Dear friends,
Last week I spoke at Coursera Connect, the company’s annual conference in Las Vegas, where a major topic was AI and education. There has been a lot of hype about generative AI’s ability to transform industries overnight. Certainly many industries — including education — will be transformed. But we’re about 15 years into the deep learning revolution, and we’re not yet done identifying and building useful deep learning applications. Despite the exciting progress to date with generative AI, I expect that a decade from now we will still be far from finished identifying and building generative AI applications for education and numerous other sectors.
This was the first time since 2019 that Coursera’s conference was held in person. It was great to see so many people dedicated to the educational mission coming together to discuss innovations, including generative AI innovations, that serve learners.
Coursera’s CEO Jeff Maggioncalda and the company’s executive team demonstrated multiple generative AI products, such as:
- Coursera Coach, a chatbot that understands the context of a learner's journey and answers their questions (without giving away exact answers to quiz questions!)
- Course Builder, which businesses are using to customize long courses or specializations quickly, for example, by selecting the parts most relevant to their business
- Coach for Interactive Instruction, which lets learners have a Socratic dialog and learn or practice new concepts in conversation
Because AI is a general-purpose technology, there are many opportunities to apply it to different tasks in education. I was thrilled at the volume of experimentation happening across Coursera, DeepLearning.AI, and the broader ecosystem of partners and customers. I was also proud to present awards to many partners and customers who are doing great work to serve learners.
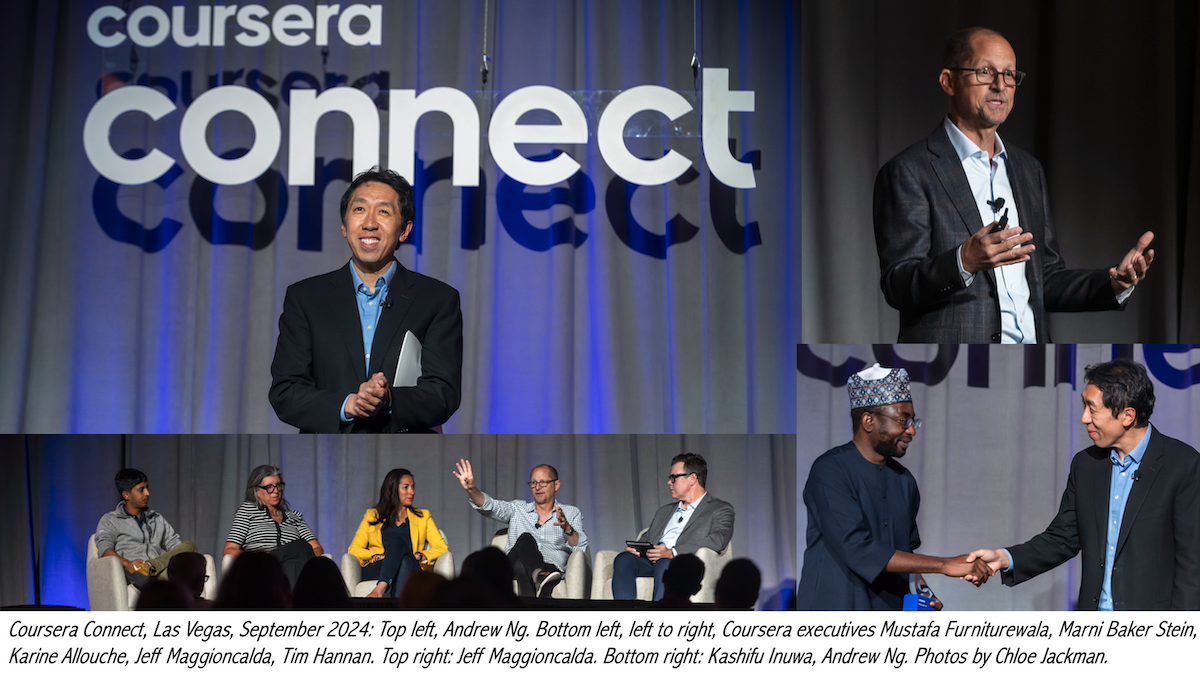
I was particularly gratified by the number of people coming together in service of the education mission. Even before the recent rise of AI, education was already urgently in need of improvement. With AI transforming jobs, the need has become even more acute. My heart was warmed by the conversations I had with many people from universities, high schools, businesses, and the Coursera team who have a deep desire to help others through education.
