Dear friends,
ChatGPT has raised fears that students will harm their learning by using it to complete assignments. Voice cloning, another generative AI technology, has fooled people into giving large sums of money to scammers, as you can read below in this issue of The Batch. Why don’t we watermark AI-generated content to make it easy to distinguish from human-generated content? Wouldn’t that make ChatGPT-enabled cheating harder and voice cloning less of a threat? While watermarking can help, unfortunately financial incentives in the competitive market for generative AI make their adoption challenging.
Effective watermarking technology exists. OpenAI has talked about developing it to detect text produced by ChatGPT, and this tweet storm describes one approach. Similarly, a watermark can be applied invisibly to generated images or audio. While it may be possible to circumvent these watermarks (for instance, by erasing them), they certainly would pose a barrier to AI-generated content that masquerades as human-generated.
Unfortunately, I’m not optimistic that this solution will gain widespread adoption. Numerous providers are racing to provide text-, image-, and voice-generation services. If one of them watermarks its output, it will risk imposing on itself a competitive disadvantage (even if it may make society as a whole better off).
For example, assuming that search engines downranked AI-generated text, SEO marketers who wanted to produce high-ranking content would have a clear incentive to make sure their text wasn’t easily identifiable as generated. Similarly, a student who made unauthorized use of a text generator to do their homework would like it to be difficult for the teacher to find out.
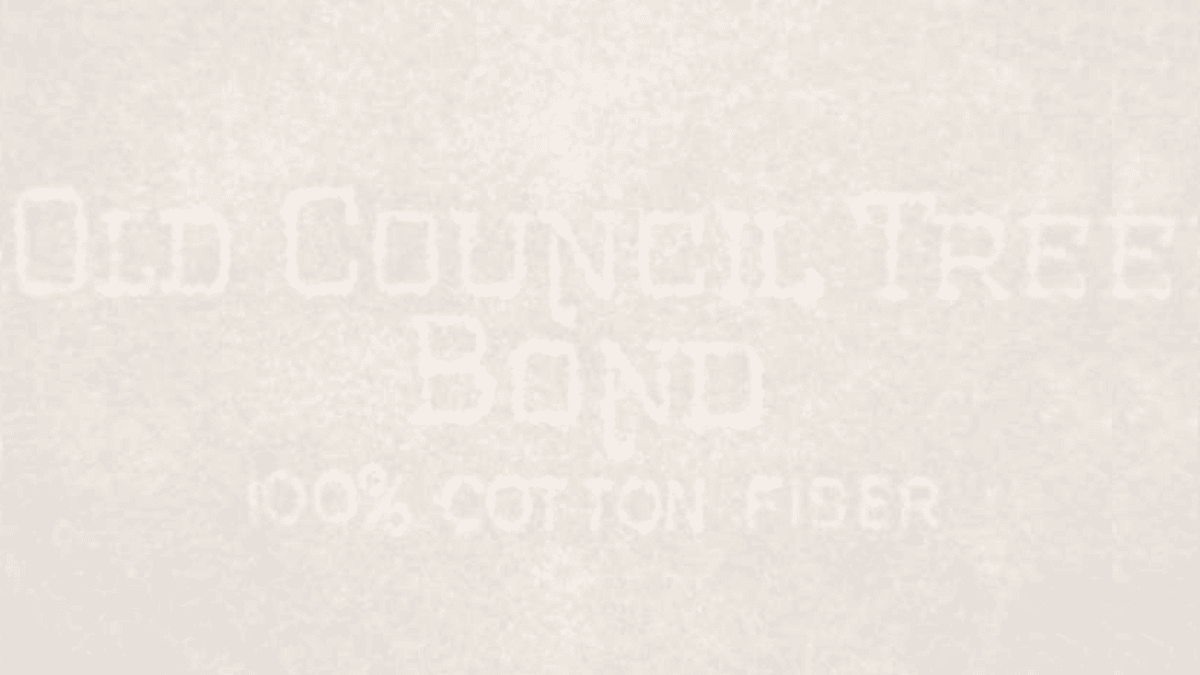
Even if a particular country were to mandate watermarking of AI-generated content, the global nature of competition in this market likely would incentivize providers in other countries to ignore that law and keep generating human-like output without watermarking.
