Dear friends,
On Monday, a number of large music labels sued AI music makers Suno and Udio for copyright infringement. Their lawsuit echoes The New York Times’ lawsuit against OpenAI in December. The question of what’s fair when it comes to AI software remains a difficult one.
I spoke out in favor of OpenAI’s side in the earlier lawsuit. Humans can learn from online articles and use what they learn to produce novel works, so I’d like to be allowed to use AI to do so. Some people criticized my view as making an unjustifiable equivalence between humans and AI. This made me realize that people have at least two views of AI: I view AI as a tool we can use and direct to our own purposes, while some people see it as akin to a separate species, distinct from us, with its own goals and desires.
If I’m allowed to build a house, I want to be allowed to use a hammer, saw, drill, or any other tool that might get the job done efficiently. If I’m allowed to read a webpage, I’d like to be allowed to read it with any web browser, and perhaps even have the browser modify the page’s formatting for accessibility. More generally, if we agree that humans are allowed to do certain things — such as read and synthesize information on the web — then my inclination is to let humans direct AI to automate this task.
In contrast to this view of AI as a tool, if someone thinks humans and AI are akin to separate species, they’ll frame the question differently. Few people today think all species should have identical rights. If a mosquito annoys a human, the mosquito can be evicted (or worse). In this view, there’s no reason to think that, just because humans are allowed to do something, AI should be allowed to do it as well.
To be clear, just as humans aren’t allowed to reproduce large parts of copyrighted works verbatim (or nearly verbatim) without permission, AI shouldn’t be allowed to do so either. The lawsuit against Suno and Udio points out that, when prompted in a particular way, these services can nearly reproduce pieces of copyrighted music.
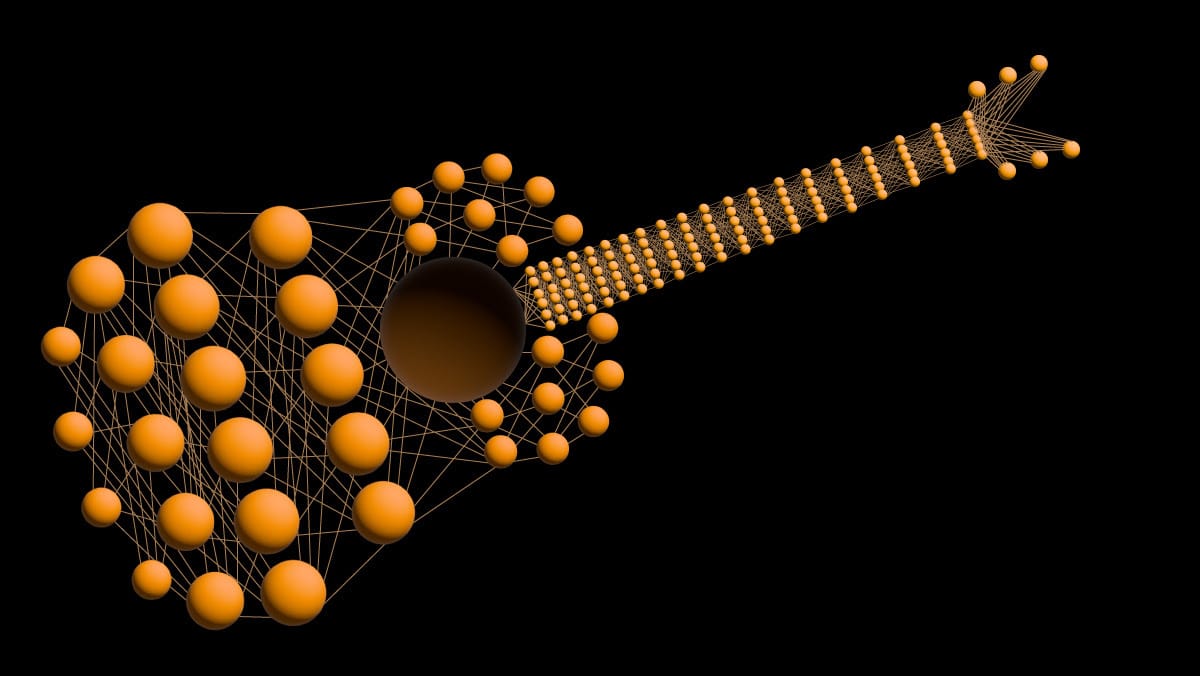
But here, too, there are complex issues. If someone were to use a public cloud to distribute online content in violation of copyright, typically the person who did that would be at fault, not the cloud company (so long as the company took reasonable precautions and didn’t enable copyright infringement deliberately). The plaintiffs in the lawsuit against Suno and Udio managed to write prompts that caused the systems to reproduce copyrighted work. But is this like someone managing to get a public cloud to scrape and distribute content in a way that violates copyright? Or is this — as OpenAI said — a rare bug that AI companies are working to eliminate? (Disclaimer: I’m not a lawyer and I’m not giving legal advice.)
