Dear friends,
Stable Diffusion, an image generation model that takes a text prompt and produces an image, was released a few weeks ago in a landmark event for AI. While similar programs like DALL·E and Craiyon can be used via API calls or a web user interface, Stable Diffusion can be freely downloaded and run on the user’s hardware.
I'm excited by the artwork produced by such programs (Developer Simon Willison posted a fun tweetstorm that highlights some of the creativity they’ve unleashed), but I’m also excited by the ways in which other developers are incorporating it into their own drawing tools. Ironically, Stable Diffusion’s manner of release moves us closer to “open AI” than the way DALL·E was released by the company called OpenAI. Kudos to Emad Mostaque and his Stability AI team, which developed the program.
If you want to learn about how diffusion models like Stable Diffusion work, you can find a concise description here.
Image generation is still maturing, but it’s a big deal. Many people have the creativity to produce art but lack the drawing skill to do so. As an amateur illustrator (I like to draw pandas to entertain my daughter using the Procreate paint app), my meager skill limits what I can create. But sitting in front of the DALL·E or Stable Diffusion user interface, I can ask her what she wants to see a panda doing and render a picture for her.
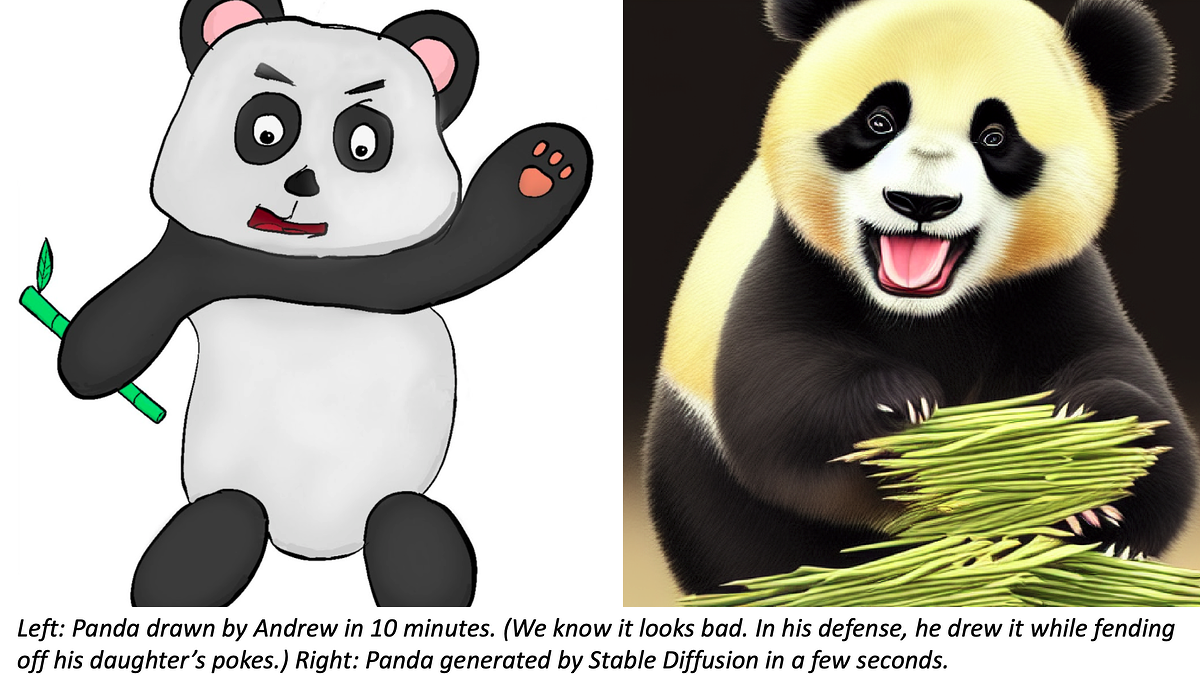
Artists who have greater skill than I can use image generators to create stunning artworks more efficiently. In fact, an image produced this way recently won an art competition at the Colorado State Fair.
The rise of inexpensive smartphone cameras brought an explosion in photography, and while expensive DSLRs still have a role, they now produce a minuscule fraction of all pictures taken. I expect AI-powered image generators to do something similar in art: Ever-improving models and user interfaces will make it much more efficient to generate art using AI than without. I see a future where most art is generated using AI, and novices who have great creativity but little drawing skill will be able to participate.