Some companies likely will whitewash these issues by talking about developing watermarking technology without actually implementing it. An alternative to watermarking is to use machine learning to classify text as either AI- or human-generated. However, systems like GPTzero that attempt to do so have a high error rate and don’t provide a robust solution.
If one company were to establish a monopoly or near-monopoly, then it would have the market power to implement watermarking without risking losing significant market share. Given the many downsides of monopolies, this is absolutely not the outcome we should hope for.
So what’s next? I think we’re entering an era when, in many circumstances, it will be practically impossible to tell if a piece of content is human- or AI-generated. We will need to figure out how to re-architect both human systems such as schools and computer systems such as biometric security to operate in this new — and sometimes exciting — reality. Years ago when Photoshop was new, we learned what images to trust and not trust. With generative AI, we have another set of discoveries ahead of us.
Keep learning!
Andrew
DeepLearning.AI Exclusive
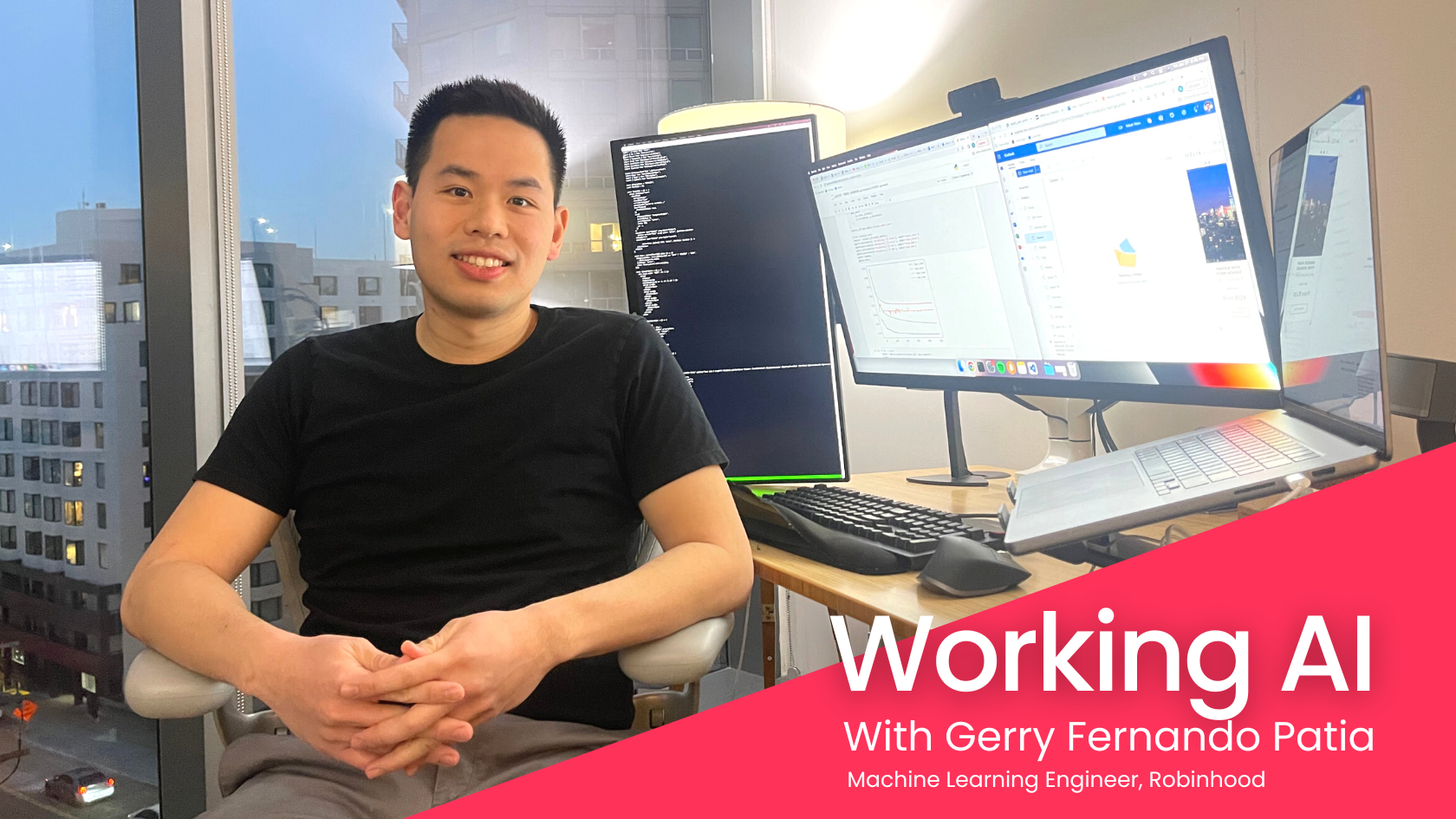
Working AI: Hackathon Hero
Gerry Fernando Patia didn’t come from a privileged background or attend a big-name university. So how did he land at Facebook right out of school? Read his story and learn how he used hackathons to attract recruiters.
