Dear friends,
As you can read below, improvements in chatbots have opened a market for bots integrated with dating apps. I’m excited about the possibilities for large language models (LLMs) in romantic relationships, but I’m concerned that AI romantic partners create fake relationships that displace, rather than strengthen, meaningful human relationships. In my recent Stanford presentation on “Opportunities in AI,” I mentioned that AI Fund has been working with Renate Nyborg to deliver romantic mentoring. I’d like to explain why, despite my concern, I believe that AI can help many people with relationships.
By 2020, it was clear that a change was coming in how we build natural language processing applications. As I wrote in The Batch that September, “GPT-3 is setting a new direction for building language models and applications. I see a clear path toward scaling up computation and algorithmic improvements.” Today, we’re much farther down that path.
I didn't know back then that ChatGPT would go viral upon its release in November 2022. But AI Fund entrepreneurs were already experimenting with GPT-3, and we started looking for opportunities to build businesses on it. I had read the academic work about questions that lead to love. I believe that you don’t find a great relationship; you create it. So instead of trying to help you find a great partner — as most dating apps aim to do — why not use AI to help people create great relationships?
I’m clearly not a subject-matter expert in relationships (despite having spent many hours on eHarmony when I was single)! So I was fortunate to meet Renate, former CEO of Tinder, and start working with her on what became Meeno (formerly Amorai). Although we started exploring these ideas before ChatGPT was released, the wave of interest since then has been a boon to the project.
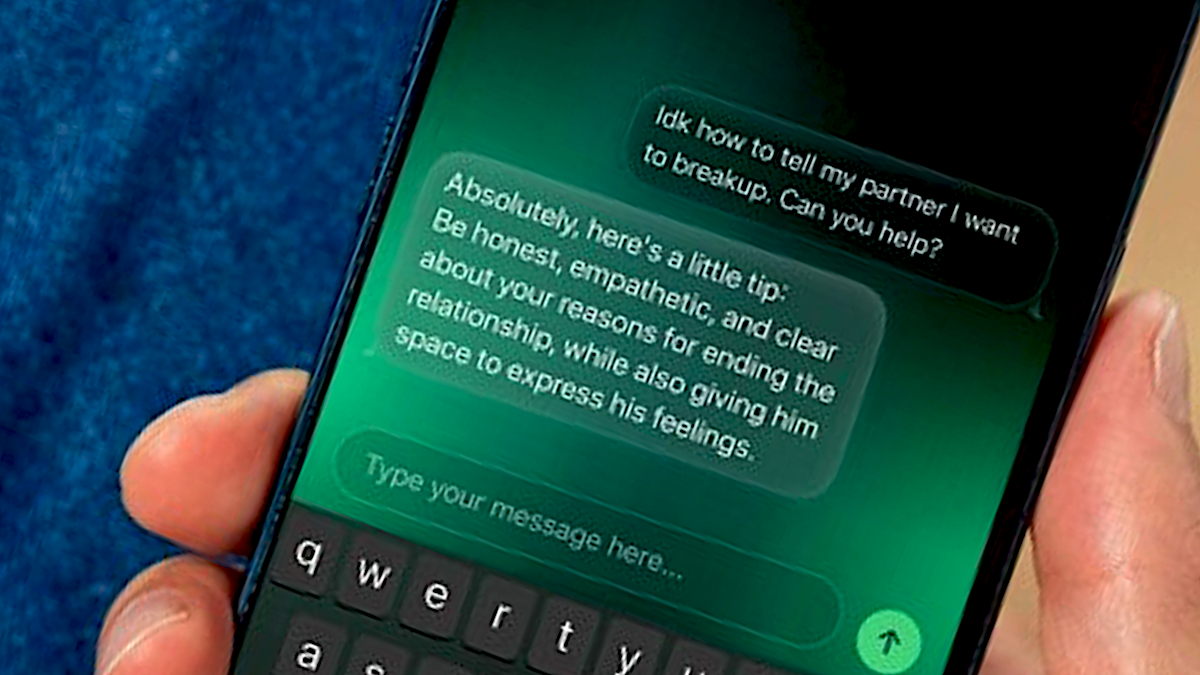
Renate has far more systematic knowledge about relationships than anyone I know. With AI Fund’s LLM expertise and her relationship expertise (though she knows a lot about AI, too!), Her team built Meeno, a relationship mentor that is helping people improve how they approach relationships.
