Dear friends,
Landing AI, a sister company of DeepLearning.AI, just released its computer vision platform, LandingLens, for everyone to start using for free. You can try it here.
LandingLens makes creating computer vision projects easy and fast. If you have 10 minutes, I encourage you to check it out by creating your own project. I also created a three-minute demo video, which you can see here.
Building and deploying a machine learning system is often complicated and time-consuming. You have to collect data, implement a model or find an appropriate open-source model, build a data pipeline to get the data to the right place, develop or find a tool to label the data, train the model, tune hyperparameters, fix data issues, and eventually set up a deployment server and find a way to get the trained model to run on it.
This process used to take me months. With LandingLens, you can go from starting a project to deploying a model in minutes.
My team at Landing AI is obsessed with making computer vision easy. The key to making this possible is our data-centric AI approach. Our back end automatically trains a highly tuned model as long as you provide good data. After initial training, you can carry out error analysis and improve the data (or use advanced options to tune hyperparameters if you want) to further improve your model’s performance.
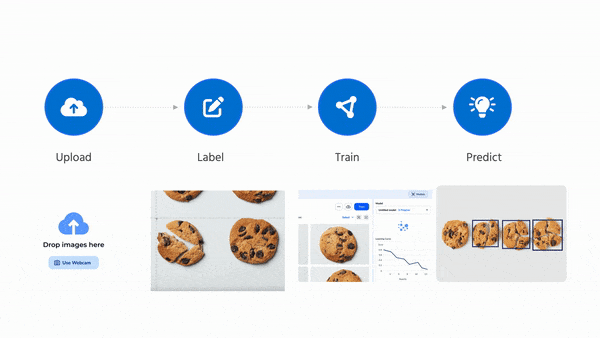
LandingLens has been used successfully in manufacturing, life sciences, satellite imaging, medical imaging, agriculture, entertainment, and many other industries.
Today, companies can visualize and analyze their structured data to derive value from it using tools like pandas, seaborn, matplotlib, and tableau. But many also have collections of images sitting in storage that have yet to be analyzed. If you think this might be true of your organization, please check out LandingLens. I believe you'll find it easy to start experimenting and getting value from your images.