News
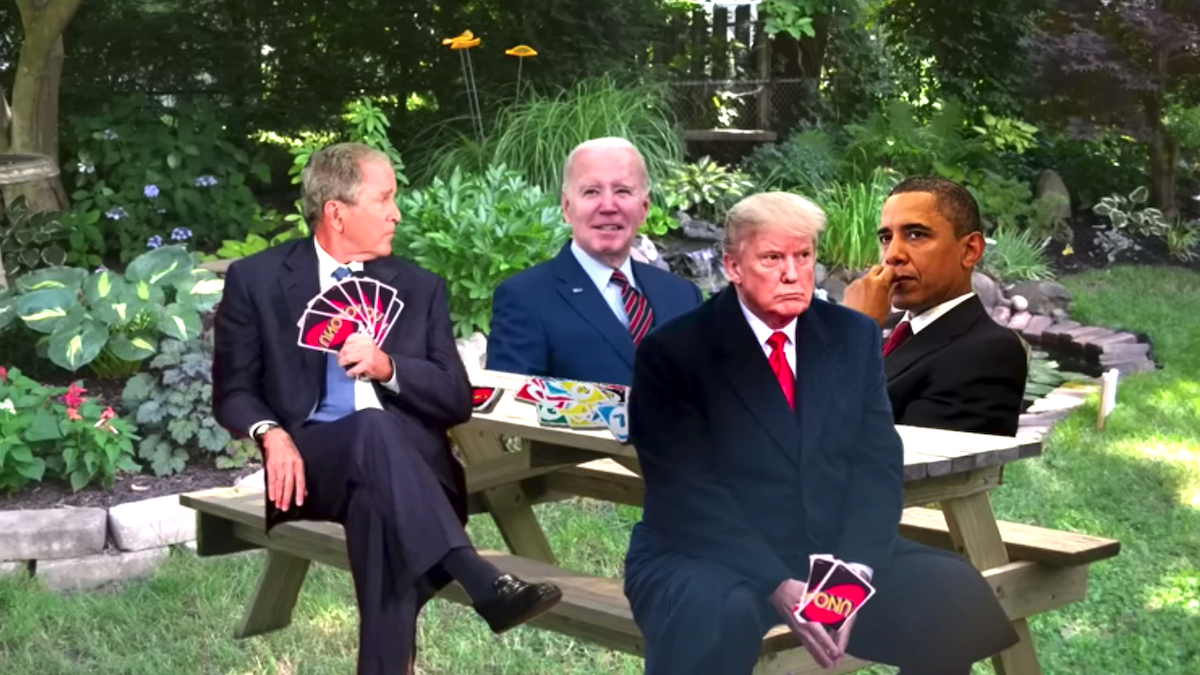
Voice Clones Go Viral
Tired of rap battles composed by ChatGPT? Get ready for the next wave of AI-generated fun and profit.
What’s new: Cloned voices are taking center stage in productions by upstart creators and monied corporations alike.
How it works: Companies including ElevenLabs, Resemble AI, Respeecher, and Play.ht recently launched free services that clone a speaker’s voice from brief samples. Such offerings unleashed a chorus of generated voices.
- YouTube creators attracted hundreds of thousands of viewers to videos that purportedly capture the voices of recent U.S. presidents arguing over a card game, playing Minecraft, and debating Pokemon.