Humans and software systems use very different mechanisms for processing information. So in terms of what humans can do — and thus what I’d like to be allowed to use software to help me do — it’s helpful to consider the inputs and outputs. Specifically, if I’m allowed to listen to a lot of music and then compose a novel piece of music, I would like to be allowed to use AI to implement a similar input-to-output mapping. The process for implementing this mapping may be training a neural network on music that’s legally published on the open internet for people to enjoy without encumbrances.
To acknowledge a weakness of my argument, just because humans are allowed to emit a few pounds of carbon dioxide per day simply by breathing doesn’t mean we should allow machines to emit massively more carbon dioxide without restrictions. Scale can change the nature of an act.
When I was a high-school student in an internship job, I spent numerous hours photocopying, and I remember wishing I could automate that repetitive work. Humans do lots of valuable work, and AI, used as a tool to automate what we do, will create lots of value. I hope we can empower people to use tools to automate activities they’re allowed to do, and erect barriers to this only in extraordinary circumstances, when we have clear evidence that it creates more harm than benefit to society.
Keep learning!
Andrew
A MESSAGE FROM DEEPLEARNING.AI
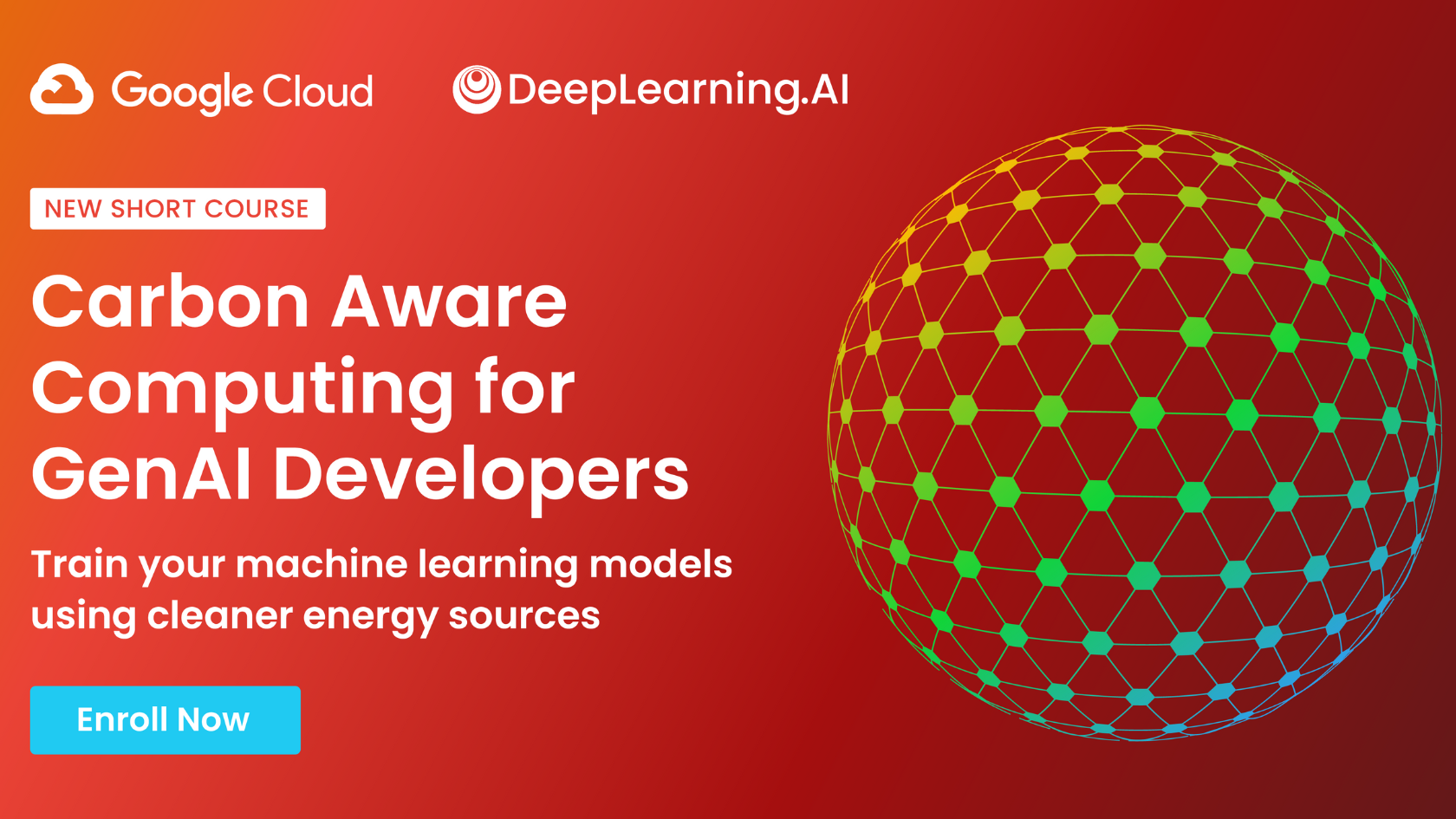
Learn to reduce the carbon footprints of your AI projects in “Carbon Aware Computing for GenAI Developers,” a new course built in collaboration with Google Cloud. Perform model training and inference jobs with cleaner, low-carbon energy and make your AI development greener! Join today
News
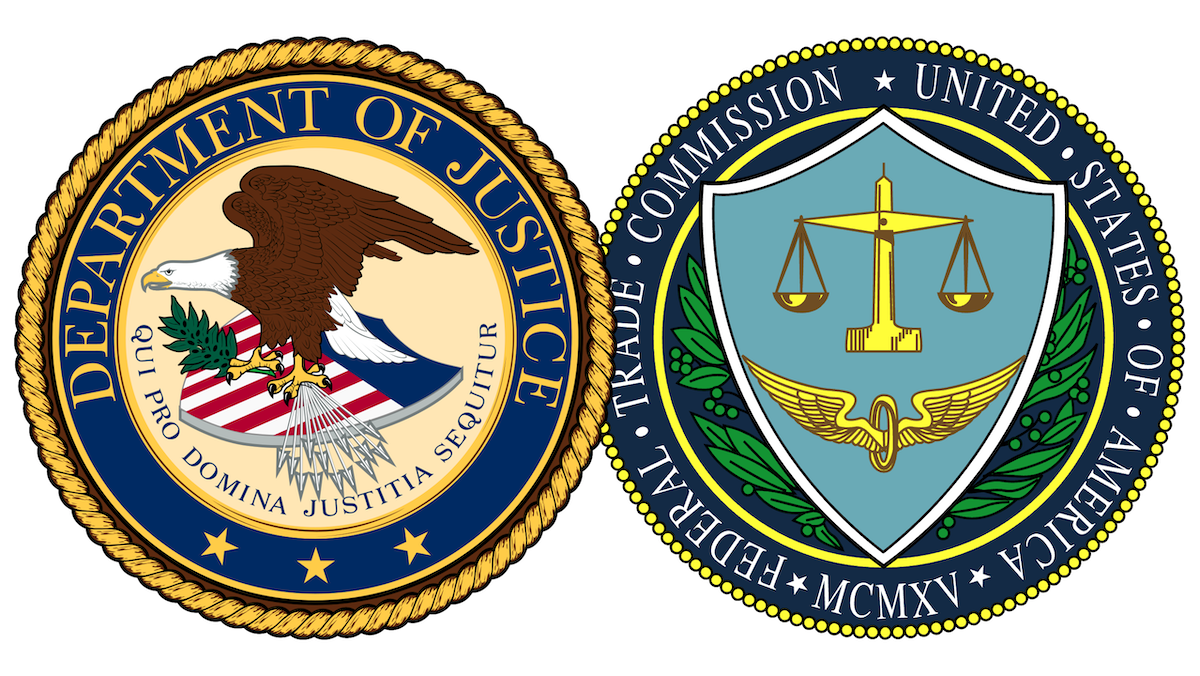
U.S. to Probe AI Monopoly Concerns
U.S. antitrust regulators are preparing to investigate a trio of AI giants.