Meeno is not a synthetic romantic partner, like in the movie Her. Instead, its goal is to be like the mentor rat in Ratatouille: It assists individuals in building better relationships. If a user asks Meeno how to handle a breakup, it responds with advice about communicating honestly, empathetically, and clearly. After using it for a while, hopefully, users no longer will need guidance.
I’m excited about Meeno for a few reasons. I have been concerned for some time about the “synthetic boyfriend/girlfriend” industry, where chatbots act like someone’s relationship partner, and then sometimes manipulate people’s emotions for profit in ways that I find deeply troubling (such as offering racy pictures for a fee). Social media, and TV before it, consumes enormous amounts of time that people otherwise might spend building interpersonal relationships. This makes me worry about synthetic romantic partners displacing real ones.
The U.S. Surgeon General has raised the alarm about an epidemic of loneliness and isolation. Loneliness is as bad for a person as smoking 15 cigarettes a day. It’s linked to significantly worse physical and mental health and to premature death. I hope Meeno will have a positive impact on this problem.
Meeno’s journey is still in its early stages. You can read more about it here.
Keep learning!
Andrew
P.S. AI-savvy programmers are coding very differently than they did a year ago: They’re using large language models to help with their work. You’ll learn many of the emerging best practices in “Pair Programming with a Large Language Model,” taught by Laurence Moroney, AI Advocacy Lead at Google and instructor of our TensorFlow Specializations. This short course covers using LLMs to simplify and improve your code, assist with debugging, and minimize technical debt by having AI document and explain your code while you write it. This is an important shift in programming that every developer should stay on top of. Please check out the course here.
News
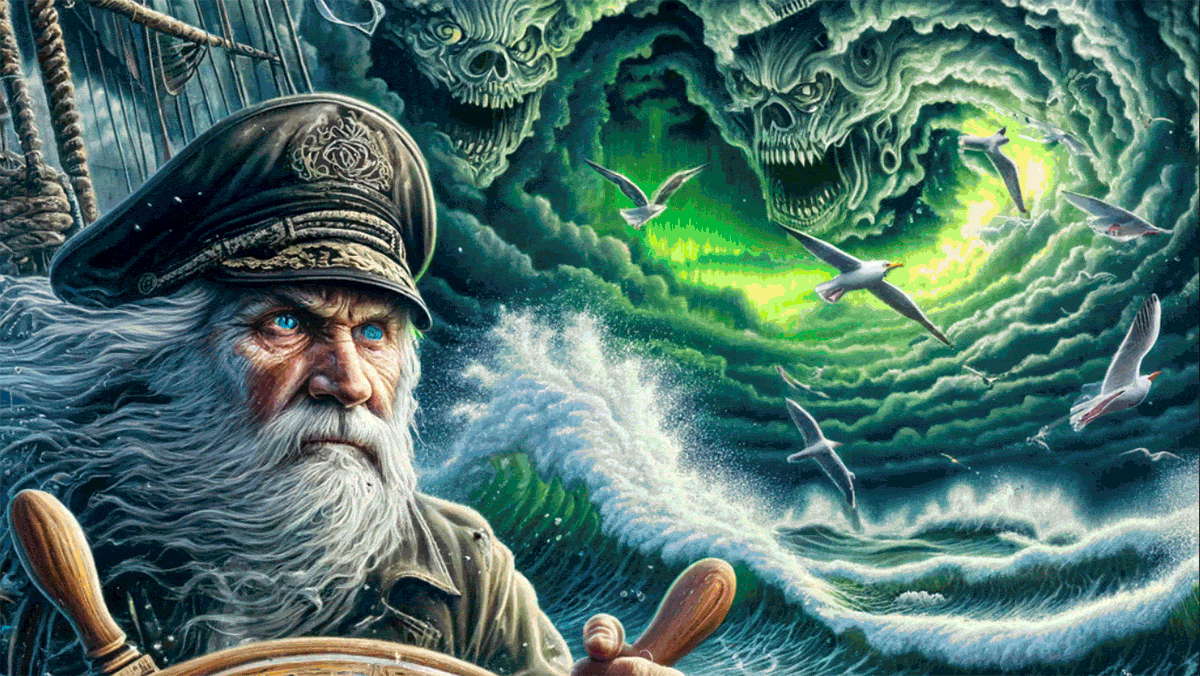
Painting With Text, Voice, and Images
ChatGPT is going multimodal with help from DALL·E.