Coursera held its first conference in 2013, when the online education movement was in its early days, and we all had high hopes for where it could go. Today, there are over 155 million learners on Coursera. Despite that, given society’s heightened need for education and AI’s potential to transform the field, I feel the opportunities for edtech at this moment are greater than at any moment over the past decade.
Keep learning!
Andrew
P.S. I’m excited to announce our new specialization, Generative AI for Software Development, taught by Laurence Moroney! Using chatbots to generate code is not the only way AI can help developers. This three-course series shows you how to use AI throughout the software development lifecycle – from design and architecture to coding, testing, deployment, and maintenance. Everyone who writes software can benefit from these skills. Please sign up here!
A MESSAGE FROM DEEPLEARNING.AI
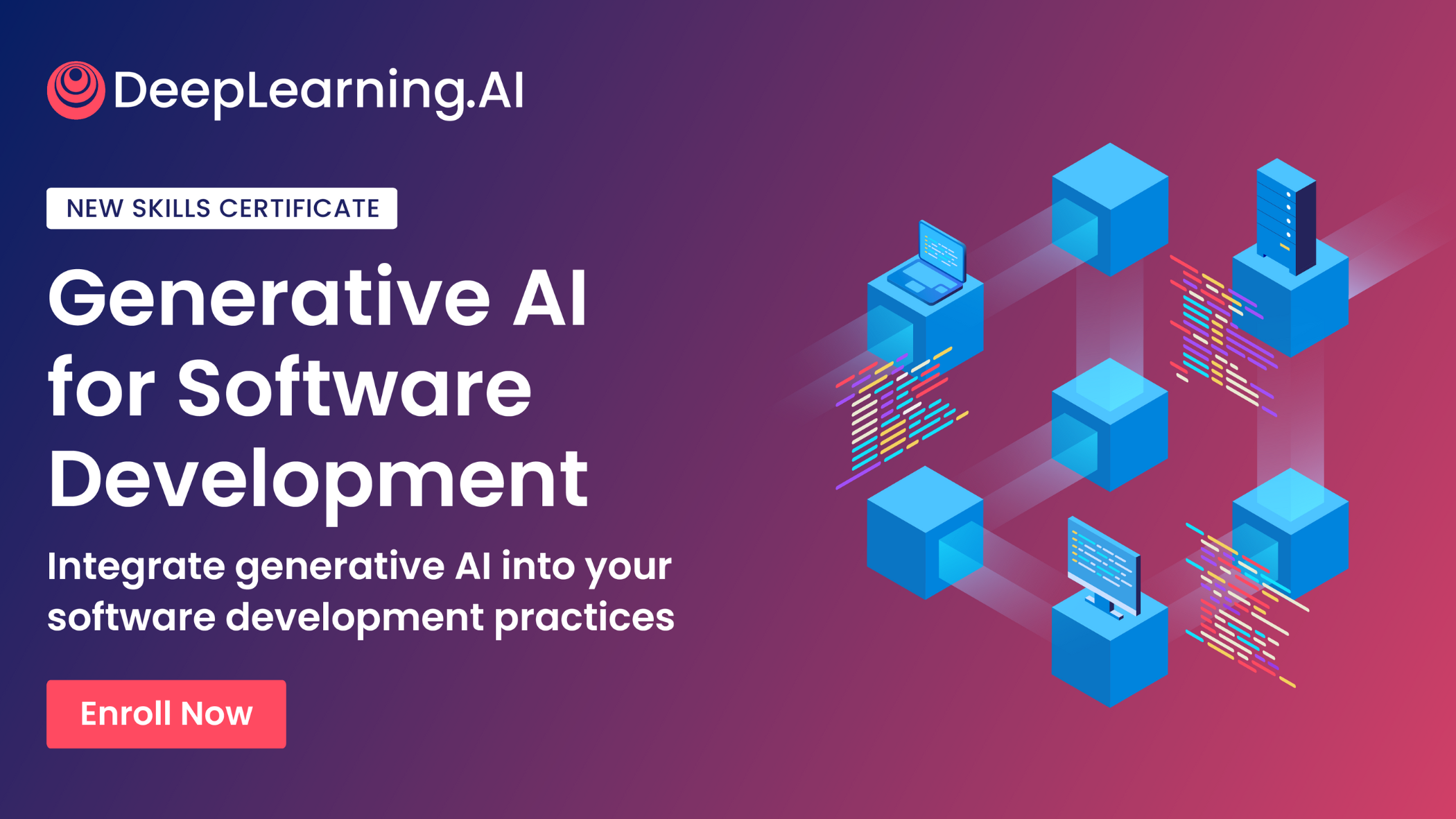
Generative AI for Software Development, our new skill certificate, gives you practical experience applying AI to coding, debugging, optimization, and documentation as it explores AI’s role across the entire development lifecycle—design, architecture, coding, testing, deployment, and maintenance. Equip yourself with the tools to enhance every step of your dev workflow. Enroll now
News
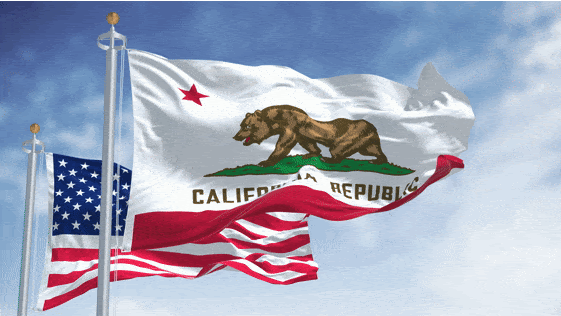
California Restricts Deepfakes
California, a jurisdiction that often influences legislators worldwide, passed a slew of new laws that regulate deepfakes.
What’s new: California Governor Gavin Newsom signed into law eight bills that aim to curb the use of generative AI in politics and entertainment.
How it works: The legislation prohibits deceptive AI-generated media in political campaigns; requires permission for using digital stand-ins for actors, musicians, and other entertainers; and criminalizes generation of sexually explicit imagery without the subject’s consent.
- One law prohibits knowingly distributing deceptive AI-generated information about candidates, elections officials, or voting processes between 120 days before and 60 days after elections. The bill defines “materially deceptive content” as images, audio, or video that were intentionally created or modified but would appear to a reasonable person to be authentic.
