Dear friends,
I’ve been reflecting on the NeurIPS 2019 conference, which ended on Saturday. It’s always a wonderful event, but this year I found it a bittersweet experience.
Bitter because the conference has grown so much that we no longer focus on a handful of ideas. I missed the feeling of a community coming together. I was excited about the progress in self-supervised learning. Others were buzzing about Bayesian networks and causality, federated learning in healthcare applications, or using DL to predict biological sequences such as proteins. These are fascinating areas, but it’s clear the AI community no longer marches to only one beat.
The sweet part is that NeurIPS is growing up. As Karen Hao wrote in MIT Technology Review, NeurIPS has matured from a venue with great science, hard partying, and wild dancing into a forum with great science and a focus on using AI for good. The AI community is getting better at diversity, inclusion, and taking responsibility for our actions, though there’s still room to grow.
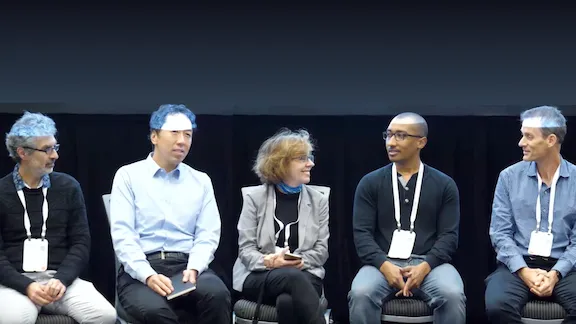
As part of the panel during the climate change workshop, I spoke about the importance of building an actionable ethical code for AI. Ideally written by the AI community, for the AI community. You can hear my remarks on that subject here at 1:15.
It was great fun speaking on the panel with Yoshua Bengio, Jeff Dean, Carla Gomes, and Lester Mackey. Thanks to David Rolnick, Priya Donti, Lynn Kaack, and others for organizing the great workshop.
Keep learning!
Andrew
News
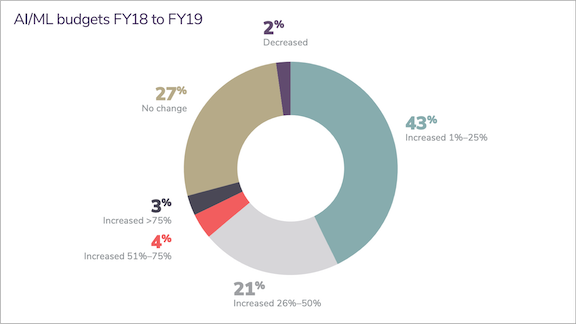
Deployment Gap
More and more companies are developing machine learning models for internal use. But many are still struggling to bridge the gap to practical deployments.
What’s new: Many companies haven’t figured out how to realize their AI ambitions, according to a report by Algorithmia, a marketplace for algorithms. Although AI budgets are on the rise, only 22 percent of companies using machine learning have successfully deployed a model, the study found.
What the report says: The 2020 State of Enterprise Report is based on a survey of nearly 750 people including machine learning practitioners, managers overseeing machine learning projects, and executives at large tech corporations.
- More than two-thirds of the subgroup that was asked about budgets reported increased spending on AI between 2018 and 2019 (see the graph above).
- Nonetheless, 43 percent of respondents cited difficulty scaling machine learning projects to their company’s needs, up 13 percent from last year’s survey.
- Half of respondents said their company takes between a week and three months to deploy a model. 18 percent said it takes from three months to a year.
Why it matters: AI is rapidly expanding into new applications and industries, and research is making tremendous strides. Yet building successful projects is still difficult. This report highlights both the great value of practical experience in the field and the need to establish effective practices and processes around designing, building, and deploying models.
We’re thinking: There’s a huge difference between building a Jupyter notebook model in the lab and deploying a production system that generates business value. AI as a field sometimes seems crowded but, in fact, it’s wide open to professionals who know what they’re doing.