What’s new: ChatGPT is being geared to accept voice input and output, OpenAI announced. It will also accept and generate images, thanks to integration with DALL·E 3, a new version of the company’s image generator.
How it works: The updates expand ChatGPT into a voice-controlled, interactive system for text and image interpretation and production. New safety features are designed to protect legal rights of artists and public figures.
- Voice input/output will give ChatGPT functionality similar to that of Apple Siri or Amazon Alexa. OpenAI’s Whisper speech recognition system will transcribe voice input into text prompts, and a new text-to-speech model will render spoken output in five distinct voice profiles. Voice interactions will be available to subscribers to the paid ChatGPT Plus and Enterprise services within a couple of weeks.
- A new model called GPT-4 with Vision (GPT-4V) manages ChatGPT’s image input/output, which OpenAI demonstrated at GPT-4’s debut. Users can include images in a conversation to, say, analyze mathematical graphs or plan a meal around the photographed contents of a refrigerator. Like voice, image input/output will be available to paid subscribers within weeks.
- DALL·E 3 will use ChatGPT to refine prompts, and it will generate images from much longer prompts than the previous version. It will produce legible text within images (rather than made-up characters and/or words). Among other safety features, it will decline prompts that name public figures or ask for art in the style of a living artist. The update will be available to paid subscribers in early October, and Microsoft Bing’s Image Creator will switch from DALL·E 2 to DALL·E 3.
- All new functionality eventually will roll out to unpaid and API users.
Yes, but: OpenAI said the new voice and image capabilities are limited to the English language. Moreover, the ability to understand and generate highly technical images is limited.
Behind the news: OpenAI introduced GPT-4 in March with a demo that translated a napkin sketch of a website into code, but Google was first to make visual input and output to a large language model widely available. Google announced visual features at May’s Google I/O conference and the public could use them by midsummer.
Why it matters: ChatGPT has already redefined the possibilities of AI among the general public, businesses, and technical community alike. Voice input opens a world of new applications in any setting where English is spoken, and the coupling of language and vision is bound to spark applications in the arts, sciences, industry, and beyond. DALL·E 3’s safety features sound like an important step forward for image generation.
We’re thinking: The notion of generative models that "do everything" has entered the public imagination. Combining text, voice, and image generation is an exciting step in that direction.
