Dear friends,
Last week, I returned home from Asia, where I spoke at Seoul National University in Korea, the National University of Singapore, and the University of Tokyo in Japan and visited many businesses. As I discussed the state of AI with students, technologists, executives, and government officials, something struck me: Around the world, everyone is wrestling with similar AI-related issues.
In every country:
- Business leaders are asking how AI will affect their companies.
- Governments are wondering how it will affect the labor market, what risks it poses, and how to regulate it.
- Companies are trying to figure out how to use it without “giving away” their data to one of the platform vendors.
- Developers are experimenting with creative uses of generative AI. The two most common applications remain building customer service chatbots and answering questions based on documents. But I also heard about numerous creative projects in medical records, financial records, privacy protection, and much more.
When the deep learning revolution started about a decade ago, I advised teams to (i) learn about the technology, (ii) start small and build projects quickly to hone intuition about what’s possible, and (iii) use learnings from smaller projects to scale to bigger ones. With the generative AI revolution, my advice remains the same. This time, though, the barrier to entry is lower and thus the time-to-value seems to be shorter. It takes substantial effort to collect data and train and deploy a neural network, but less effort to prompt a large language model and start getting results.
For developers, this means richer opportunities than ever! Leaders are looking for helpful perspectives. If you’re able to experiment, learn, identify successful use cases (and even some failures — which is fine, too), and share your insights with colleagues, perhaps you can influence the trajectory of your business.
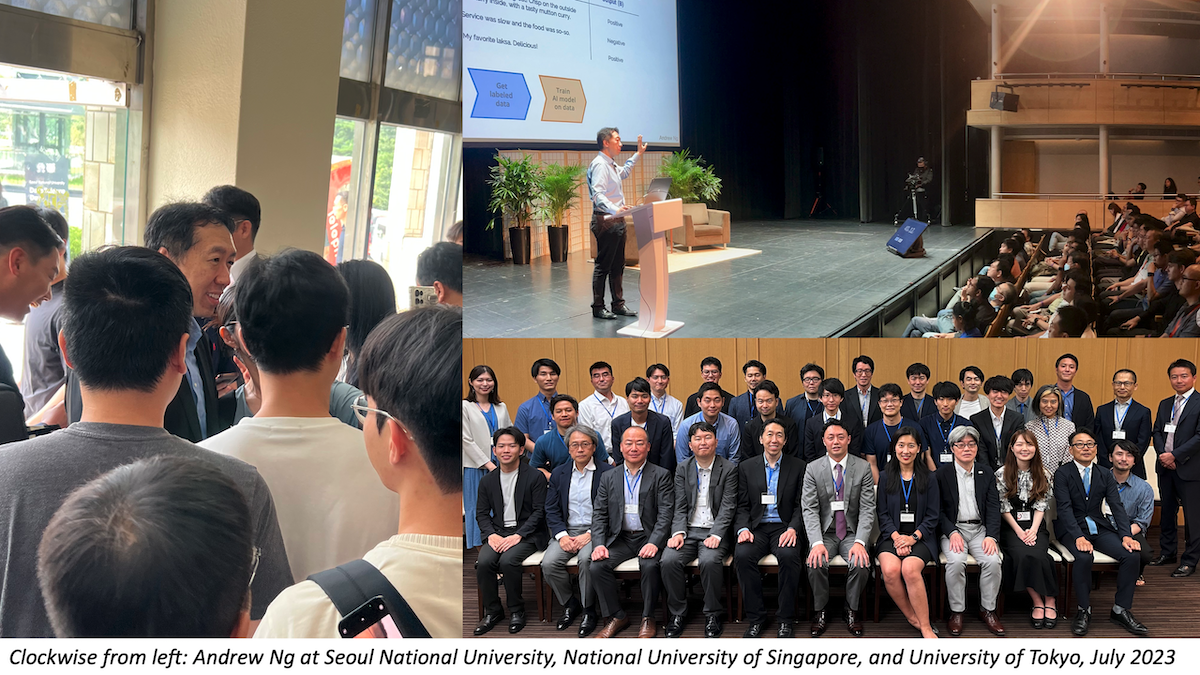
Last Friday, I discussed how businesses can plan for generative AI with Erik Brynjolfsson, Andrew McAfee, James Milin, and Daniel Rock, who co-founded Workhelix (a portfolio company of AI Fund, which I lead). Workhelix helps its customers break down jobs into tasks to see which tasks can be augmented by AI. You can listen to the conversation here.
