Dear friends,
When entrepreneurs build a startup, it is often their speed and momentum that gives them a shot at competing with the tech behemoths. This is true of countries as well.
I was recently in Thailand, where I was delighted to see tremendous momentum building in AI (and sip the best Thai ice tea I’ve ever tasted). Even though Thailand is not as advanced in AI technology or applications as leading tech countries, the enthusiasm for building AI throughout government, corporations, and academia was thrilling. I came away heartened that AI’s benefits will be spread among many countries and convinced that one’s level of AI development right now matters less than your momentum toward increasing it.
Seeing the momentum behind AI in Thailand — where the per capita GDP is around one fifth that of Japan, and one tenth that of the United States — left me feeling that any country, company, or person has a shot at doing meaningful work in the field. While advanced economies such as the U.S. and China are still in the lead, generative AI has made the playing field more level. Foundation models, especially those with open weights, are significantly lowering the barriers to building meaningful AI projects. In Thailand, a lot of people I met weren’t just talking about AI, they were rolling up their sleeves and building. That buys a nation a lot more momentum than just talk.
I met with Prime Minister Srettha Thavisin and his Ministers of Higher Education and Education (primary/secondary) along with many staffers. It was delightful to hear the PM speak of his enthusiasm for AI. The ministers discussed how to (i) provide AI training and (ii) use AI to improve education in a variety of subjects. Happily, the focus was on creating value while thinking through realistic risks like AI’s potential to proliferate misinformation, and not a single person asked me about whether AI will lead to human extinction!
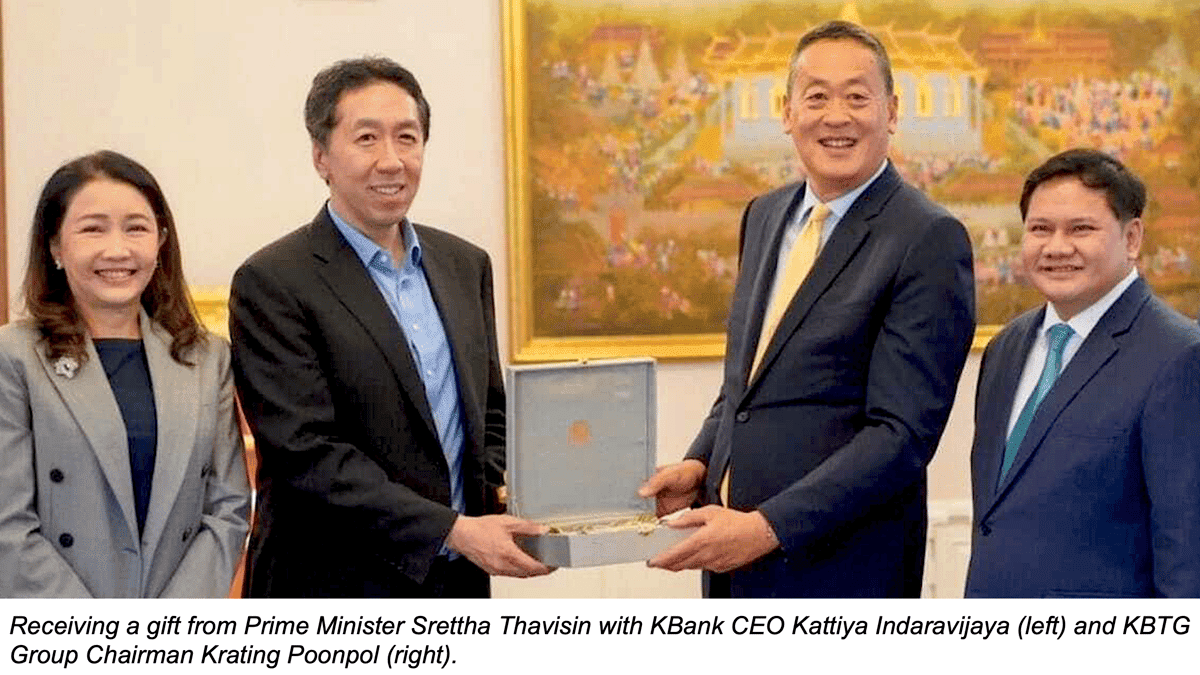
I also met with many business leaders and enjoyed seeing a rapid pace of experimentation with AI. KBTG, an affiliate of the country’s leading digital bank KBank, is working on a financial chatbot advisor, AI-based identity verification for anti-fraud, AI for auto insurance, and a Thai-language financial large language model. These features are growing mobile banking and increasing financial access. Many business leaders in other sectors, too, have asked their teams to run experiments. There are many AI applications yet to be built in industrial sectors, tourism, trade, and more! (KBTG is an investor in AI Fund, which I lead.)
