Dear friends,
Suddenly it seems like everyone wants to regulate AI. The European Union is on the verge of enacting a comprehensive AI Act that’s intended to mitigate risks and protect individual rights. In the United States, Senate Majority leader Chuck Schumer foresees legislation possibly within months.
I’m in favor of regulation, too. But I’m very concerned about whether we’re on a trajectory toward helpful and effective regulation. At the moment, few regulators have sufficient understanding of AI’s potential benefits and harms to craft effective laws. The only thing more dangerous than knowing too little is knowing too little without understanding just how little that is.
I’m glad regulators are seeking to learn more about AI (as you can read about below). This is a wonderful step! But I see a dangerous situation emerging in which regulators speak with a number of academic and business leaders and come away thinking they understand things well enough. At best, only a few people in the world have the information to answer questions such as:
- How are AI-enabled paid online ads affecting elections in various countries right now?
- Is any social media company contributing to genocide or similarly dire events in the world?
- What types of AI-generated content are being produced (by the recent wave of chatbot companies and others), and how do they influence people?
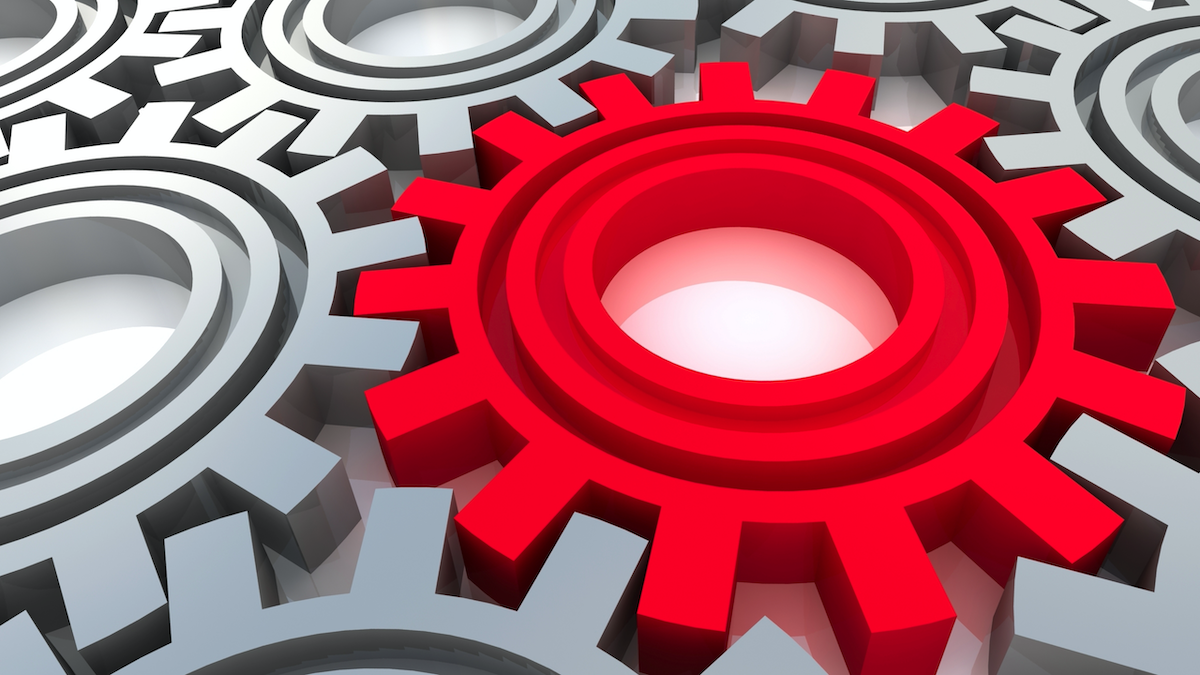
Answering questions like these requires far greater visibility into large AI companies than we currently have. In many countries, publicly traded companies are required to make substantial financial disclosures. Companies may find these requirements intrusive or burdensome, but the resulting transparency builds trust in the financial system. Similarly, the countries of the world need to compel large AI companies to disclose their activities in detail.