What’s new: Two government agencies responsible for enforcing United States anti-monopoly laws agreed to investigate Microsoft, Nvidia, and OpenAI, The New York Times reported.
How it works: The Department of Justice (DOJ) will investigate Nvidia, which dominates the market for chips that train and run neural networks. The Federal Trade Commission (FTC) will probe Microsoft and its relationship with OpenAI, which together control the distribution of OpenAI’s popular GPT-series models. In February, FTC chair Lina Khan said the agency would look for possible anti-competitive forces in the AI market.
- The DOJ is concerned that Nvidia may use unfair practices to maintain its market dominance. They may look into Nvidia’s CUDA software, which strengthens users’ reliance on its chips. They may also explore claims raised by French authorities that Nvidia favors some cloud computing firms over others.
- The FTC worries that the partnership between OpenAI and Microsoft, which owns 49 percent of OpenAI and holds a non-voting seat on OpenAI’s board of directors, may work to limit consumer choice. Microsoft’s April agreement with Inflection AI to hire most of its staff in return for a $650 million payment, which resembled an acquisition but left Inflection’s corporate structure intact, raised suspicions that the deal had been structured to avoid automatic antitrust scrutiny.
- The FTC previously investigated investments in Anthropic by Amazon and Google as well as whether OpenAI gathered training data in ways that harmed consumers.
Behind the news: Government attention to top AI companies is rising worldwide. Microsoft’s partnership with OpenAI faces additional scrutiny by European Union regulators, who are probing whether the relationship violates EU regulations that govern corporate mergers. U.K. regulators are investigating Amazon’s relationship with Anthropic and Microsoft’s relationship with Mistral and Inflection AI. Last year, French regulators raided an Nvidia office over suspected anti-competitive practices. In 2022, Nvidia withdrew a bid to acquire chip designer Arm Holdings after the proposal attracted international regulatory scrutiny including an FTC lawsuit.
Why it matters: Microsoft, Nvidia, and OpenAI have put tens of billions of dollars each into the AI market, and lawsuits, settlements, judgments, or other interventions could shape the fate of those investments. The FTC and DOJ similarly divided their jurisdictions in 2019, resulting in investigations into — and ongoing lawsuits against — Amazon, Apple, Google, and Meta for alleged anti-competitive practices in search, social media, and consumer electronics. Their inquiries into the AI market could have similar impacts.
We’re thinking: Governments must limit unfair corporate behavior without stifling legitimate activities. Recently, in the U.S. and Europe, the pendulum has swung toward overly aggressive enforcement. For example, government opposition to Adobe’s purchase of Figma had a chilling effect on acquisitions that seems likely to hurt startups. The UK blocked Meta’s acquisition of Giphy, which didn’t seem especially anticompetitive. We appreciate antitrust regulators’ efforts to create a level playing field, and we hope they’ll take a balanced approach to antitrust.