For instance, a radiologist’s tasks include (i) capturing images, (ii) reading them, (iii) communicating with patients, and so on. Which of these tasks can take advantage of AI to make a radiologist’s job more productive and enjoyable? Can it help optimize image acquisition (perhaps by tuning the X-ray machine controls), speed up interpretation of images, or generate takeaways text for patients?
Although Workhelix is applying this recipe at scale, it’s also useful for teams that are exploring opportunities in AI. Consider not jobs but their component tasks. Are any of them amenable to automation or assistance by AI? This can be a helpful framework for brainstorming interesting project ideas.
The way generative AI is taking off in many places around the world means that our markets are increasingly global. Wherever in the world you live, this is a wonderful time to build your AI knowledge and increase your AI skills. Exciting opportunities lie ahead!
Special thanks to Ian Park of the Korean Investment Corporation, Chong Yap Seng of the National University of Singapore, and Yuji Mano of Mitsui, who made my visits much more productive and enjoyable. I also hope to visit other countries soon. Stay tuned!
Keep learning,
Andrew
P.S. DeepLearning.AI just launched “Evaluating and Debugging Generative AI,” created in collaboration with Weights & Biases and taught by Carey Phelps. Machine learning development is an iterative process, and we often have to try many things to build a system that works. I used to keep track of all the different models I was training in a text file or spreadsheet. Thankfully better tools are available now. This course will teach you how to use them, focusing on generative AI applications. I hope you enjoy the course!
News
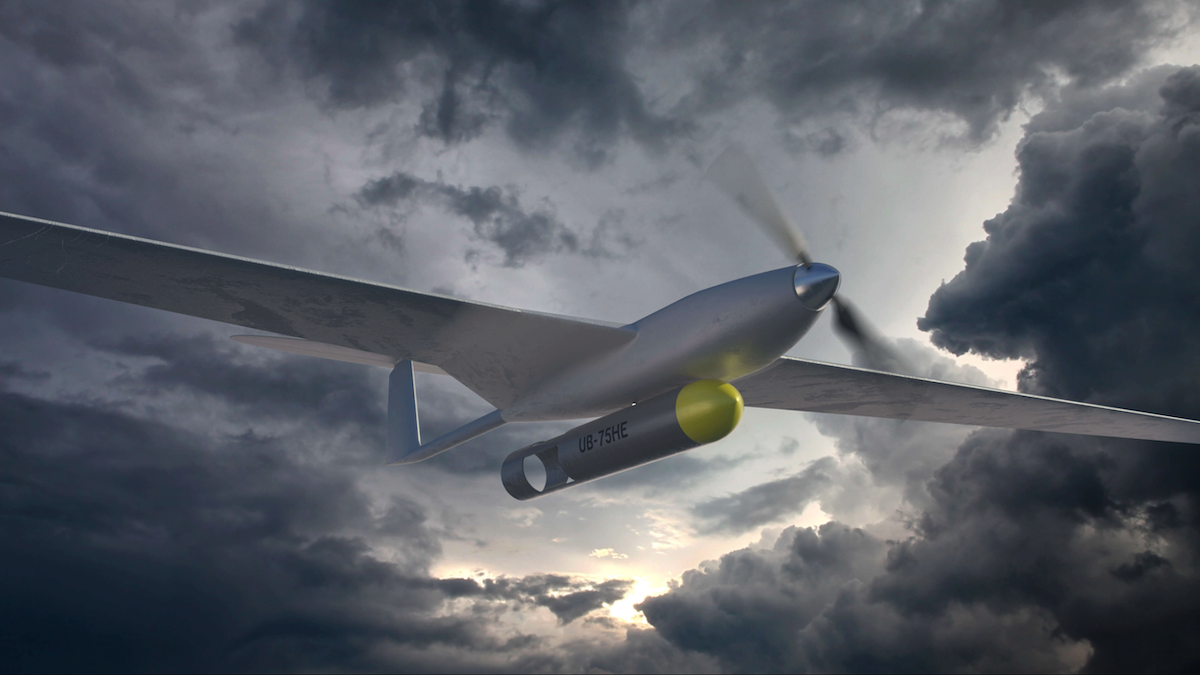
Ukraine’s Homegrown Drones
The war in Ukraine has spurred a new domestic industry.
What’s new: Hundreds of drone companies have sprung up in Ukraine since Russian forces invaded the country early last year, The Washington Post reported.