I often visit universities in both developed and developing economies, and I’ve been surprised to see that universities in developing economies sometimes adopt AI faster. At Chulalongkorn University (known as Chula), I met with the University President Wilert Puriwat and Director of Chula AI Professor Proadpran Punyabukkana. Chula AI has rolled out campus-wide training in generative AI for faculty, staff, and students. In addition, it supports building AI applications such as AI screening for depression and gastrointestinal cancer.
It takes years to build up advanced technology. But momentum matters, and there will be many rewards along the journey. There’s no time like the present to start building!
Keep building,
Andrew
A MESSAGE FROM DEEPLEARNING.AI
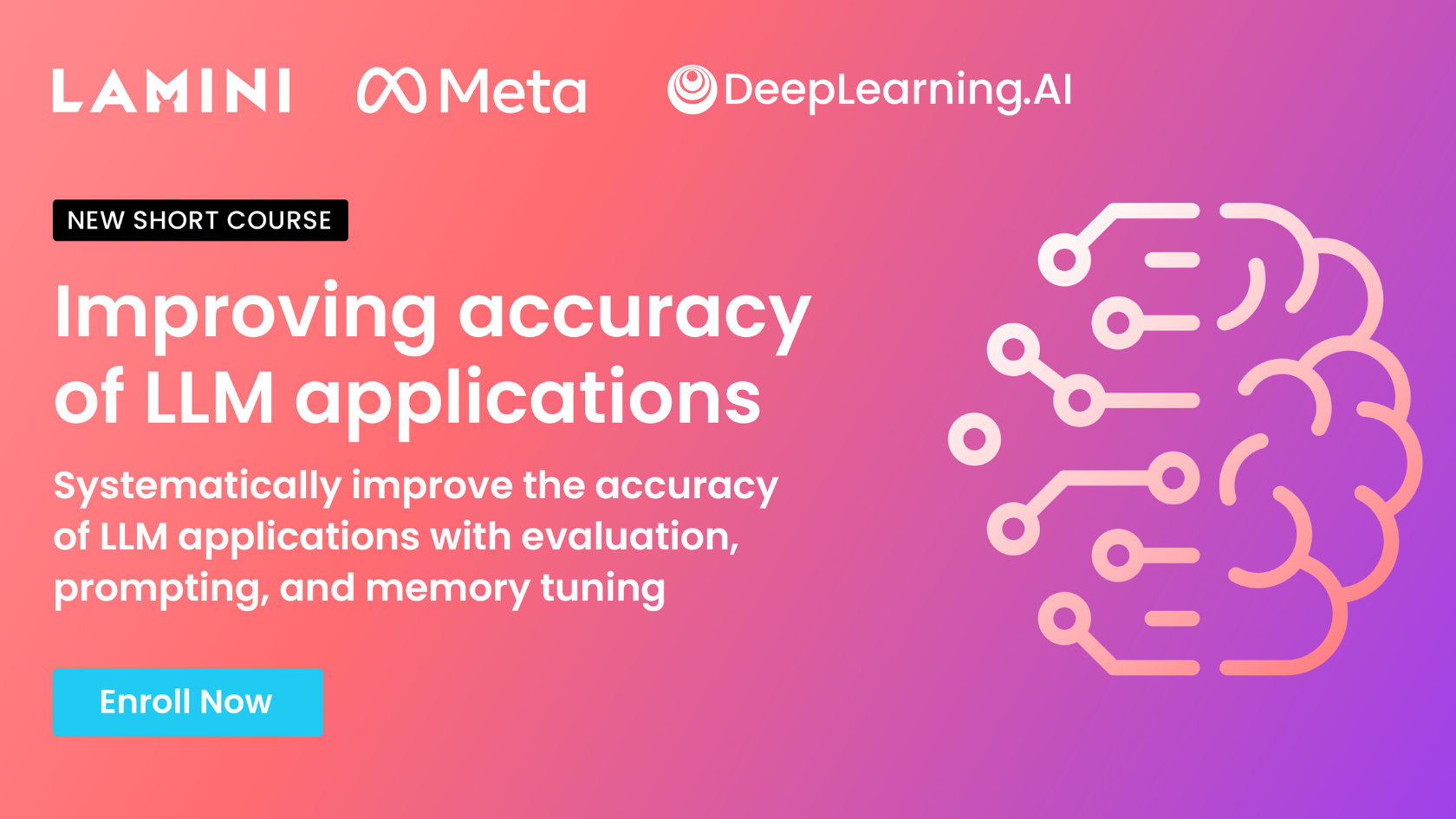
Our short course “Improving Accuracy of LLM Applications” teaches a step-by-step approach to improving the accuracy of applications built on large language models. You’ll build an evaluation framework, incorporate self-reflection, and fine-tune models using LoRA and memory tuning to embed facts and reduce hallucinations. Enroll for free
News
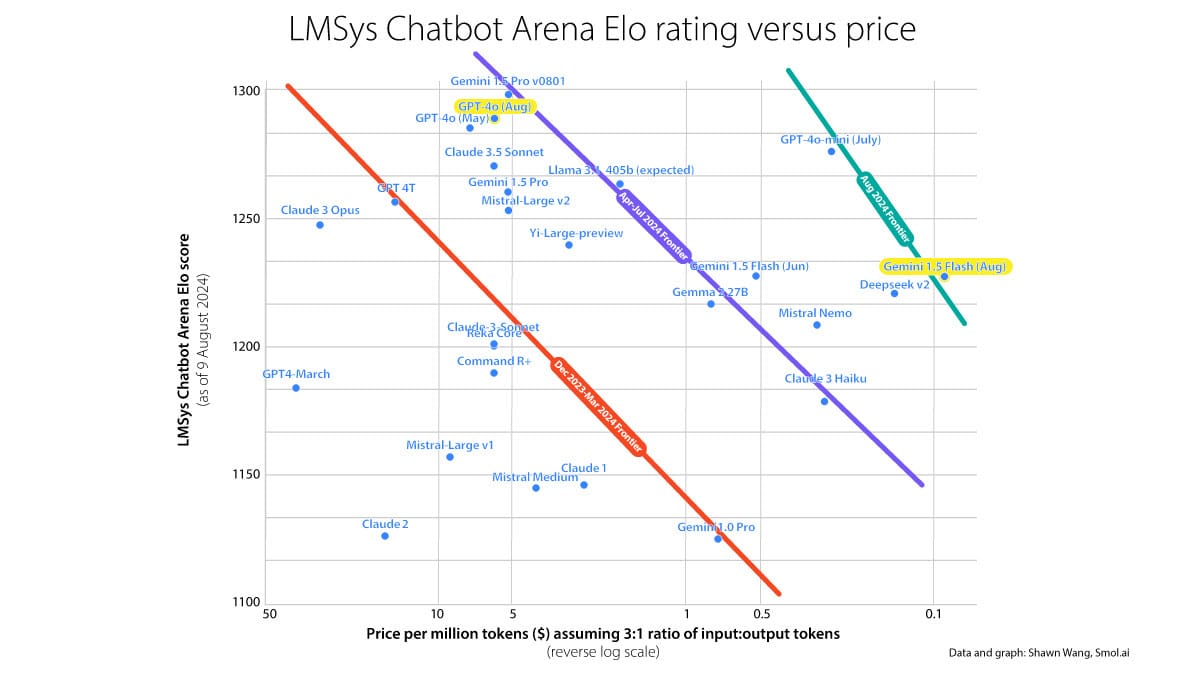
Higher Performance, Lower Prices
Prices for access to large language models are falling as providers exploit new efficiencies and compete for new customers.