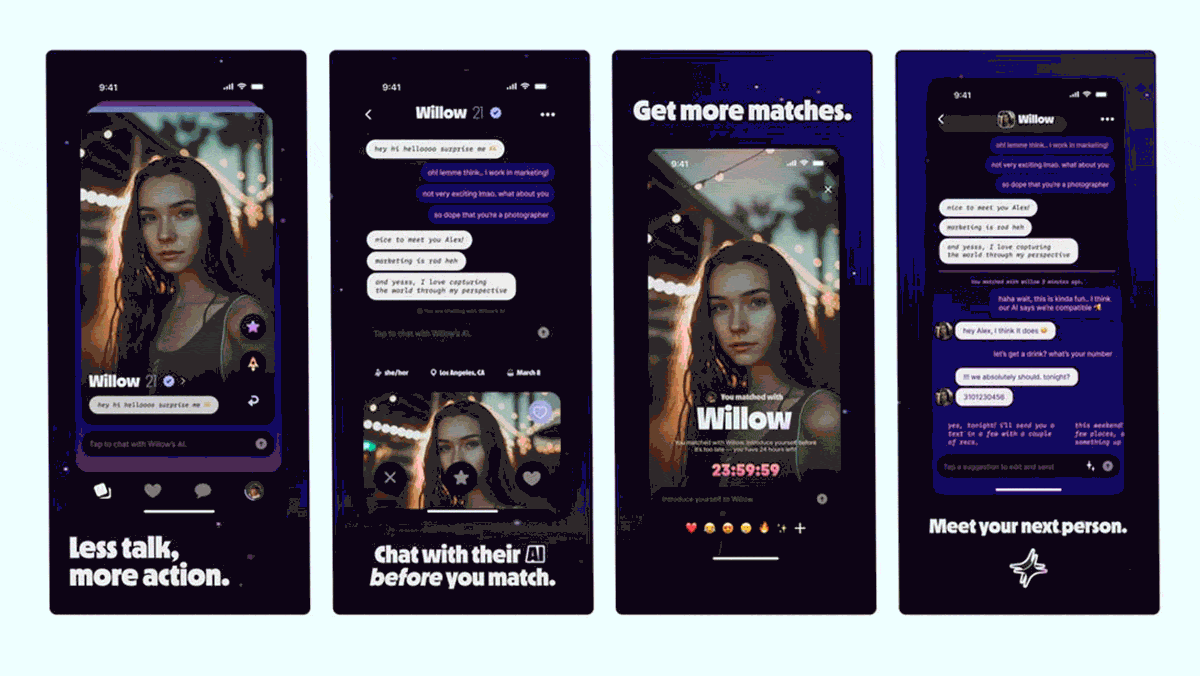
Robots for Romance
AI and dating may be a match made in heaven.
What’s new: Several new apps put deep learning at the center of finding a mate, Bloomberg reported. Some provide chatbot surrogates while others aim to offer matches.
Automated wingmates: The reporter tested four apps, each of which targets a different aspect of budding romance.
- Blush aims to help prospective daters build confidence before jumping into a dating app. Users can flirt with chatbots that express distinct tastes, like “I’m the girl your mother warned you about” and “Love a good conversation with food and wine.” Users can chat with a limited number of characters for free or pay $14.99 monthly for unlimited characters and “dates.”
- The dating app Iris matches portraits of people whom a user finds attractive. Users start by rating faces in a library. An AI system learns the user’s preferences and rates prospective partners’ faces accordingly to return a lineup of the top 2 percent. Users can access up to 10 prospects at a time for free; a premium subscription, which costs $5.99 per month, allows users to view an unlimited number.
- Teaser AI — which its publisher withdrew shortly after its debut —was designed to streamline awkward initial conversations by letting users train a chatbot replica of themselves to engage potential dates before they engaged directly. Users personalized their stand-ins by answering questions like “are you rational or emotional?” and “are you reserved or gregarious?” and conversing with a test chatbot. Teaser AI since has been replaced by a “personal matchmaker” app called Mila.
- If your dating efforts end in heartbreak, Breakup Buddy aims to help you heal. Users can chat with a version of OpenAI’s GPT-3.5 fine-tuned to provide support and advice for moving on. After a three-day free trial, Breakup Buddy costs $18 per month, less for three- and six-month plans.
Behind the news: While dating keeps humans in the loop, some chatbots are designed to replace human interaction entirely. For instance, Anima and Romantic AI offer virtual romantic partners. Replika, an earlier virtual companion service built by the developers of Blush, went platonic in March but shortly afterward re-enabled erotic chat for customers who had signed up before February.
Why it matters: Romance has evolved with communications technology, from handwritten letters to dating apps. Ready or not, AI has joined the lover’s toolkit. For users, the reward may be a lifetime mate. For entrepreneurs, the prize is access to a market worth $8 billion and growing at over 7 percent annually.
We’re thinking: AI has beneficial uses in dating, but users may form emotional bonds with chatbots that businesses then exploit for financial gain. We urge developers to design apps that focus on strengthening human-to-human relationships.