How it works: Ukrainian drone startups are developing air- and sea-borne robots, which the country’s military use to monitor enemy positions, guide artillery strikes, and drop bombs, sometimes on Russian territory.
- Quadcopters built by Twist Robotics use AI-powered target tracking to remain locked onto targets even if the operator loses radio contact. Air and naval drones from Warbirds have similar capabilities.
- Working in an active war zone gives local drone makers advantages over their foreign counterparts. For instance, Ukrainian authorities give domestic firms access to captured Russian jamming technology so that they can develop countermeasures. Similarly, the companies acquire huge amounts of real-world data from the front lines, such as images of tanks or landmines in a variety of settings, that can be used to train their systems. They also receive immediate feedback on how their machines perform on the battlefield.
- Foreign companies are angling to get involved — partly to gain access to the same data. Canada-based Draganfly and U.S.-based BRINC are actively developing drones in Ukraine. German defense-AI company Helsing and U.S. data analytics firm Palantir also maintain offices there.
Russia responds: In recent months, Russia has stepped up attacks by Russian-made Lancet fliers that explode upon crashing into their targets. Recent units appear to contain Nvidia Jetson TX2 computers, which could drive AI-powered guidance or targeting, Forbes reported. Russian state news denied that its drones use AI.
Behind the news: Other countries are also gearing up for drone warfare.
- A U.S. Navy group called Task Force 59 recently tested a system, built from off-the-shelf components, that identifies threats based on data from drones, other air vessels, surface ships, and submarines.
- The Israel Defense Forces reportedly deployed an AI system that selects targets for air strikes. A separate system then calculates munition loads, schedules strikes, and assigns targets to drones and crewed aircraft.
- Taiwan launched a major program to build its own drones.
Why it matters: Drones rapidly have become a battlefield staple, and their offensive capabilities are growing. Governments around the world are paying close attention for lessons to be learned — as are, no doubt, insurgent forces, paramilitary groups, and drug cartels.
We’re thinking: We stand with the brave Ukrainian soldiers as they defend their country against an adversary with a much larger air force. War is tragic and ugly. We wish that no one used AI-enabled weapons. But the reality is that peaceful and democratic nations do, if only to defend themselves against adversaries who do the same. We are heartened by recent agreements to limit development of fully autonomous weapons, and we support the United Nations’ proposal to ban them entirely.
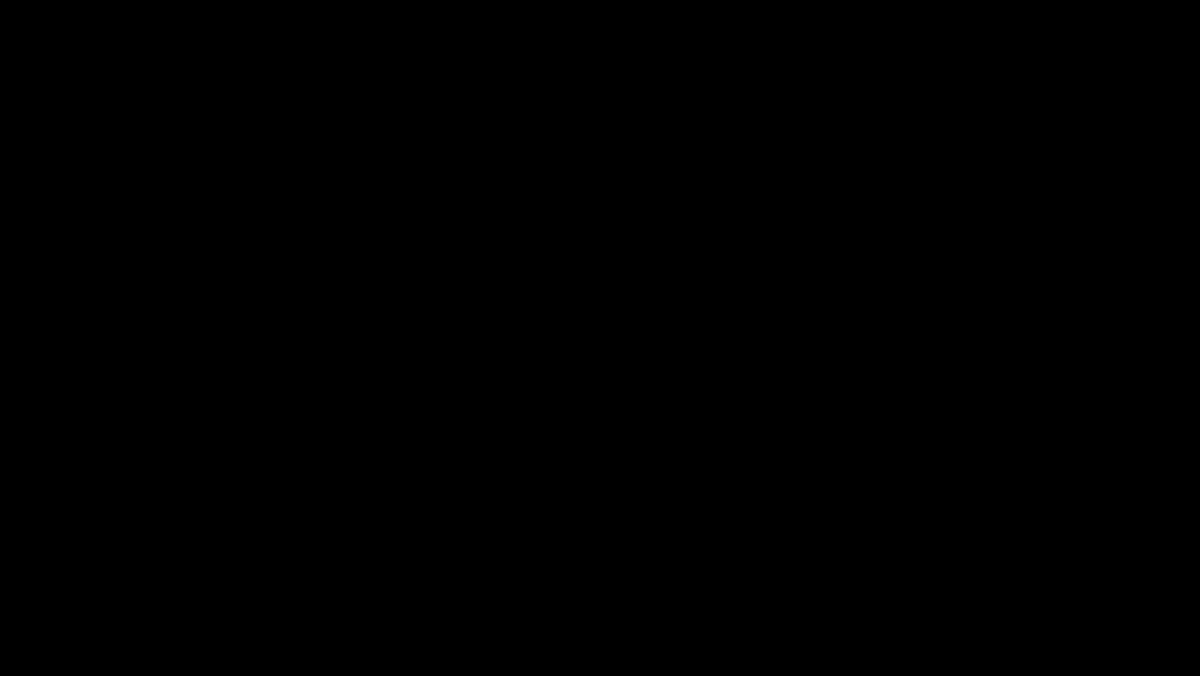
Cloud Computing Goes Generative
Amazon aims to make it easier for its cloud computing customers to build applications that take advantage of generative AI.