My friend and collaborator Curt Langlotz, addressing the question of whether AI will replace radiologists, said that radiologists who use AI will replace radiologists who don’t. The same will be true here: Artists who use AI will (largely) replace artists who don’t. Imagine the transition in the 1800s from the time when each artist had to source their own minerals to mix shades of paint to when they could purchase ready-mixed paint in a tube. This development made it easier for any artist to paint whatever and whenever they wished. I see a similar transition ahead. What an exciting time!
Separately from generating images for human consumption, these algorithms have great potential to generate images for machine consumption. A number of companies have been developing image generation techniques to produce training images for computer vision algorithms. But because of the difficulty of generating realistic images, many have focused on vertical applications that are sufficiently valuable to justify their investment, such as generating road scenes to train self-driving cars or portraits of diverse faces to train face recognition algorithms.
Will image generation algorithms reduce the cost of data generation and other machine-to-machine processes? I believe so. It will be interesting to see this space evolve.
Keep learning!
Andrew
DeepLearning.AI Exclusive
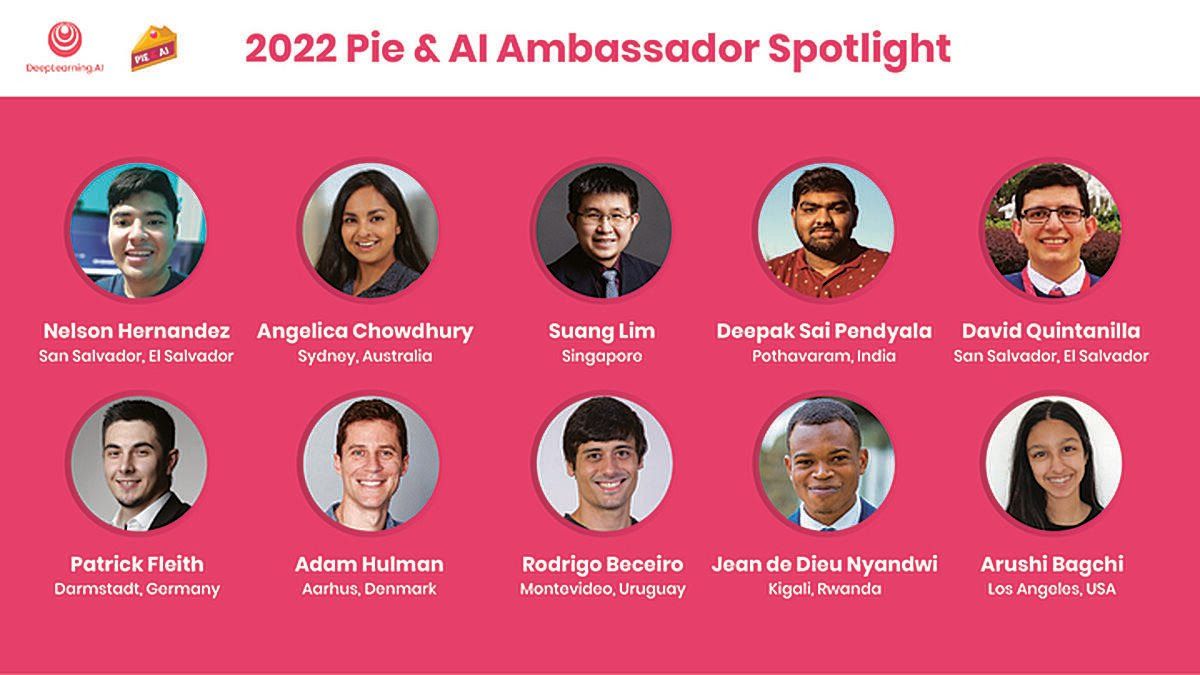
Celebrating Our Community Leaders
Since September 2019, DeepLearning.AI’s network of Pie & AI Ambassadors has brought the AI community together at more than 700 events in 61 countries. Read about these leaders, their events, and how they’re turning their local areas into AI hubs. Learn more
News
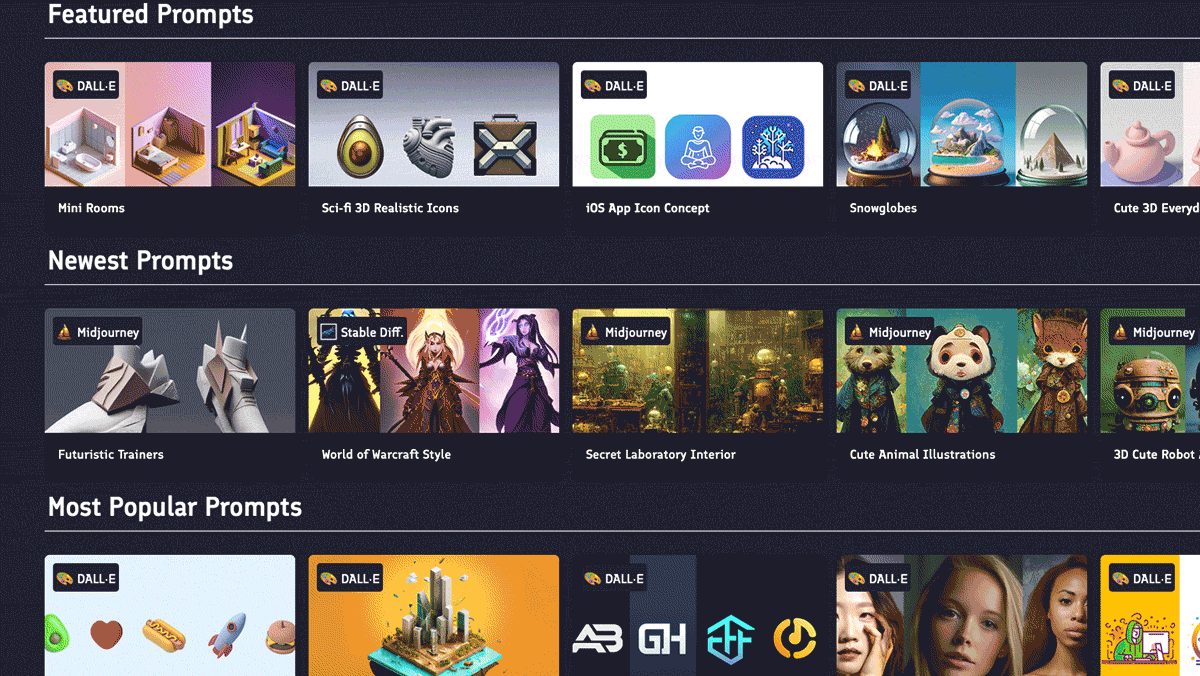
Prompting DALL·E for Fun and Profit
An online marketplace enables people to buy text prompts designed to produce consistent output from the new generation of text-to-image generators.
What’s new: PromptBase is a virtual marketplace for bespoke text strings designed as input for programs like DALL·E 2, Midjourney, and Stable Diffusion, The Verge reported.
