Dear friends,
We won! California’s anti-innovation bill SB 1047 was vetoed by Governor Newsom over the weekend. Open source came closer to taking a major blow than many people realize, and I’m grateful to the experts, engineers, and activists who worked hard to combat this bill.
The fight to protect open source is not yet over, and we have to continue our work to make sure regulations are based on science, not science-fiction.
As I wrote previously, SB 1047 makes a fundamental mistake of trying to regulate technology rather than applications. It was also a very confusing law that would have been hard to comply with. That would have driven up costs without improving safety.
While I’m glad that SB 1047 has been defeated, I wish it had never made it to the governor’s desk. It would not have made AI safer. In fact, many of its opponents were champions of responsible AI and making AI safe long before the rise of generative AI. Sadly, as the Santa Fe Institute’s Melanie Mitchell pointed out, the term “AI safety” has been co-opted to refer to a broad set of speculative risks that have little basis in science — as demonstrated by the security theater SB 1047 would have required — that don’t actually make anything safer. This leaves room for lobbying that can enrich a small number of people while making everyone else worse off.
As Newsom wrote to explain his decision, SB 1047 is “not informed by an empirical trajectory analysis of AI systems and capabilities.” In contrast, the United States federal government’s work is “informed by evidence-based approaches, to guard against demonstrable risks to public safety.” As the governor says, evidence-based regulation is important!
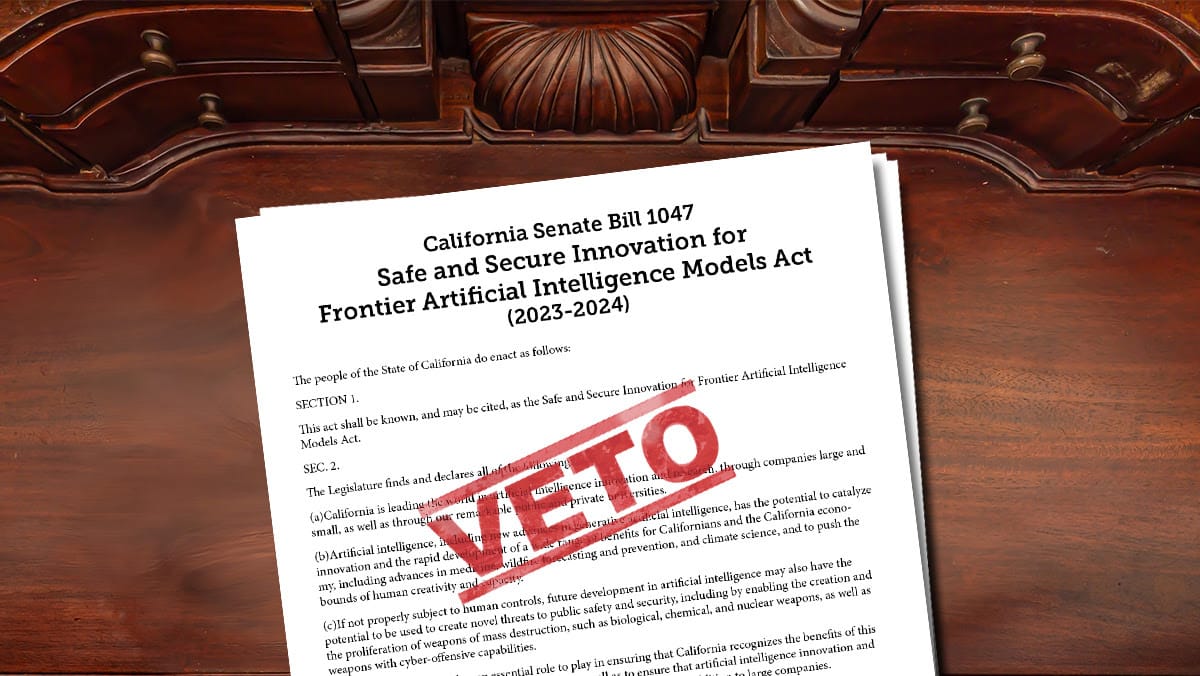
Many people in the AI community were instrumental in defeating the bill. We're lucky to have Martin Casado, who organized significant community efforts; Clément Delangue, who championed openness; Yann LeCun, a powerful advocate for open research and open source; Chris Lengerich, who published deep legal analysis of the bill; Fei-Fei Li and Stanford's HAI, who connected with politicians; and Garry Tan, who organized the startup accelerator Y Combinator against the bill. Legendary investors Marc Andreessen and Roelof Botha were also influential. Plus far too many others to name here. I’m also delighted that brilliant artists like MC Hammer support the veto!