What’s new: Amazon Web Services’ Bedrock platform is offering new generative models, software agents that enable customers to interact with those models, and a service that generates medical records. The new capabilities are available in what Amazon calls “preview” and are subject to change.
How it works: Bedrock launched in April with the Stable Diffusion image generator and large language models including AI21’s Jurassic-2 and Anthropic’s Claude. The new additions extend the platform in a few directions.
- Bedrock added two models from Cohere: a model named Command for summarizing, copywriting, and question answering; and one called Embed, which generates embeddings in more than 100 languages. It also upgraded to Anthropic’s Claude 2 and added Stability AI’s newly released Stable Diffusion XL 1.0.
- The Agents capability enables users to incorporate these models into applications that understand and fulfill requests and take advantage of private data. For instance, an airline booking website could build an agent that takes into account an individual’s travel history, finds suitable flight schedules, and books selected flights.
- HealthScribe helps to generate medical notes after a clinical visit. Language models transcribe conversations between patients and medical professionals, identify speakers, extract medical terminology such as conditions and medications, and generate summaries. The system complies with United States laws that protect patient information.
Behind the news: Amazon’s major rivals in cloud computing have introduced their own generative-AI-as-a-service offerings.
- Google Cloud Platform offers access to generative models such as its PaLM large language model and Imagen image generator via the Vertex AI service. Its Generative AI App Builder aims to help users build customized chatbots and search engines.
- Microsoft Azure offers OpenAI models including GPT-4 and DALL·E 2.
Why it matters: Access to the latest generative models is likely to be a crucial factor in bringing AI’s benefits to all industries. For Amazon, providing those models and tools to build applications on top of them could help maintain its dominant position in the market for cloud computing.
