Dear friends,
A few weeks ago, the White House required that research papers funded by the U.S. government be available online promptly and freely by the end of 2025. Data that underlies those publications must also be made available.
I’m thrilled! Paywalled journals that block free access to scientific research are the bane of the academic community.
The AI world is fortunate to have shifted years ago to free online distribution of research papers, primarily through the arXiv site. I have no doubt that this has contributed to the rapid rise of AI and am confident that, thanks to the new U.S. policy, promoting a similar shift in other disciplines will accelerate global scientific progress.
In the year 2000 — before modern deep learning, and when dinosaurs still roamed the planet — AI researchers were up in arms against paywalled journals. Machine Learning Journal, a prominent journal of the time, refused to open up access. With widespread support from the AI community, MIT computer scientist Leslie Kaelbling started the free Journal of Machine Learning Research, and many researchers promptly began publishing there instead. This move led to the rapid decline of Machine Learning Journal. The Journal of Machine Learning Research remains a respected institution today, edited by David Blei and Francis Bach (both of who are my former officemates at UC Berkeley).
Before the modern internet, journal publishers played an important role by printing and disseminating hard copies of papers. It was only fair that they could charge fees to recoup their costs and make a modest profit. But in today’s research environment, for-profit journals rely mainly on academics to review papers for free, and they harvest the journals’ reputations (as reflected in metrics such as impact factor) to extract a profit.
Today, there are peer-reviewed journal papers, peer-reviewed conference papers, and non-peer-reviewed papers posted online directly by the authors. Journal articles tend to be longer and undergo peer review and careful revisions. In contrast, conference papers (such as NeurIPS, ICML and ICLR articles) tend to be shorter and less carefully edited, and thus they can be published more quickly. And papers published on arXiv aren’t peer reviewed, so they can be published and reach interested readers immediately.
The benefits of rapid publication and distribution have caused a lot of the action to shift away from journals and toward conferences and arXiv. While the volume of research is overwhelming (that’s why The Batch tries to summarize the AI research that matters), the velocity at which ideas circulate has contributed to AI’s rise.
By the time the new White House guidance takes effect, a quarter century will have passed since machine learning researchers took a key step toward unlocking journal access. When I apply AI to healthcare, climate change, and other topics, I occasionally bump into an annoyingly paywalled article from these other disciplines. I look forward to seeing these walls come down.
Don’t underestimate the impact of freeing up knowledge. I wish all these changes had taken place a quarter century ago, but I’m glad we’re getting there and look forward to the acceleration of research in all disciplines!
Keep learning!
Andrew
News
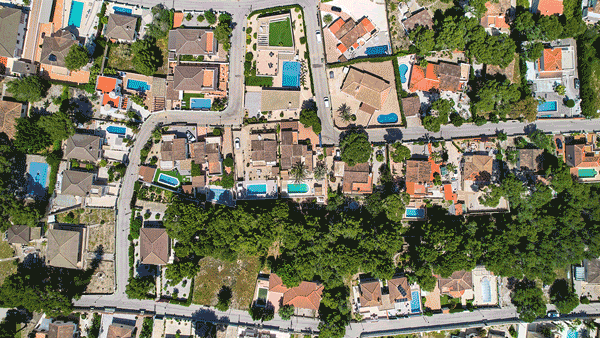
Spotting Tax Cheats From Overhead
Tax dodgers can’t hide from AI — especially those who like to swim.
What’s new: French tax authorities, which tax swimming pools according to their size because they increase a home’s property value, netted nearly €10 million using an automated system to identify unregistered pools, Le Parisien reported.
Diving in: Developed by Google and Paris-based consultancy Capgemini, the system spots pools in a public database of aerial images. It then cross-checks them with land-registry data to determine whether they’re registered. France plans to roll it out nationwide this month.
- In trials across nine French regions since October 2021, the system identified 20,356 suspected undeclared swimming pools. Of taxpayers whose pools were flagged, 94 percent did have an unregistered pool.
- Officials plan to expand the system to identify undeclared improvements like gazebos and verandas that can raise a home’s property taxes.
- They believe that the extended system will capture as much as €40 million in 2023.
Beneath the surface: At least 17 other European Union tax-collection agencies use AI for tasks that include identifying who should be audited, scraping taxpayer data from ecommerce sites, and powering chatbots that help taxpayers file. Last year, U.S. tax authorities implemented technology from Palantir that identifies fraud by analyzing tax returns, bank statements, property records, and social media activity.