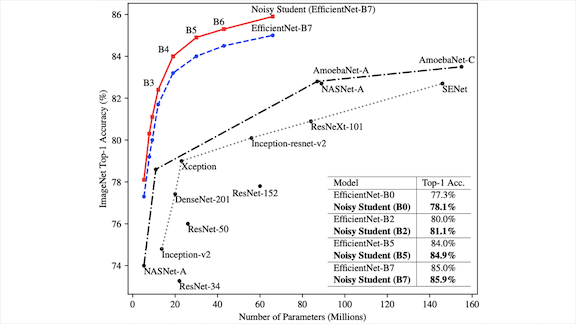
Self-Training for Sharper Vision
The previous state-of-the-art image classifier was trained on the ImageNet dataset plus 3.5 billion supplemental images from a different database. A new method achieved higher accuracy with one-tenth as many supplemental examples — and they were unlabeled, to boot.
What’s new: Qizhe Xie and a team at Google Brain plus Carnegie Mellon’s Eduard Hovy introduced a method they call Noisy Student, in which a model learns from another model in a teacher-student relationship. Noisy Student achieved better performance on ImageNet.
Key insight: In the learning approach known as self-training, a model that’s designated the teacher trains on labeled data and then generates pseudo-labels on unlabeled data. Then a student model trains on both the labeled data and pseudo-labeled data. Noisy Student adds two tweaks: The student network is larger than that of the teacher, and the student’s training data is adulterated with noise.
How it works: Both teacher and student use an EfficientNet architecture. The higher-capacity architecture is good for the student, which has more parameters and processes more data than the teacher.
- The teacher is trained on ImageNet’s training set. It then predicts pseudo labels for 300 million unlabeled images from Google’s private JFT dataset.
- The student training dataset consists of ImageNet’s training set plus the 130 thousand JFT images with the highest confidence predictions for each pseudo label class.
- During the student’s training, the algorithm applies data augmentation and also uses dropout and the stochastic depth method to perturb the model weights. These steps nudge the student to generalize beyond its teacher’s ability.
- The teacher-student training cycle can be repeated, treating each previous student as a new teacher.
Results: Noisy Student improved state-of-the-art accuracy on ImageNet as a whole and on specialized subsets. On ImageNet, it increased top-5 accuracy, meaning the true label was in the top five predictions, by 0.2 percent to 98.2 percent. Noisy Student also boosted the top-1 accuracy by 1 percent to 87.4 percent. Furthermore, it matched or exceeded previously established records for ImageNet A,C, and P, which are subsets that have been corrupted or perturbed or are commonly misclassified.
Why it matters: These results are another step forward for using unlabeled data to boost image classification accuracy.
We’re thinking: Unlabeled examples are far more plentiful than labeled datasets. Techniques like this may be key to enabling learning algorithms to exploit far more data than was possible before.
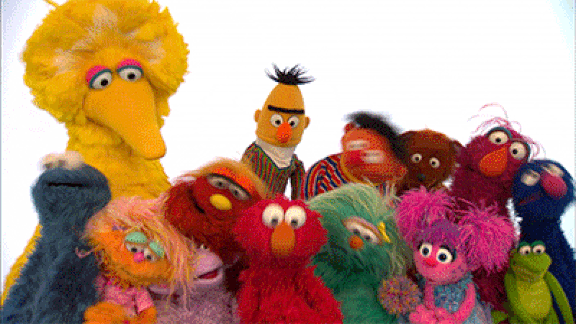
Inside AI’s Muppet Empire
As language models show increasing power, a parallel trend has received less notice: The vogue for naming models after characters in the children’s TV show Sesame Street.
What’s new: In a recent feature article, The Verge gets to the bottom of the Muppetware phenomenon.
How to get to Sesame Street: The trend encompasses tech giants including Google, Facebook, Baidu, and China’s prestigious Tsinghua University.
- It began in 2017 with ELMo (Embeddings from Language Models), a product of the Allen Institute for AI. Its creators liked the name’s whimsical flavor, and the model’s way with words garnered headlines in the tech press.
- The following year, researchers at Google debuted BERT (Bidirectional Encoder Representations from Transformers), acknowledging a debt to ELMo.
- Other researchers paid homage by perpetuating what had become an inside joke. Today, the Muppetware family includes Big BIRD, ERNIE, Kermit, Grover, RoBERTA, and Rosita.
- But what about GPT-2? The article reveals that OpenAI’s extraordinarily loquacious model was almost called Snuffleupagus. The researchers dropped the name because it wasn’t serious enough.
Why it matters: Muppet names are fun! But the article points out that they’re also memorable. They brand individual models for other researchers and elevate natural language processing for the broader public. Beyond that, the informal naming convention signifies the AI community’s collaborative attitude.