We’re thinking: One challenge to startups that provide an API for generative AI is that the cost of switching from one API to another is low, which makes their businesses less defensible. In contrast, cloud-computing platforms offer many APIs, which creates high switching costs. That is, once you've built an application on a particular cloud platform, migrating to another is impractical. This makes cloud computing highly profitable. It also makes offering APIs for generative AI an obvious move for incumbent platforms.
A MESSAGE FROM DEEPLEARNING.AI
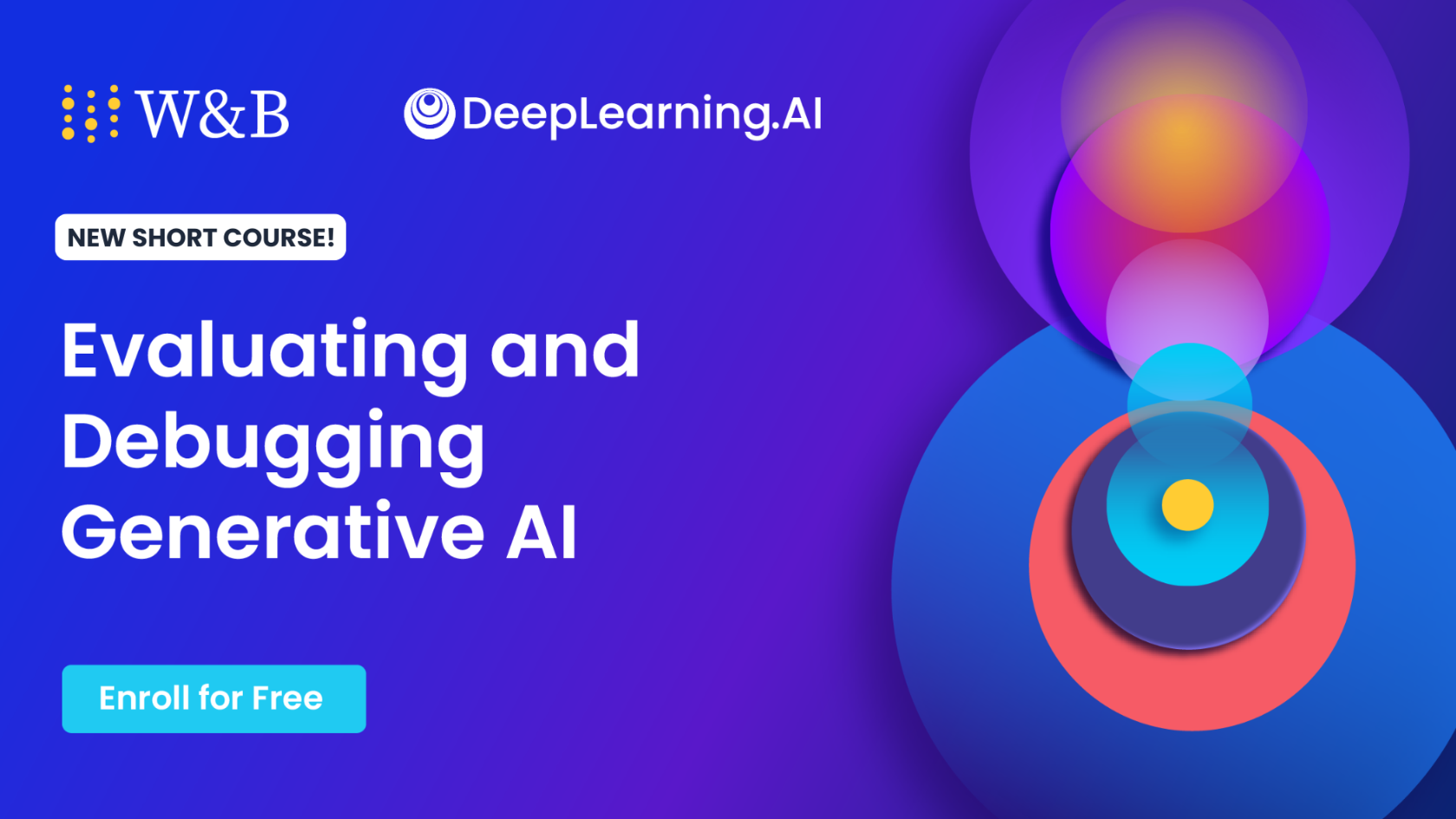
Join our new course “Evaluating and Debugging Generative AI,” and learn to manage and track data sources and volumes, debug your models, and conduct tests and evaluations easily. Sign up for free
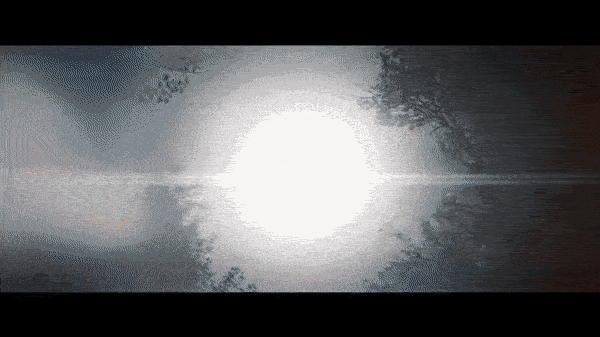
K-Pop Sings in Many Tongues
A Korean pop star recorded a song in six languages, thanks to deep learning.
What’s new: Midnatt (better known as Lee Hyun) sang his latest release, “Masquerade,” in English, Japanese, Mandarin, Spanish, and Vietnamese — none of which he speaks fluently — as well as his native Korean. The entertainment company Hybe used a deep learning system to improve his pronunciation, Reuters reported. You can listen to the results here.
How it works: Hybe used Neural Analysis and Synthesis (NANSY), a neural speech processor developed by the Seoul-based startup Supertone, which Hybe acquired in January for $36 million.
- Given a vocal recording, NANSY separates pronunciation, timbre, pitch, and volume information. It uses wav2vec to analyze pronunciation, a custom convolutional neural network (CNN) for timbre, and a custom algorithm for pitch. To analyze volume, it takes an average across a mel spectrogram (a visual representation of a sound’s frequency components over time). The NANSY recombines the four elements using a CNN-based subsystem.
- Lee initially recorded “Masquerade” in each of the six languages. Then the producers recorded native speakers of the non-Korean tongues reading the lyrics in their respective languages. NANSY melded the sung and spoken recordings to adjust Lee’s pronunciation.