While the details of any required disclosure need to be worked out, I can imagine, for example, requiring large companies to analyze, or allow independent organizations to analyze, how much content of different flavors (such as pro/con various social issues) they deliver to different subsets of their audience (such as users in a particular region or demographic group). By presenting aggregate results, this can be done in a way that preserves individual privacy. Information like this would enable regulators to draw a straight line between the technology and events in the world. Without it, governments won’t know enough to craft sound regulations.
AI is making society richer, and governments have an important role in maximizing its benefits and minimizing its harms. But until there is greater transparency, it will be difficult for lawmakers to recognize the technology’s impacts in either direction. It will be difficult to prevent lobbyists from steering legislation to block competitors or otherwise further their interests in ways that don’t align with society’s.
I have deep respect for democratically elected legislators and the important work they do. I hope that all of us in AI — especially the many engineers and scientists who want to make the world better for everyone — can engage to help regulators play a constructive role in AI’s advance.
Keep learning!
Andrew
P.S. We just launched “Generative AI with Large Language Models,” a course built in collaboration with Amazon Web Services. Gain hands-on practice with techniques like reinforcement learning from human feedback; zero-, few-, and one-shot learning; fine-tuning; and advanced prompting using ReAct. You can sign up here.
News

Generated Data Fouls Human Datasets
The crowdworkers you hire to provide human data may use AI to produce it.
What's new: Researchers at École Polytechnique Fédérale de Lausanne found that written material supplied by workers hired via Amazon Mechanical Turk showed signs of being generated by ChatGPT.
How it works: 44 Mechanical Turk workers summarized medical research abstracts in roughly 100 words. The authors analyzed each summary for evidence that it had been generated by ChatGPT. The analysis relied on two methods:
- The authors fine-tuned e5-base to differentiate between summaries written by humans prior to the experiment and summaries generated by the authors, who prompted ChatGPT with the Mechanical Turk instructions.
- They also tracked the keystrokes of Mechanical Turk workers. Matching keystrokes and submissions counted as evidence that the writing was human-written. On the other hand, keystrokes that indicated copying and pasting indicated that submissions were generated.
Results: The authors analyzed 46 summaries written by 44 workers. The classifier found 21 summaries that showed 50 percent or greater likelihood of having been written by ChatGPT and 15 summaries that showed at least a 98 percent or greater likelihood. 41 of the summaries involved copying and pasting.
Yes, but: The researchers studied 46 summaries, a rather small sample. Furthermore, summarization is labor-intensive for humans but well within the capabilities of large language models. Other crowdsourced tasks may not be so easy to automate.
Behind the news: Mechanical Turk, founded by Amazon in 2005, has played an outsize role in machine learning. Many of the field’s most important datasets including ImageNet employed crowdsourced labor.
Why it matters: Machine learning engineers often use services like Mechanical Turk to collect and annotate training data on the assumption that humans are doing the work. If a significant number of crowdworkers instead rely on AI, it raises questions about the quality of the data and the validity of the output from models trained on it. Recent work found that, as the amount of model-generated content in a training set increases, the trained model’s performance decreases.
We're thinking: Training on machine-generated data seems likely to affect model performance unless you’re training a smaller model to mimic a larger one (known as model distillation). For example, it’s hard to imagine a language model trained only on the output of ChatGPT surpassing ChatGPT, whereas one trained on human data might. The lack of transparency with respect to which data comes from humans and which comes from machines presents a huge challenge for AI practitioners.
