Dear friends,
Will the future of large language models limit users to cutting-edge models from a handful of companies, or will users be able to choose among powerful models from a large number of developers? We’re still early in the development of large language models (LLMs), but I believe that users will have access to models from many companies. This will be good for innovation.
We've seen repeatedly that yesterday’s supercomputer is tomorrow’s pocket watch. Even though training an LLM currently requires massive data and infrastructure, I see encouraging progress toward wider availability and access along three dimensions:
- Open models are gaining traction and delivering solid performance, such as BigScience’s BLOOM, Tsinghua University’s GLM, and Meta’s OPT (released under a restrictive license that welcomes researchers but bars commercial use). Today’s open models aren’t as good as some proprietary models, but they will continue to improve rapidly.
- Researchers are developing techniques to make training more efficient. DeepMind published recommendations for how to train LLMs given a fixed computational budget, leading to significant gains in efficiency. Although it addresses smaller models, cramming improves the performance that can be achieved with one day of training language models on a single GPU. Recent work using eight-bit and even four-bit computation is also pushing the possibilities for inference.
- As more teams develop and publish LLMs, there will be systematic comparisons that empower users to pick the right one based on cost, availability, and other criteria. For example, a team led by Percy Liang carried out an extensive study that compares LLMs. (Skip to the “Our Findings” section if you’re impatient to see their conclusions.)
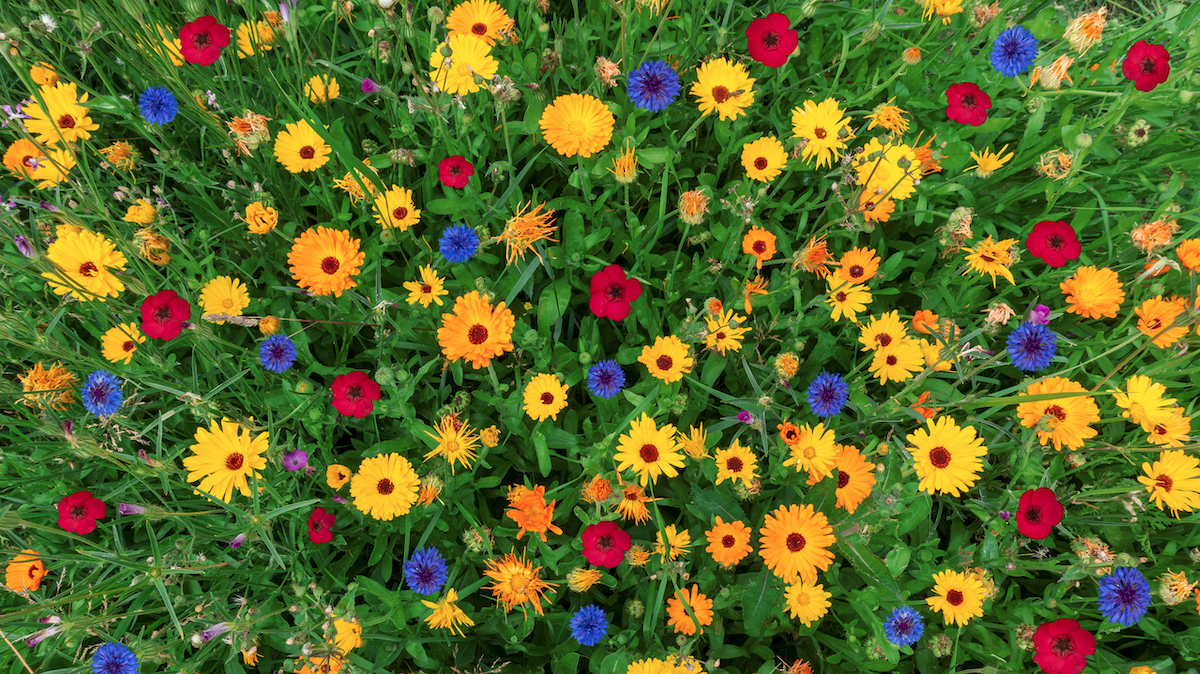
There were times in my career when I worked with some of the world’s biggest systems dedicated to training deep learning models, but they didn’t last. I had access to massive parallel computing power at Google, and my teams built an early GPU server at Stanford and a high-performance computing system focused on speech recognition. Faster systems soon left those formerly cutting-edge systems in the dust. Even though training an LLM currently requires a daunting amount of computation, I see little reason to believe that it won’t quickly become much easier, particularly given the widespread excitement and massive investment around them.