Looking ahead, far more work remains to be done to realize AI’s benefits. Just this week, OpenAI released an exciting new voice API that opens numerous possibilities for beneficial applications! In addition, we should continue to mitigate current and potential harms. UC Berkeley computer scientist Dawn Song and collaborators recently published a roadmap to that end. This includes investing more to enable researchers to study AI risks and increasing transparency of AI models (for which open source and red teaming will be a big help).
Unfortunately, some segments of society still have incentives to pass bad laws like SB 1047 and use science fiction narratives of dangerous AI superintelligence to advance their agendas. The more light we can shine on what AI really is and isn’t, the harder it will be for legislators to pass laws based on science fiction rather than science.
Keep learning!
Andrew
A MESSAGE FROM DEEPLEARNING.AI
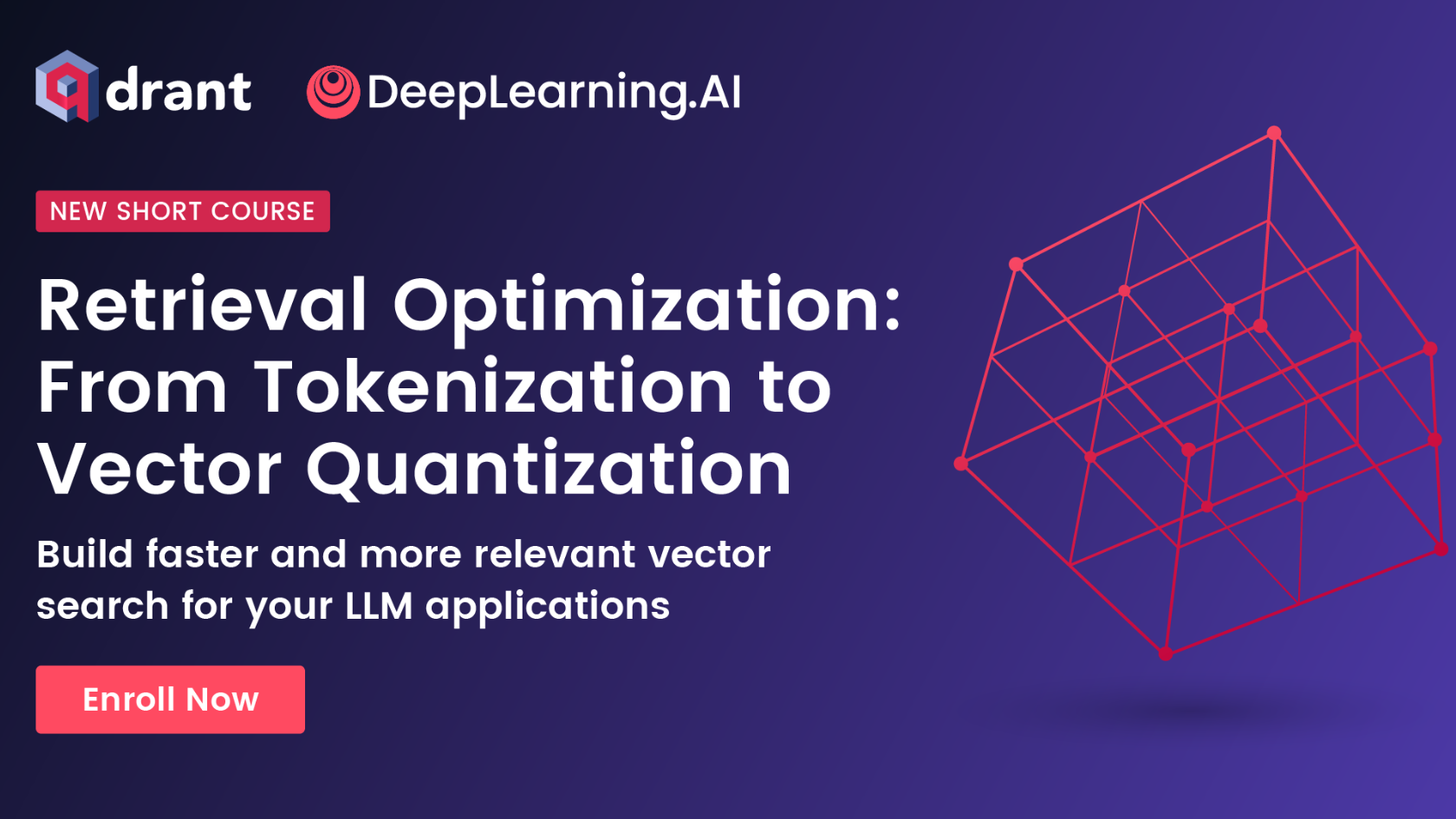
In this short course, you’ll learn how tokenization affects vector search and how to optimize search in LLM applications that use RAG. You’ll explore Byte-Pair Encoding, WordPiece, and Unigram; fine-tune HNSW parameters; and use vector quantization to improve performance. Sign up for free
News
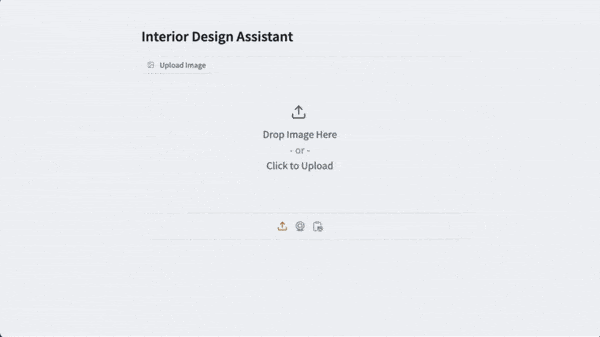
Llama Herd Expands
Meta extended its Llama family of models into two new categories: vision-language and sizes that are small enough to fit in edge devices.
What’s new: Meta introduced Llama 3.2, including two larger vision-language models and two smaller text-only models as well as developer tools for building agentic applications based on the new models. Weights and code are free to developers who have less than 700 million monthly active users. Multiple providers offer cloud access.
How it works: Llama 3.2 90B and 11B accept images as well as text and generate text output (image processing is not available in the European Union). Llama 3.2 1B and 3B accept and generate text. All four models can process 131,072 tokens of input context and generate 2,048 tokens of output.