- Athene AI Show, a fictional talk show that streams nonstop on Twitch, accepts interview questions provided by viewers in the chat channel. Generated voices of celebrities or fictional characters answer in a generated conversation with the host (an Internet personality named Athene). The channel has over 16,000 followers.
- Musician David Guetta, using unspecified text- and voice-generation models available on the web, synthesized lines in the style of Eminem “as a joke.” He played it during a live performance and “people went nuts!”
- Music-streaming service Spotify launched an “AI DJ” that generates bespoke playlists for users punctuated by commentary in the cloned voice of Xavier Jernigan, the company’s Head of Cultural Partnerships. Sonantic AI, a startup that Spotify acquired last year, supplied the synthesized voice, which intones a combination of human-written words and text generated by an unspecified model from OpenAI.
Yes, but: The democratization of voice cloning opens doors to criminals and pranksters.
- Scammers conned their victims out of money by mimicking voices of their relatives asking for money.
- A Vice reporter used ElevenLabs to clone his own voice. The facsimile was convincing enough to enable him to access his bank account.
- 4Chan users used ElevenLabs’ technology to generate hate speech in synthesized celebrity voices.
- ElevenLabs responded to the deluge of fake voices by verifying user identities, identifying clones, and banning accounts that abuse its services.
Why it matters: Voice cloning has entered the cultural mainstream facilitated by online platforms that offer AI services free of charge. Images, text, and now voices rapidly have become convincing and accessible enough to serve as expressive tools for media producers of all sorts.
We’re thinking: With new capabilities come new challenges. Many social and security practices will need to be revised for an era when a person’s voice is no longer a reliable mark of their identity.
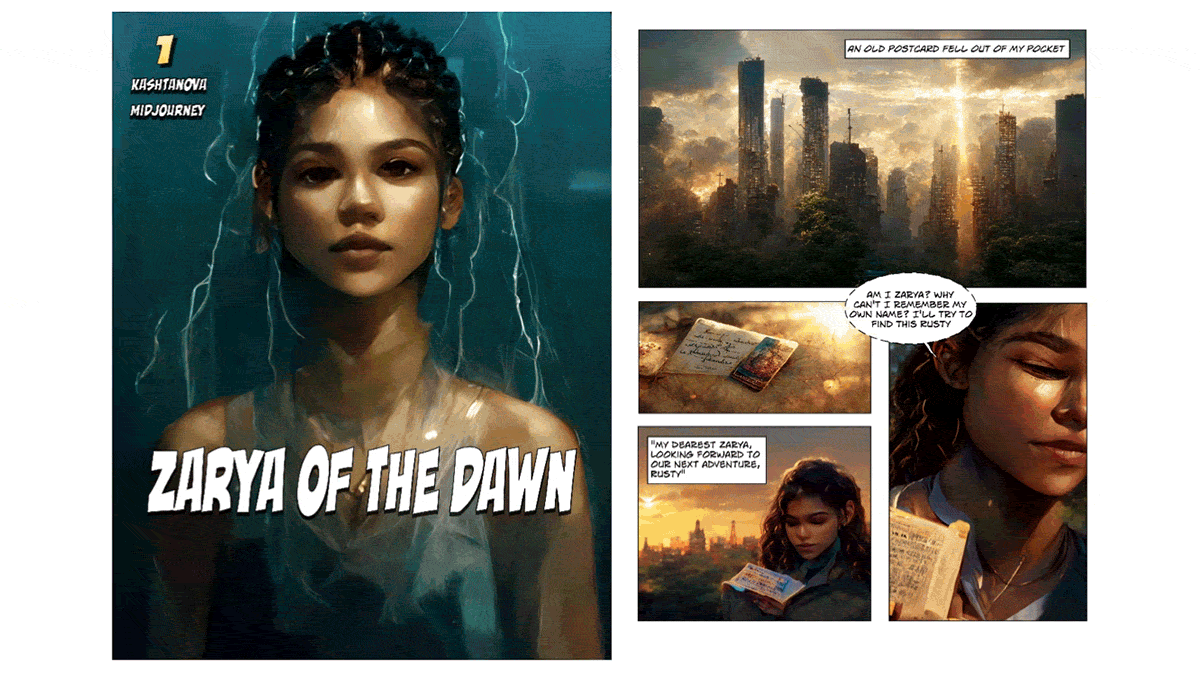
No Copyright for Generated Images
The output of AI-driven image generators is not protected by copyright in the United States.