What does this mean for businesses? Many companies have built valuable and defensible businesses using early innovations in deep learning, and I foresee that similarly valuable and defensible systems will be built using recent innovations in LLMs and, more broadly, generative AI.
I will explore this topic more in future letters. Until then,
Keep learning!
Andrew
News
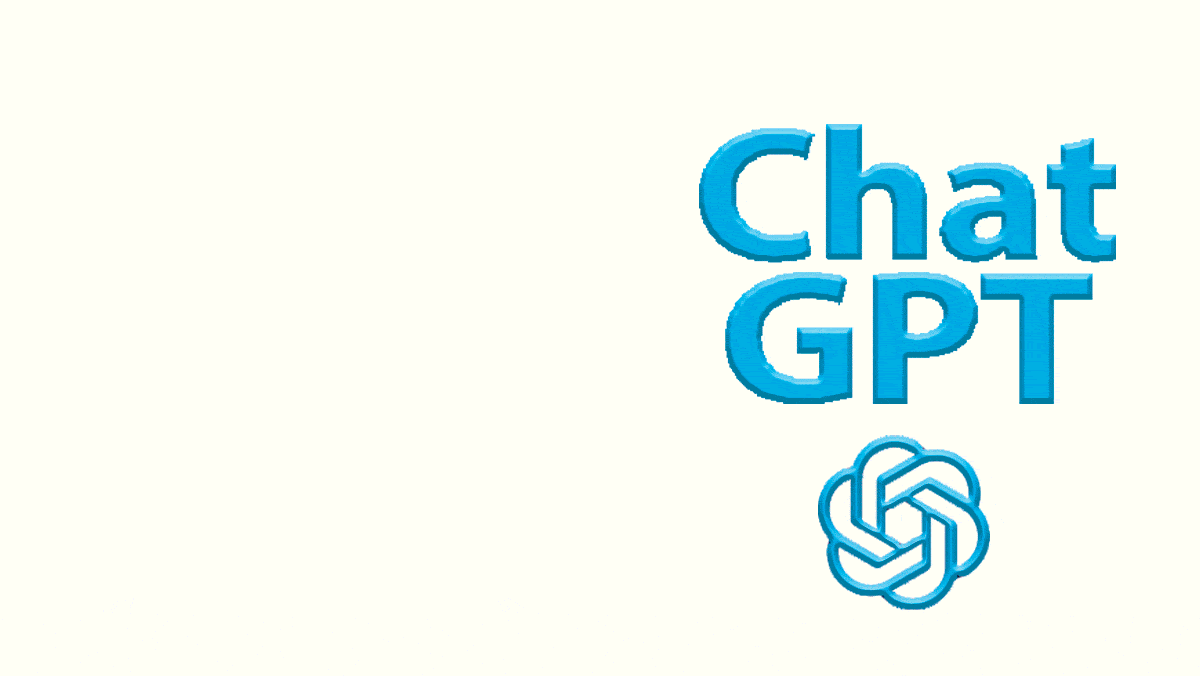
ChatGPT Backlash
The breakout text generator faces resistance — even within the AI community.
What's new: Organizations including the International Conference on Machine Learning (ICML) and the New York Department of Education banned OpenAI's ChatGPT amid debate over the implications of its use and limitations of its output.