- Llama 3.2 90B and 11B are based on Llama 3.1. The team froze a Llama 3.1 model and added an image encoder and cross-attention layers. They trained these new elements, given matching images and text, to produce image embeddings that matched the resulting text embeddings. To enhance the model’s ability to interpret images, the team fine-tuned the new elements via supervised learning and DPO. Given an image, they learned to generate questions and answers that ranked highly according to a reward model. Thus Llama 3.2 responds to text input identically to Llama 3.1, making it a viable drop-in replacement.
- Likewise, Llama 3.2 3B and 1B are based on Llama 3.1 8B. The team members pruned each model using an unspecified method. Then they used Llama 3.1 8B and 70B as teacher models, training the Llama 3.2 students to mimic their output. Finally, they fine-tuned the models to follow instructions, summarize text, use tools, and perform other tasks using synthetic data generated by Llama 3.1 405B.
- On popular benchmarks, Llama 3.2 90B and 11B perform roughly comparably to Claude 3 Haiku and GPT-4o-mini, the smaller vision-language models from Anthropic and OpenAI respectively. For example, Llama 3.2 90B beats both closed models on MMMU and MMMU-Pro, answering visual questions about graphs, charts, diagrams, and other images. They also beat Claude 3 Haiku and GPT-4o-mini on GPQA, which tests graduate-level reasoning in various academic subjects. However, on these benchmarks, larger Llama 3.2 models are well behind larger, proprietary models like o1 and Sonnet 3.5 as well as the similarly sized, open Qwen-2VL.
- Llama 3.2’s vision-language capabilities now drive the company’s Meta AI chatbot. For example, users can upload a photo of a flower and ask the chatbot to identify it or post a picture of food and request a recipe. Meta AI also uses Llama 3.2’s image understanding to edit images given text instructions.
New tools for developers: Meta announced Llama Stack, a series of APIs for customizing Llama models and building Llama-based agentic applications. Among other services, Llama Stack has APIs for tool use, memory, post-training, and evaluation. Llama Guard, a model designed to evaluate content for sexual themes, violence, criminal planning, and other issues, now flags problematic images as well as text. Llama Guard 3 11B Vision comes with Llama.com’s distributions of Llama 3.2 90B and 11B, while Llama Guard 3 1B comes with Llama 3.2 3B and 1B.