Why it matters: As AI analyzes every nook and cranny of an individual’s data trail, reluctant taxpayers will find it harder to avoid paying up.
We’re thinking: There’s irony in a tech behemoth that’s known for aggressive tax-avoidance strategies helping a government collect tax revenue.
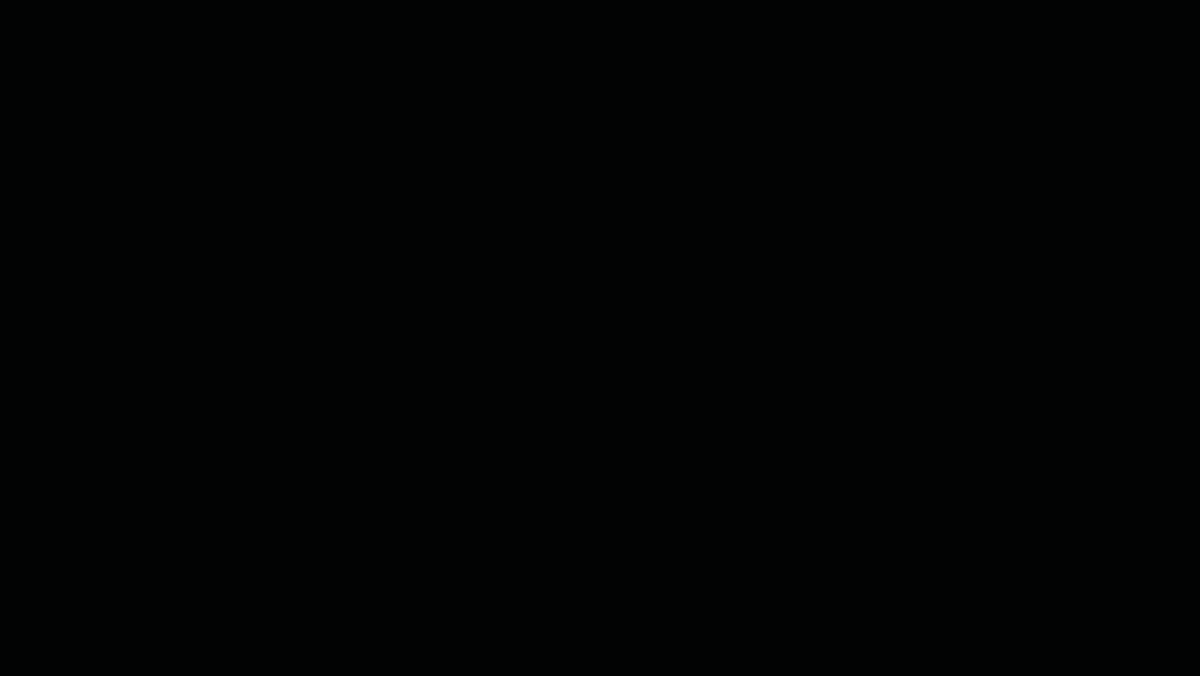
The Geopolitics of GPUs
The U.S. government blocked U.S. makers of AI chips from selling to China, adding to existing sanctions that target Russia.
What’s new: The Department of Commerce restricted sales of Nvidia’s and AMD’s most-advanced chips for training and running large AI models, Reuters reported.
How it works: U.S. officials didn’t detail the specifics of the ban. Nvidia said it would stop selling its A100 and H100 graphics processing units (GPUs) to China. AMD said the action affects its MI250 GPU.
- U.S. officials told AMD that the rule “will address the risk that products may be used in, or diverted to, a ‘military end use’ or ‘military end user’ in China.”
- AMD said the restrictions will not significantly impact its bottom line. Nvidia said it could lose $400 million in sales in the third quarter, about 6 percent of sales in the same quarter of last year.
- The U.S. also blocked sales of equipment for fabricating cutting-edge chips to Semiconductor Manufacturing International Corp., which is owned partly by the Chinese government.
China’s reaction: “This violates the rules of the market economy, undermines the international economic and trade order, and disrupts the stability of global industrial and supply chains,” a foreign ministry spokesperson said. China hasn’t announced countermeasures, but some analysts anticipate that it will further increase funding to its domestic semiconductor sector.
Behind the news: Russia has faced chip embargoes by South Korea, Taiwan, and the U.S. in response to its February invasion of Ukraine. In 2020, the U.S. government required foreign chip makers that use U.S. equipment to receive special permission before doing business with the Chinese tech company Huawei.