Behind the news: Sesame Street debuted in 1969 aiming to create TV that held children’s attention while also educating them. It was the first children’s show to base its episodes on research.
We’re thinking: This edition of The Batch was brought to you by the letters A and I.
A MESSAGE FROM DEEPLEARNING.AI
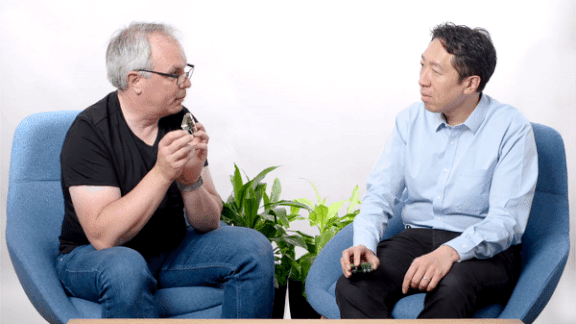
Want to deploy a TensorFlow model in your web browser, or on your smartphone? Course 1 and 2 of the deeplearning.ai TensorFlow: Data and Deployment Specialization will teach you how. Enroll now
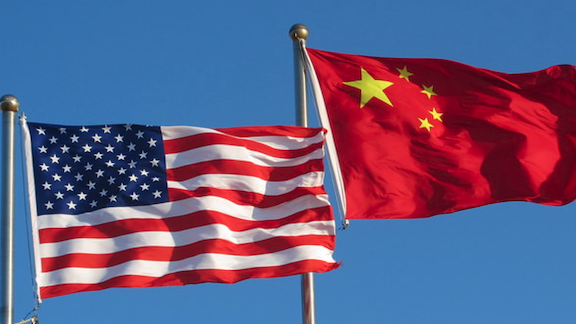
Competition or Cooperation?
Some politicians view international competition in AI as an arms race. That mindset could lead to escalating conflict, experts said.
What’s new: If global powers like the U.S. and China adopt a winner-take-all approach to AI, they will lose out on the benefits of international collaboration, Tim Hwang and Alex Pascal argue in Foreign Policy.
The analysis: The arms-race mentality springs primarily from the notion that autonomous weapons will prove to be a trump card in international conflicts, the authors say. This belief encourages nations to keep research and development to themselves, but the total benefit of collaboration is often greater than that of any particular initiative, they say.
- Both the U.S. and China would benefit from AI that targets issues like climate change, global health, and disaster response.
- Powerful nations can use cooperative projects to shape global norms and rules for AI.
- A national agenda that prioritizes AI for warfare is likely to divert funding from non-defense applications.
- Countries that undertake arms races tend to escalate conflict rather than tamp it down.
Behind the news: Scientific partnerships between the U.S. and USSR mitigated tensions during the Cold War era. In 1957, the rival nations agreed to send scientists to collaborate on projects. These cooperative relationships influenced diplomatic discussion and helped ease disagreements over issues like nuclear disarmament.
Why it matters: AI’s potential role in warfare is still unclear, and the technology is far from fully developed. The gap creates breathing room for national leaders to establish policies that will mutually benefit their own countries and the world at large. For instance, the National Security Commission on AI advocates that the U.S. engage with China and Russia to control military uses of AI.
We’re thinking: Electricity has uses in warfare, yet countries didn’t keep that technology to themselves, and the whole world is better off for it.
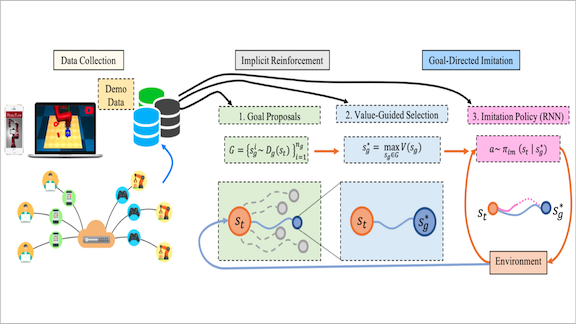
Different Skills From Different Demos
Reinforcement learning trains models by trial and error. In batch reinforcement learning (BRL), models learn by observing many demonstrations by a variety of actors. For instance, a robot might learn how to fix ingrown toenails by watching hundreds of surgeons perform the procedure. But what if one doctor is handier with a scalpel while another excels at suturing? A new method lets models absorb the best skills from each.