What happened: Professional societies, schools, and social media sites alike reacted to the potential of ChatGPT and other large language models to produce falsehoods, socially biased information, and other undesirable output in the guise of reasonable-sounding text.
- The organizers of the upcoming ICML in Honolulu prohibited paper submissions that include text generated by large language models, including ChatGPT, unless the text is included for analytical purposes. They cited including novelty and ownership of generated material. However, the conference will allow papers with text that has been polished using AI-powered services like Grammarly. The organizers plan to re-evaluate the policy in advance of the 2024 meeting in Vienna.
- New York City blocked access to ChatGPT in the city's 1,851 public schools, which serve over one million students. Officials expressed concern that the tool enables plagiarism and generates falsehoods.
- Social media app WeChat prohibited a mini-program that allowed users to access ChatGPT from within the app.
- In December, question-and-answer website Stack Overflow banned ChatGPT-generated content due to the model's propensity for outputting incorrect answers to technical questions.
Behind the news: Researchers have raised red flags around the issues that have prompted organizations to ban ChatGPT since large language models first showed a propensity to generate plausible but unreliable text. The latest efforts seek to identify generated output.
- OpenAI aims to embed cryptographic tags into ChatGPT’s output to watermark the text. The organization told TechCrunch it’s working on other approaches to identify the model’s output.
- Princeton University student Edward Tian built GPTZero, an app that determines if a passage's author was human or machine by examining the randomness of its words and sentences. Humans are more prone to use unpredictable words and write sentences with dissimilar styles.
Yes, but: Users may find ways to circumvent safeguards. For instance, OpenAI’s watermarking proposal can be defeated by lightly rewording the text, MIT computer science professor Srini Devadas told TechCrunch. The result could be an ongoing cat-and-mouse struggle between users and model-makers.
Why it matters: Many observers worry that generative text will disrupt society. EvenOpenAI CEO Sam Altman tweeted that the model was currently unsuitable for real-world tasks due to its deficiencies in truth-telling. Bans are an understandable, if regrettable, reaction by authorities who feel threatened by the increasingly sophisticated abilities of large language models.
We're thinking: Math teachers once protested the presence of calculators in the classroom. Since then, they’ve learned to integrate these tools into their lessons. We urge authorities to take a similarly forward-looking approach to assistance from AI.
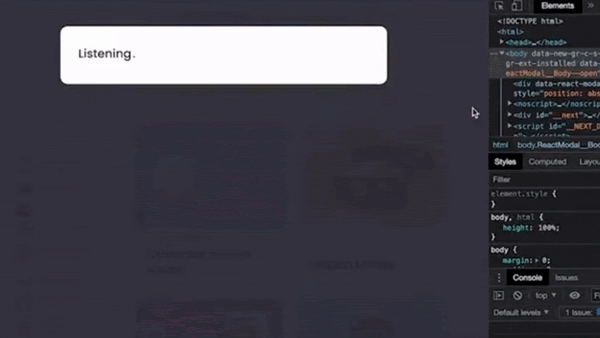
Your Personal Deepfaked Agent
Hate talking to customer service? An AI-powered tool may soon do it for you.
What's new: Joshua Browder, chief executive of the consumer advocacy organization DoNotPay, demonstrated a system that autonomously navigates phone menus and converses with customer service representatives in a deepfaked version of his own voice. DoNotPay plans to offer a free version that uses generic voices as well as a paid option that lets users clone their own voice, Browder told Vice.
How it works: In the video demo that has been removed from YouTube, the system could be seen and heard negotiating with a bank representative to refund wire-transfer fees.
- The system interacts with corporate voice portals using an instance of OpenAI’s GPT-3.5 language model that was fine-tuned on automated customer-service prompts.
- Resemble.AI’s Clone service generates a synthetic version of Browder’s voice.
- Having reached a human representative, the system generates conversational responses and feeds them to Clone using GPT-J, an open source language model from HuggingFace. (Browder told The Batch he believes using GPT-3.5 to impersonate a human being would violate that model’s terms of service.)