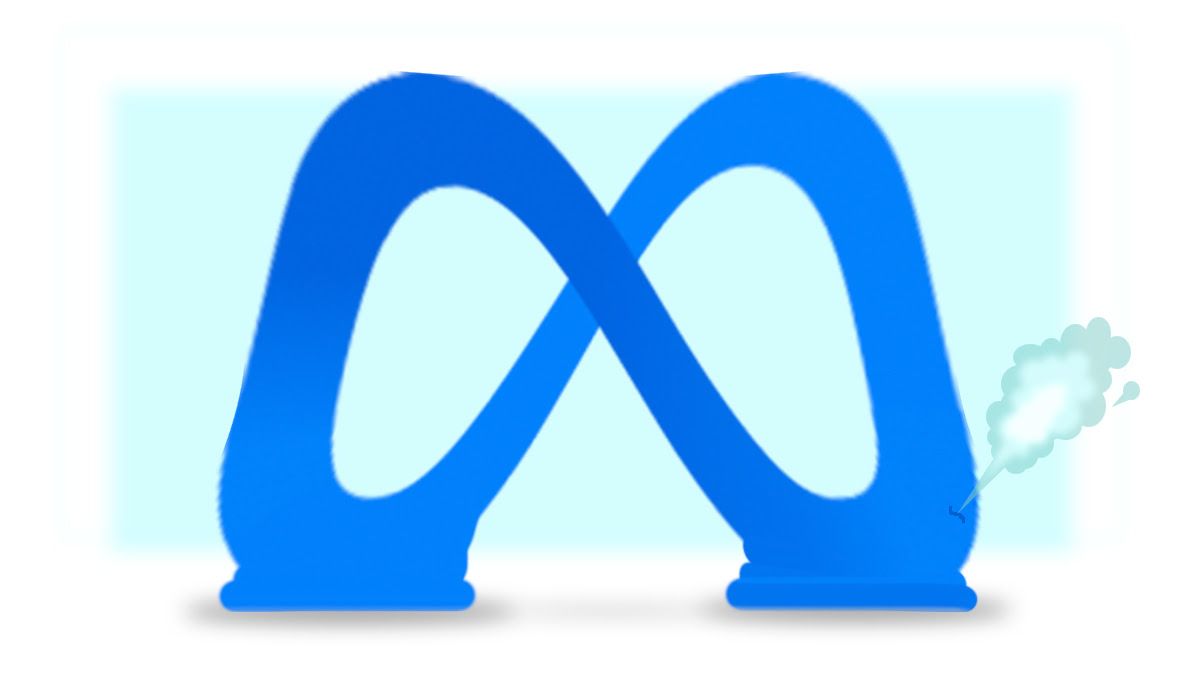
Where Is Meta’s Generative Play?
While Microsoft and Google scramble to supercharge their businesses with text generation, Meta has yet to launch a flagship generative AI service. Reporters went looking for reasons why.
What’s new: Staff turnover, misaligned priorities, insufficient processing power, and caution in the wake of earlier controversies have hindered Meta’s ability to take advantage of generative AI, The Wall Street Journal reported.
Challenges: Reporters spoke to more than a dozen current and former Meta employees to determine why, despite extensive investments in large language models (LLMs) and vision models like DINOv2 and SAM, the company lacks a high-profile generative initiative. They pointed to several factors:
- Over the past year, Meta lost many researchers who worked on LLMs. Six of the 14 authors of the LLaMA paper and eight of the 19 authors of the OPT paper either were laid off or departed for other jobs.
- Researchers who worked on LLMs struggled to get processing and engineering resources because chief AI scientist Yann LeCun was unenthusiastic about the technology, according to insiders who spoke to the reporters anonymously. The company prioritized recruiting scientists over engineers and valued research over building products, further impeding progress on products based on LLMs.
- Meta’s effort to equip its data centers to run such models suffered from strategic shifts and a shortage of high-end AI chips.The resources that were available often supported individual researchers’ pet projects rather than fulfilling a cohesive strategy.
- The public failures of Meta LLMs such as Galactica and BlenderBot 3, which Meta withdrew amid controversy over their generation of false statements, left the company more cautious — especially after years of outrage over negative social impacts of Facebook and Instagram.
