Dear friends,
The economic downturn of the past six months has hit many individuals and companies hard, and I’ve written about the impact of rising interest rates on AI. The effects of high inflation, the Russian war in Ukraine, and an economic slowdown in China are rippling across the globe. Even though unemployment in the U.S. is low, within the tech world, I continue to hear things that point to the possibility that we might go through a challenging time for many months to come.
The layoffs at Twitter and Meta are well publicized. Anecdotally, I’ve heard many worrisome stories: Students are having a hard time finding internships for next summer, entrepreneurs are having greater difficulty raising capital, companies are freezing hiring and reducing headcount, and employees are facing effective pay cuts as falling share prices reduce the value of their stock-based compensation. Some managers have told me they want to preserve their machine learning teams — which they hired with great difficulty — but the tech market has cooled and likely will take a while to pick up.
What can we do amid the turmoil? Even if the tech world slumps, the long-term value of AI is still clear to me, and it’s worth lifting our eyes toward the future to position ourselves for the eventual rebound.
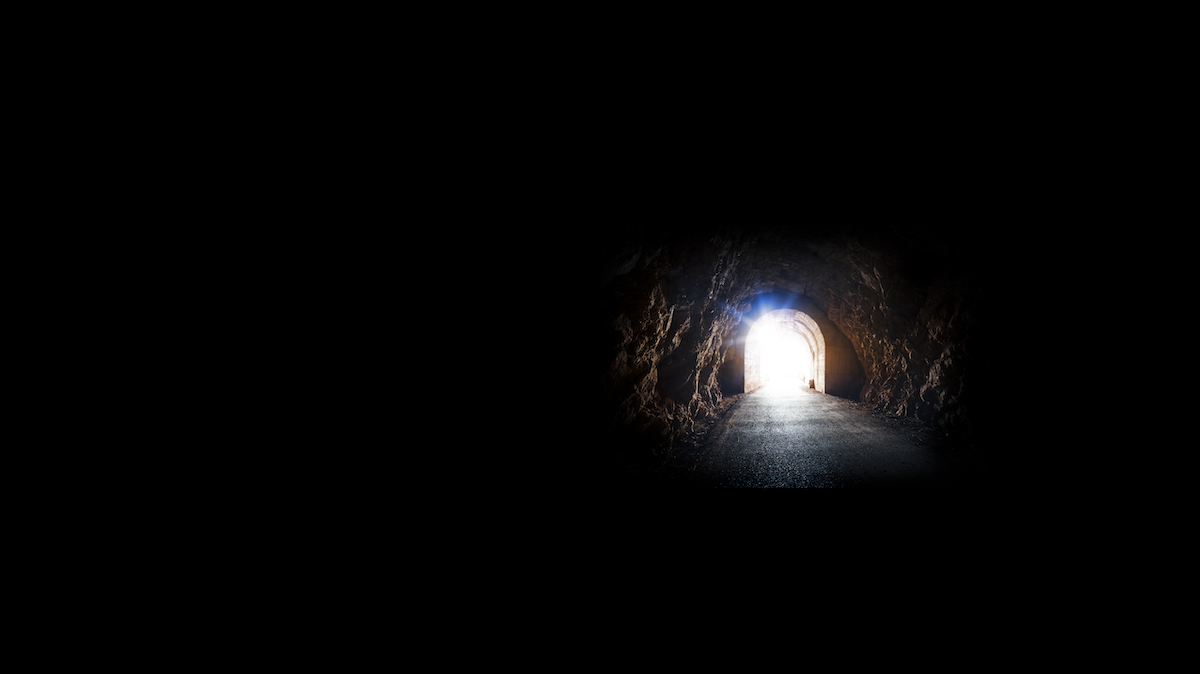
I’d like to draw attention to three investments that I believe will retain or increase their value even in uncertain times. If you’re wondering where to put your effort, attention, or money, consider these areas:
- Deep technology. AI technologies from programming frameworks like TensorFlow and PyTorch to algorithmic breakthroughs like transformers and diffusion models have deep and long-lasting value. Deep tech is difficult to build, and it transforms the way we do AI. I’m continuing to work on deep tech in data-centric AI. Collectively we should keep working to build deep tech, and I’m confident that the long-term benefits to society will be profound.