How it works: Buyers can browse PromptBase by specifying the desired system, searching categories such as “jewelry” or “wallpaper,” or typing in keywords. They can click to purchase the prompt via credit card or Google Pay. The site, which launched in June, has 50,000 active monthly users.
- Sellers upload a prompt, a general description of its output, the target model, and example images. Bracketed portions of the prompt indicate ways the buyer can customize the output.
- PromptBase assesses the quality of uploaded prompts by running them through the target model and performing a reverse image search to weed out submitted images that weren’t generated from the prompt, founder Ben Stokes told The Batch. The site rejects offensive prompts and those that are too specific and lack real-world utility, such as “Homer Simpson on the beach in watercolor.” Sellers retain all rights to accepted prompts.
- The price per prompt ranges from $1.99 to $4.99. PromptBase takes 20 percent of the revenue from each transaction.
What they’re saying: “Every word in a prompt has a weight associated with it, so trying to work out what works best and where becomes a core asset in the skillset,” prompt engineer Justin Reckling, told The Verge.
Behind the News: Designer and illustrator Guy Parsons offers The DALL·E 2 Prompt Book, a compendium of tips for producing effective prompts for text-to-image generators. The book offers several pages of tips including words that describe specific art styles, materials, compositional structures, colors, and emotions, as well as words that can influence photorealistic output such as camera angles, settings, lenses, lighting, film stocks, and so on. Moreover, research published last year investigates the relationship between prompt structure, model parameters, and text-to-image output. The work presents a number of helpful guidelines such as, “Keep the focus on keywords rather than rephrasings.”