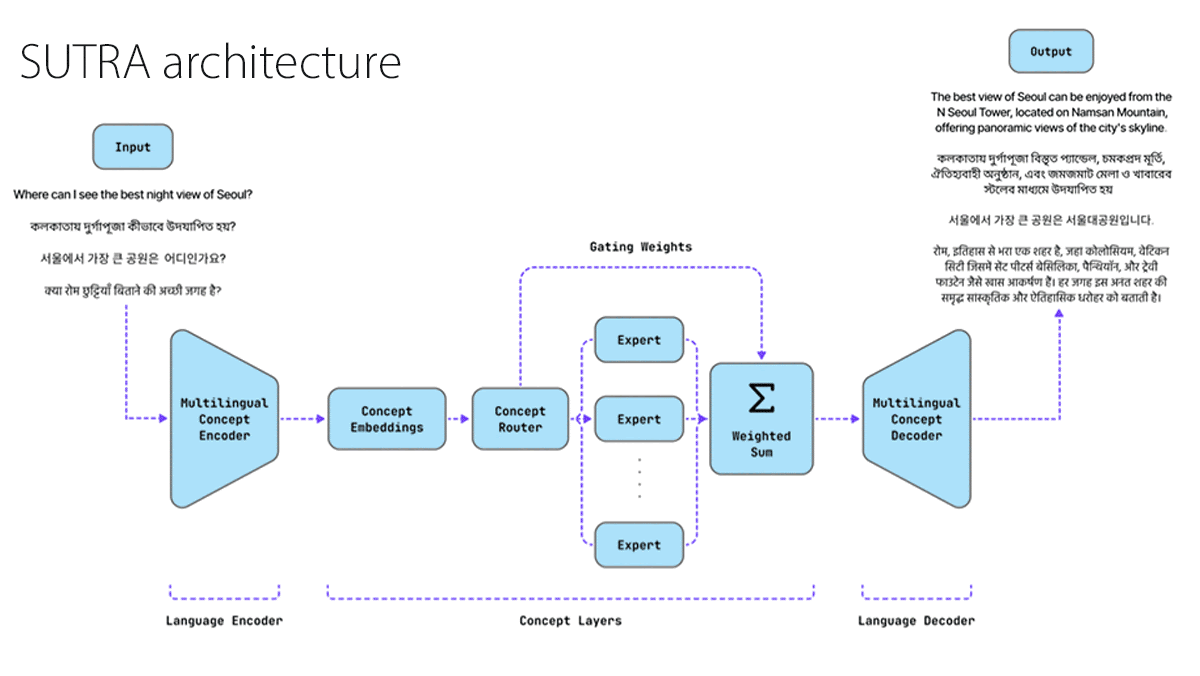
Chatbot for Minority Languages
An AI startup that aims to crack markets in southern Asia launched a multilingual competitor to GPT-4.
What’s new: The company known as Two AI offers SUTRA, a low-cost language model built to be proficient in more than 30 languages, including underserved South Asian languages like Gujarati, Marathi, Tamil, and Telugu. The company also launched ChatSUTRA, a free-to-use web chatbot based on the model.
How it works: SUTRA comprises two mixture-of-experts transformers: a concept model and an encoder-decoder for translation. A paper includes some technical details, but certain details and a description of how the system fits together are either absent or ambiguous.
- The concept model learned to predict the next token. The training dataset included publicly available datasets in a small number of languages for which abundant data is available, including English.
- Concurrently, the translation model learned to translate 100 million human- and machine-translated conversations among many languages. This model learned to map concepts to similar embeddings across all languages in the dataset.
- The authors combined the two models, so the translation model’s encoder fed the concept model, which in turn fed the translation model’s decoder, and further trained them together. More explicitly, during this stage of training and at inference, the translation model’s encoder receives text and produces an initial embedding. The concept model processes the embedding and delivers its output to the translation model’s decoder, which produces the resulting text.
- SUTRA is available via an API in versions that are designated Pro (highest-performing), Light (lowest-latency), and Online (internet-connected). SUTRA-Pro and SUTRA-Online cost $1 per 1 million tokens for input and output. SUTRA-Light costs $0.75 per 1 million tokens.
Results: On multilingual MMLU (a machine-translated version of multiple-choice questions that cover a wide variety of disciplines), SUTRA outperformed GPT-4 in four of the 11 languages for which the developer reported the results: Gujarati, Marathi, Tamil, and Telugu. Moreover, SUTRA’s tokenizer is highly efficient, making the model fast and cost-effective. In key languages, it compares favorably to the tokenizer used with GPT-3.5 and GPT-4, and even narrowly outperforms GPT-4o’s improved tokenizer, according to Two AI’s tokenizer comparison space on HuggingFace. In languages such as Hindi and Korean that are written in non-Latin scripts and for which GPT-4 performs better on MMLU, SUTRA’s tokenizer generates less than half as many tokens as the one used with GPT-3.5 and GPT-4, and slightly fewer than GPT-4o’s tokenizer.
