object detection. That’s because a CNN’s hierarchical architecture, in which different-sized layers produce representations at different scales of an image, helps to spot objects of any size. Consequently, copying this architecture is a natural choice for transformers for vision tasks, and many ViT variations for object detection feature a hierarchical implementation (known as a backbone that supports a detection-specific head/neck). A simpler solution, though, is to add hierarchical layers to the end of a vanilla ViT backbone. This avoids the need to redesign the network, and it enables object detection models to benefit from pretrained ViTs that weren’t developed with that task in mind.
How it works: ViTDet combines a ViT pretrained on ImageNet, which produces a representation of an input image, with Mask R-CNN’s prediction layers, an established component for object detection and image segmentation. The authors fine-tuned the system for those tasks on an augmented version of COCO. They made the following alterations prior to fine-tuning:
- To help the system recognize objects of different scales in the input image, they applied convolutions and deconvolutions to ViT’s representation, producing representations at four scales. For each representation, the Mask R-CNN layers computed object labels, bounding boxes, and segmentation masks.
- To enable the self-attention mechanism to process higher-resolution input, they split its input into non-overlapping windows (the size of the normal input during pretraining) and limited self-attention to occur within those windows. To enable information to propagate across the windows, they added four convolutional layers to ViT. To avoid the need to retrain ViT from scratch, they initialized the convolutional layers to pass the representation through the layer without modification.
- They augmented the fine-tuning set via large-scale jittering augmentation. This augmentation helps a model learn how objects look at different scales by shrinking images by a random factor and placing them at the top-left corner of an upscaled 1024x1024-pixel canvas.
Results: A ViTDet based on ViT-Huge performed bounding-box detection with 61.3 average precision (a measure of how many objects were correctly identified in their correct location, higher is better) and instance segmentation with 53.1 average precision. SwinV2-L, based on a transformer with a hierarchical architecture, performed bounding-box detection with 60.2 average precision and instance segmentation with 52.1 average precision.
Why it matters: Decoupling the vision model’s design and training from the object-detection stage is bound to accelerate progress on transformer-based object detection systems. If any pretrained transformer can be used for object detection directly off the shelf, then any improvement in pretrained transformers will yield better representations for object detection.
We’re thinking: This work opens opportunities to improve all manner of object detection and segmentation subtasks.