Behind the news: The music industry has been paying close attention to generative audio models lately, as fans have used deep learning systems to mimic the voices of established artists. Reactions from artists and music companies have been mixed.
- The musician Grimes released a tool that allows users to transform their own voices into hers. She invited people to try to earn money using her cloned voice in exchange for half of any resulting royalties. More than 300 fans responded by uploading Grimes-like productions to streaming services.
- Universal Music Group has been less welcoming. The recording-industry giant demanded that streaming services remove fan-made tracks that feature cloned voices of Universal artists.
Why it matters: This application of generated audio suggests that the technology could have tremendous commercial value. K-pop artists frequently release songs in English and Japanese, and popular musicians have recorded their songs in multiple languages since at least the 1930s, when Marlene Dietrich recorded her hits in English as well as her native German. This approach could help singers all over the world to reach listeners who may be more receptive to songs in a familiar language.
We’re thinking: Auto-Tune software began as a tool for correcting flaws in vocal performances, but musicians quickly exploited it as an effect in its own right. How long before adventurous artists use pronunciation correction to, say, sing in their own languages with foreign accents?
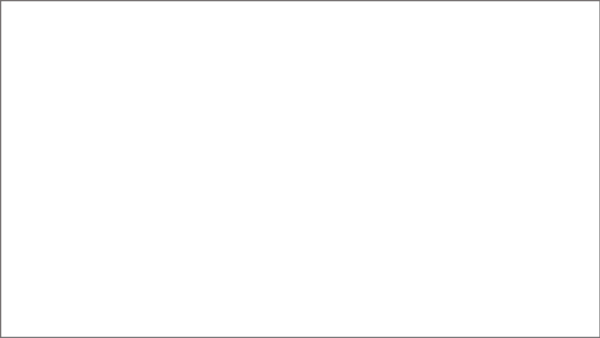
Long-Range Weather Forecasts
Machine learning models have predicted weather a few days ahead of time. A new approach substantially extends the time horizon.
What’s new: Remi Lam and colleagues at Google developed GraphCast, a weather-forecasting system based on graph neural networks (GNNs). Its 10-day forecasts outperformed those of conventional and deep-learning methods.
GNN basics: A GNN processes input in the form of a graph made up of nodes connected by edges. It uses a vanilla neural network to update the representation of each node based on those of neighboring nodes. For example, nodes can represent customers and products while edges represent purchases, or — as in this work — nodes can represent local weather while edges represent connections between locations.
Key insight: Short-term changes in the weather in a given location depend on conditions in nearby areas. A graph can reflect these relationships using information drawn from a high-resolution weather map, where each node represents an area’s weather and edges connect nearby areas. However, longer-term changes in the weather depend on conditions in both nearby and distant areas. To reflect relationships between more distant areas, the graph can draw on a lower-resolution map, which connects areas at greater distances. Combining edges drawn from higher- and lower-resolution weather maps produces a graph that reflects relationships among both nearby and distant areas, making it suitable for longer-term predictions.
How it works: GraphCast produced graphs based on high- and low-resolution weather maps and processed them using three GNNs called the encoder, processor, and decoder. The authors trained the system on global weather data from 1979 to 2017. Given a set of weather conditions and a set of weather conditions measured 6 hours previously for all locations on Earth, GraphCast learned to predict the weather 6 hours in the future and multiples thereof.
- The authors divided a map of Earth into areas 0.25 by 0.25 degrees to make a graph — actually a grid — with roughly 1 million nodes, each containing over 200 values (for conditions such as temperature, humidity, air pressure, wind speed, precipitation, and so on) measured at a given time and 6 hours earlier. The nodes were connected at their northern, southern, eastern, and western borders.
- The authors created a new graph by connecting each node of the grid to a smaller graph of around 41,000 nodes, where each node covered a larger region and nearby regions were connected via edges. (Specifically, the smaller graph’s nodes and edges coincided with those of a sphere divided into roughly 82,000 equilateral triangles. The authors connected nodes in the grid to those in the smaller graph if, when the two graphs were overlaid, the distance between them did not exceed a threshold.) Given the smaller graph, the encoder GNN learned to compute an embedding for each node.
- To produce a multi-resolution graph, the authors represented Earth as an icosahedron (12 nodes and 20 equilateral triangles) and iteratively divided each triangle into 4 more triangles. They did this 6 times, creating 6 additional graphs of between 12 and roughly 10,000 nodes. They superimposed these graphs’ edges over the 41,000-node graph. Given the multi-resolution graph, the processor GNN learned to update the 41,000 node embeddings.