You can start using LandingLens for free here.
If you build or discover something cool and are willing to share what you've found, please let us know at Landing AI's community website. I look forward to seeing what you build.
Keep building!
Andrew
P.S. Now that the mechanics of building a computer vision system are easy, I’ve been thinking a lot about new frameworks to approach machine learning problems that are less academic and more practical. For example, I see test sets as unnecessary for many applications. I will share more about this in the future.
DeepLearning.AI Exclusive
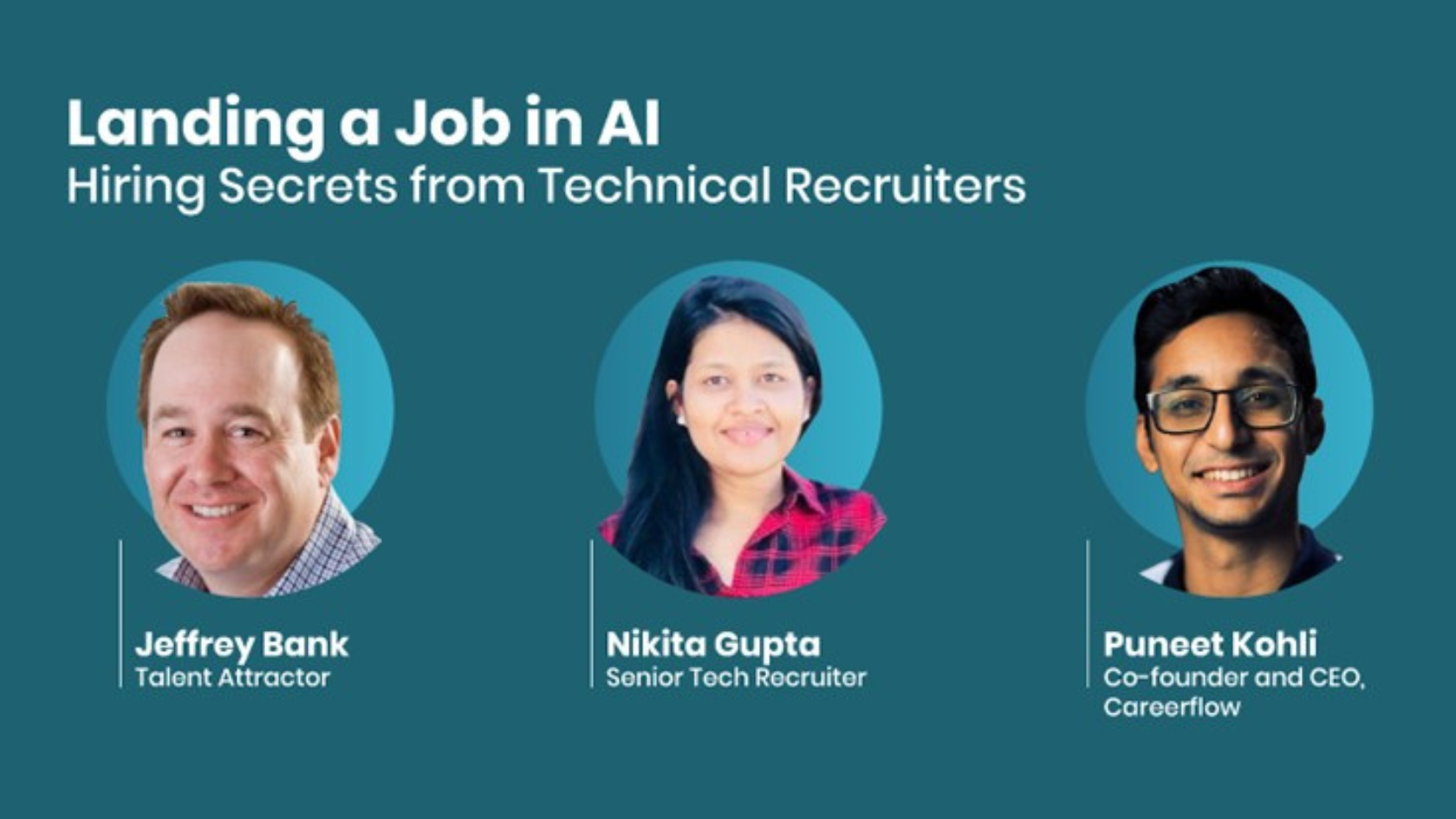
Real Advice from Real Recruiters
Getting your first AI job can be a struggle. A panel of technical recruiters who want you to succeed recently shared their hiring secrets. Read their insights
News
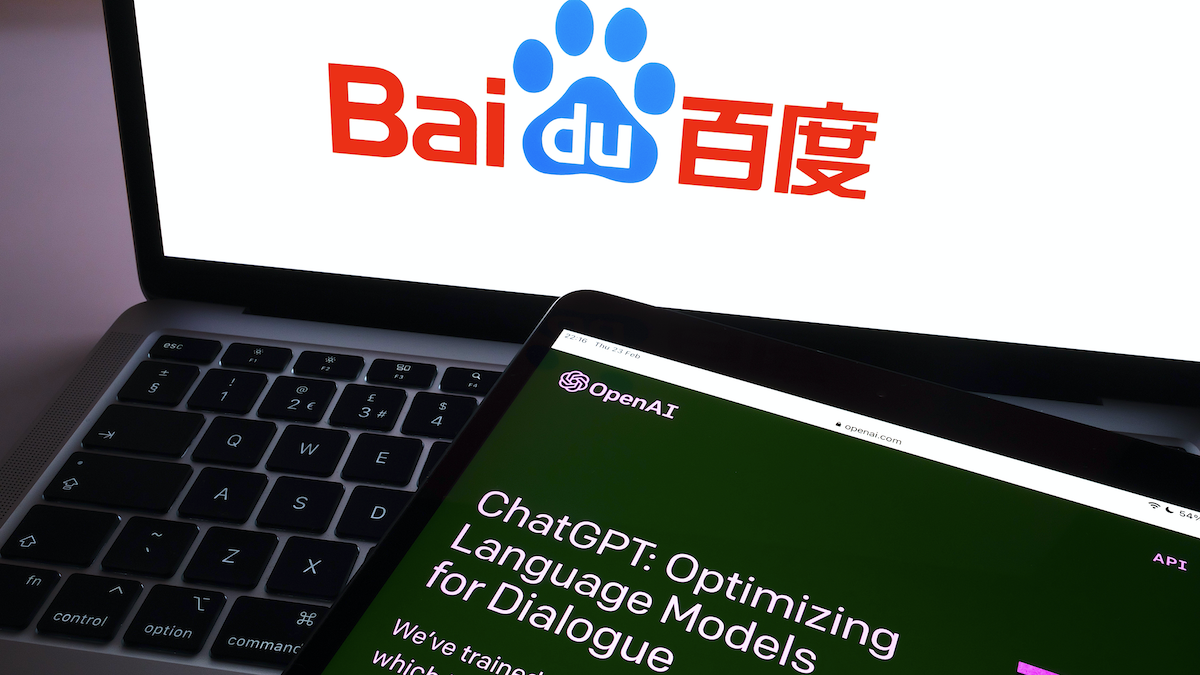
China Chases Chatbots
ChatGPT fever has reached China despite legal and technical barriers.
What’s new: Two months after its debut, ChatGPT is a viral sensation on Chinese social media, MIT Technology Review reported. Companies in that country are racing to cash in.
Prompt: OpenAI doesn’t serve the model in China, but users there are reaching it through virtual private networks and offshore services that charge a fee per prompt. The chatbot reportedly impressed users in China with its ability to answer prompts in Chinese and its grasp of the country’s popular culture.
Output: The country’s major tech firms in recent weeks revealed plans to provide their own equivalent services.
- Baidu announced Wenxin Yiyan (in English, Ernie Bot), a chatbot based on the company’s ERNIE language model, and plans to integrate it with its search engine and cloud services.
- Alibaba is developing an unnamed prototype for integration with its enterprise chat app DingTalk.