- Training. During a bumpy job market, many people stay in school longer (if they can afford it) in the hope of graduating into a healthier job market. Real expertise in technology will continue to hold tremendous value because it helps you to shape the future. So if you’re not sure what to invest in, keep investing in your own technical skills. Wherever the world ends up, people with deep technical skill in AI will be in demand.
- Community. Having lived in different places, I’ve seen first-hand how some cities have strong communities, where neighbors watch out for each other and lend a helping hand when people are down on their luck, and weak ones, where hardly anyone knows anyone else, and falling sick means having to take care of yourself. The AI community has always been stronger together. If we can step back from wondering how to build our next project or get that promotion and, instead, ask how we can help others around us, the investment in human relationships will have tremendous value.
Whether or not the economic downturn affects you, I’m here to support you. As we sail through a potentially tough time in the coming months, remember that the long-term impact of AI has been and will continue to be huge. Let’s keep helping each other and investing in things that will make us stronger for when the world exits its current slump.
Keep learning!
Andrew
News
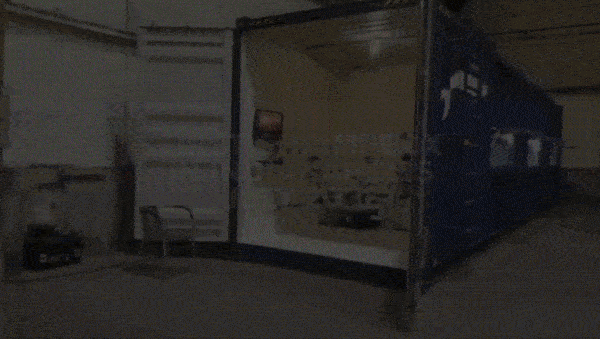
Tanks for All the Fish
Farming shrimp in an open pond produces toxic effluent that can pollute groundwater and coastal waters. An AI-driven farm in a box may offer a more sustainable alternative.
What’s new: Based in Mexico City, Atarraya modifies shipping containers into AI-controlled tanks for raising commercial shrimp, Fortune reported. The company plans to install 20 units in a warehouse in Indianapolis.
How it works: The company’s Shrimpbox contains two large water tanks equipped with sensors that track pH, nutrients, chemicals, and temperature. Machine learning models automatically dispense food and adjust conditions as needed.
- The models optimize growth of algae and fungi that consume shrimp waste. This keeps the creatures healthier and reduces the need to flush the water. The microorganisms’ own waste serves as a secondary food source.
- Users can adjust settings and feed the shrimp remotely.
Behind the news: The seafood industry is using AI to reduce its environmental footprint in a variety of ways.
- Norway-based Aquaticode uses neural networks to scan, classify, and sort salmon, helping fish farms to breed larger stock with fewer resources.
- Aquabyte provides systems that monitor the health of farmed fish and predict optimal harvest times, helping to reduce waste.
- Shinkei Systems manufactures a ship-mounted machine that automatically kills and cleans freshly caught fish according to standards set by high-end sushi restaurants, so they reject fewer fish.
Why it matters: If it can scale, Shrimpbox addresses several pain points in aquaculture. Aquaculture can put a dent in overfishing, which threatens wild fish populations worldwide. Growing seafood in tanks rather than open water won’t leach waste, antibiotics, and other chemicals into the surrounding environment. And containerized tanks can enable food to be grown near where it will be consumed, which eliminates the need to transport it long distances.
We’re thinking: The shrimp are just prawns in this company’s game.