Reorganization: Meta has taken steps to break the logjam. Earlier this month, it announced a number of generative AI products including chatbots for Messenger and WhatsApp, a photo editor for Instagram, and a productivity assistant for internal use. In February, Meta CEO Mark Zuckerburg announced a new generative AI group that reports directly to chief product officer Chris Cox. The group will focus on training models to integrate with products such as Facebook, Instagram, and WhatsApp.
Why it matters: The rapid rise of generative AI threatens to upend the tech world’s established order. Meta — like Google in response Microsoft’s aggressive launch of Bing Chat — has found itself in a defensive position.
We’re thinking: OpenAI developed breakthrough technology using a focused team of hundreds, and since then, several organizations have restructured from handfuls of researchers who work on diverse projects to large, focused teams that include both researchers and engineers. Although this shift prompted many researchers to leave in search of freedom to pursue their interests, the focused structure strikes us as a more promising approach from a business point of view.
A MESSAGE FROM DEEPLEARNING.AI
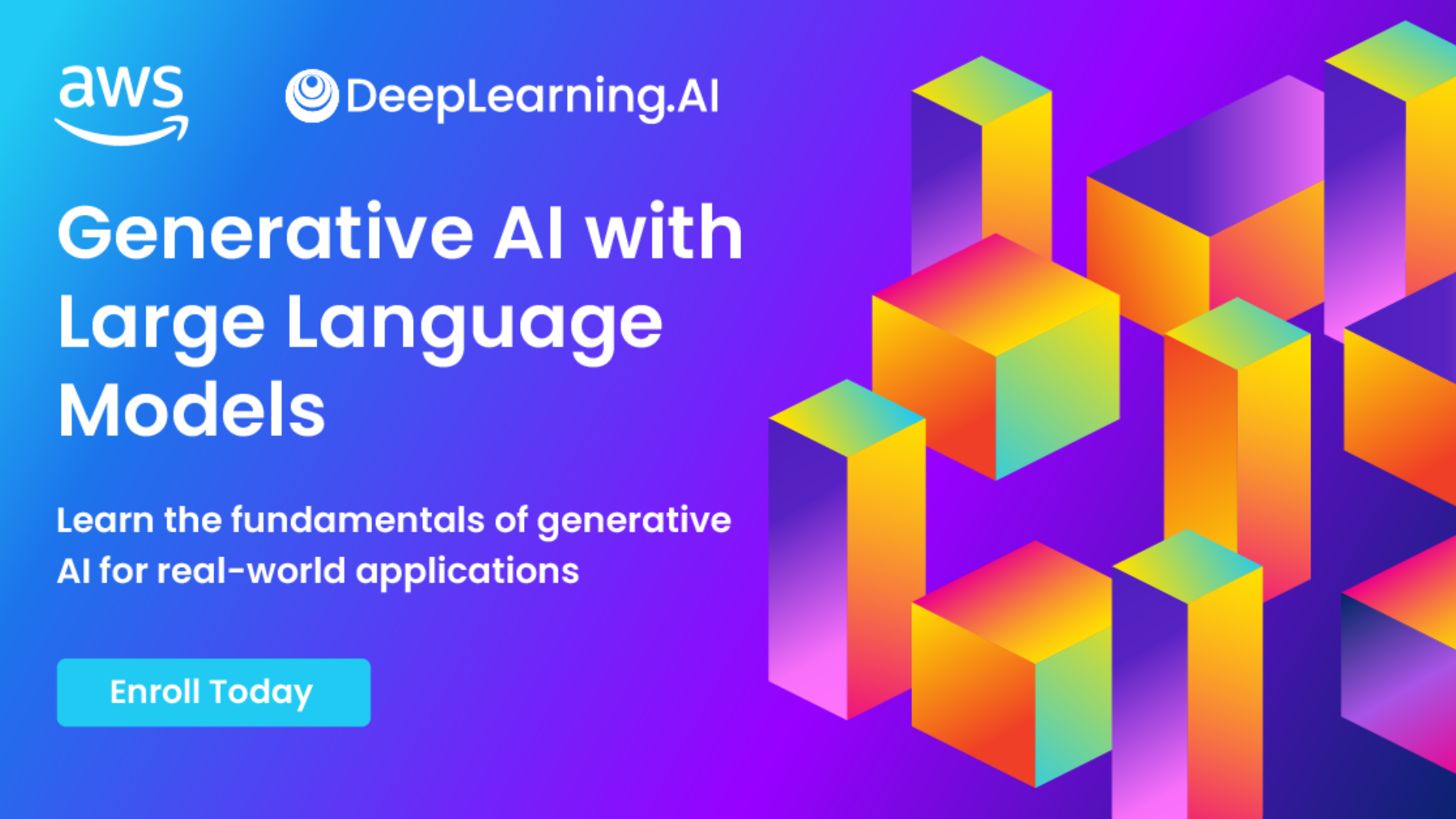
Master the technology behind large language models and learn how to fine-tune and use them to power real-world applications. Join us for “Generative AI with Large Language Models,” a new course developed in collaboration with AWS. Enroll now!