Yes, but: Multilingual MMLU tests only 11 of SUTRA’s 33 languages, making it difficult to fully evaluate the model’s multilingual performance.
Behind the news: Two AI was founded in 2021 by Pranav Mistry, former president and CEO of Samsung Technology & Advanced Research Labs. The startup has offices in California, South Korea, and India. In 2022, it raised $20 million in seed funding from Indian telecommunications firm Jio and South Korean internet firm Naver. Mistry aims to focus on predominantly non-English-speaking markets such as India, South Korea, Japan, and the Middle East, he told Analytics India.
Why it matters: Many top models work in a variety of languages, but from a practical standpoint, multilingual models remain a frontier in natural language processing. Although SUTRA doesn’t match GPT-4 in all the languages reported, its low price and comparatively high performance may make it appealing in South Asian markets, especially rural areas where people are less likely to speak English. The languages in which SUTRA excels are spoken by tens of millions of people, and they’re the most widely spoken languages in their respective regions. Users in these places have yet to experience GPT-4-level performance in their native tongues.
We’re thinking: Can a newcomer like Two AI compete with OpenAI? If SUTRA continues to improve, or if it can maintain its cost-effective service, it may yet carve out a niche.
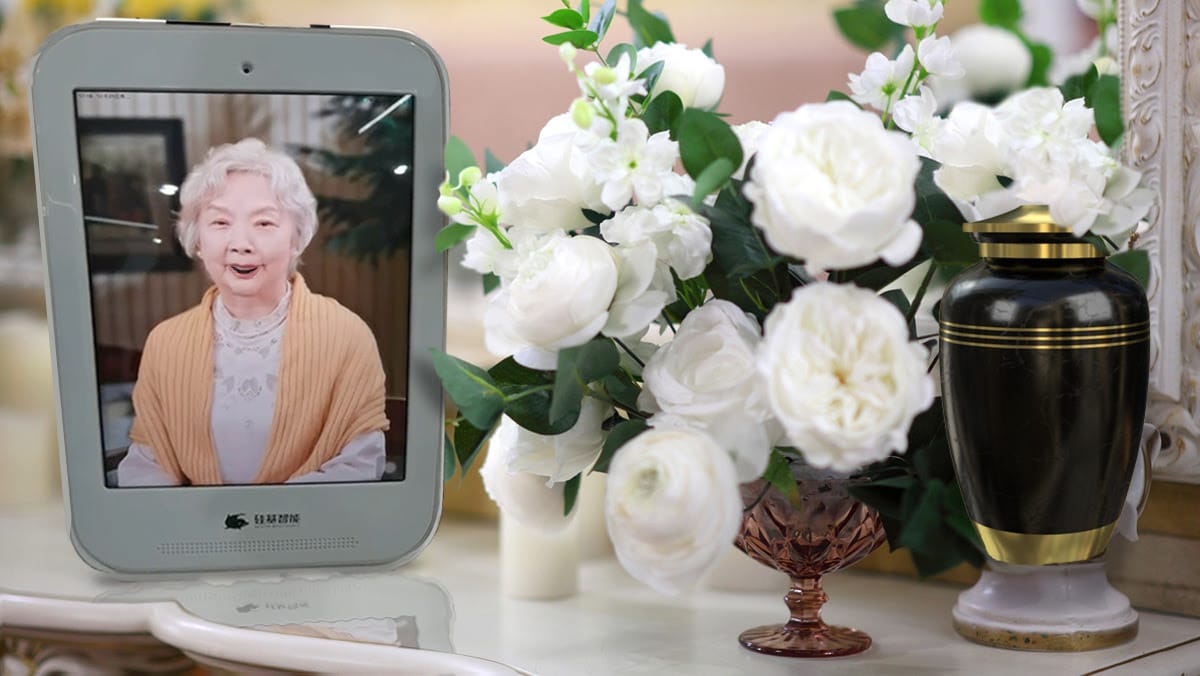
Conversing With the Departed
Advances in video generation have spawned a market for lifelike avatars of deceased loved ones.