Yes, but: The ethical question whether humans — be they consumers or customer-service reps — should be informed when they’re conversing with a bot remains open. The technology clearly invites fraud. Cybercriminals have already used OpenAI's large language models for phishing attacks, cybersecurity analyst Check Point Research found in a recent study. In 2020, a group scammed a Dubai bank out of $400,000 by synthesizing a customer’s voice.
Why it matters: Nobody likes to spend time on the phone with customer service. AI could make this obsolete, saving time and possibly gaining refunds.
We're thinking: Enjoy using your automated doppelganger to deal with customer service while you can! As corporations and financial institutions strengthen their defenses against automated fraud, they’re likely to downgrade service to automated customers as well.
A MESSAGE FROM AI FUND
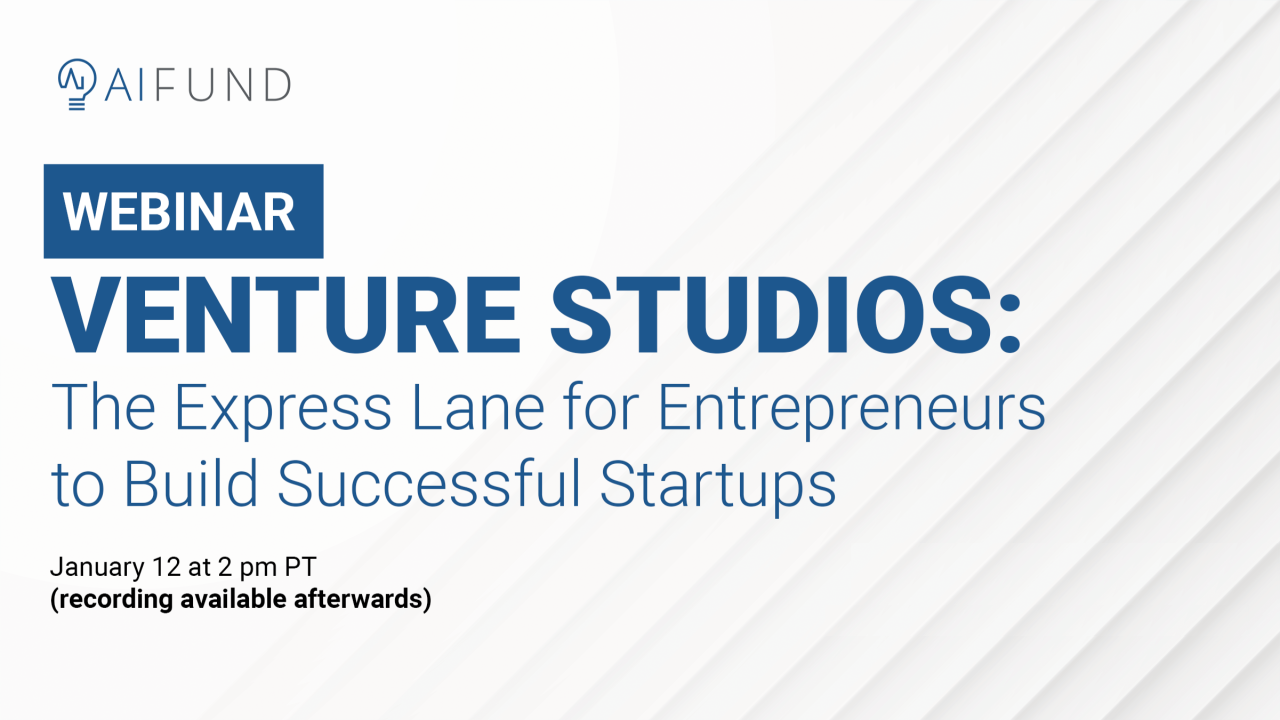
Building a startup is hard. But with a venture studio as a partner, founders dramatically increase their odds of success. Join us on January 12, 2023, at 2:00 p.m. Pacific Time to learn how venture studios work and how AI Fund sets up entrepreneurs to win. Register here
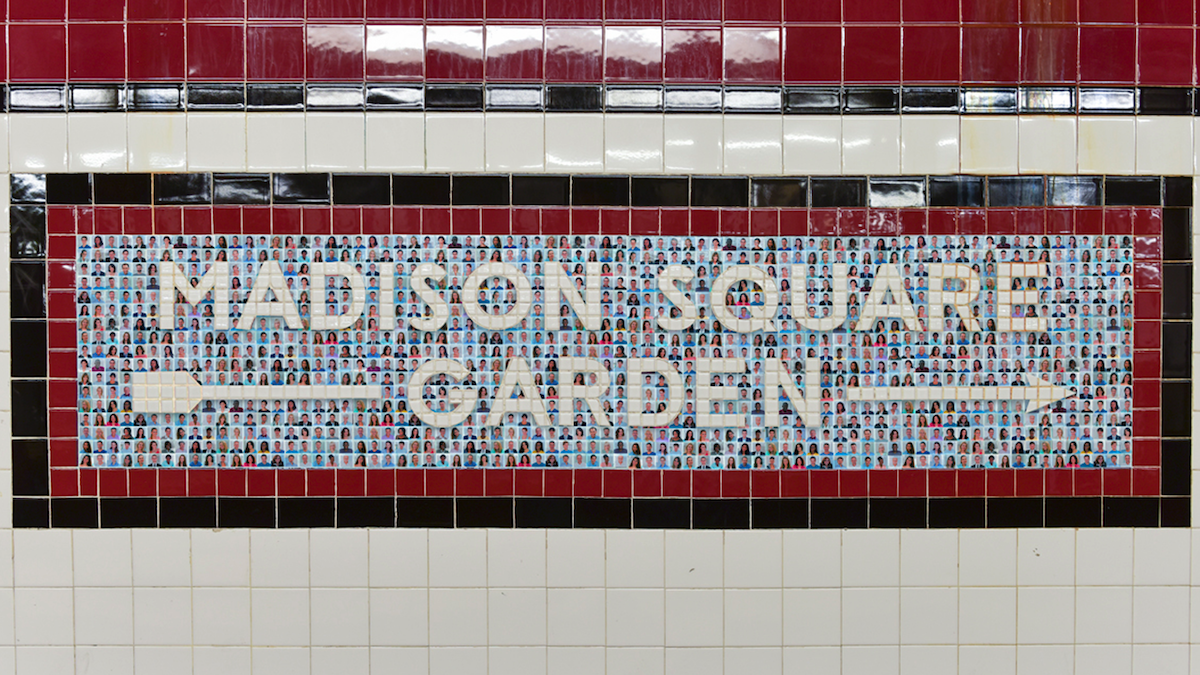
Looking for Enemies
A major company is using face recognition to settle scores.
What's new: MSG Entertainment, which operates large entertainment venues in several cities in the United States, used face recognition to block its perceived enemies from attending events at New York’s Madison Square Garden and Radio City Music Hall, The New York Times reported.
What happened: MSG used the technology on at least two occasions to eject attorneys who work at law firms involved in litigation against the company.
- In November 2022, guards at Radio City Music Hall prevented Kelly Conlon from attending a concert with her daughter after face recognition identified her as an attorney at a firm representing a personal-injury lawsuit against MSG.
- The previous month, Madison Square Garden ejected Barbara Hart after face recognition identified her as an attorney at a different firm suing MSG on behalf of some of its shareholders.
- MSG claimed that the actions were legal and in accordance with its established policy of barring attorneys employed by firms engaged in active lawsuits against the company, regardless of whether the attorney is involved in the lawsuit.
Behind the news: New York does not restrict use of face recognition by private companies. MSG venues have used the technology since at least 2018 to compare attendees’ faces to a database of photographs and flag individuals the company considers undesirable. Prior to Conlon’s ejection, a judge ruled that MSG has a right to deny entry to anyone who doesn’t hold a valid ticket; Conlon’s employer sued in a case that is ongoing.