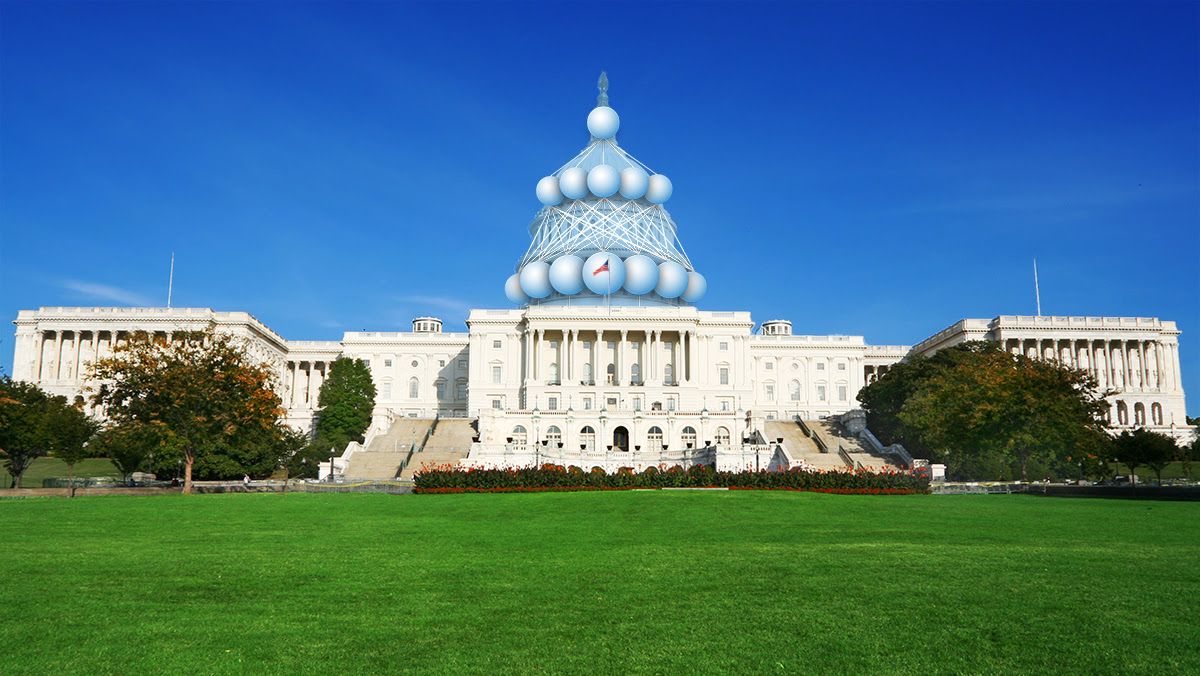
Washington Gears Up to Regulate
United States lawmakers are getting a crash course in AI.
What’s new: Chuck Schumer, the majority leader in the U.S. Senate, announced an unusual plan to educate legislators who are crafting AI regulations, The New York Times reported. It could lead to legislation “within months,” he said.