What’s new: The U.S. Copyright Office concluded that copyright does not apply to images generated by the image generator Midjourney.
Split decision: In September, 2022, the agency granted a copyright for the comic book Zarya of the Dawn. The following month, however, it alerted author Kris Kashtanova of their intent to cancel the copyright after they learned from the author’s social media posts that Midjourney had produced the images. Kashtanova appealed the decision, and the agency revised its decision by granting a copyright for the text and arrangement of the images on its pages.
Humans versus machines: The agency explained its rationale:
- The Copyright Office’s code of practices state that it “will refuse to register a claim if it determines that a human being did not create the work.” (Remember the battle over the famous monkey selfie?) An 1884 U.S. Supreme Court decision defined a work’s copyright holder as its “inventive or master mind.”
- Users can’t control Midjourney’s output. In this way, the model differs from “human-guided” hardware like cameras or software like Adobe Photoshop whose output is subject to copyright.
- Even if Kashtanova had expended great effort writing prompts, the author had not created the images.
- Kashtanova subsequently edited the images using Photoshop, but the alterations were too small to affect the works’ eligibility for copyright.
Mixed results: Kashtanova said the agency’s decision to protect the text and layout was “great news” but vowed to continue lobbying for copyright protection of the images as well.
Yes, but: Different countries are likely to decide such issues differently, creating potential conflicts as intellectual property moves over the internet. While the U.S. has denied protection for intellectual property created by AI, in 2021 South Africa issued a patent that names an AI system as the inventor of a food container with unique properties.
Why it matters: Who owns the output of generative AI models? No one — in the U.S., at least. This decision is bound to influence business strategies throughout the publishing and creative communities as generated text, images, video, sound, and the like proliferate.