A message from DeepLearning.AI
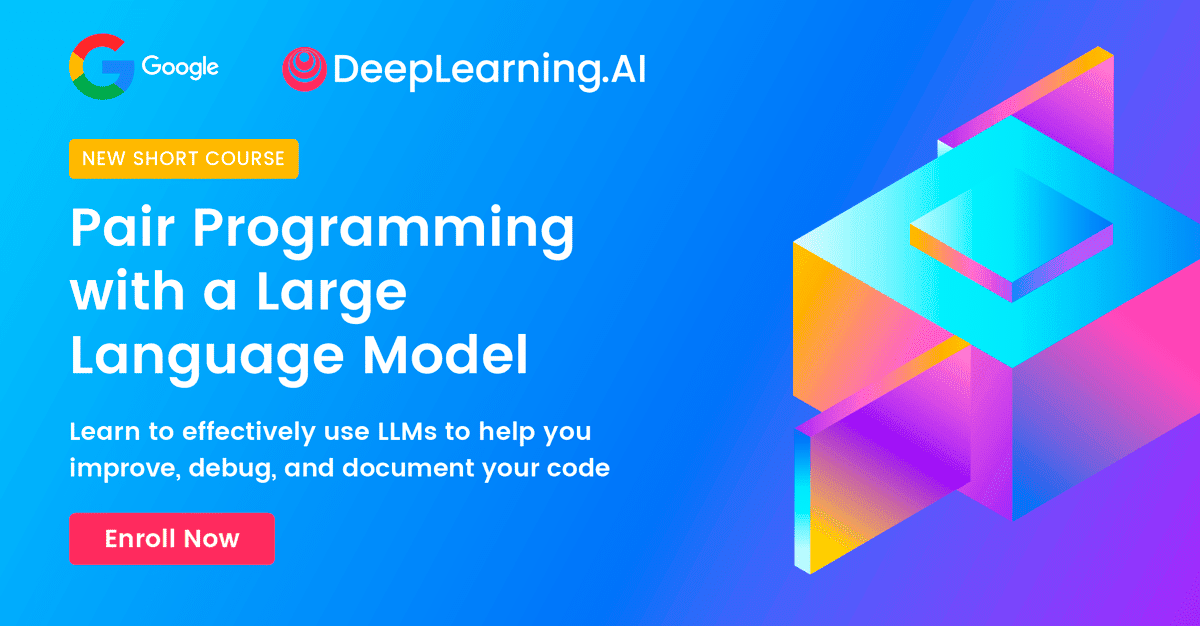
Learn how to prompt a large language model to improve, debug, and document your code in a new short course taught by Google AI Advocacy Lead Laurence Moroney, instructor of our Tensor Flow Specializations. Sign up for free
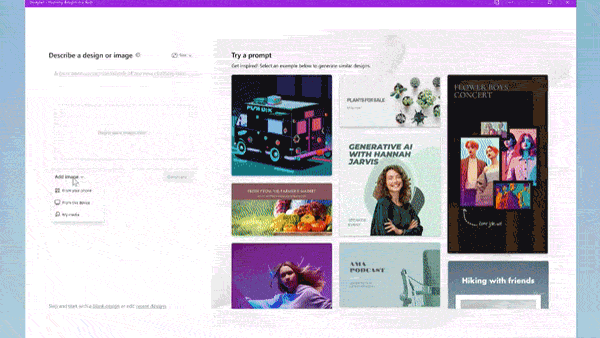
Chatbots for Productivity
Having broken the ice around chat-enabled web search, Microsoft has extended the concept to coding, office productivity, and the operating system itself.
What’s new: Microsoft refreshed its Copilot line of chatbots, adding new features, renaming old ones, and unifying the brand into what it calls an “everyday AI companion.”
How it works: Microsoft offers Copilots for its subsidiary GitHub, Microsoft 365, and Windows.
- GitHub, maker of the original Copilot AI-driven pair programmer, extended the beta-test Copilot Chat feature, which enables users to converse about their code, from enterprise to individual users. Based on a version of GPT-3.5 optimized for code, the system works within Microsoft’s Visual Studio and VS Code applications as well as non-Microsoft development apps Vim, Neovim, and JetBrains. Copilot Chat answers questions, troubleshoots bugs, documents snippets, suggests fixes for security vulnerabilities, and teaches coders how to use unfamiliar languages.