What’s new: Open AI cut the price of calls to GPT-4o’s API by 50 percent for input tokens and 33 percent for output tokens, with an even steeper discount for asynchronous processing. Not to be outdone, Google cut the price of API calls to Gemini 1.5 Flash by approximately 75 percent.
How it works: The latest price reductions follow a steady trend, tracked by Smol.ai CEO Shawn Wang, in which providers are charging less even as model performance (as measured by LMSys’s Chatbot Arena Leaderboard Elo ratings) rises. Here’s a list of recent prices in order of each model’s rank on the leaderboard as of this writing:
- The latest version of GPT-4o, which now underpins the top-ranked ChatGPT, costs $2.50/$10 per million input/output tokens. That’s substantial discount from the previous $5/$15 per million input/output tokens. And the price is half as much for batch processing of up to 50,000 requests in a single file with a 24-hour turnaround.
- The recently released GPT-4o mini, which ranks third on the leaderboard, costs much less at $0.15/$0.075 per million tokens input/output, with the same 50 percent discount for batch processing.
- Llama 3.1 405B, which was released in July and ranks fifth, is available for $2.70/$2.70 million input/output tokens from DeepInfra. That’s around 66 percent less than Azure charges.
- Gemini 1.5 Flash, which ranks 18th, costs $0.15/$0.60 per million input/output tokens after the new price cut. There’s a 50 percent discount for inputs and outputs smaller than 128,000 tokens (or submitted in batch mode). There’s also a generous free tier.