We’re thinking: It takes imagination and skill to generate a satisfying picture using Midjourney including envisioning an image, composing an effective prompt, and following a disciplined process over multiple attempts. Denying the creativity, expertise, and contribution of people who use AI as a creative tool strikes us as a mistake.
A MESSAGE FROM WORKERA
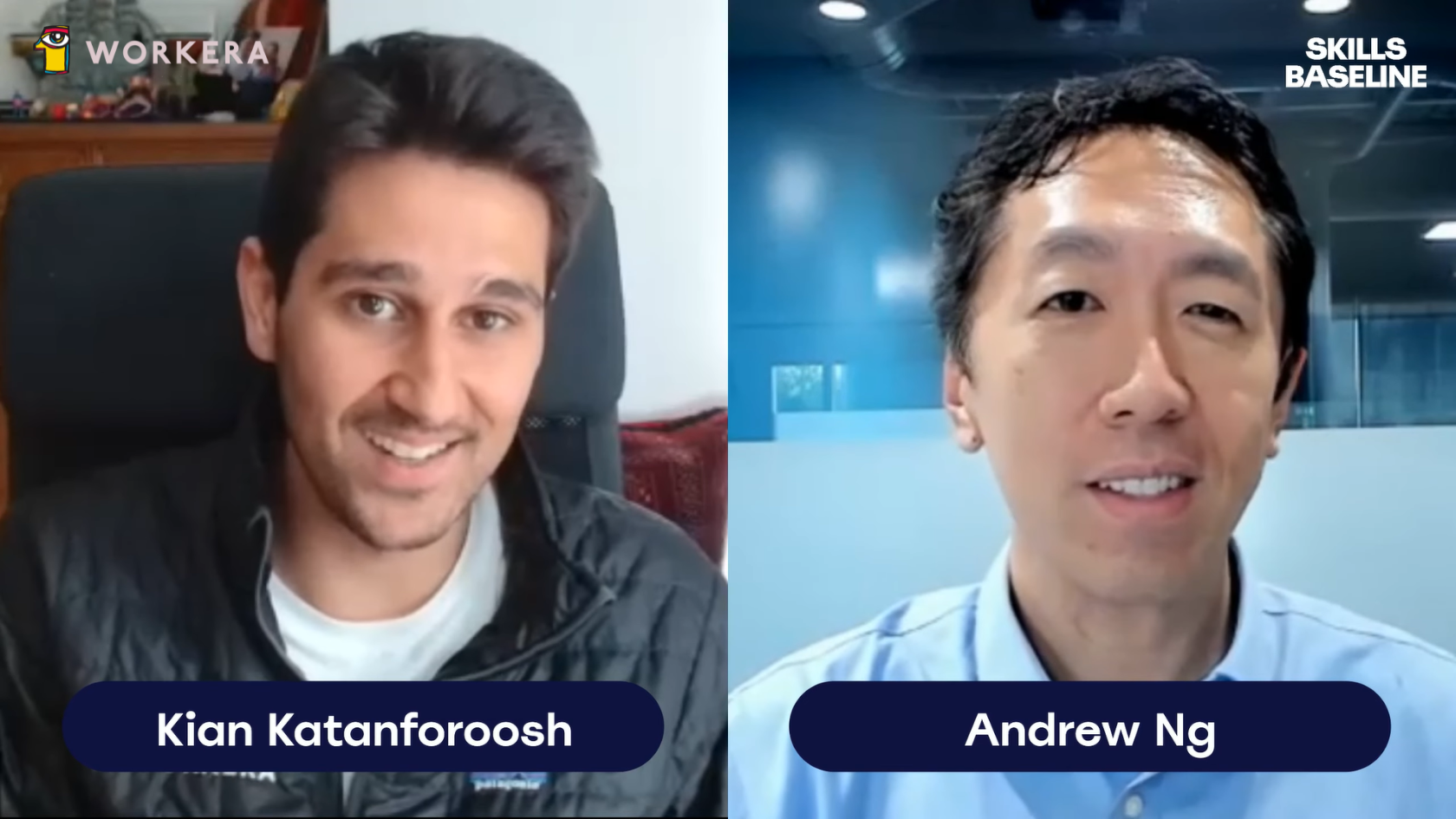
Andrew Ng talks with Workera CEO Kian Katanforoosh about upskilling in machine learning and how he hires world-class AI teams in the newest episode of Workera’s Skills Baseline podcast. Watch it here
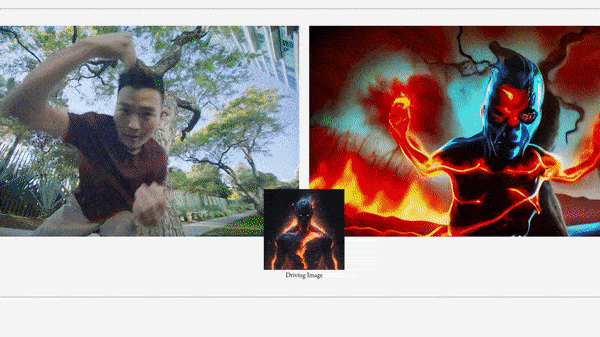
Text-Driven Video Alteration
On the heels of systems that generate video directly from text, new work uses text to adjust the imagery in existing videos.
What’s new: Patrick Esser and colleagues at Runway unveiled Gen-1, a system that uses a text prompt or image to modify the setting (say, from suburban yard to fiery hellscape) or style (for instance, from photorealism to claymation) of an existing video without changing its original shapes and motions. You can see examples and request access here.
Key insight: A video can be considered to have what the authors call structure (shapes and how they move) and content (the appearance of each shape including its color, lighting, and style). A video generator can learn to encode structure and content in separate embeddings. At inference, given a clip, it can replace the content embedding to produce a video with the same structure but different content.
How it works: Gen-1 generates video frames much like a diffusion model, and the authors trained it following the typical diffusion-model training procedure: Add to each training example varying amounts of noise — nearly up to 100 percent — then train the model to remove it. To generate a video frame, the model starts with 100 percent noise and, guided by a text prompt or image, removes it over several steps. The system used three embeddings: (i) a frame embedding for each video frame (to which noise was added and removed), (ii) a structure embedding for each video frame, and (iii) a content embedding for the entire clip. The dataset comprised 6.4 million eight-frame videos and 240 million images, which the system treated as single-frame videos.
- During training, given an input video, the encoder component of a pretrained autoencoder produced a frame embedding for each video frame. The authors added a consistent amount of noise to each frame embedding.