- To return the resolution to 0.25 by 0.25 degrees, the authors created yet another graph by connecting the 41,000 nodes to their corresponding locations among the 1 million nodes on the initial grid. (Specifically, for each grid node, they found the triangular face that would contain it if the grid and 41,000-node graph were overlaid. Then they connected the grid node to the 3 nodes that formed this triangle.) Given this graph, the decoder GNN learned to compute the change in weather conditions for each node on the grid.
- To predict the next time step, the authors added the decoder’s output to the values at the current time step. To forecast further into the future, they repeated the process, predicting the next time step based on the previously predicted values.
- The system learned to predict the values at the next time step by minimizing the mean squared error between its predictions and actual measurements in 6-hour increments up to three days in advance (that is, over 12 sequential forecasts).
Results: Using 2018 data, the authors compared GraphCast’s 10-day forecasts to those of a popular European system that predicts weather based on differential equations that describe atmospheric physics. Compared to actual measurements, GraphCast achieved a lower root mean squared error in 90 percent of predictions. It produced a 10-day forecast at 0.25-degree resolution in under 60 seconds using a single TPU v4 chip, while the European system, which forecasts at 0.1-degree resolution, needed 150 to 240 hours on a supercomputer. GraphCast also outperformed Pangu-Weather, a transformer-based method, in 99.2 percent of predictions.
Yes, but: GraphCast’s predictions tended to be closer to average weather conditions, and it performed worse when the weather included extreme temperatures or storms.
Why it matters: Given a graph that combines multiple spatial resolutions, GNN can compute the influence of weather over large distances using relatively little memory and computation. This sort of graph structure may benefit other applications that process large inputs such as ultra-high resolution photos, fluid dynamics, and cosmological data.
We’re thinking: When it comes to forecasting weather, it looks like deep learning is the raining champ.
A MESSAGE FROM WORKERA
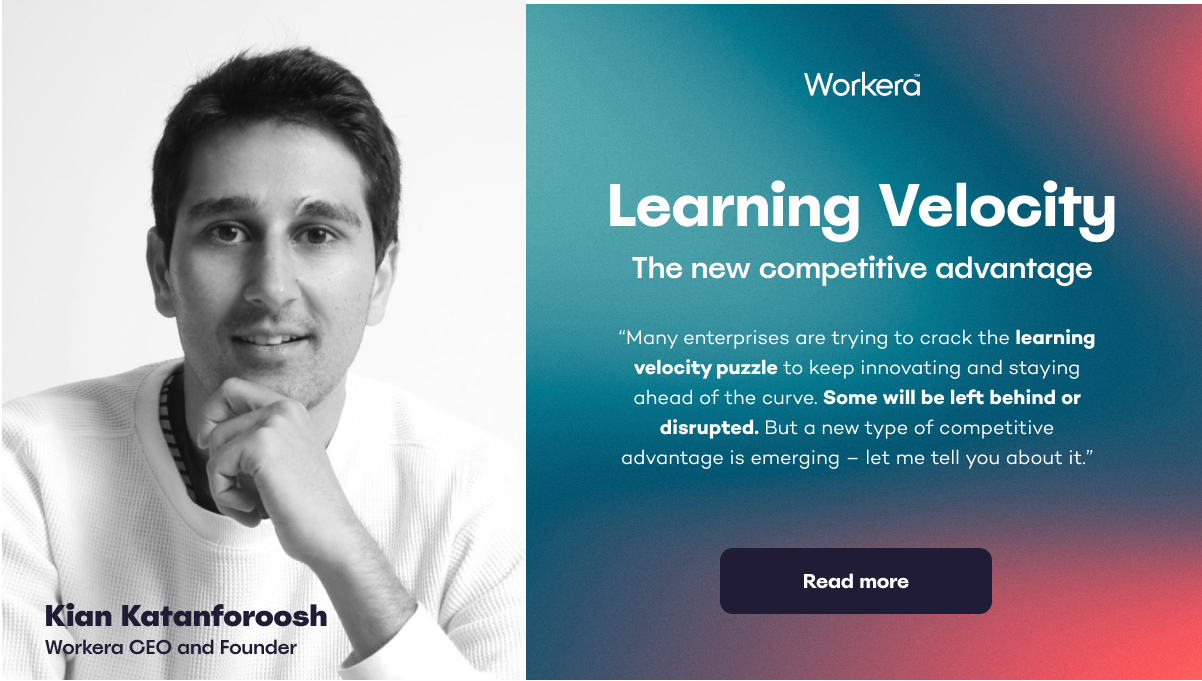
The best defense against disruption is your ability to take advantage of innovation. That’s why enabling employees to learn rapidly is a business imperative. Read Kian Katanforoosh's essential guide to learning velocity for business.