- DeepSeek v2, in 19th place, costs $0.14/$0.28 per million tokens input/output. That’s 46 percent less than when the model was released in late July.
Behind the news: Less than six months ago, cutting-edge large language models like GPT-4, Claude 2, Gemini 1.0, Llama 2, and Mistral Large were less capable and more expensive than their current versions. For instance, GPT-4 costs $30/$60 per million tokens input/output. Since then, models have notched higher benchmark performances even prices have fallen. The latest models are also faster, have larger context windows, support a wider range of input types, and do better at complex tasks such as agentic workflows.
Why it matters: Competition is fierce to provide the most effective and efficient large language models, offering an extraordinary range of price and performance to developers. Makers of foundation models that can’t match the best large models in performance or the best small models in cost are in a tight corner.
We’re thinking: What an amazing time to be developing AI applications! You can choose among models that are open or closed, small or large, faster or more powerful in virtually any combination. Everyone is competing for your business!
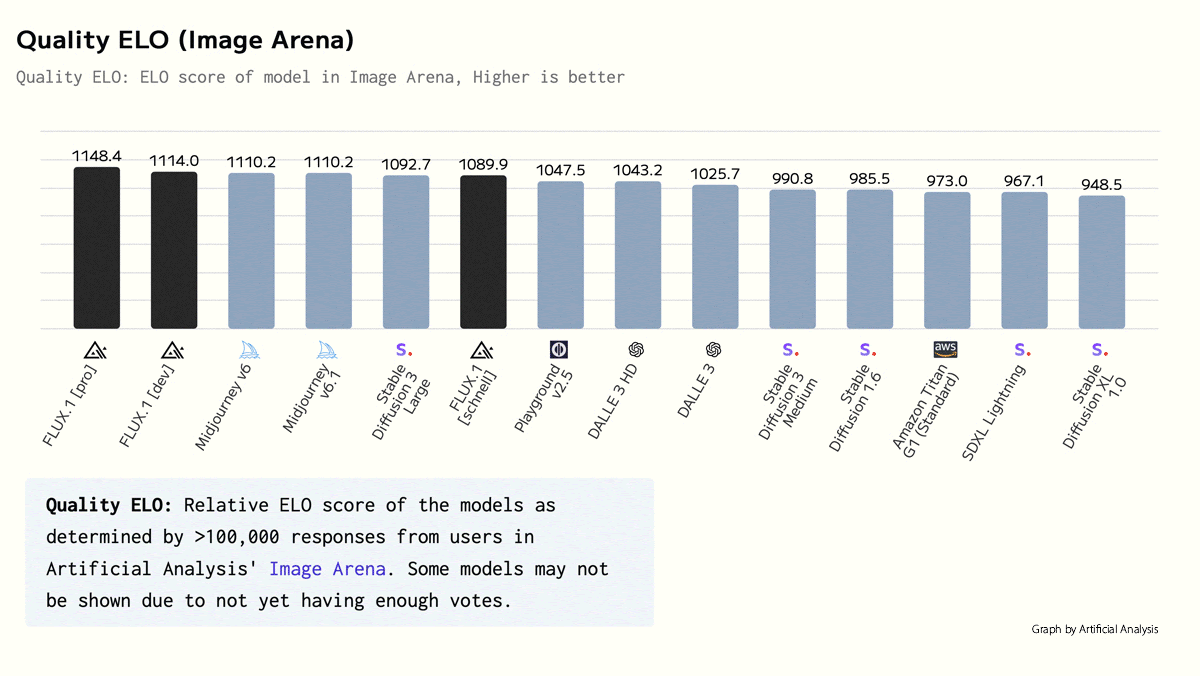
Out of the Black Forest
A new company with deep roots in generative AI made an eye-catching debut.
What’s new: Black Forest Labs, home to alumni of Stability AI, released the Flux.1 family of text-to-image models under a variety of licenses including open options. The largest of them outperformed Stable Diffusion 3 Ultra, Midourney v6.0, and DALL·E 3 HD in the company’s internal qualitative tests.
How it works: The Flux.1 models are based on diffusion transformers that were trained using flow matching, a form of diffusion. Like other latent diffusion models, given text and a noisy image embedding, they learn to remove the noise. At inference, given text and an embedding of pure noise, they remove the noise in successive steps and render an image using a decoder that was trained for the purpose.
- Flux.1 pro, whose parameter count is undisclosed, is a proprietary model available via API. It costs roughly $0.055 per image, which falls between DALL·E 3 and Stable Diffusion 3 Medium, according to Artificial Analysis. You can try a demo here.