- Two related laws mandate disclosure when AI is used to produce political advertisements. The first requires that AI-generated campaign ads include the statement, “ad generated or substantially altered using artificial intelligence.” The other calls for large online platforms to label or remove AI-generated media related to elections.
- Two further laws protect performers by controlling “digital replicas,” defined as “computer-generated, highly realistic electronic representation[s] of an individual’s voice or likeness.” One voids contracts for the use of digital replicas if performers didn’t have legal or union representation when they made the agreements. The other prohibits commercial use of deceased performers’ digital replicas without permission of their estates.
- Two laws regulate sexually explicit synthetic content. One establishes the creation and distribution of non-consensual, AI-generated sexually explicit content as a disorderly conduct misdemeanor. The other requires social media platforms to report sexually explicit deepfakes.
- An additional law requires that AI-generated media include a disclosure of its provenance.
Behind the news: Newsom has not yet acted on Senate Bill 1047, a controversial law that would impose significant burdens on AI model developers. He has expressed that the bill could interfere with innovation, especially with respect to open source projects.
Why it matters: Laws passed in California often point the way for legislators in other U.S. states, the federal government, and consequently other countries. The new laws that regulate deepfakes in political campaigns fill a gap left by the Federal Election Commission (FEC), which has said it lacks authority to regulate the use of AI in political ads. Meanwhile, the Federal Communications Commission (FCC) proposed rules that would mandate disclosure of uses of AI in political ads but has yet to implement them.
We’re thinking: We’re glad to see California target undesirable applications rather than AI models. Regulating applications rather than general-purpose technology that has a wide variety of uses — many of which are beneficial — avoids the dangers of California SB-1047, which is still awaiting the governor’s signature or veto. That law, which seeks to restrict AI models, would endanger innovation and especially open source.