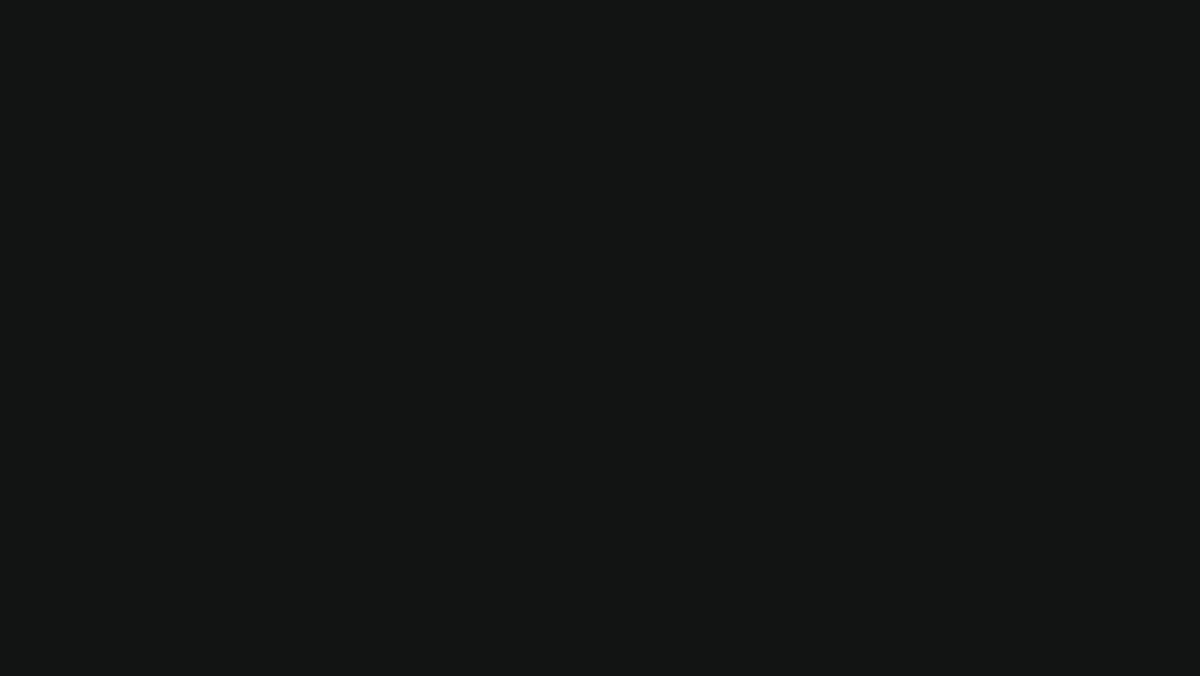
When Safety Becomes Surveillance
United States colleges tracked activists using a natural language processing system intended to monitor their mental health.
What’s new: An investigation by The Dallas Morning News and UC Berkeley Graduate School of Journalism found that schools in Georgia, North Carolina, and elsewhere used Social Sentinel, which monitors social media posts to identify individuals who intend to harm themselves or others, to keep tabs on protestors from 2015 to 2019 and possibly beyond.
What they found: The system, which was renamed Navigate360 Detect in 2020, uses an “evolving AI language engine” to analyze public communications. Users can query social media posts to Facebook, Instagram, Reddit, Twitter, and YouTube, although searches are limited to eight topics and 25 subtopics related to safety and security. The reporters studied documents acquired through leaks and requests to the government along with interviews with school employees. Among their findings:
- Beyond public posts, the system also scans emails, Google Docs, Google Hangouts, and Facebook Messages. It can also detect web searches of domains that a customer deems harmful.
- The developer privately promoted the system to school officials to mitigate and forestall campus protests.
- North Carolina Agricultural and Technical State College in 2019 used the software to track social-media comments made by a student who criticized university authorities for mishandling her rape complaint.
- Kennesaw State University in Georgia used the software to monitor protestors — including at least one person who did not attend the university — in at least three demonstrations in 2017.
- UNC-Chapel Hill’s campus police used the software to monitor participants in pro- and anti-abortion protests in 2015, and demonstrations in 2018 calling to remove a statue that celebrated the rebel army in the U.S. Civil War of the mid-1800s.
The response: Navigate360, the Ohio-based company that acquired Social Sentinel in 2020, stated that the investigation was inaccurate and that the word “protest” was not in the system’s list of search topics. School officials didn’t respond to the reporters’ requests for comment and declined to discuss policies that govern their use of such software.
Why it matters: Campuses must tread a line between keeping students safe and hosting free expression. Protests can spiral out of control, causing injury and loss of life. Yet students have a reasonable expectation that educational institutions have their best interests at heart and will support their intellectual inquiries — even if they lead to peaceful protests.
We’re thinking: AI can do good by alerting school officials to students who are severely disturbed or distressed. It should go without saying that systems designed for this purpose should never be used to stifle dissent.