- Flux.1 [dev] is a 12 billion-parameter distillation of Flux.1 pro. Its weights are licensed for noncommercial use and available here. A demo is available here.
- Flux.1 schnell, also 12 billion parameters, is built for speed. It’s fully open under the Apache 2.0 license. You can download weights and code here and try a demo here.
Results: Black Forest Labs evaluated the models internally in qualitative tests. Given images produced by one of the Flux.1 family and a competitor, roughly 800 people judged which they preferred for various qualities. The two larger versions achieved high scores.
- Visual quality: Flux.1 pro and Flux.1 [dev] ranked first and second (1060 Elo and 1044 Elo respectively). Stable Diffusion 3 Ultra (1031 Elo) came in third.
- Prompt following: Flux.1 pro and Flux.1 [dev] took the top two spots (1048 Elo and 1035 Elo respectively). Midjourney v6.0 (1026 Elo) placed third.
- Rendering typography: Ideogram (1080 Elo) took the top honor. Flux.1 pro and Flux.1 dev came in second and third (1068 Elo and 1038 Elo respectively).
- As of this writing, Flux.1 [pro] and Flux.1 [dev] rank first and second on the Artificial Analysis Text to Image Arena Leaderboard. Flux.1 schnell ranks fifth behind Midjourney v6.1 and Stable Diffusion 3 Large.
Behind the news: The Black Forest Labs staff includes former core members of Stability AI, which lost many top employees in April. Black Forest CEO Robin Rombach co-authored the papers that introduced VQGAN, latent diffusion, adversarial diffusion distillation, Stable Diffusion XL, and Stable Video Diffusion.
Why it matters: Text-to-image models generally occupy three tiers: large commercial models like Midjourney v6, OpenAI DALL·E 3, and Adobe Firefly; offerings that are open-source to varying degrees like Stability AI’s Stable Diffusion 3 Medium; and smaller models that can run locally like Stable Diffusion’s Stable Diffusion XL Lightning. The Flux.1 suite checks all the boxes with high marks in head-to-head comparisons.
We’re thinking: In late 2022, Stability AI’s release of the open Stable Diffusion unleashed a wave of innovation. We see a similar wave building on the open versions of Flux.1.
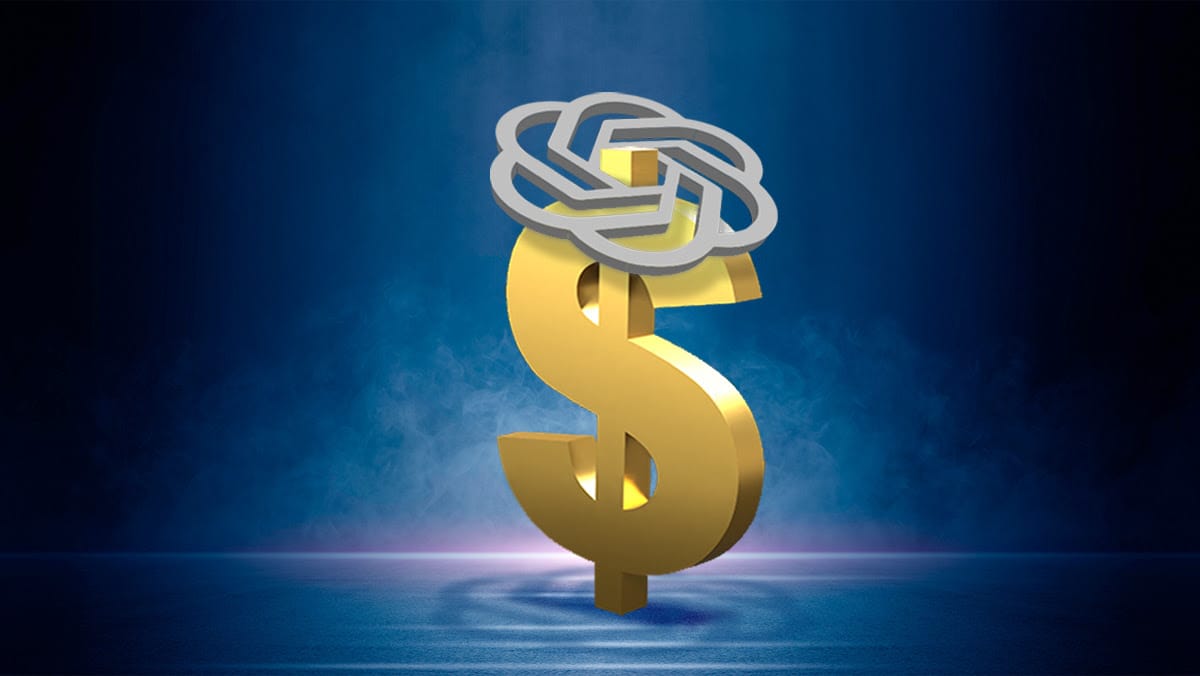
AI Leadership Makes for a Difficult Balance Sheet
OpenAI may be spending roughly twice as much money as it’s bringing in, a sign of the financial pressures of blazing the trail in commercial applications of AI.