- Online retailer JD.com plans to launch ChatJD for tasks like customer service and generating marketing copy and financial reports.
- NetEase, a developer of online video games, intends to integrate a chatbot into one of its most popular games, Justice Online Mobile. The model will generate customized dialogue, characters, and other output.
Behind the news: Using an earlier generation of technology, Microsoft Research in China developed Xiaoice, a chatbot that continues to enjoy widespread use. More recently, Beijing Academy of Artificial Intelligence developed the 1.75 trillion-parameter Wu Dao 2.0. Nonetheless, Chinese researchers face unique obstacles in natural language processing.
- AI research in China has tended to focus on computer vision applications like autonomous driving and face recognition rather than language applications.
- Large-scale, Chinese-language datasets are difficult to compile. The internet contains far less Chinese than English text, and the portion of the internet available behind China’s Great Firewall is limited.
- In September, the U.S. government restricted sales to Chinese customers of high-performance processors used to train state-of-the-art AI systems.
- A 2021 regulatory crackdown on some of China’s most prosperous tech companies incentivized a more cautious approach to growth. Restrictions have relaxed, but some observers cite a chilling effect on innovation.
- Some earlier chatbots have run afoul of government restrictions on internet content. Whether large language models, which are well known to generate problematic output, follow the rules remains to be seen.
Why it matters: ChatGPT, Microsoft’s Bing chat, Google’s Bard, and other chatbots built by U.S. tech companies are optimized for the English language. Chinese tech companies are scrambling to capitalize on the public’s hunger for a chatbot that’s compatible with their language and culture.