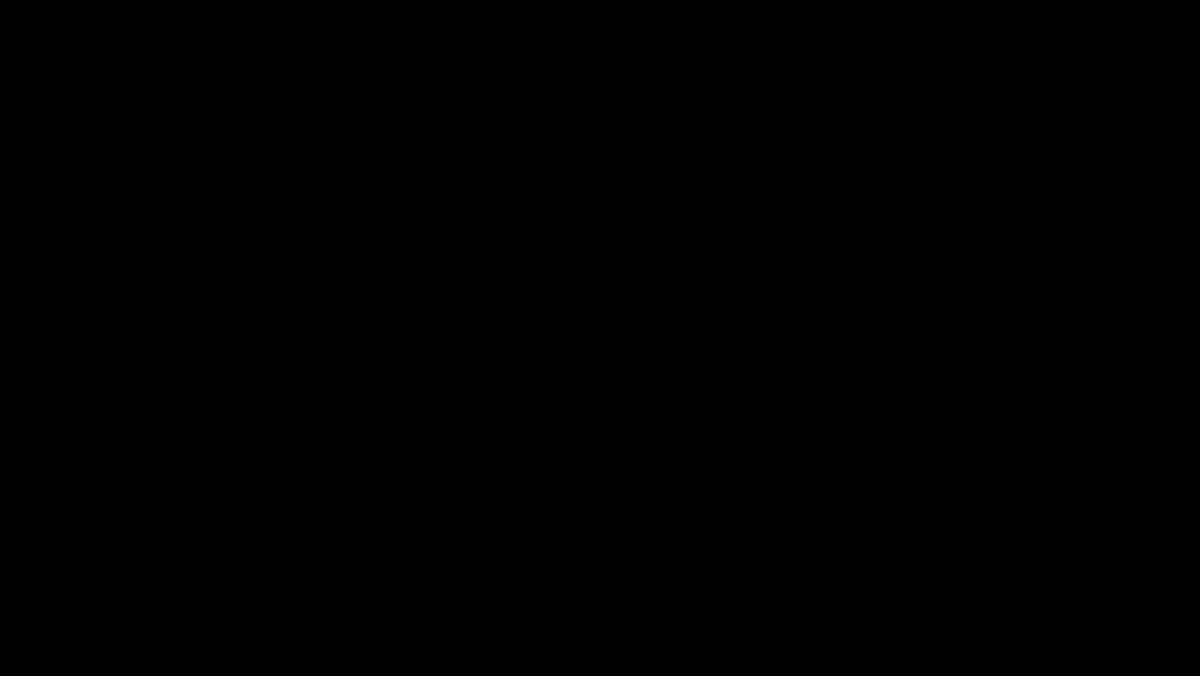
More, Better Open Source Options
The parade of ever more capable LLMs continues with Qwen 2.5.
What’s new: Alibaba released Qwen 2.5 in several sizes, the API variants Qwen Plus and Qwen Turbo, and the specialized models Qwen 2.5-Coder and Qwen 2.5-Coder-Instruct and Qwen 2.5-Math and Qwen 2.5-Math-Instruct. Many are freely available for commercial use under the Apache 2.0 license here. The 3B and 72B models are also free, but their license requires special arrangements for commercial use.
How it works: The Qwen 2.5 family ranges from 500 million parameters to 72 billion parameters.
- Qwen 2.5 models were pretrained on 18 trillion tokens. Sizes up to 3 billion parameters can process up to 32,000 input tokens; the larger models can process up to 128,000 input tokens. All versions can have an output length of 8,000 tokens.
- Qwen 2.5-Coder was further pretrained on 5.5 trillion tokens of code. It can process up to 128,000 input tokens and generate up to 2,000 output tokens. It comes in 1.5B and 7B versions.
- Qwen 2.5-Math further pretrained on 1 trillion tokens of math problems, including Chinese math problems scraped from the web and generated by the earlier Qwen 2-Math-72B-Instruct. Qwen 2.5-Math can process 4,000 input tokens and generate up to 2,000 output tokens. It comes in 1.5B, 7B, and 72B versions. In addition to solving math problems, Qwen 2.5-Math can generate code to help solve a given math problem.