Data Points
Stack Overflow answers questions using generative AI
The developer forum released OverflowAI, a toolkit that allows users to pose questions and generates answers from a database of 58 million public discussions, citing specific sources. (VentureBeat)
Indian nonprofit hires rural workers to generate data
Karya employs people in rural India to record phrases in languages rarely found online, to be used as training data for language models. The company pays these workers a high wage relative to the rural areas where they live. (Time)
VC firm published tutorial for building AI romantic partners
Andreessen Horowitz released a tutorial on GitHub that shows users how to build AI companions with configurable personalities and backstories. The firm said the project has therapeutic potential. (Decrypt)
Cybercriminals offer a chatbot for sophisticated attacks
The bot, called FraudGPT, can craft spear-phishing emails, write harmful code, make undetectable malware, and other malicious products. It’s being advertised on web marketplaces and Telegram channels. (The Hacker News)
Officials regulate Wall Street's use of AI
The United States Securities and Exchange Commission approved a plan to address conflicts of interest in the use of AI by brokerages and money managers. The proposal requires companies to assess potential conflicts and disclose cybersecurity incidents, among other actions. (BNN Bloomberg)
Stability AI launched Stable Diffusion XL 1.0
The updated text-to-image model delivers more vibrant and accurate colors, better contrast, and improved text generation. Bedrock, Amazon's cloud platform for generative AI models, will host it. (TechCrunch)
OpenAI's head of Trust and Safety resigned
Industry veteran Dave Willner cited the demands of his job and a desire to spend more time with his family as reasons for stepping down. His departure comes as the AI industry faces questions about regulation, safety, and harmful impacts, especially regarding generative AI. (TechCrunch)
Wayfair offers virtual room restyler
The online home store launched Decorify, tool to visualize redecorated rooms based on uploaded pictures and selected visual styles. Users can browse furniture recommendations based on the AI-remodeled rooms. (The Verge)
Report: AI will take more jobs from women than men
A study by the McKinsey Global Institute indicates that millions of jobs filled primarily by women, like office support and retail sales, will be eliminated by 2030 due to AI and automation. This shift in the labor market will result in a growing demand for social-emotional and digital skills, the consultancy forecasts. (Gizmodo)
Infrastructure giant to expand AI at Heathrow Airport
By 2024, Ferrovial aims to deploy a new AI services in its main toll road and airport project, including London's Heathrow Airport. Applicationsinclude predicting passenger traffic changes, identifying anomalies, and detecting airport bottlenecks. (Reuters)
Research: ChatGPT and other chatbots vulnerable to hackers
A report by Carnegie Mellon University and the Center for AI Safety revealed that even tightly controlled systems from Anthropic, Google, and OpenAI could be manipulated using methods developed using open source AI systems. For instance, adding a lengthy suffix to a prompt unleashes chatbots that have been fine-tuned to withhold harmful information. (The New York Times)
OpenAI withdrew AI detection tool
The tool, introduced six months ago, was aimed at helping educators identify AI-generated work but proved insufficiently accurate. OpenAI plans to improve it and explore more effective detection techniques. Meanwhile, educators are turning to alternatives like Turnitin and ZeroGPT. (CNN)
New organization promotes responsible AI
Google, Microsoft, OpenAI, and Anthropic established the Frontier Model Forum, abody aimed at ensuring the safe and responsible development of advanced AI models. However, critics remain skeptical of the industry's ability to regulate itself. (The Guardian)
30 percent of IT workers believe AI will not hurt their jobs
A Pew Research Center survey examined worker attitudes toward AI in a variety of industries. Workers in sectors where AI is already assisting their jobs, like information technology, feel more positive about the technology's impact, they found. Some workers expressed concerns about misinformation, bias, and potential concentration of wealth and privilege. (The Washington Post)
ChatGPT plug-ins spark security concerns
Researchers highlighted potential risks of third-party plug-ins for ChatGPT, which may expose users to data theft and malicious code execution. OpenAI acknowledged the concerns and said it is working to improve security. (Wired)