How it works: The senator calls his program SAFE Innovation, an acronym for four regulatory priorities: security, accountability, foundations, and explain [sic].
- The SAFE’s centerpiece is a series of nonpartisan listening sessions with industry executives, researchers, and civil rights activists, set to kick off later this year.
- The framework seeks to illuminate fundamental questions such as how to ensure safety, security, and accountability without hindering innovation, which is a linchpin in social, economic, and geopolitical priorities; the centralized versus distributed authority over AI; the relative roles of taxation and subsidies; and the optimal balance between protecting proprietary developments and encouraging open technology.
- The plan aims to encourage politicians from both major U.S. parties to craft legislation jointly.
Behind the news: Schumer’s move reflects growing interest in regulating AI among U.S. lawmakers.
- Representatives of both parties introduced a bill that would create a 20-member commission to develop guidelines for further legislation. Meanwhile, a Senate subcommittee recently probed the technology’s risks and opportunities in a hearing attended by executives at IBM and OpenAI as well as cognitive scientist and AI critic Gary Marcus, and the White House met with leaders of Google, Microsoft, OpenAI, and the startup Anthropic.
- Ten U.S. states and several local jurisdictions have enacted AI-related restrictions such as bans on police use of face recognition or New York City’s law, set to take effect in July, that will penalize employers who use automated hiring software.
- In October 2022, the Biden administration released an AI Bill of Rights that focuses on five key themes: safety and effectiveness, personal privacy, protection against algorithmic discrimination, disclosure of impact on users, and human alternatives to AI.
Yes, but: Any proposal must overcome fundamental disagreements between the two major parties, especially over whether a new, dedicated agency should oversee AI or whether that can be left to existing agencies. Moreover, some observers worry that Schumer’s deliberative approach could slow down legislative efforts that are already underway.
Why it matters: Thoughtful AI regulations must strike a delicate balance between encouraging innovation and protecting the public. It’s imperative that lawmakers — few of whom have a background in technology or science — understand the nuances.
We’re thinking: U.S. politics are increasingly divided. Bipartisan listening sessions on AI may serve a dual goal of educating lawmakers and uniting them around a shared vision.
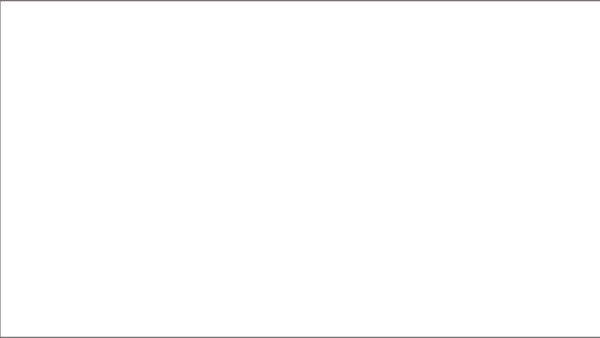
Finer Tuning
Fine-tuning a neural network typically involves retraining every layer on new data. But research shows that networks may perform better when fine-tuning modifies only a subset of layers.
What’s new: Yoonho Lee, Annie S. Chen, and colleagues at Stanford demonstrated surgical fine-tuning, a method that chooses specific layers to modify depending on how the fine-tuning dataset differs from the pretraining data.
Key insight: Earlier layers in a neural network learn to produce representations of fundamental features of the input, such as edges and shapes in an image, while later layers combine these features in a way that contributes to predicting a desired output, such as the image’s label. During fine-tuning, if the new images differ from the pretraining images in appearance, only earlier layers require modification. If the new images resemble the pretraining images but differ in their labels, only later layers require modification. Fine-tuning the appropriate layers updates a network effectively by prioritizing the weights most relevant to the new data.
How it works: The authors fine-tuned a ResNet-26 model pretrained on CIFAR-10 using manual and automated approaches.
- In the manual approach, the authors fine-tuned each layer individually, producing a new network each time. They identified the best layers to fine-tune by comparing the performance of each network.