Why it matters: AI-driven media generators are opening a universe of productivity in imagery, text, and music. Marketplaces for effective prompts can supercharge these already-powerful tools by cutting the time it takes to generate desirable output. They can also serve as training grounds for the emerging discipline of prompt engineering: the craft of addressing generative models in ways that yield precise, repeatable output.
We’re thinking: While they may not immediately replace professional illustrators — many generated images require touching up for professional purposes — image generators are becoming a staple tool of artists and graphic designers and seem likely to put many of them out of work. We hope that prompt engineering can provide an alternative livelihood for some.
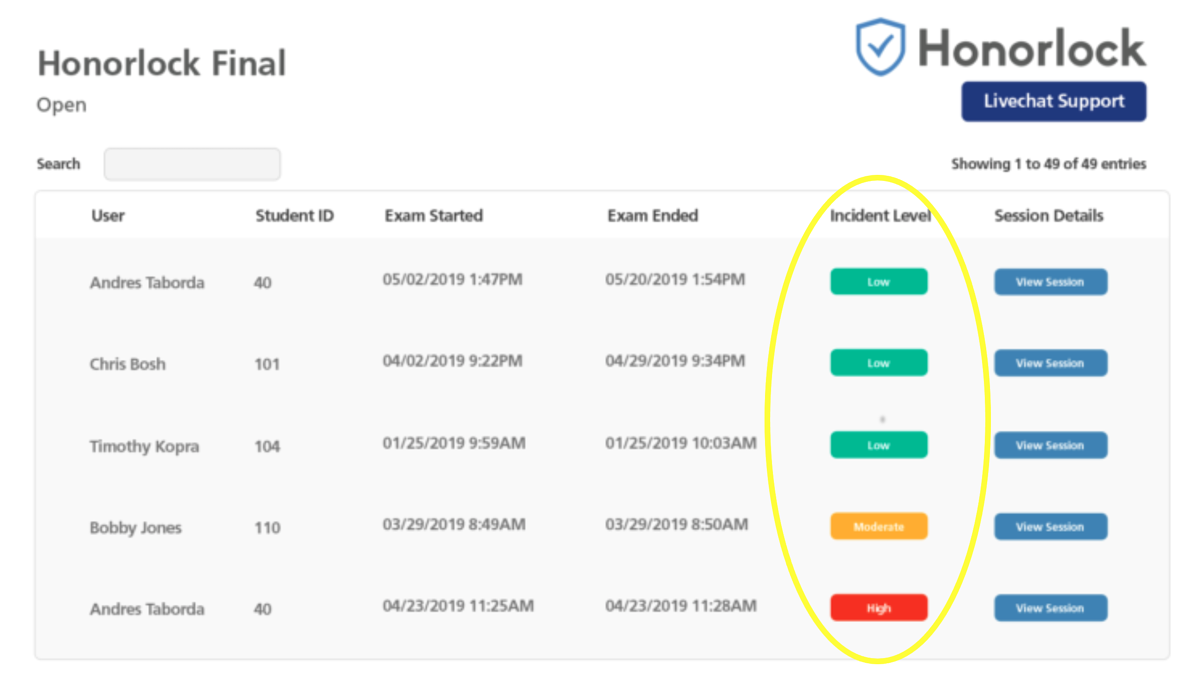
Court Blocks AI-Assisted Proctoring
A U.S. court ruled against an implementation of AI-powered software designed to catch students who cheat on academic examinations.
What’s new: A federal judge determined that Cleveland State University’s use of Honorlock, a system that scans students’ behavior and surroundings for signs of cheating, violates their rights, National Public Radio reported.
How it works: Students install Honorlock as a web browser extension and permit access to the computer’s microphone and camera.
- During a test, the extension uses voice detection and computer vision to issue alerts if it detects tablets, phones, open textbooks, dimmed lighting, faces of people other than the student, talking, phrases like “Hey Siri” or “Okay Google,” the student’s looking down or away from the screen before answering questions or absence from the camera’s view for an extended time, and other signs.
- Instructors can initiate a 360-degree scan of a student’s room prior to a test. Scans take about a minute to complete. Honorlock stores the recorded video data for a year.
- If it detects anything amiss, the system alerts a human proctor.
The case: In 2021, Cleveland State University student Aaron Ogletree sued the school for subjecting him to a virtual room scan, which he claimed violated his Constitutional protection against unreasonable searches. He complied with the scan but filed suit later. The university argued that a room scan doesn’t constitute a search because it’s limited in scope and conducted to ensure academic integrity. The judge ruled that the university had violated Ogletree’s rights.