What’s new: Ajay Mandlekar and collaborators at Nvidia, Stanford, and the University of Toronto devised a BRL technique that enables models to learn different portions of a task from different examples. This way, the model can gain useful information from inconsistent examples. Implicit Reinforcement without Interaction at Scale (IRIS) achieved state-of-the-art BRL performance in three tasks performed in a virtual environment.
Key insight: Learning from demonstrations is a double-edged sword. An agent gets to see how to complete a task, but the scope of its action is limited to the most complete demonstration of a given task. IRIS breaks down tasks into sequences of intermediate subgoals. Then it performs the actions required to accomplish each subgoal. In this way, the agent learns from the best parts of each demonstration and combines them to accomplish the task.
How it works: IRIS includes a subgoal selection model that predicts intermediate points on the way to accomplishing an assigned task. These subgoals are defined automatically by the algorithm, and may not correspond to parts of a task as humans would describe them. A controller network tries to replicate the optimal sequence of actions leading to a given subgoal.
- The subgoal selection model is made up of a conditional variational autoencoder that produces a set of possible subgoals and a value function (trained via a BRL version of Q-learning) that predicts which next subgoal will lead to the highest reward.
- The controller is a recurrent neural network that decides on the actions required to accomplish the current subgoal. It learns to predict how demonstrations tend to unfold, and to imitate short sequences of actions from specific demonstrations.
- Once it’s trained, the subgoal selection model determines the next subgoal. The controller takes the requisite actions. Then the subgoal selection model evaluates the current state and computes a new subgoal, and so on.
Results: In the Robosuite’s lifting and pick-and-place tasks, previous state-of-the-art BRL approaches couldn’t pick up objects reliably, nor place them elsewhere at all. IRIS learned to pick up objects with over 80 percent success and placed them with 30 percent success.
Why it matters: Automatically identifying subgoals has been a holy grail in reinforcement learning, with active research in hierarchical RL and other areas. The method used in this paper applies to relatively simple tasks where things happen in a predictable sequence (such as picking and then placing), but might be a small step in an important direction.
We’re thinking: Batch reinforcement learning is useful when a model must be interpretable or safe — after all, a robotic surgeon shouldn’t experiment on living patients — but it hasn’t been terribly effective. IRIS could make it a viable option.
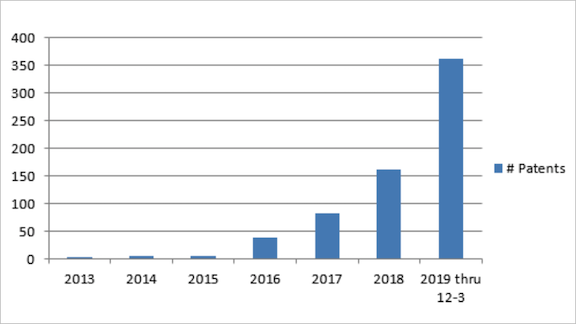
IP for AI
The number of patents issued for deep learning has doubled every year since 2013.
What’s new: Inventor, engineer, and lawyer Nick Brestoff tracks deep learning patents. He detailed his findings in a blog on InsideBigData and offers advice on how to get patent applications approved.
AI on the rise: Breston searches weekly for filings containing keywords including “deep learning,” “deep neural,” or “multi-layer neural.” He found that IBM holds the most deep learning patents (51), followed by Google (39) and Microsoft (28).
- Between 2013 and 2015, the Patent Office issued just three to four deep learning patents a year.
- In 2016, that number jumped to 36 and has more than doubled each year since. As of December 3, the agency had issued 361 deep learning patents in 2019.
- A change in the law helps explain the 2016 jump. That year, a federal court lowered the bar for approval of software patents.
Senior privilege: Inventors age 65 and over — even those listed as co-authors — can fast-track patent applications using a loophole called a Petition to Make Special. This trick has allowed Brestoff, who is 71 years old and holds eight patents on deep learning techniques, to complete the process in as little as three months, rather than the usual years-long wait. “There’s a wonderful advantage to having a knowledgeable senior on your innovation team,” he said.
We’re thinking: Patents have a bad name in some circles, because of patent trolls and frivolous lawsuits that have destroyed value and slowed down innovation. But we’re also not sure a world with no patents whatsoever would be one with more innovation.