What’s new: Several companies in China produce interactive videos that enable customers to chat with animated likenesses of dead friends and relatives, MIT Technology Review reported.
How it works: Super Brain and Silicon Intelligence have built such models for several thousand customers. They provide a modern equivalent of portrait photos of deceased relatives and a vivid way to commune with ancestors.
- The developers use undisclosed tools to stitch photos, videos, audio recordings, and writings supplied by customers into interactive talking-head avatars of deceased loved ones.
- The cost has dropped dramatically. In December 2023, Super Brain charged between $1,400 and $2,800 for a basic chat avatar wrapped in a phone app. Today it charges between $700 and $1,400 and plans eventually to drop the price to around $140. Silicon Intelligence charges between several hundred dollars for a phone-based avatar to several thousand for one displayed on a tablet.
Behind the news: The desire to interact with the dead in the form of an AI-generated avatar is neither new nor limited to China. In the U.S., the startup HereAfter AI builds chatbots that mimic the deceased based on interviews conducted while they were alive. Another startup, StoryFile, markets similar capabilities to elders (pitched by 93-year-old Star Trek star William Shatner) to keep their memory alive for younger family members. The chatbot app Replika began as a project by founder Eugenia Kuyda to virtually resurrect a friend who perished in a car accident in 2015.
Yes, but: In China, language models struggle with the variety of dialects spoken by many elders.
Why it matters: Virtual newscasters and influencers are increasingly visible on the web, but the technology has more poignant uses. People long to feel close to loved ones who are no longer present. AI can foster that sense of closeness and rapport, helping to fulfill a deep need to remember, honor, and consult the dead.
We’re thinking: No doubt, virtual avatars of the dead can bring comfort to the bereaved. But they also bring the risk that providers might manipulate their customers’ emotional attachments for profit. We urge developers to focus on strengthening relationships among living family and friends.
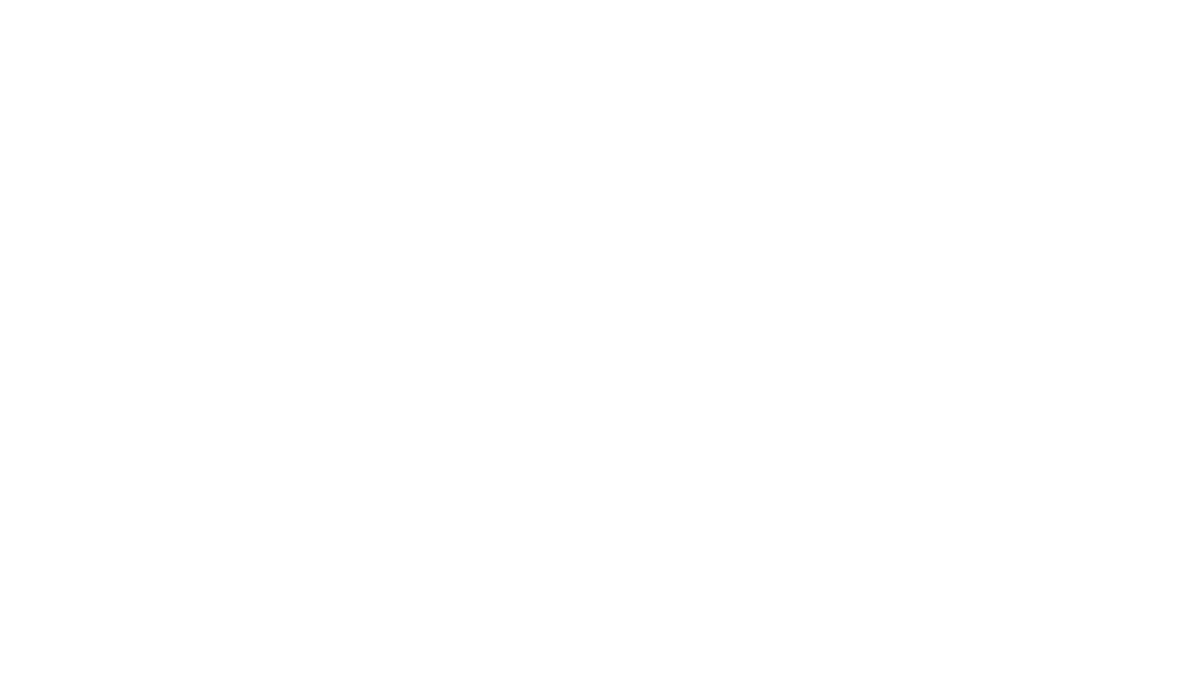
Benchmarks for Agentic Behaviors
Tool use and planning are key behaviors in agentic workflows that enable large language models (LLMs) to execute complex sequences of steps. New benchmarks measure these capabilities in common workplace tasks.