A MESSAGE FROM DEEPLEARNING.AI
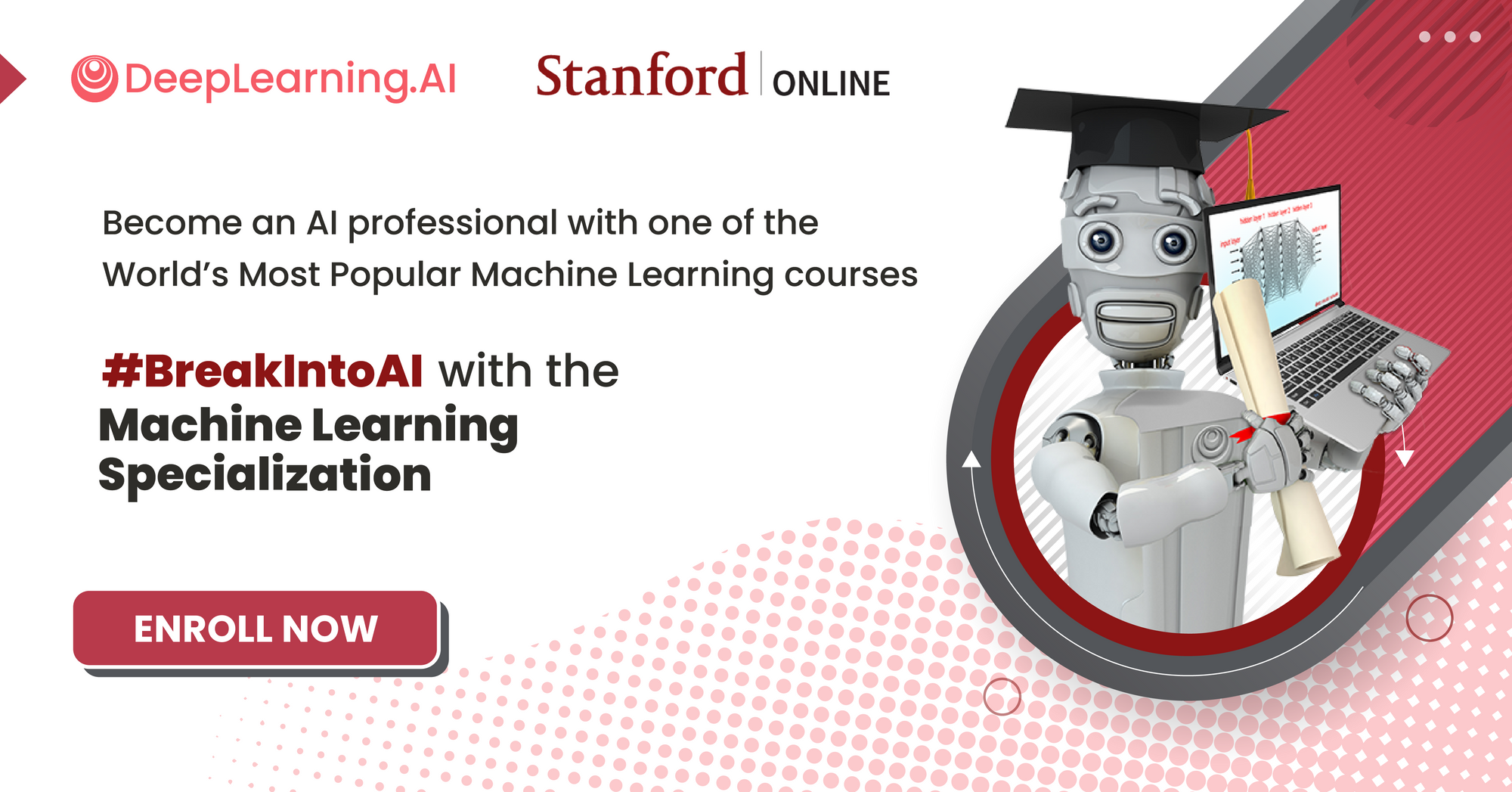
Gain the skills to thrive in an uncertain economy! Companies are seeking qualified professionals who can tap AI’s potential. Break into AI with the new Machine Learning Specialization, an updated program for beginners created by Andrew Ng. Learn more
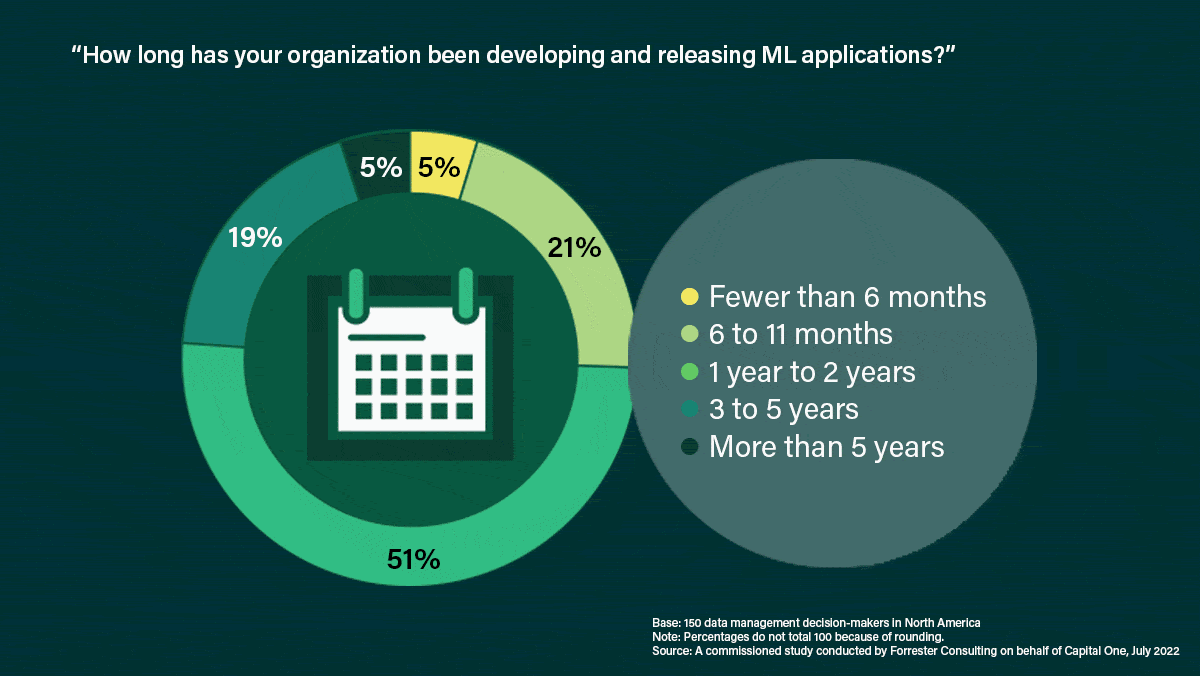
What Businesses Want from AI
In a new report, business leaders share their machine-learning successes and struggles.
What’s new: Many businesses plan to increase their use of machine learning, but their efforts so far don’t always yield the results they seek, according to a study performed by the market analyst Forrester and commissioned by the bank Capital One.
Machine learning on the rise: The authors surveyed 150 “data-management decision-makers” who work for North American companies in banking, information technology, manufacturing, and retail about how their organizations have used — and hope to use — machine learning.
- The respondents used machine learning primarily to analyze data. A high priority for this group in the next one to three years was detecting anomalies such as fraudulent bank transactions. Further priorities included improving customer experiences and growing revenue.
- Two-thirds planned to increase the use of machine learning across their organizations.
- 77 percent began using machine learning in the past two years, and 24 percent started more than two years ago.
Room for improvement: The respondents also outlined several worries.
- Around half of respondents said their teams lacked sufficient machine learning expertise. Two-thirds said their organizations were partnering with proven leaders to overcome machine learning challenges.
- 57 percent said that organizational barriers between data scientists and other departments inhibited deployment of machine learning projects, and 41 percent stated that their primary challenge is breaking down those barriers.
- 47 percent said their organizations struggled to use machine learning to inform strategic decisions, and 73 percent struggled to explain the business value of their machine learning applications to executives.
Behind the news: The talent shortage in machine learning and data science is well documented. A 2020 Deloitte survey found that companies across all industries struggled to find the machine learning engineers that would help them meet their business goals. Some companies offer incentives to attract people skilled in AI, such as offering remote work at Silicon Valley pay rates and providing time off to pursue personal projects.