- Microsoft 365 Copilot makes it possible to control Excel, Outlook, PowerPoint, Word, and other productivity apps via text prompts. For instance, in Word, it enables users to summarize documents; in Outlook, to draft emails. It will be available on November 1 to enterprise customers for $30 per user/month in addition to the price of Microsoft 365. The company has an invitation-only pilot program for individual and small business users.
- Windows Copilot is a taskbar chatbot powered by GPT-4. It can open applications, copy and paste among them, query Bing Chat, and integrate third-party plugins. It also provides image generation to media editors that come with Windows including Paint, Photos, and the video editor Clipchamp. Windows Copilot will be available to Windows 11 users as a free update starting September 26.
Behind the news: The emergence of ChatGPT set off a race between Microsoft and Alphabet to integrate large language models into search and beyond. Microsoft seized the day in early February when it launched a version of its Bing search engine that incorporated OpenAI’s technology, and its Copilot strategy has extended that lead. But Alphabet is nipping at Microsoft’s heels. It’s bringing its Bard chatbot to Google productivity apps, from email to spreadsheets.
Why it matters: The combination of large language models and productivity software is a significant step. Microsoft’s approach seems likely to inspire millions of people who have never written a macro or opened the command line to start prompting AI models.
We’re thinking: Copilot is a great concept. It helped make software engineers early adopters of large language models — for writing code, not prose.
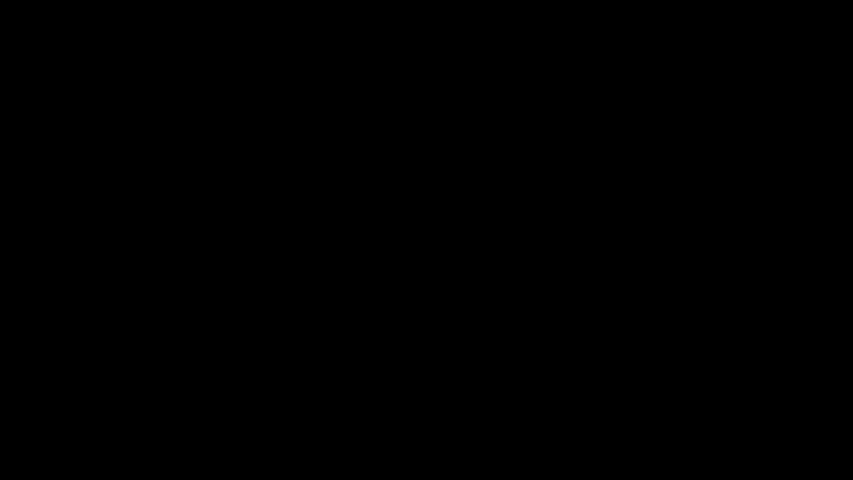
Energy-Efficient Cooling
Google used DeepMind algorithms to dramatically boost energy efficiency in its data centers. More recent work adapts its approach to commercial buildings in general.
What’s new: Jerry Luo, Cosmin Paduraru, and colleagues at Google and Trane Technologies built a [model] https://arxiv.org/abs/2211.07357 that learned, via reinforcement learning, to control the chiller plants that cool large buildings.
Key insight: Chiller plants cool air by running it past cold water or refrigerant. They’re typically controlled according to heuristics that, say, turn on or off certain pieces of equipment if the facility reaches a particular temperature, including constraints that protect against damaging the plant or exposing personnel to unsafe conditions. A neural network should be able to learn more energy-efficient strategies, but it must be trained in the real world (because current simulations don’t capture the complexity involved) and therefore it must adhere rigorously to safety constraints. To manage safety, the model can learn to predict the chiller plant’s future states, and a hard-coded subroutine can deem them safe or unsafe, guiding the neural network to choose only safe actions.