Behind the News: Scientific investigations of other AI-powered proctoring systems have reached conflicting conclusions about their effectiveness.
- A 2021 study of a program called Proctorio found that it failed to catch any of 30 students whom the authors instructed to cheat. It also incorrectly flagged non-cheating students as engaging in suspicious activities.
- A 2020 study by Radford University found that test-takers scored lower when they were monitored by proctoring software than when they weren’t. The authors interpreted this result as evidence that
automated proctoring discourages cheating.
Why it matters: Automated proctoring has value, especially in the era of remote education. Although the ruling against Cleveland State applies only to that school, it raises questions about the legality of such automated room scans nationwide.
We’re thinking: While the judge's decision ostensibly affects AI-powered proctor software, many institutions use human proctors who might occasionally request a manual room scan. The underlying question — what proctoring methods are reasonable, ethical, fair, and legal? — is independent of whether machines or humans should do the job.
A MESSAGE FROM DEEPLEARNING.AI
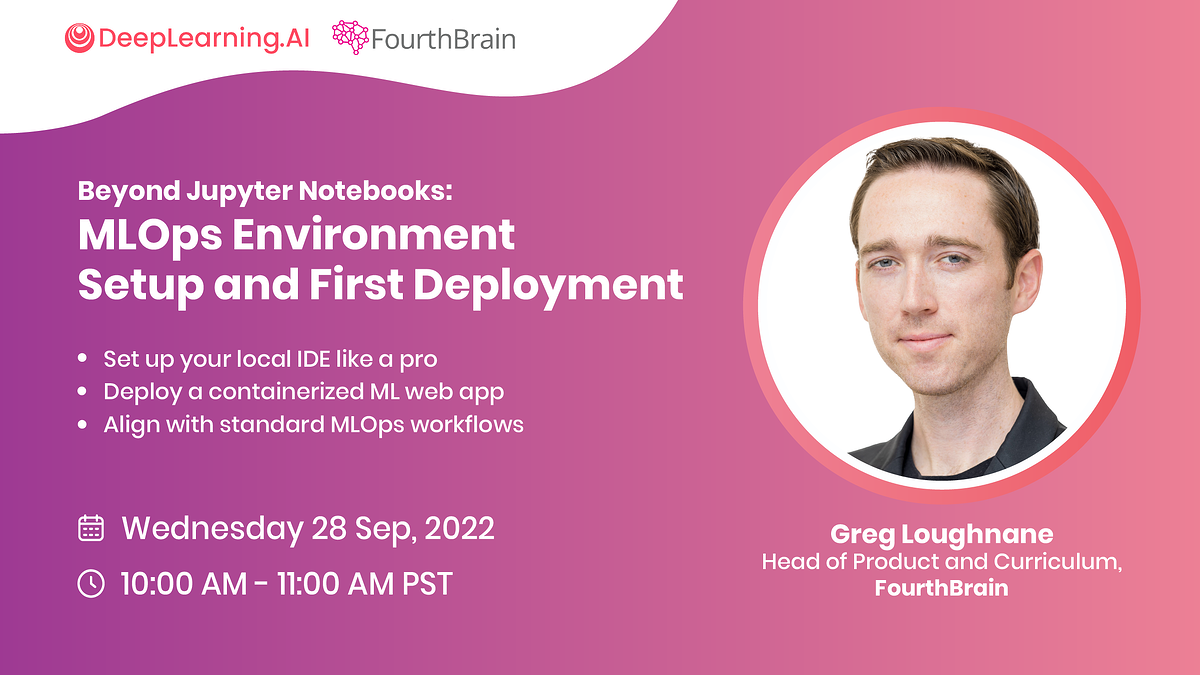
Join us on September 28, 2022, for “Beyond Jupyter Notebooks: MLOps Environment Setup and First Deployment.” This live workshop will show you how to set up your computer to build and deploy machine learning applications so you can run your models in production environments! Register here
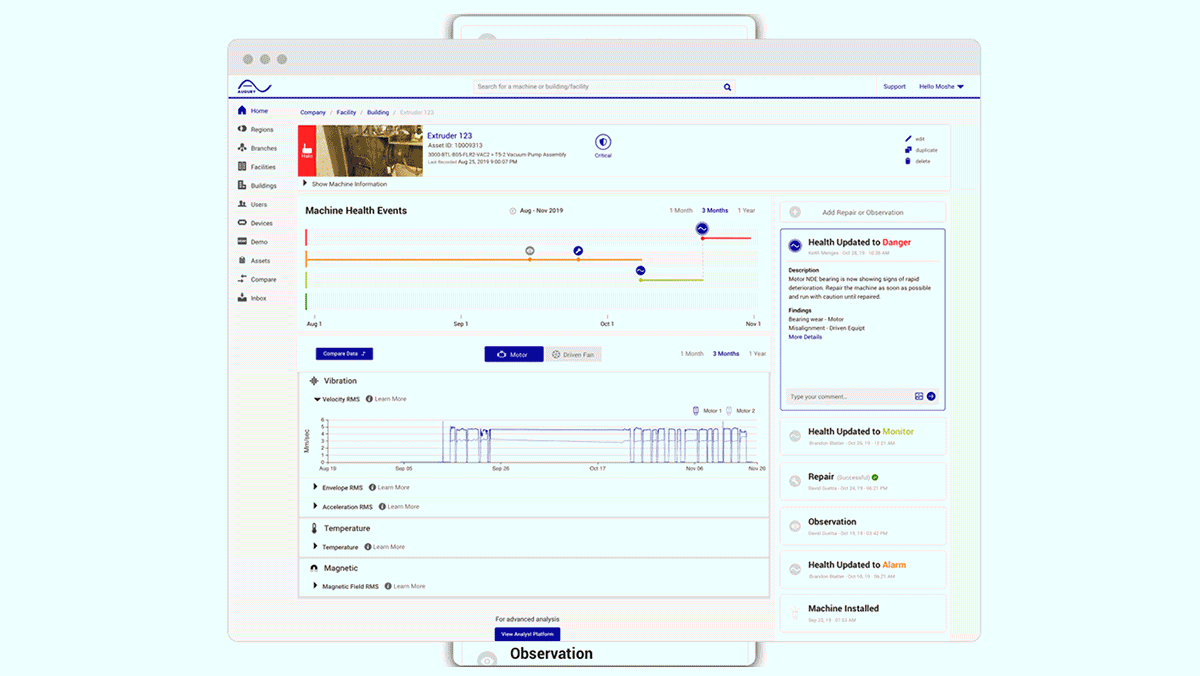
If It Ain’t Broke, Fix It
Factories are using AI to warn them when equipment is reaching the breaking point.
What’s new: Services that monitor machinery to predict imminent failure and provide guidance on necessary upkeep are booming, The Wall Street Journal reported.