Why it matters: Machine learning continues to expand in mainstream businesses, and with it opportunities for machine learning engineers and data scientists. An earlier Forrester study found that business leaders who see clear value in AI are (a) using or expanding their use of the technology and (b) effectively using the resulting insights to drive their business strategies. The new report shows that they believe the potential is greater still — and that bringing more machine learning engineers onboard could make the difference.
We’re thinking: Many industries are still figuring out how to get the most out of AI. If you can make its value clear to executives in your organization — one of the top issues in this study — you can play a big role in moving things forward.
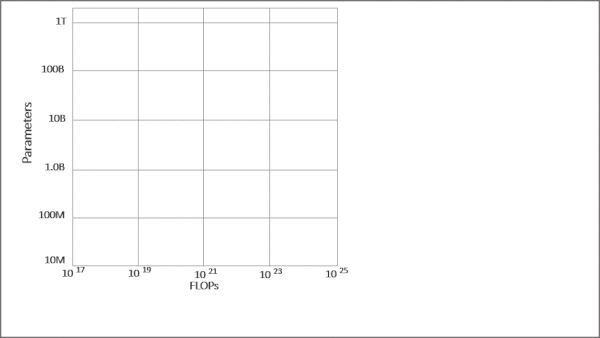
Right-Sizing Models for the Dataset
The route to improving transformer-based language models like GPT-3 and Gopher, which are trained on immense quantities of text scraped from the web, has been to increase their size. But research into the relationship between dataset size and parameter count shows that, given a processing budget, bigger doesn’t necessarily mean better.
What’s new: Jordan Hoffmann, Sebastian Borgeaud, Arthur Mensch, and colleagues at DeepMind determined the optimal data-to-parameter ratio for a range of processing budgets. They used this knowledge to train Chinchilla, a smaller but higher-performance version of Gopher.
Key insight: Pumping up dataset and architecture sizes can improve the performance of language models (with diminishing returns as they increase). But past studies didn’t account for the impact of the number of training tokens (the number of training steps multiplied by the number of tokens per step) or the learning rate. A systematic study of these variables makes it possible to estimate the optimal model and data size for a particular processing budget.
How it works: The authors trained and tested hundreds of transformer-based language models using various combinations of parameter count, dataset size, training token count, and learning rate. They trained the models to complete sentences in 2.35 billion documents scraped from the web.
- The authors experimented with a range of processing budgets (between 10^18 and 10^21 floating point operations, or FLOPs) by varying the number of model parameters (from 70 million to 10 billion) and training tokens (from 10^9 to 10^12). For each model, the authors also searched for the learning rate that resulted in the smallest loss at the end of training.
- The authors measured model performance by the loss value at the end of training. They determined the combinations of training token and parameter counts that led to the lowest loss value for each processing budget.
- They applied this information to the architecture and training procedure used to build Gopher, yielding Chinchilla. Both models were trained with a processing budget of 5.76 x 10^23 FLOPs. Gopher used 280 billion parameters and 300 billion training tokens, while Chinchilla used 70 billion parameters and 1.4 trillion training tokens.
Results: Doubling parameters or training tokens requires quadrupling the processing budget to reach optimal performance. In other words, if you double a model’s parameter count, doubling the number of training tokens will achieve an optimal balance between processing and performance. Given Gopher’s processing budget, Chinchilla outperformed its predecessor on several benchmarks with a quarter of its parameters. On BIG-bench, for example, Chinchilla’s average accuracy was 65.1 percent compared to Gopher’s 54.4 percent. In reading comprehension on LAMBADA, in which the model answers a question after reading a piece of text, Chinchilla attained 77.4 percent accuracy while Gopher achieved 74.5 percent and Megatron-Turing NLG, with a whopping 530 billion parameters, achieved 76.6 percent.
Why it matters: Large models like Gopher aren’t reaching their full potential. Smaller models trained on more training tokens can run faster during inference and achieve better performance.
We’re thinking: In light of this work, a monster model like Megatron-Turing NLG 530B should train on 11 trillion tokens. All the text on the web encompasses only a couple trillion!