- Given a video frame, a pretrained MiDaS extracted a depth map, an image that outlines shapes without colors — in other words, the video frame’s structure. The encoder embedded the depth map to produce a structure embedding for each frame.
- Given one video frame selected at random, a pretrained CLIP, which maps corresponding text and images to the same embedding, created a content embedding. The authors used a single content embedding for the entire video, rather than one for each frame, to ensure that it didn’t determine the structure of each frame.
- Given the frame embeddings (with added noise), structure embeddings, and single content embedding, a modified U-Net learned to estimate the added noise.
- At inference, CLIP received a text prompt or image and generated its own embedding. This replaced the content embedding. For each video frame to be generated, the system received a random — that is, 100 percent noise — frame embedding. Given the noisy frame embeddings, the structure embeddings, and CLIP’s embedding, the U-Net removed the noise over several steps.
- Given the denoised embeddings, the decoder constructed the video frames.
Results: Five human evaluators compared Gen-1 to SDEdit, which alters each frame individually. Testing 35 prompts, the evaluators judged Gen-1’s output to better reflect the text 75 percent of the time.
Why it matters: Using different embeddings to represent different aspects of data gives Gen-1 control over the surface characteristics of shapes in a frame without affecting the shapes themselves. The same idea may be useful in manipulating other media types. For instance, MusicLM extracted separate embeddings for large-scale composition and instrumental details. A Gen-1-type system might impose one musical passage’s composition over another’s instruments.
We’re thinking: Gen-1 doesn’t allow changes in objects in a frame, such as switching the type of flower in a vase, but it does a great job of retaining the shapes of objects while changing the overall scenery. The authors put this capability to especially imaginative use when they transformed books standing upright on a table into urban skyscrapers.
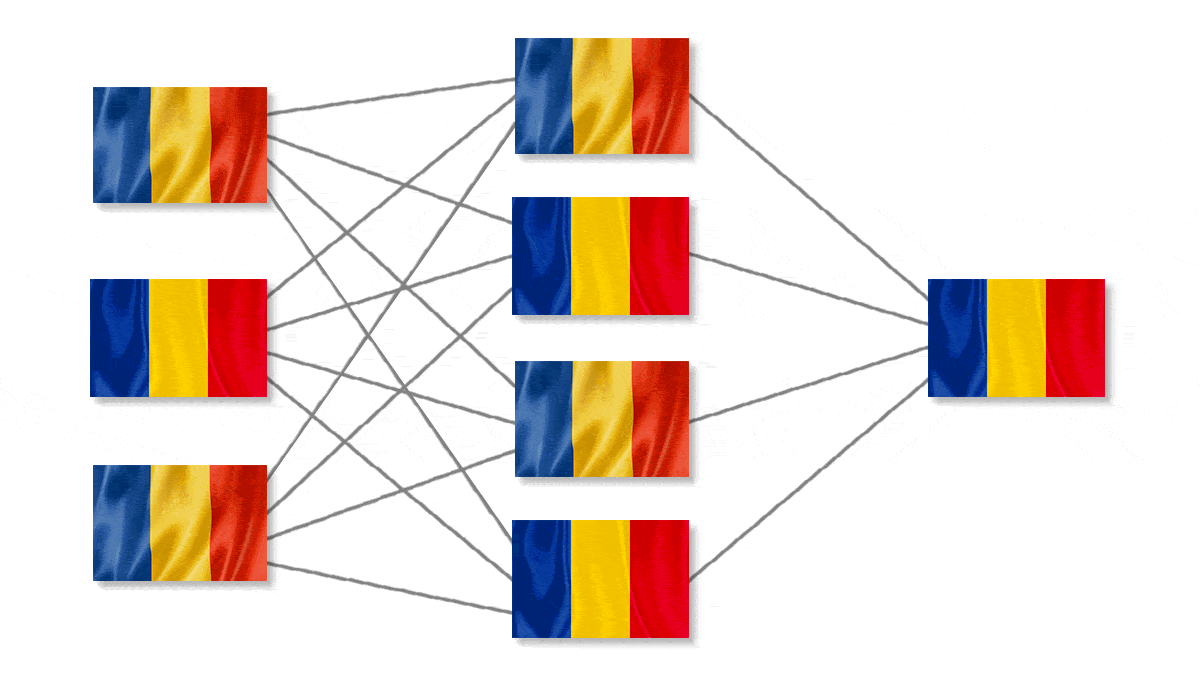
Deep (Learning) State
Meet the Romanian government’s automated political adviser.