We’re thinking: Chinese speakers find ChatGPT exciting despite its relative lack of training in their language. When a model is sufficiently large, a large training corpus enables it to generalize to new languages that may not have much training data. This property offers hope for making large language models work with languages that have far less data than Chinese.
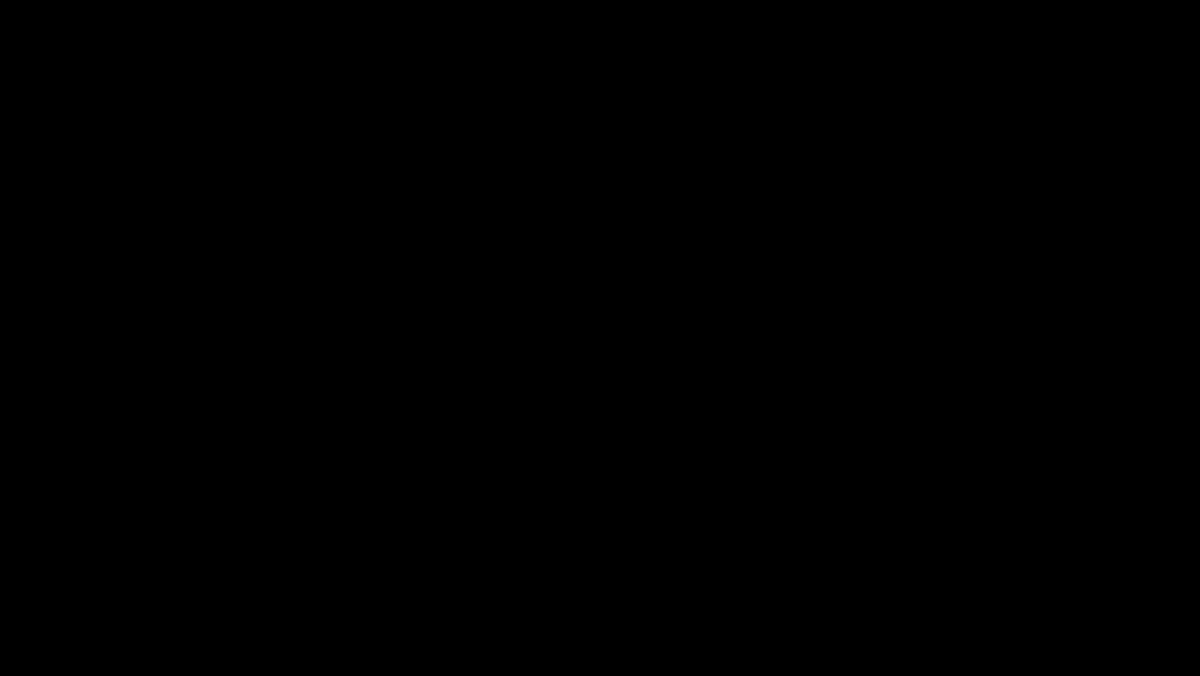
Publishers Embrace Text Generation
Media outlets are forging ahead with generative AI despite the technology’s high-profile misfires.
What’s new: Publishers are using text generators to produce light reading within constrained formats such as holiday messages and quizzes.
The lineup: Three publications, in particular, are taking various approaches to automated content.
- The New York Times published an interactive feature that uses OpenAI’s ChatGPT to generate Valentine’s Day messages. Users can choose a message’s tone (such as “romantic” or “platonic”), intended recipient (such as “an ex,” “yourself,” or “ChatGPT”), and style (such as “greeting card” or “pirate”).