- In the automated approach, they calculated the gradients for each layer. They divided the gradients by the magnitude of the layer’s weights to obtain relative gradients. They normalized the relative gradients across each layer at the beginning of fine-tuning and periodically throughout. This effectively ranked the layers from lowest to highest relative gradient on a scale from 0 to 1.
- During training, they assigned the learning rate for each layer according to the product of its normalized relative gradient (its score between 0 and 1) and a standard learning rate. This way, layers with the largest relative gradient would have the largest learning rate, while layers with the smallest relative gradient would have an effective learning rate of 0 and remain unchanged by fine-tuning.
Results: Evaluated on CIFAR-C, a version of the CFAR dataset deliberately corrupted by noise, the authors’ manual method classified images with 82.8 percent accuracy, while fine-tuning the whole network achieved 79.9 percent accuracy. The automated approach achieved 81.4 percent.
Why it matters: The authors drew on knowledge of how the neural networks process input to propose an efficient fine-tuning method. Better understanding of how a network extracts features could yield further ways to improve machine learning models.
We’re thinking: On datasets more complex than CIFAR-C, it can be hard to judge the difference between a pretraining dataset and a fine-tuning dataset. This may make the authors’ automated approach more valuable, even though it didn’t yield the best results.
Data Points
Disney+ TV series faces backlash over use of AI
Marvel series Secret Invasion used an AI-generated title card in its intro. Artists are labeling this decision unethical and dangerous to the artistic community. (Venture Beat)
ChatGPT-powered device allows trees to “talk” to people
The TreeTag, a device that helps monitor a tree's vital signs, integrates generative AI to enable the tree to communicate in text form its condition and needs. (CNET)
U.S. workers and employers out of sync on new technology
A survey found that employees are enthusiastic about emerging technologies. However, these technologies become outdated by the time their company adopts them. The age difference between younger employers and older managersmight account for late adoption. (Ernst & Young)
Google is warning employees over chatbot use
The tech giant reportedly advised its employees not to share confidential information with chatbots, including its own, as user input to chatbots is stored by the companies who own the technology. (ZDNet)
OpenAI lobbied EU to revise proposed AI regulation
The company behind ChatGPT attempted to soften a draft of the AI Act, which would impose stringent restrictions on general-purpose AI systems. Legislators integrated some of the suggested changes into the draft. (The Verge)
Chatbot leads church service in Germany
ChatGPT, personified by an avatar on a screen, delivered a 40-minute service at a convention of Protestants. The chatbot generated 98 percent of the sermon. (AP News)
OpenAI considers launching an app store
The company is reportedly contemplating a marketplace for users to sell customized AI models. (The Information)
You.com introduced subscription to AI products
You.com, which offers a personalized search engine, launched a paid service called YouPro. It provides unlimited access to a suite of text and image generation tools based on the latest models for $9.99/month. (Business Wire)
China's black market defies U.S. ban on chip exports
Underground vendors in Shenzen are evading U.S. restrictions on chip exports to China. They typically sell A100 Nvidia chips at double the usual price. (Reuters)
Chatbots occupy trusted jobs in Indonesia
AI avatars are working as immigration officers, news anchors in the world’s fourth most populous country. (Rest of World)
Research: AI Reveals new figures in Nazca Lines
Scientists used a deep learning model to scan aerial photographs captured in Peru’s Nazca Desert. They discovered three new geoglyphs, or huge line drawings cut into the desert floor thousands of years ago. (Live Science)
Google launched an anti-money-laundering tool
The tool accurately detected two to four times more incidents than earlier methods and reduced alert volumes by 60 percent in tests conducted with HSBC. (The Wall Street Journal)
AI models are failing to meet proposed EU regulations
Researchers assessed whether 10 popular AI models meet standards outlined in the EU’s draft AI Act, a comprehensive AI regulation that is expected to become law by early next year. The models evaluated include BLOOM, GPT-4, LLaMA, and Stable Diffusion. All ten fell short in various areas, and six scored below 50 percent. (Financial Times)