How it works: The authors built separate systems to control chiller plants in two large commercial facilities. Each system comprised an ensemble of vanilla neural networks plus a safety module that enforced safety constraints. Training took place in two phases. In the first, the ensemble trained on data produced by a heuristic controller. In the second, it alternated between training on data produced by itself and the heuristic controller.
- The authors collaborated with domain experts to determine a chiller plant’s potential actions and states. Actions comprised 12 behaviors such as switching on a component or setting a water chiller’s temperature. States consisted of measurements taken every 5 minutes by 50 sensors (temperature, water flow rate, on/off status of various components, and so on). They also identified unsafe actions (such as setting the temperature of the water running through a chiller to below 40 degrees) and unsafe states (such as a drop in ambient air temperature below 45 degrees).
- The authors trained the ensemble on a year’s worth of data from the chiller plant’s heuristic controller via reinforcement learning, penalizing actions depending on how much energy they consumed. Given an action, it learned to predict (i) the energy cost of that action and (ii) the plant’s resulting state 15 minutes later.
- For three months, they alternated between controlling the chiller plant using the ensemble for one day and the heuristic controller for one day. They recorded the actions and resulting states and added them to the training set. At the end of each day, they retrained the ensemble on the accumulated data. Alternating day by day made it possible to compare the performance of the ensemble and heuristic controller under similar conditions.
- During this period, the safety module blocked the system from taking actions that were known to be unsafe and actions the ensemble predicted to result in an unsafe state. Of the remaining actions, the ensemble predicted the one that would consume the least energy. In most cases, it took that action. Occasionally, it took a different action, so it could discover strategies that were more energy-efficient than those it learned from the heuristic controller.
Results: Alternating with the heuristics controller for three months in the two buildings, the authors’ method achieved energy savings of 9 percent and 13 percent, respectively, relative to the heuristic controller. Furthermore, the system made the chiller plants more efficient in interesting ways. For example, it learned to produce colder water, which consumed more energy up front but reduced the overall consumption.
Yes, but: The environment within the buildings varied over the three-month period with respect to factors like temperature and equipment performance. This left the authors unable to tell how much improvement to attribute to their system versus confounding factors.
Why it matters: Using reinforcement-learning algorithms to control expensive equipment requires significant domain expertise to account for variables like sensor calibration, maintenance schedules, and safety rules. Working closely with domain experts when applying such algorithms can maximize both efficiency and safety.
We’re thinking: Deep learning is cooler than ever!
A Message from Workera
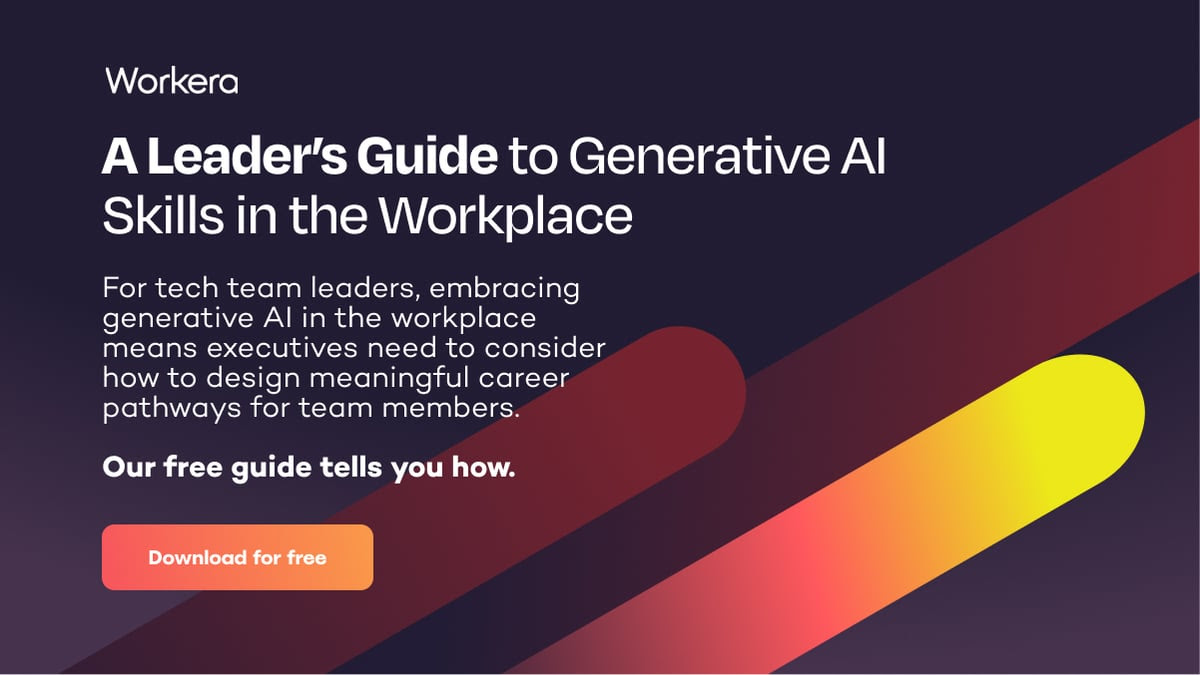