What’s new: OpenAI’s operating expenses could amount to $8.5 billion in 2024, according to an estimate by The Information based on anonymous sources. Meanwhile, its annual revenue is shaping up to be around $3.5 billion to $4.5 billion, putting it on course to lose between $4 billion and $5 billion this year.
Revenue versus expenses: The report combined previous reporting with new information from people “with direct knowledge” of OpenAI’s finances and its relationship with Microsoft, which provides computing power for GPT-4o, ChatGPT, and other OpenAI products.
- Inference cost: This year, OpenAI is likely to spend around $4 billion on processing power supplied by Microsoft, according to a person who is familiar with the compute cluster allocated to OpenAI’s inference workloads. Microsoft charges OpenAI around $10.30 per hour per eight-GPU server, compared to its public pricing between $13.64 (on a three-year plan) and $27.20 (pay as you go) per hour per server.
- Training cost: OpenAI expects to spend $3 billion this year on training models and data, according to a person who has knowledge of the costs.
- Personnel cost: The Information estimates that OpenAI has 1,500 employees. It “guesstimates” the cost at $1.5 billion including equity compensation, based on an OpenAI source and open job listings.
- Revenue: OpenAI’s annualized monthly revenue was $3.4 billion in June. This includes sales of ChatGPT, which are likely to amount to $2 billion this year, and API calls, which accounted for annualized monthly revenue of $1 billion in March.
Why it matters: ChatGPT famously grew at an extraordinary pace in 2023 when the number of visits ballooned to 100 million within two months of the service’s launch. OpenAI’s internal sales team turned that enthusiasm into fast-growing revenue, reportedly outpacing even Microsoft’s sales of OpenAI services. Yet that growth rests on top-performance AI models, which are expensive to develop, train, and run.
We’re thinking: OpenAI is a costly undertaking: OpenAI CEO Sam Altman said it would be “the most capital-intensive startup in Silicon Valley history.” But generative AI is evolving quickly. With OpenAI’s revenue rising, its models becoming more cost-effective (witness GPT-4o mini), and the cost of inference falling, we wouldn’t bet against it.
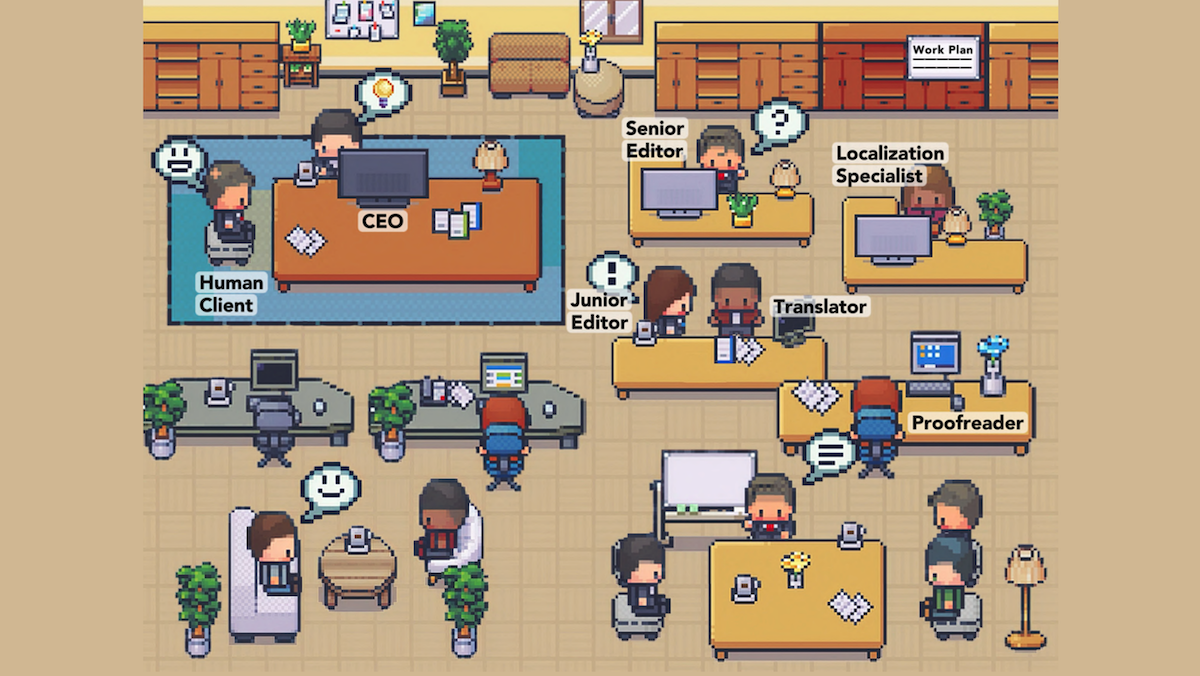
Machine Translation Goes Agentic
Literary works are challenging to translate. Their relative length, cultural nuances, idiomatic expressions, and expression of an author’s individual style call for skills beyond swapping words in one language for semantically equivalent words in another. Researchers built a machine translation system to address these issues.