Results: Compared to other models with open weights, Qwen 2.5-72B-Instruct beats LLama 3.1 405B Instruct and Mistral Large 2 Instruct (123 billion parameters) on seven of 14 benchmarks including LiveCodeBench, MATH (solving math word problems), and MMLU (answering questions on a variety of topics). Compared to other models that respond to API calls, Qwen-Plus beats LLama 3.1 405B, Claude 3.5 Sonnet, and GPT-4o on MATH, LiveCodeBench, and ArenaHard. Smaller versions also deliver outstanding performance. For instance, Qwen 2.5-14B-Instruct outperforms Gemma 2 27B Instruct and GPT-4o mini on seven benchmarks.
Behind the news: Qwen 2.5 extends a parade of ever more capable LLMs that include Claude 3.5 Sonnet, GPT-4o, and LLama 3.1 as well as the earlier Qwen 2 family.
Why it matters: The new models raise the bar for open weights models of similar sizes. They also rival some proprietary models, offering options to users who seek to balance performance and cost.
We’re thinking: Some companies encourage developers to use their paid APIs by locking their LLMs behind non-commercial licenses or blocking commercial applications beyond a certain threshold of revenue. We applaud Qwen’s approach, which keeps most models in the family open.
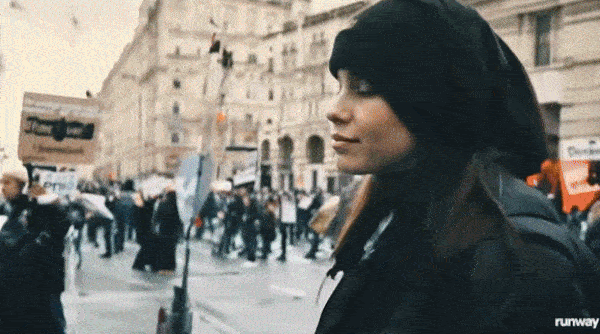
Hollywood Embraces Video Generation
The AI startup Runway is helping to retool Lionsgate, the producer of blockbuster movie franchises like The Hunger Games and John Wick, for the era of generated video.
What’s new: Runway will build a custom video generator to help Lionsgate streamline its production processes. It also launched an API for its Gen-3 Alpha Turbo model.
Runway + Lionsgate: Runway will fine-tune its proprietary models on Lionsgate productions to enable the filmmaker to generate new imagery based on its previous work. The companies didn’t disclose financial terms of the arrangement.
- Lionsgate plans to use the custom model for pre-production tasks like visualization and storyboarding, and for post-production processes like editing and special effects.
- The custom model could save Lionsgate “millions and millions of dollars,” a Lionsgate executive told The Wall Street Journal.
- Other studios, too, are looking into building video generation models that are fine-tuned on their own productions, Variety reported. Runway is in talks with some of them, the startup’s CEO Cristóbal Valenzuela told Axios.
Gen-3 API: Concurrently with announcing the Lionsgate deal, Runway unveiled an API that drives its Gen-3 Alpha and Gen-3 Alpha Turbo models as well as updates to Gen-3 Alpha.
- The company charges around $0.60 to $1.20, depending on the service tier, to generate outputs up to 5 seconds long and twice that for up to 10 seconds long.
- Third-party user interfaces that connect to the API must include a “Powered by Runway” banner that links to Runway’s website.
- Gen-3 Alpha now allows users to transform existing videos into new styles using text prompts and steer its output using video input in addition to a prompt. The model’s output will follow the input video’s shapes and motions.
Why it matters: Although the plan is to use Runway’s technology for pre- and post-production, this deal puts state-of-the-art video generation at the heart of Lionsgate’s operations and encourages professional cinematographers, editors, special effects artists, and other cinematic specialists to see what they can do with it. For Lionsgate, it’s a bid to stay ahead of competitors. For AI, it could be a major move into the Hollywood spotlight.
We’re thinking: While upstart competitors are using pretrained models, Lionsgate will be using a model that has internalized its own style and capabilities.