Why it matters: Meta’s open models are widely used by everyone from hobbyists to major industry players. Llama 3.2 extends the line in valuable ways. The growing competition between Llama and Qwen shows that smaller, open models can offer multimodal capabilities that are beginning to rival their larger, proprietary counterparts.
We’re thinking: By offering tools to build agentic workflows, Llama Stack takes Llama 3.2 well beyond the models themselves. Our new short course “Introducing Multimodal Llama 3.2” shows you how to put these models to use.
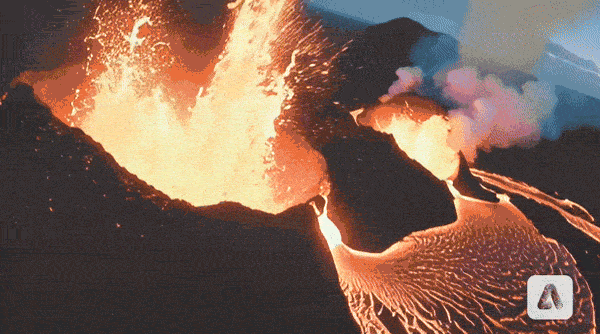
Generative Video in the Editing Suite
Adobe is putting a video generator directly into its popular video editing application.
What’s new: Adobe announced its Firefly Video Model, which will be available as a web service and integrated into the company’s Premiere Pro software later this year. The model takes around two minutes to generate video clips up to five seconds long from a text prompt or still image, and it can modify or extend existing videos. Prospective users can join a waitlist for access.
How it works: Adobe has yet to publish details about the model’s size, architecture, or training. It touts uses such as generating B-roll footage, creating scenes from individual frames, adding text and effects, animation, and video-to-video generation like extending existing clips by up to two seconds.
- The company licensed the model’s training data specifically for that purpose, so the model’s output shouldn’t run afoul of copyright claims. This practice stands in stark contrast to video generators that were trained on data scraped from the web.
- Adobe plans to integrate the model with Premiere Pro, enhancing its traditional video editing environment with generative capabilities. For instance, among the demo clips, one shows a real-world shot of a child looking into a magnifying glass immediately followed by a generated shot of the child’s view.
Behind the news: Adobe’s move into video generation builds on its Firefly image generator and reflects its broader strategy to integrate generative AI with creative tools. In April, Adobe announced that it would integrate multiple video generators with Premiere, including models from partners like OpenAI and Runway. Runway itself recently extended its own offering with video-to-video generation and an API.
Why it matters: Adobe is betting that AI-generated video will augment rather than replace professional filmmakers and editors. Putting a full-fledged generative model in a time-tested user interface for video editing promises to make video generation more useful as well as an integral part of the creative process. Moreover, Adobe’s use of licensed training data may attract videographers who are concerned about violating copyrights or supporting fellow artists.
We’re thinking: Video-to-video generation crossing from frontier capability to common feature. Firefly's (and Runway’s) ability to extend existing videos offers a glimpse.
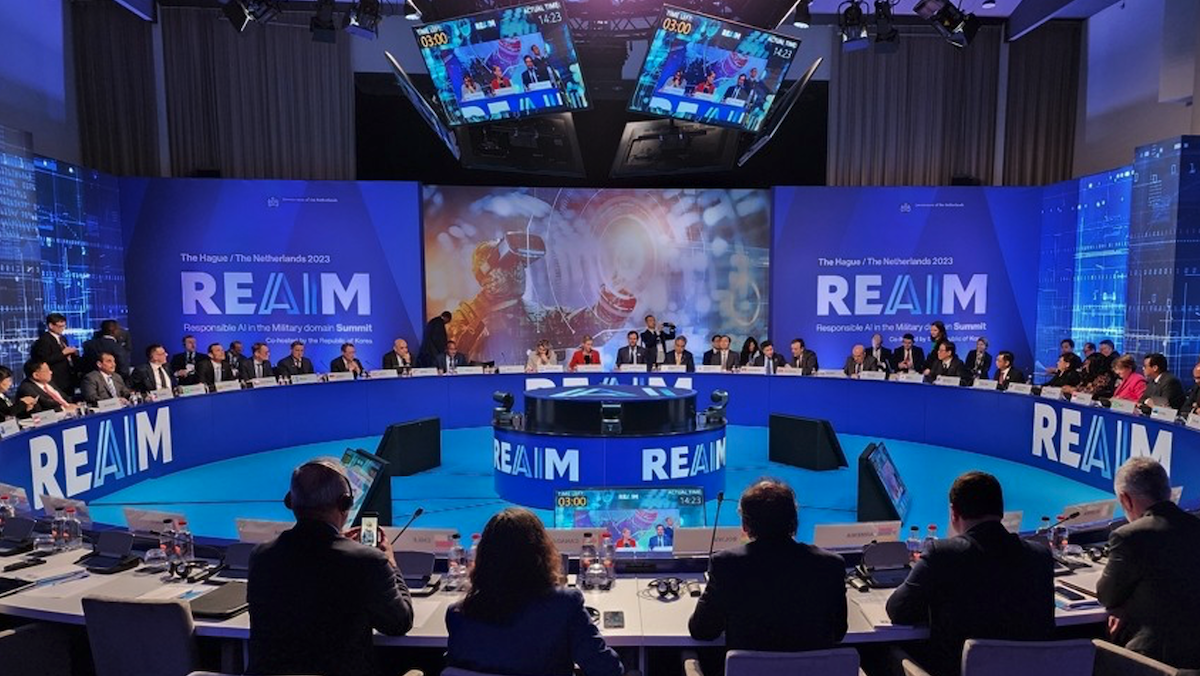
International Guidelines for Military AI
Dozens of countries endorsed a “blueprint for action” designed to guide the use of artificial intelligence in military applications.