Why it matters: AI is increasingly intertwined with geopolitics. China has repeatedly stated its intention to achieve “AI supremacy” and outpace the U.S. China, however, is still largely reliant on imported semiconductors, so the U.S. ban could hobble its ambitions.
We’re thinking: An AI chip may be designed in the U.S. and manufactured in Taiwan using equipment from the Netherlands. This globalized supply chain works well when international tensions are low, but rising tensions pose risks to both progress in AI and the security of several countries.
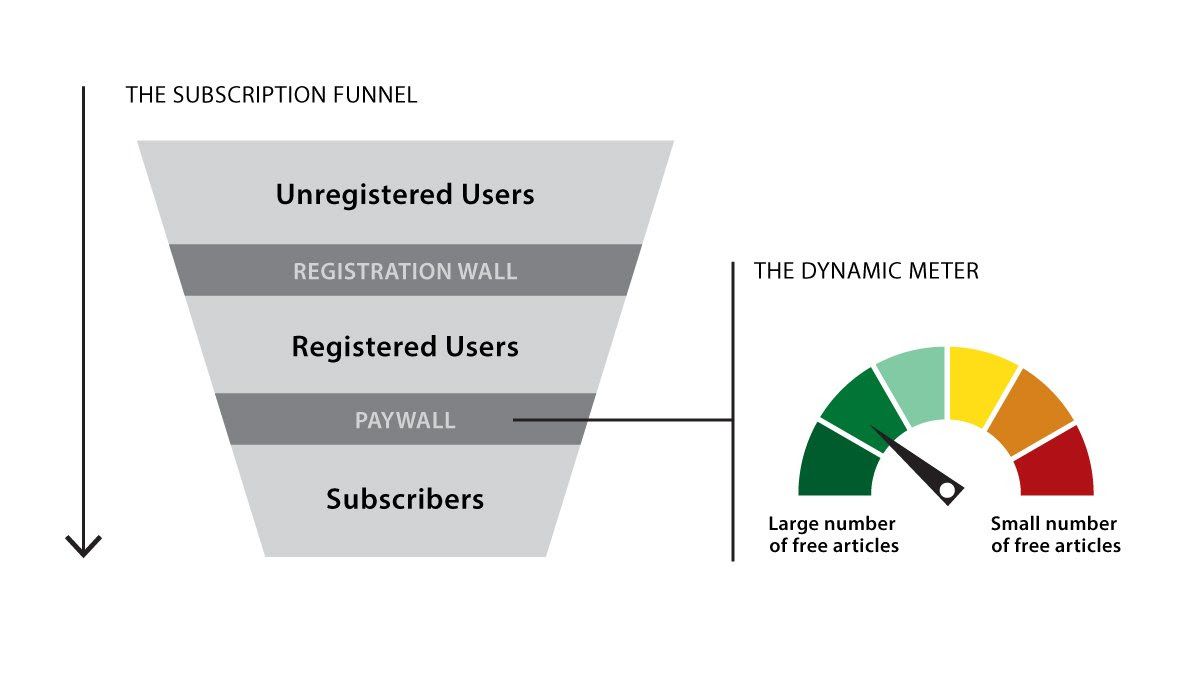
Reading Readers
A smart news paywall is optimizing subscriptions without driving away casual readers by showing them come-ons subscribe.
What’s new: The New York Times described Dynamic Meter, a machine learning system that decides how many free articles to provide to a given user before prompting them to register or subscribe.
How it works: The newspaper’s data science team ran a randomized, controlled trial and found that delivering more pop-ups that ask readers to subscribe resulted in more subscriptions but fewer page views, while delivering fewer popups resulted in fewer subscriptions but greater page views.
How it works: The New York Times’ data science team collected a dataset by running a randomized, controlled trial that tracked the behavior of registered — but not yet subscribed — users with various characteristics. Generally, delivering more pop-ups that asked them to subscribe resulted in more subscriptions but fewer page views (prior to subscribing), while delivering fewer popups resulted in fewer subscriptions but greater page views.
- The authors trained two S-learner models on anonymized user behavior and profile data from the trial. One learned to predict the number of pages a given user would view without any intervention. The other learned to predict the user’s likelihood to subscribe. The authors combined the loss functions, so the system optimized them simultaneously.
- An adjustable parameter set the degree to which the models would optimize for page views versus subscriptions. The authors adjusted that parameter and retrained the models for each value throughout its 0-to-1 range. This produced a set of optimal solutions, called a Pareto front, depending on the user’s features.