What’s new: The Prime Minister of Romania launched ION, a system that summarizes and organizes public comments for cabinet ministers, Politico.eu reported.
How it works: Romanian citizens can submit comments via a website or by embellishing Twitter, Facebook, and Instagram posts with the tag @noisuntemION (“we are ION”). An online document describes the system in detail.
- The system uses an unsupervised semantic similarity model to prioritize comments depending on whether they’re relevant to national or international affairs.
- A natural language model extracts each comment’s topic (government activity, economics, healthcare, energy, sports, and so on) and references to people, locations, or events. A sentiment analyzer determines whether a comment is positive or negative and how strongly it expresses an opinion.
- A clustering algorithm groups similar messages; for instance, all messages that express a particular sentiment about a specific issue. The system generates a succinct description of each cluster.
- Another clustering algorithm maps relationships between clusters and creates superclusters. For instance, an issue’s supercluster may contain clusters that collect different sentiments.
- A subsystem monitors the clusters for changes. Officials can check the system for significant changes that may inform policy decisions.
Behind the news: Governments use AI to manage operations, dispense benefits, and administer justice. However, systems that influence policy remain largely experimental. For instance, Salesforce engineers trained a model to create a tax policy that promoted general income equality and productivity more effectively than the current United States tax code.
Why it matters: Politicians and policymakers must often interpret the will of the people through polls, press reports, or lobbyists. Romania’s experiment may tell officials more directly what constituents want.
We’re thinking: Many companies analyze social media to understand customer sentiment; for instance, clustering tweets to see what people are saying about a brand. Policymakers' embrace of a similar approach is a welcome step.
Data Points
Research group EleutherAI plans to launch a nonprofit institute backed by AI startups and industry leaders
The EleutherAI Institute aims to contribute to open source AI research through donations and grants from major backers like Hugging Face and Canva. (TechCrunch)
Eyeball, anthe first online video scouting platform for youth soccer, is helping elite teams like AC Milan and Benfica recruit new players
The system tracks players on the pitch and produces action clips and stats for scouts to analyze. (Forbes)
Consulting company Bain joined with OpenAI to develop a suite of marketing and customer service tools
The partnership will help Bain’s clients develop contact center scripts, ad copy, and more. The Coca-Cola Company will play an unspecified role. (Bain)
The Culture AI Games and Film Festival will preview AI-generated movies and video games coming soon
The festival, which will take place in San Francisco on May 9, 2023, will celebrate the impact of generative AI in the film and video game industry. (VentureBeat)
The United States plans to use face recognition on military drones
The U.S. Military signed a contract to deploy this AI-powered technology for surveillance,target acquisition, and other functions during special operations. (Vice)
Research: Meta released LLaMA, an open large language model
The model is available at several sizes (7B, 13B, 33B, and 65B parameters). You can apply for access to the models here. (Meta AI)
Research: Scientists developed a machine learning model to predict biodiversity of coral
The model is helping conservationists examine the impacts of climate change on the connectivity and biodiversity in the Coral Triangle, an area of the western Pacific Ocean that is one of the planet’s most diverse and biologically complex marine ecosystems. (Mongabay)
AI-written books flood Amazon’s Kindle store
More than 200 eBooks, from how-to guides to poetry collections, list ChatGPT as author or co-author. (Reuters)
A case before the UK's Supreme Court asks whether patents can list AI as an inventor
The UK Intellectual Property Office (IPO) rejected a developer’s bid to name an AI as the inventor for two patents. The developer is taking the case to the highest court. (Evening Standard)
SK Telecom plans to launch an AI chatbot
The South Korean company’s chatbot called “A.” (pronounced A period) will integrate third-party services like payment and ecommerce apps. It’s still in the early stages of an international launch. (CNBC)
Quizlet, which provides learning tools to students, launched a beta test of an AI tutor
Q-chat is a one-on-one tutor that tests reading comprehension, asks in-depth questions, and encourages students. It’s based on ChatGPT. (Quizlet)