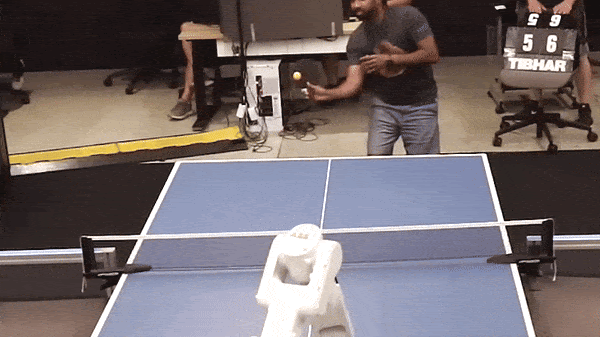
Robot Server
A robot that plays table tennis beats human beginners and entertains experts.
What’s new: David B. D’Ambrosio, Saminda Abeyruwan, Laura Graesser, Atil Iscen, Pannag R. Sanketi and colleagues at Google showed off a robot arm that challenges human players at table tennis. You can see it in action here.
Key insight: A table tennis match can be broken into individual volleys that start when an opponent hits the ball and end when the robot returns the ball to the opponent’s side of the table or the ball goes out of play. This simple scheme enables a robotic control system to learn how to return a ball without attending to strategy.
The robot: The authors mounted a robotic arm atop two linear gantries that enabled the arm to move to the left and right, and forward and backward. Two cameras captured images of the ball and fed them to a perception system that estimated ball positions. A 20-camera motion-capture system tracked the position of the opponent’s paddle.
How it works: Instead of training an end-to-end system or using a robotics foundation model, the authors broke down the gameplay into subtasks, delegated them to separate modules, and orchestrated them to work together. The robot was controlled by a high-level controller: a custom algorithm including a convolutional neural network (CNN) that classified whether to return the ball using a forehand or backhand stroke and a vanilla neural network that classified spin. The high-level controller selected among 17 low-level controllers (all CNNs). Each low-level controller executed a different skill, enabling the system to return serves or rallies, adjust for ball spin, target different spots on the table, and so on.
- The authors collected a dataset of ball positions from human-to-human play. Using the perception system, they derived the ball’s initial positions, velocities, and angular velocities. After training the system the first time, they collected similar data for human-robot play and trained their system further using those examples.
- Training took place in a simulation (except the high-level controller’s vanilla neural network, which learned to classify spin via supervision).The high-level controller’s CNN learned to choose forehand or backhand to maximize the rate at which the robot successfully returned the ball. The low-level controllers learned using blackbox gradient sensing, an evolutionary algorithm, based on several rewards, such as rewarding the controller if it successfully returned the ball and punishing it if the robot collided with itself or the table.
- Each time the opponent hit the ball, the high-level controller decided which low-level controller to use. The decision was based on factors such as whether the ball had topspin or underspin and estimated statistics such as return rate, opponent’s paddle velocity, and estimated position where the ball would land on the opponent’s side.
- Given the last 0.14 seconds of the ball’s position and velocity, as well as the robot’s joint positions and its position on the gantries, the selected low-level controller determined how fast to move the robot to return the ball.
Results: The robot played 29 three-game matches against 29 players of varying skill (beginner, intermediate, advanced, and advanced+ as rated by a professional coach).
- It won all 7 (100 percent) of its matches against beginners, 6 (55 percent) of its matches against intermediate players, and zero matches against advanced or advanced+ players.
- On a point-by-point basis, it won 72 percent of points against beginners, 50 percent against intermediate players, and 34 percent of points against advanced and advanced+ players.
- When asked if they would like to play against the robot again on a scale of 1 (definitely not) to 5 (definitely yes), the average response was 4.87.
Why it matters: Roboticists have been programming robot arms to play table tennis for at least a decade. Earlier projects enabled robots to perform various aspects of the game, like aiming at a specific target or smashing, but none tackled complete gameplay against competitive human opponents. Breaking the problem into two parts — a library of individual skills (low-level controllers) and an algorithm that chooses which to use — simplifies the task. Weaknesses in the robot’s performance (for example, difficulty returning underspin) can be addressed by adding a skill that compensates.
We’re thinking: Even expert players had enough fun playing against this robot to want to play more. That’s a successful gaming system!