Why it matters: Privacy advocates have long feared that face recognition could enable powerful interests to single out individuals for retribution. MSG’s use of the technology to target its perceived enemies certainly fits that description.
We're thinking: Face recognition is a flashpoint in AI, and rightly so. We need to protect privacy and fairness even as we improve safety and productivity. But outrage over such ill-considered uses of the technology could lead regulators to ban it despite its potential for good — for instance, by helping security personnel identify people who are legally barred from an area. Regulators who focus on face recognition should address ethical gray areas as well as outright abuses.
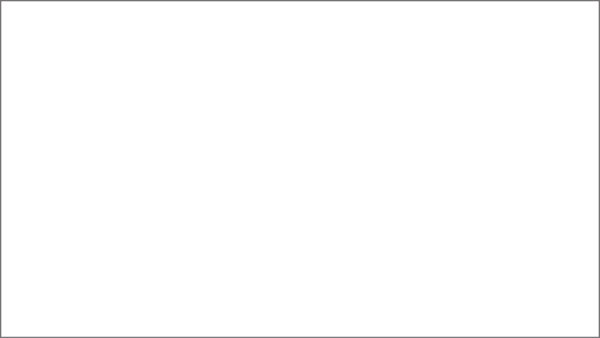
Segmented Images, No Labeled Data
Training a model to separate the objects in a picture typically requires labeled images for best results. Recent work upped the ante for training without labels.
What’s new: Mark Hamilton and colleagues at Cornell, Google, and Massachusetts Institute of Technology developed Self-supervised Transformer with Energy-based Graph Optimization STEGO, an architecture and training method for semantic segmentation that substantially improved the state of the art for unsupervised learning of this task.
Key insight: A computer vision model pretrained on images produces similar representations of pixels that belong to similar objects, such as patches of sky. By clustering those representations, a model can learn to identify groups of pixels that share a label without referring to the labels themselves. (If the feature extractor learns in an self-supervised way, it doesn’t need labels either.)
How it works: A feature extractor (the transformer DINO, which was pretrained in an unsupervised manner on ImageNet) generated features for each pixel of input images. A vanilla neural network trained on COCO-Stuff refined the features into a representation of each pixel.
- DINO received an image and produced features for each pixel. The features were stored.
- During training, the vanilla neural network received the features of three images: the target image, an image with similar features (according to k-nearest neighbors), and a randomly selected image. Its loss function compared the representations it produced with the stored features and encouraged the model to make its representations similar to features of the similar image and different from features of the randomly selected image. This pushed the representations of similar pixels into tight clusters that would be easy to separate.
- At inference, given an image, DINO created pixel-wise features and the vanilla neural network produced representations. The authors grouped the representations via k-means clustering. Based on the clusters, they produced a segmentation map that showed which pixels belong to which objects.
Results: To measure how well their model separated the objects in an image, the authors used a matching algorithm to match grouped pixels with ground-truth labels (that is, they labeled the pixels). Their method achieved 28.2 percent mean intersection over union (the ratio of the number of correctly labeled pixels to total number of pixels, averaged over all classes) on the 27-class COCO-Stuff validation set. Its closest unsupervised rival, PiCIE+H, achieved 14.4 percent mean intersection over union. As for supervised approaches, the state-of-the-art, ViT-Adapter-L, achieved 52.9 percent mean intersection over union.
Why it matters: This system is designed to be easily upgraded as datasets and architectures improve. The authors didn’t fine-tune the feature extractor, so it could be swapped for a better one in the future. Upgrading would require retraining the relatively small vanilla neural network, which is faster and simpler than training a typical semantic segmentation model.
We’re thinking: Since it didn’t learn from labels, the authors’ vanilla neural network can’t identify the objects it segments. Could it learn to do that, CLIP-style, from images with corresponding captions?