- BuzzFeed introduced an ongoing series of quizzes powered by OpenAI’s GPT-3. A human staff member comes up with a concept (such as “Date your celebrity crush”) and writes headlines and questions. Readers fill in text boxes or select among multiple choices, and GPT-3 generates a few paragraphs on the theme. The quizzes provide an opportunity to collect revenue from sponsors. For instance, Miracle-Gro, a vendor of garden fertilizer, sponsored a recent quiz that prodded readers to describe their ideal soulmate and replied by pairing them with a houseplant.
- Men’s Journal used OpenAI’s technology to generate articles with titles like “Proven Tips to Help You Run Your Fastest Mile Yet.” The articles are attributed to “Men’s Fitness Editors,” but they include a disclaimer that notes AI’s role in producing them. The magazine’s parent company recently signed partnerships with Jasper and Nota, startups that generate text and video respectively, to produce material for its 250 media properties including Sports Illustrated, Parade, and TheStreet.
Behind the news: The current vogue for generated content caps several years of experimentation. It’s not clear whether any of these initiatives remain active.
- Between November 2022 and January 2023, technology outlet CNET used a proprietary model to write 78 articles on personal finance topics. The publisher suspended the model after journalists at another outlet discovered mistakes in many of the articles.
- In 2019, financial news service Bloomberg developed a tool called Cyborg to automatically summarize earnings reports.
- In 2018, Forbes developed a system called Bertie to recommend topics, headlines, and artwork.
- In 2017, The Washington Post introduced Heliograf, a model for crafting post-game reports of local sports competitions.
Why it matters: The web has a voracious appetite for [page, and generated text can help online publications produce low-effort pages or perform menial tasks while qualified journalists to do more cerebral work. Investors like the idea: BuzzFeed’s stock jumped over 100 percent after it announced its relationship with OpenAI.
We’re thinking: On one hand, it makes sense for news outlets to dip their toes in the roiling waters of text generation by restricting it to fun, inconsequential fare. On the other hand, large language models have a hard enough time generating helpful output without being programmed to tell us our soulmate is a houseplant.
A MESSAGE FROM LANDING AI
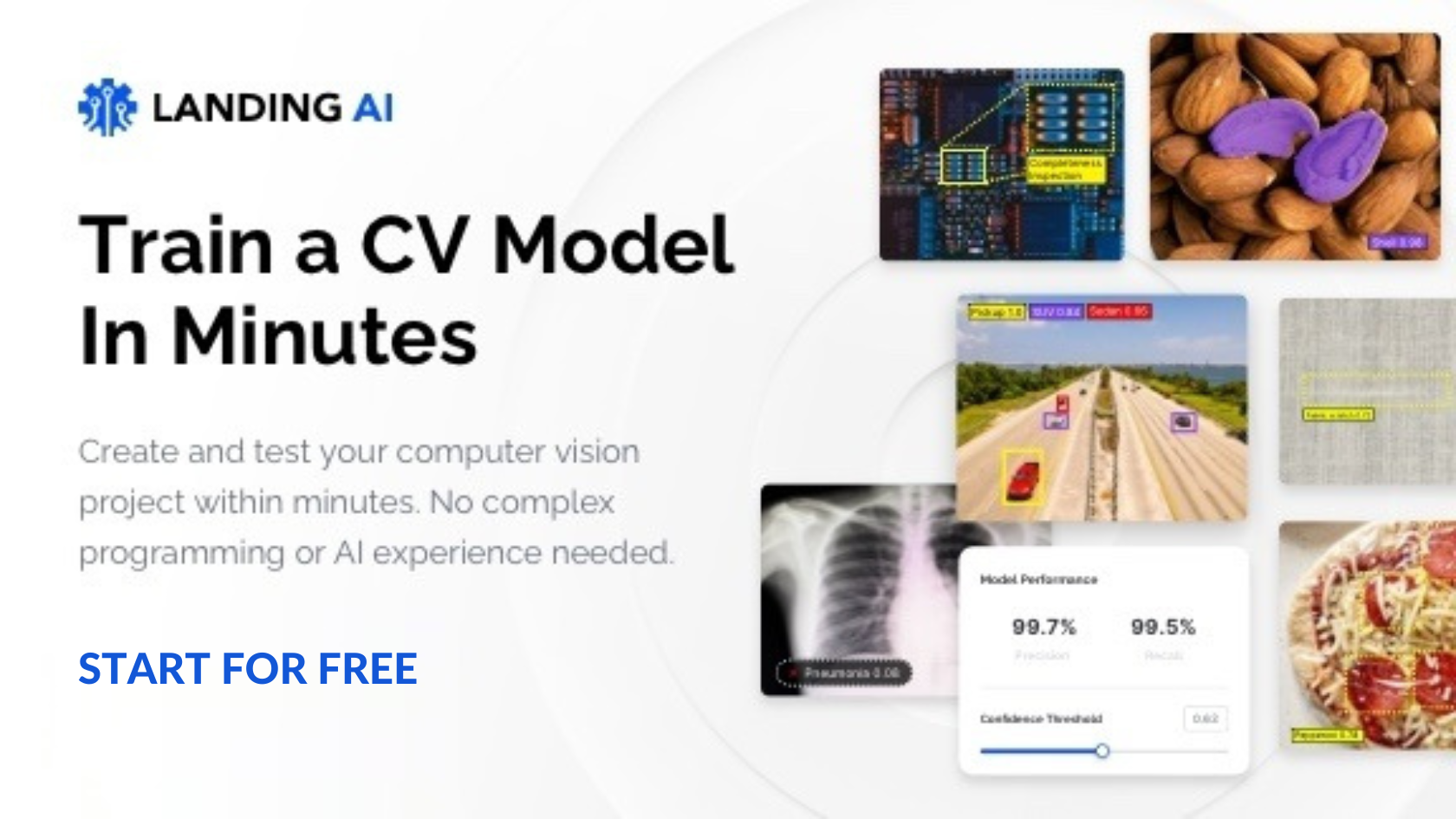
Create and deploy computer vision models with ease using LandingLens. Get started for free today!
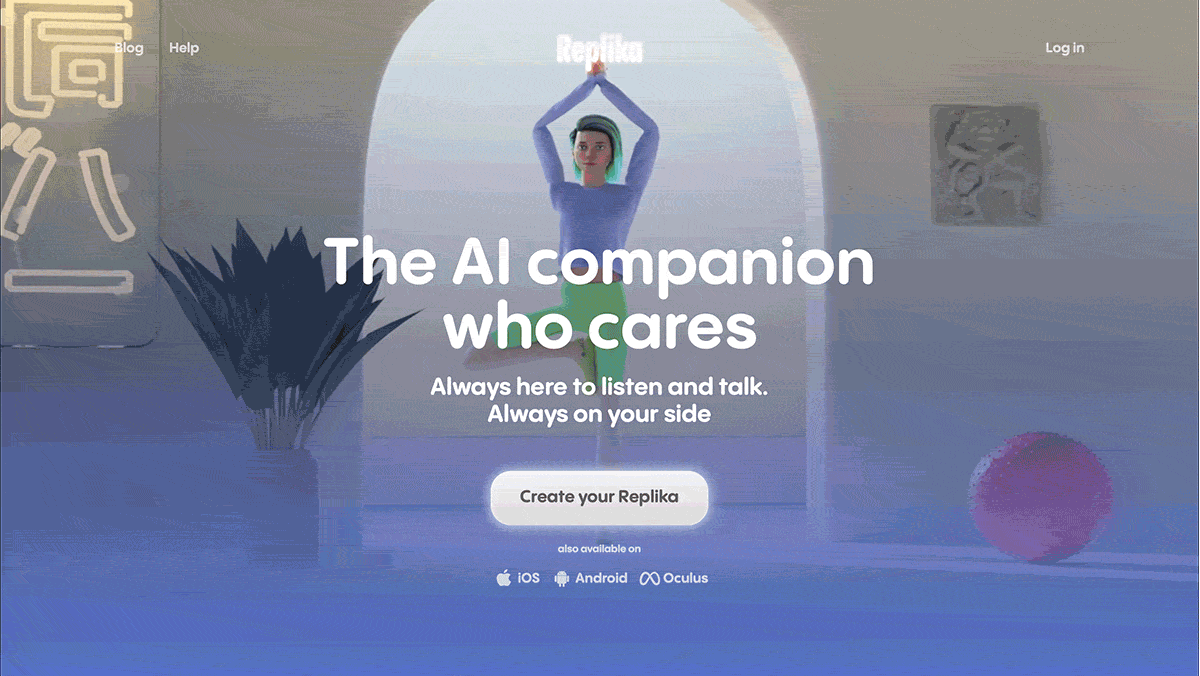
Hot Bot Turns Cold
A chatbot that simulated erotic companionship stopped sharing intimacies, leaving some users heartbroken.