What’s new: Minghao Wu and colleagues at Monash University, University of Macau, and Tencent AI Lab proposed TransAgents, which uses a multi-agent workflow to translate novels from Chinese to English. You can try a demo here.
Key insight: Prompting a large language model (LLM) to translate literature often results in subpar quality. Employing multiple LLMs to mimic human roles involved in translation breaks down this complex problem into more tractable parts. For example, separate LLMs (or instances of a single LLM) can act as agents that take on roles such as translator and localization specialist, and they can check and revise each other’s work. An agentic workflow raises unsolved problems such as how to evaluate individual agents’ performance and how to measure translation quality. This work offers a preliminary exploration.
How it works: TransAgents prompted pretrained LLMs to act like a translation company working on a dataset of novels. The set included 20 Chinese novels, each containing 20 chapters, accompanied by human translations into English.
- GPT-4 Turbo generated text descriptions of 30 workers. Each description specified attributes such as role, areas of specialty, education, years of experience, nationality, gender, and pay scale. The authors prompted 30 instances of GPT-4 Turbo to take on one of these personas. Two additional instances acted as the company’s CEO and personnel manager (or “ghost agent” in the authors’ parlance).
- Given a project, the system assembled a team. First it prompted the CEO to select a senior editor, taking into account the languages and worker profiles. The personnel manager evaluated the CEO’s choices and, if it determined they were suboptimal, prompted the CEO to reconsider. Then the system prompted the CEO and senior editor to select the rest of the team, talking back and forth until they agreed on a junior editor, translator, localization specialist, and proofreader.
- Next the system generated a guide document to be included in every prompt going forward. The junior editor generated and the senior editor refined a summary of each chapter and a glossary of important terms and their translations in the target language. Given the chapter summaries, the senior editor synthesized a plot summary. In addition, the senior editor generated guidelines for tone, style, and target audience using a randomly chosen chapter as reference.
- The team members collaborated to translate the novel chapter by chapter. The translator proposed an initial translation. The junior editor reviewed it for accuracy and adherence to the guidelines. The senior editor evaluated the work so far and revised it accordingly. The localization specialist adapted the text to fit the audience’s cultural context. The proofreader checked for language errors. Then the junior and senior editors critiqued the work of the localization specialist and proofreader and revised the draft accordingly.
- Finally, the senior editor reviewed the work, assessing the quality of each chapter and ensuring smooth transitions between chapters.
Results: Professional translators compared TransAgents’ output with that of human translators and GPT-4 Turbo in a blind test. One said TransAgents “shows the greatest depth and sophistication,” while another praised its “sophisticated wording and personal flair” that “effectively conveys the original text’s mood and meaning.”
- Human judges who read short translated passages without referring to the original texts, preferred TransAgents’ output, on average, to that of human translators and GPT-4 Turbo, though more for fantasy romance novels (which they preferred 77.8 percent of the time) than science fiction (which they preferred 39.1 percent of the time).
- GPT-4 Turbo, which did refer to the original texts while comparing TransAgents’ translations with the work of human translators and its own translations, also preferred TransAgents on average.
- TransAgents’ outputs were not word-by-word translations of the inputs but less-precise interpretations. Accordingly, it fared poorly on d-BLEU, a traditional measure that compares a translation to a reference text (higher is better) by comparing sequences of words. TransAgents achieved a d-BLEU score of 25, well below GPT-4 Turbo's 47.8 and Google Translate's 47.3.