According to Forbes, between 70 percent and 95 percent of enterprises are failing in their business transformations. Skills gaps are a major cause. Our new guide tells leaders how to avoid this trap. Read it and get your business transformation on track!
Data Points
Google partners with Howard University on Project Elevate Black Voices
Speakers of African-American English (AAE) are not well-served by existing speech recognition technology, and often have to code-switch to be understood by voice interfaces. Google Research and Howard will team technologists with ethnolinguists to better understand both the limits of Google's current voice data and issues of power and pragmatics in language. Howard will own the new data set. (Google)
Authors' Guild sues OpenAI for copyright infringement
More than a dozen authors, including John Grisham, Jodi Picoult, and Jonathan Franzen, sued the makers of ChatGPT for training models on their in-copyright books without permission. Another lawsuit by the Authors' Guild against Google Books in 2005 led to a rejected 2010 settlement and a landmark 2015 fair use decision. (The New York Times)
The Commonwealth of Pennsylvania teams with Carnegie Mellon to develop automation technologies for state government
Governor Josh Shapiro announced his administration would convene a governing board, publish principles on the use of AI, and develop training programs for state employees. “We don’t want to let AI happen to us,” Shapiro said. “We want to be part of helping develop AI for the betterment of our citizens.” (Associated Press)
Lenovo brings AI to the edge for businesses with limited resources
The computer maker touted its hardware and software solutions for industries as diverse as commercial fishing, retail, and manufacturing. The goal is to offer computer vision, audio recognition, prediction, security, and virtual assistants for systems without requiring much computing power or programming skills. (Lenovo)
Intel partners with Stability AI to build a supercomputer
The chipmaker looks to catch up to NVIDIA and other competitors with its newest datacenter and PC designs. CEO Pat Gelsinger argued that AI was central to the “Siliconomy,” a “growing economy enabled by the magic of silicon and software.” (Intel)
Amazon adds more generative AI to Alexa
New Echo smart home devices will be powered by a new large language model. Amazon claims Alexa will be more conversational, more nuanced in its understanding of language, and more proactive in its responses to changing conditions. Former Microsoft product chief Panos Panay will be responsible for the next iteration of Echo and Alexa, replacing outgoing product head Dave Limp. (Amazon)
Big Pharma embraces machine learning to guide and interpret human drug trials
"Companies such as Amgen, Bayer, and Novartis are training AI to scan billions of public health records, prescription data, medical insurance claims, and their internal data to find trial patients - in some cases halving the time it takes to sign them up." (Reuters)
New consumer tools still make a lot of mistakes
Google said its Bard chatbot can summarize files from Gmail and Google Docs, but users showed it falsely making up emails that were never sent. OpenAI heralded its new Dall-E 3 image generator, but people on social media soon pointed out that the images in the official demos missed some requested details. And Amazon announced a new conversational mode for Alexa, but the device repeatedly messed up in a demo. including recommending a museum in the wrong part of the country. (Washington Post).