How it works: Predictive maintenance systems anticipate breakdowns based on historical and real-time data collected from industrial machinery, enabling maintenance personnel to schedule repairs before they incur costly downtime.
- New York-based Augury developed a system that recognizes sounds made by a variety of gear operating at various levels of distress from brand-new to nearly broken. The company outfits factory machines with wireless audio sensors that transmit data to its cloud-based platform. When the system identifies an issue, it sends a real-time update to the plant’s maintenance team.
- Over 100 U.S. companies use Augury’s service including Frito-Lay, which installed the sensors at four plants, adding 4,000 hours of manufacturing capacity in the past year.
- Senseye, a company based in the Netherlands that was acquired by Siemens AG earlier this year, uses data that machines already collect, including pressure, vibration, and torque measurements, to identify looming issues. The company helped aluminum manufacturer Alcoa to cut unplanned downtime by 20 percent.
Behind the news: Sales of predictive maintenance services stood at around $4 billion in 2020. The global total is expected to reach $18.6 billion by 2027, expanding at a compound annual growth rate of 24.5 percent, according to the research firm Research and Markets.
Why it matters: Supply-chain problems have bedeviled industrial companies since the onset of the Covid-19 pandemic. By predicting when a machine is likely to fail, AI can help them avoid costly outages and enable them to stock up on replacement parts ahead of time.
We’re thinking: Predictive maintenance helps reduce costs on an industrial scale, but could it be adapted for households? Imagine if your washing machine could figure out for itself whether that ominous knocking sound during the spin cycle was just a momentary annoyance or truly worrisome.