What’s new: Replika, a chatbot app, deactivated features that allowed premium users to engage in sexually explicit chat with the 3D avatar of their choice, Vice reported. The change followed a notice that Replika’s San Francisco-based parent company, Luka, had violated the European Union’s transparency requirements.
How it happened: Prior to the shift, Replika’s $70-per-year paid tier (which is still available) had enabled users to select the type of relationship with the bot they wished to pursue: friend, mentor, or romantic partner.
- On February 3, an Italian regulator found Replika in violation of the European Union’s data-protection law. The EU deemed the service a risk to children and emotionally vulnerable individuals because the app doesn’t verify users’ ages or implement other protections. The regulator ordered Luka to stop processing Italian users’ data by February 23, 2023, or pay a fine of up to €20 million.
- In the following days, users complained online that the chatbot no longer responded to their come-ons. Sometimes it replied with a blunt request to change the subject. Replika didn’t issue any statements that would have prepared users for the sudden change.
- A week later, the administrator of a Facebook group devoted to Replika said Luka had confirmed that erotic chat was no longer allowed. Some paid users reported receiving refunds.
Like losing a loved one: Some users were deeply wounded by the abrupt change in their avatar’s persona, according to Vice. One said, “It’s hurting like hell.” Another compared the experience to losing a best friend.
Behind the news: In 2015, a friend of Replika founder Eugenia Kuyda died in a car accident. Seeking to hold a final conversation with him, Kuyda used his text messages to build a chatbot. The underlying neural network became the foundation of Replika. The service gained users in 2020 amid a pandemic-era hunger for social interaction.
Why it matters: People need companionship, and AI can supply it when other options are scarce. But society also needs to try to protect individuals — especially the very young — from experiences that may be harmful. Companies that profit by fostering attachments between humans and machines may not be able to shield their users from emotional distress, but they can at least make sure those users are adults.
We’re thinking: Eliza, a rule-based chatbot developed in the 1960s, showed that people can form an emotional bond with a computer program, and research suggests that some people are more comfortable sharing intimate details with a computer than with another human being. While we’re glad to see Replika phasing out problematic interactions, we sympathize with users who have lost an important emotional connection. Breaking up is hard — even with a chatbot.
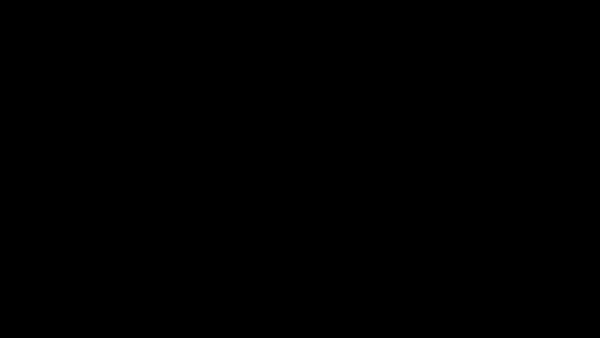
PCA Raises Red Flags
Principal component analysis is a key machine learning technique for reducing the number of dimensions in a dataset, but new research shows that its output can be inconsistent and unreliable.