What’s new: Recent benchmarks gauge the ability of a large language model (LLM) to use external tools to manipulate corporate databases and to plan events such as travel and meetings.
Tool use: Olly Styles, Sam Miller, and colleagues at Mindsdb, University of Warwick, and University of Glasgow proposed WorkBench, which tests an LLM’s ability to use 26 software tools to operate on five simulated workplace databases: email, calendar, web analytics, projects, and customer relationship management. Tools include deleting emails, looking up calendar events, creating graphs, and looking up tasks in a to-do list.
- The benchmark includes 690 problems that require using between zero to 12 tools to succeed. It evaluates individual examples based on whether the databases changed as expected after the final tool had been called (rather than simply whether particular tools were used, as in earlier work). In this way, a model can use tools in any sequence and/or revise its initial choices if they prove unproductive and still receive credit for responding correctly.
- Upon receiving a problem, models are given a list of all tools and an example of how to use each one. Following the ReAct prompting strategy, they’re asked first to reason about the problem and then use a tool. After they’ve received a tool’s output (typically either information or an error message), they’re asked to reason again and choose another tool. The cycle of reasoning, tool selection, and receiving output repeats until the model decides it doesn’t need to use another tool.
- The authors evaluated GPT-4, GPT-3.5, Claude 2, Llama2-70B, and Mixtral-8x7B. GPT-4 performed the best by a large margin: It modified the databases correctly 43 percent of the time. The closest competitor, Claude 2, modified the databases correctly 26 percent of the time.
Planning: Huaixiu Steven Zheng, Swaroop Mishra, Hugh Zhang, and colleagues at Google published Natural Plan, a benchmark that evaluates an LLM’s ability to (i) plan trips, (ii) arrange a series of meeting times and locations, and (iii) schedule a group meeting. Each example has only one solution.
- The benchmark includes 1,600 prompts that ask the model to plan a trip based on an itinerary of cities, time to be spent in each city, total duration of the trip, days when other people are available to meet, and available flights between cities.
- 1,000 prompts ask the model to plan a schedule to meet as many people as possible. The prompts include places, times when people will be in each place, and how long it takes to drive from one place to another.
- 1,000 prompts ask the model, given the existing schedules of a number of people, to find a good time for them to meet.
- The authors tested GPT 3.5, GPT-4, GPT-4o, Gemini 1.5 Flash, and Gemini 1.5 Pro, using five-shot prompts (that is, providing five examples for context). Gemini 1.5 Pro achieved the highest scores on planning trips (34.8 percent) and scheduling group meetings (48.9 percent). GPT-4 ranked second for planning trips (31.1), and GPT-4o ranked second for scheduling meetings (43.7 percent). GPT-4 dominated in arranging meetings (47 percent), followed by GPT-40 (45.2 percent).
Why it matters: When building agentic workflows, developers must decide on LLM choices, prompting strategies, sequencing of steps to be carried out, tool designs, single- versus multi-agent architectures, and so on. Good benchmarks can reveal which approaches work best.
We're thinking: These tests have unambiguous right answers, so agent outputs can be evaluated automatically as correct or incorrect. We look forward to further work to evaluate agents that generate free text output.