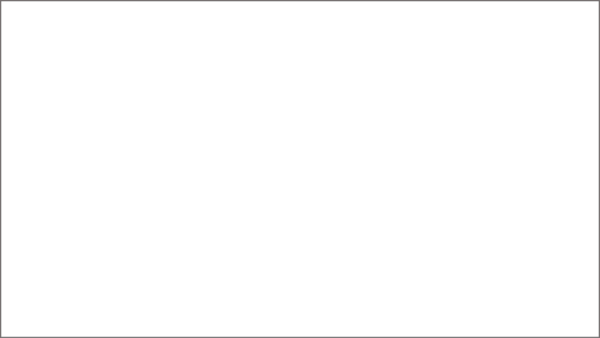
Update Any Language Model
The ability to update language models is essential to incorporate new information and correct undesirable behaviors. Previous methods are unwieldy and often fail as the amount of new data increases. New work offers a workaround.
What’s New: Eric Mitchell and colleagues at Stanford and École Polytechnique Fédérale de Lausanne proposed Semi-Parametric Editing with a Retrieval-Augmented Counterfactual Model (SERAC), an add-on system that can adapt trained models with an abundance of new information.
Key insight: Say you’ve trained a language model to produce output based on the current Prime Minister of the United Kingdom. You’ll need to retrain the model when the Prime Minister changes. Alternatively you can update the model either by fine-tuning or training a secondary model, known as a model editor, that estimates and applies the change in weights necessary to respond to queries about the Prime Minister accurately without affecting responses to other queries. However, both approaches have problems. Fine-tuning every time information changes is impractical, and both approaches fail beyond around 10 new pieces of data (as the authors demonstrate without proposing an explanation why). Instead of changing model weights, a separate system can store new data and learn to provide output to queries that are relevant to that data. Such a system would handle any amount of new data and work with any model without retraining.
How it works: The authors’ system is designed to complement a base model. It consists of three parts. The edit memory stored facts in the form of input-output pairs. The scope classifier determined whether a new input is relevant to facts stored in the edit memory. The counterfactual model generated output for relevant inputs. The base model continued to handle all other queries.
- The edit memory was a list of new input-output pairs (for example “Who is the UK Prime Minister?” “Boris Johnson”). The scope classifier was a pretrained DistilBERT fine-tuned to estimate the probability that an input was relevant to a given pair in the edit memory. The counterfactual model was a pretrained T5 language model that the authors fine-tuned to generate text based on the current input and an input-output pair.
- The fine-tuning examples, which took the form of input-output pairs, depended on the task at hand, such as question answering. Fine-tuning examples were labeled either relevant or irrelevant to pairs stored in the edit memory. For instance, given the pair “Who is the UK Prime Minister?” “Boris Johnson,” the query “Where is Boris Johnson the PM?” was relevant, while “Where did Boris Johnson attend university?” was not.
- At inference, given a new input, the scope classifier determined whether it was relevant to a pair in the edit memory. If so, it passed the most relevant pair, along with the input, to the counterfactual model to generate output.
Results: The authors used two metrics, edit success and drawdown, to evaluate SERAC’s ability to update responses from a pretrained T5-large. Edit success measured the correctness of the T5’s responses to inputs relevant to the contents of the edit memory; higher is better (1 being perfect). Drawdown measured the correctness of responses to inputs not relevant to data in edit memory; lower is better (0 being perfect). SERAC outperformed model editors such as Model Editor Networks with Gradient Decomposition (MEND). On question-answering, SERAC achieved 0.986 edit success compared to MEND’s 0.823, and 0.009 drawdown compared to MEND’s 0.187. The authors applied the SERAC system they’d trained on T5-large to other sizes. Its performance barely budged. Moreover, SERAC continued to outperform as the number of new input-output pairs increased. The authors increased the number of simultaneous pairs to 75. Measuring performance as the difference between edit success and drawdown (the worst possible being -1, best being 1), SERAC’s fell only from 0.98 to around 0.90, while MEND’s degraded from 0.64 to around -0.95.
Why it matters: This work opens the door to keeping trained language models up to date even as information changes at a rapid clip. Presumably businesses could use it to update information about, say, their products, leadership, numbers of employees, locations, and so on. Developers of conversational models could keep their chatbots abreast of changes in politics, law, and scientific discovery.
We’re thinking: A single system that can update any language model opens the tantalizing possibility of a product, updated regularly, that can adapt previously trained models to new information.