What’s new: More than 60 countries including Australia, Japan, the United Kingdom, and the United States endorsed nonbinding guidelines for military use of AI, Reuters reported. The document, presented at the Responsible Artificial Intelligence in the Military (REAIM) summit in Seoul, South Korea, stresses the need for human control, thorough risk assessments, and safeguards against using AI to develop weapons of mass destruction. China and roughly 30 other countries did not sign.
How it works: Key agreements in the blueprint include commitments to ensure that AI doesn’t threaten peace and stability, violate human rights, evade human control, and hamper other global initiatives regarding military technology.
- The blueprint advocates for robust governance, human oversight, and accountability to prevent escalations and misuse of AI-enabled weapons. It calls for national strategies and international standards that align with laws that govern human rights. It also urges countries to share information and collaborate to manage risks both foreseeable and unforeseeable and maintain human control over uses of force.
- It leaves to individual nations the development of technical standards, enforcement mechanisms, and specific regulations for technologies like autonomous weapons systems.
- The agreement notes that AI can enhance situational awareness, precision, and efficiency in military operations, helping to reduce collateral damage and civilian fatalities. AI can also support international humanitarian law, peacekeeping, and arms control by improving monitoring and compliance. But the agreement also points out risks like design flaws, data and algorithmic biases, and potential misuse by malicious actors.
- The blueprint stresses preventing AI’s use in the development and spread of weapons of mass destruction, emphasizing human control in disarmament and nuclear decision-making. It also warns of AI increasing risks of global and regional arms races.
Behind the News: The Seoul summit followed last year’s REAIM summit in The Hague, where leaders similarly called for limits on AI military use without binding commitments. Other international agreements like the EU’s AI Act and Framework Convention on Artificial Intelligence and Human Rights, Democracy, and the Rule of Law regulate civilian AI, but exclude military applications. Meanwhile, AI-enabled targeting systems and autonomous, weaponized drones have been used in conflicts in Somalia, Ukraine, and Israel, highlighting the lack of international norms and controls.
Why it matters: The REAIM blueprint may guide international discussions on the ethical use of AI in defense, providing a foundation for further talks at forums like the United Nations. Though it’s nonbinding, it fosters collaboration and avoids restrictive mandates that could cause countries to disengage.
We’re thinking: AI has numerous military applications across not only combat but also intelligence, logistics, medicine, humanitarian assistance, and other areas. Nonetheless, it would be irresponsible to permit unfettered use of AI in military applications. Standards developed by democratic countries working together will help protect human rights.