- At inference, given a user, the system chooses the point in the Pareto front that matches a monthly goal for new paid subscriptions. That point, being a model that specifies a certain number of page views, supplies the number of pages to show the user.
Behind the news: The Wall Street Journal, Switzerland’s Neue Zürcher Zeitung, and Germany’s Frankfurter Allgemeine Zeitung also use machine learning to maximize subscriptions.
Why it matters: The shift in news consumption from print to online devastated publishers, in part because they’re forced to compete with the panoply of attention-grabbing content on the web. Smart paywalls can help them thrive by tantalizing readers with free content, then forcing them to decide whether they value it relative to everything else the web has to offer.
We’re thinking: News is critical to a free society, and it’s important to distribute it fairly. Does allowing some people to read more articles than others give those people an advantage over people who are allowed to read fewer articles? Is it okay to offer a wealthy person five articles and a less-wealthy person 10 before demanding that they subscribe — or vice versa? While AI can help companies capture greater financial value, many questions of social value remain to be answered.
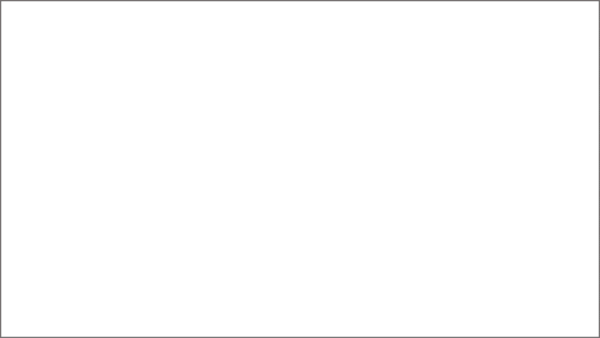
Attention to Rows and Columns
Transformers famously require quadratically more computation as input size increases, leading to a variety of methods to make them more efficient. A new approach alters the architecture’s self-attention mechanism to balance computational efficiency with performance on vision tasks.
What's new: Pale-Shaped self-Attention achieved good vision results while applying self-attention to a grid-like pattern of rows and columns within an image. Sitong Wu led the work with colleagues at Baidu Research, Chinese National Engineering Laboratory for Deep Learning Technology and Application, and Chinese Academy of Sciences.
Key insight: Previous attempts to reduce the computational cost of self-attention include axial self-attention, in which a model divides an image into patches and applies self-attention to a single row or column at a time, and cross-shaped attention, which processes a combined row and column at a time. The pale-shaped version processes patches in a pattern of rows and columns (one meaning of “pale” is fence, evoking the lattice of horizontal rails and vertical pickets). This enables self-attention to extract large-scale features from a smaller portion of an image.
How it works: The authors implemented their pale-shaped scheme in Pale Transformer, which processed an image through alternating convolutional layers and 2 or 16 transformer blocks. They trained it on ImageNet.
- The authors divided the input image into patches.
- The convolutional layers reduced the size of the image by a factor of 2 or 4.
- In each transformer block, the self-attention mechanism divided the input patches into sets of 7 overlapping, evenly spaced rows and columns. It processed each set of rows and each set of columns separately. Then it concatenated the resulting representations and passed them along to the next convolutional layer or transformer block.
- The last transformer block fed a fully connected layer for classification.
Results: The authors tested three variants of Pale Transformer, each with a different number of parameters: Pale-T (Tiny, 22 million parameters), Pale-S (Small, 48 million parameters), and Pale-B (Base, 85 million parameters). Each achieved better top-1 classification accuracy on ImageNet than competing convolutional neural networks and transformers of similar size. For example, Pale-B achieved state-of-the-art accuracy of 85.8 percent while the best competing model, VOLO-D2 (59 million parameters), scored 85.2 percent. Pale-B required somewhat more computation (15.6 gigaflops) than VOLO-D2 (14.1 gigaflops), but both required far less than a vision transformer with 86 million parameters (55.4 gigaflops). The authors also compared Pale-T against axial and cross-shaped attention. Pale-T achieved 83.4 percent accuracy on ImageNet. The same model with axial attention achieved 82.4 percent and, with cross-shaped attention, achieved 82.8 percent.
Why it matters: This work suggests that there’s room to improve the transformer’s tradeoff between efficiency and performance by changing the way inputs are processed.
We’re thinking: Will this team’s next project be beyond the pale?