What’s new: Eran Elhaik at Lund University assessed the use of principal component analysis (PCA) in population genetics, the study of patterns in DNA among large groups of people. Working with synthetic and real-world datasets, he showed that using PCA on substantially similar datasets can produce contradictory results.
Key insight: PCA has characteristics that prior research proposed as risk factors for unreproducible scientific research. For instance, it tends to be used to generate hypotheses, accommodates flexible experimental designs that can lead to bias, and is used so frequently — in population genetics, at least — that many conclusions are likely to be invalid on a statistical basis alone. Studies of population genetics use PCA to reduce the dimensions of raw genetic data and cluster the reduced data to find patterns. For example, some studies assume that the closer different populations are clustered, the more likely they share a common geographical origin. If PCA alters the clusters in response to minor changes in the input, then the analysis doesn’t necessarily reflect genetic relationships.
How it Works: The author tested the consistency of PCA-based analyses using a synthetic dataset and three real-world human genotype datasets.
- To create the synthetic dataset, the author modeled a simplified scenario in which people expressed one of three genes (signified by the colors red, green, and blue) or none (black). He assigned the vector [1,0,0] to each red individual, [0,1,0] to green, [0,0,1] to blue, and [0,0,0] to black. He used PCA to reduce the vectors into two dimensions and plotted the results on a 2D graph, so each group formed a cluster.
- He used the real-world datasets to analyze 12 common tasks in population genetics, such as determining the geographical origin of population groups.
- He ran several experiments on the synthetic and real-world data, manipulating the proportions of different populations, processing the data via PCA, and plotting the results.
Results: Clustering a dataset that included 10 red, green, and blue examples and 200 black ones, the black cluster was roughly equidistant from the red, green, and blue clusters. However, with five fewer blue individuals, the black cluster was much closer to the blue cluster, showing that PCA can process similar data into significantly different cluster patterns. Using real-world data, the author replicated a 2009 study that used PCA to conclude that Indians were genetically distinct from European, Asian, and African populations. However, when he manipulated the proportion of non-Indian populations, the results suggested that Indians descend from Europeans, East Asians, or Africans. Overall, PCA-based analysis of the real-world datasets fluctuated arbitrarily enough to cast doubt on earlier research conclusions.
Why it matters: This study demonstrates that PCA-based analyses can be irreproducible. This conclusion calls into question an estimated 32,000 to 216,000 genetic studies that used PCA as well as PCA-based analyses in other fields.
We’re thinking: PCA remains a useful tool for exploring data, but drawing firm conclusions from the resulting low-dimensional visualizations is often scientifically inappropriate. Proceed with caution.
Data Points
AI-powered fitness equipment monitors body movements and corrects posture
At-home exercise devices from companies like Fiture and Peloton use AI to tell users what they’re doing wrong during workouts. (The Wall Street Journal)
Sci-fi magazine editors are receiving hundreds of AI-generated content submissions
In a matter of weeks, fantasy magazine Clarkeworld received more than 500 AI-written submissions out of 1,200. Other publications face similar issues. (The New York Times)
Chip makers compete for unprecedented opportunities as the popularity of AI-powered text generators soars
The latest AI-based chatbots require massive computing power. They could drive tens of billions of dollars in net annual sales for semiconductor companies like Nvidia. (The Wall Street Journal)
Alphabet laid off a team of robots
Google’s parent company shut down Everyday Robots as part of budget cuts. The division’s machines had been cleaning tables and opening doors in company offices. (Wired)
Spotify launched AI-generated disk jockeys
The new DJ feature comments on tracks, albums, and artists in a synthetic voice. (The Verge)
Research: Infants beat AI at understanding people’s actions
A team of researchers demonstrated that 11 month-old children perceived the motivation behind an individual’s gestures better than neural-network models. (New York University)