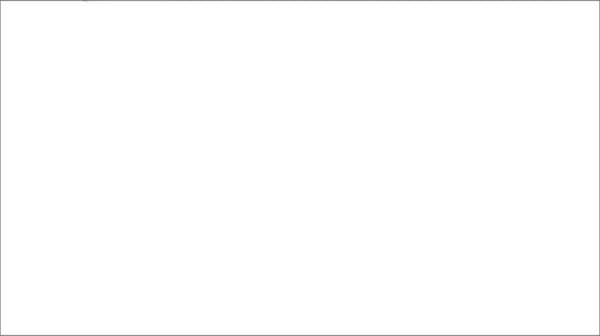
Enabling LLMs to Read Spreadsheets
Large language models can process small spreadsheets, but very large spreadsheets often exceed their limits for input length. Researchers devised a method that processes large spreadsheets so LLMs can answer questions about them.
What’s new: Yuzhang Tian, Jianbo Zhao, and colleagues at Microsoft proposed SheetCompressor, a way to represent spreadsheets that enables LLMs to identify and request the parts they need to answer specific questions.
Key insight: Most spreadsheets can be broken down into a set of tables that may be bordered by visual dividers like thick lines or empty rows and/or columns. But detecting these tables isn’t trivial, since they may contain the same kinds of markers. (See the illustration above, in which tables are denoted by red dashes.) To answer many questions, you don’t need the whole spreadsheet, only the relevant table. Moreover, given a question, an LLM can recognize the table it needs to produce an answer. However, to identify the correct table, it needs to see the whole spreadsheet, which may be too large for its input context window, and the tables, which may not be clearly separated, need to be parsed. The solution is to compress the spreadsheet, feed the compressed representation to the LLM along with the question, and ask the LLM to identify the boundaries of the table it needs to answer the question. Then, given an uncompressed version of that table, the LLM can produce an answer.
How it works: The authors built software that prepared spreadsheets by (i) parsing them into tables and (ii) compressing them while maintaining the table structure. Then they fine-tuned LLMs to detect tables in the compressed spreadsheets and prompted the fine-tuned LLMs to identify the tables relevant to a given question.
- Given a spreadsheet, the authors removed rows and columns that weren’t near likely table boundaries defined by empty cells, thick lines, changes in color, and so on.
- To compress a parsed spreadsheet, they represented each table as a JSON dictionary, using cell values as dictionary keys and cell addresses as dictionary values. (This reduces the sequence length, since duplicate cell values have the same dictionary key.) To compress it further, within each table, they detected types of values — for instance temperature, age, percentage, and so on — and merged adjacent cells that shared the same type into a single dictionary key that represented the type rather than the values. For example, merging dates that appear in the same column into a single entry: {"yyyy-mm-dd" : <cell addresses>}.
- They compressed a dataset of spreadsheets with annotated table boundaries according to this method. They used the compressed dataset to fine-tune GPT-4, Llama 3, and other LLMs to detect tables within compressed spreadsheets.
- Inference was a two-step process: (i) Prompt the LLM, given a compressed spreadsheet and a question, to output the boundaries of the table(s) most relevant to the question and (ii) prompt the LLM, given an uncompressed version of the relevant table(s), to answer the question.
Results: The authors compared the fine-tuned LLMs’ ability to detect tables in spreadsheets that were compressed using their method and in their original uncompressed form. They fed the models spreadsheets of various sizes that ranged from small (up to 4,000 tokens) to huge (more than 32,000 tokens). They gauged the models’ performance according to F1 score (higher is better).
- Small spreadsheets: Fed compressed spreadsheets, the fine-tuned Llama 3 achieved 83 percent F1 score, and the fine-tuned GPT-4 achieved 81 percent F1 score. By contrast, fed uncompressed spreadsheets, Llama 3 achieved 72 percent F1 score, and GPT-4 achieved 78 percent F1 score.
- Huge spreadsheets: Fed compressed spreadsheets, the fine-tuned Llama 3 achieved 62 percent F1 score, and the fine-tuned GPT-4 achieved 69 percent F1 score. Fed uncompressed spreadsheets, both models both achieved 0 percent F1 score.
- Answering questions: The authors also tested the fine-tuned models on their own dataset of questions about 64 spreadsheets that spanned the same range of sizes, posing questions that involved fundamental tasks like searching, comparing, and basic arithmetic. Fed compressed spreadsheets, the fine-tuned GPT-4 achieved a 74 percent accuracy on zero-shot question answering. Fed uncompressed spreadsheets, it achieved 47 percent accuracy.
Why it matters: By giving LLMs the ability to detect a spreadsheet’s functional components, this approach enables them to process a wide variety of spreadsheets regardless of their size and complexity.
We’re thinking: When considering the strengths of LLMs, we no longer have to take spreadsheets off the table.