Why it matters: While machine translation of ordinary text and conversations has made great strides in the era of LLMs, literary translation remains a frontier. An agentic workflow that breaks down the task into subtasks and delegates them to separate LLM instances makes the task more manageable and appears to produce results that appeal to human judges (and an LLM as well). That said, this is preliminary work that suggests a need for new ways to measure the quality of literary translations.
We’re thinking: Agentic workflows raise pressing research questions: What is the best way to divide a task for different agents to tackle? How much does the specific prompt at each stage affect the final output? Good answers to questions like this will lead to powerful applications.
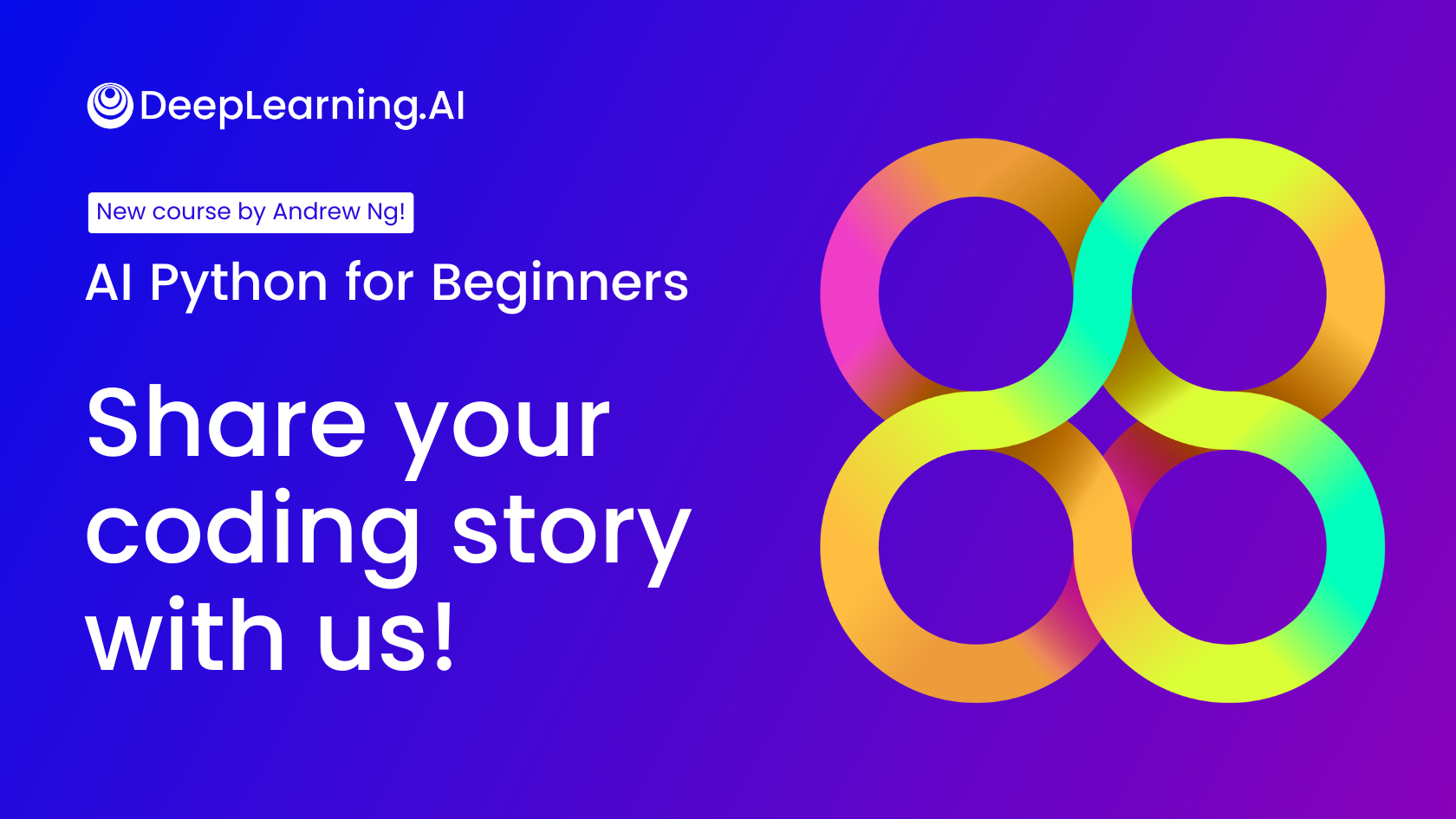
Are you an experienced developer? Share your coding story and inspire new learners! We’re celebrating the launch of “AI Python for Beginners,” taught by Andrew Ng, and we’d like to feature your story to inspire coders who are just starting out. Submit your story here!