Dear friends,
It’s high time to take geoengineering more seriously as a potential tool to mitigate climate change. 2023 was the hottest year on record, and 2024 is likely to top that. In the United States, Hurricane Helene caused over 200 deaths, and Hurricane Milton's death toll is at least two dozen. It’s well established that the hurricanes are growing stronger as global temperatures rise.
While stratospheric aerosol injection (SAI) — which sprays particles (aerosols) in the atmosphere to provide a small amount of shade from the sun — is far from a perfect solution, we should take it seriously as a possible tool for saving lives. A few months ago, my collaborators and I had released a climate emulator, Planet Parasol, that you can play with to simulate different SAI scenarios to understand its possible impact. By using AI to model its impact and thereby advance our understanding of SAI, we’ll be better prepared to decide if this is a good step.
The key idea of SAI, which is a form of climate geoengineering, is to spray reflective particles into the stratosphere to reflect a little more, say 1%, of the sunlight that otherwise would fall on Earth back into space. This small increase in reflected sunlight would be sufficient to mitigate much of the impact of human-induced warming. For example, in 1991, Mount Pinatubo ejected almost 20 tons of aerosols (sulfur dioxide) into the atmosphere and cooled down the planet by around 0.5 degrees Celsius over the following year. We should be able to induce cooling equivalent to, say, a fraction of Mount Pinatubo, via a fair, international process that’s backed by science.
There are many criticisms of SAI, such as:
- It could have unintended climate consequences, for example, disrupting local weather patterns and creating droughts or floods.
- If it were started and then stopped suddenly, it could lead to sudden warming, known as “termination shock.”
- Depending on the aerosol used (sulfur dioxide is a leading candidate), it could contribute to pollution and/or ozone depletion.
- It might reduce urgency to decarbonize (an example of a “moral hazard”).
In addition, many people have a visceral emotional reaction, as I once did before I understood the science more deeply, against “playing god” by daring to engineer the planet.
All these downsides should be balanced against the reality that people are dying.
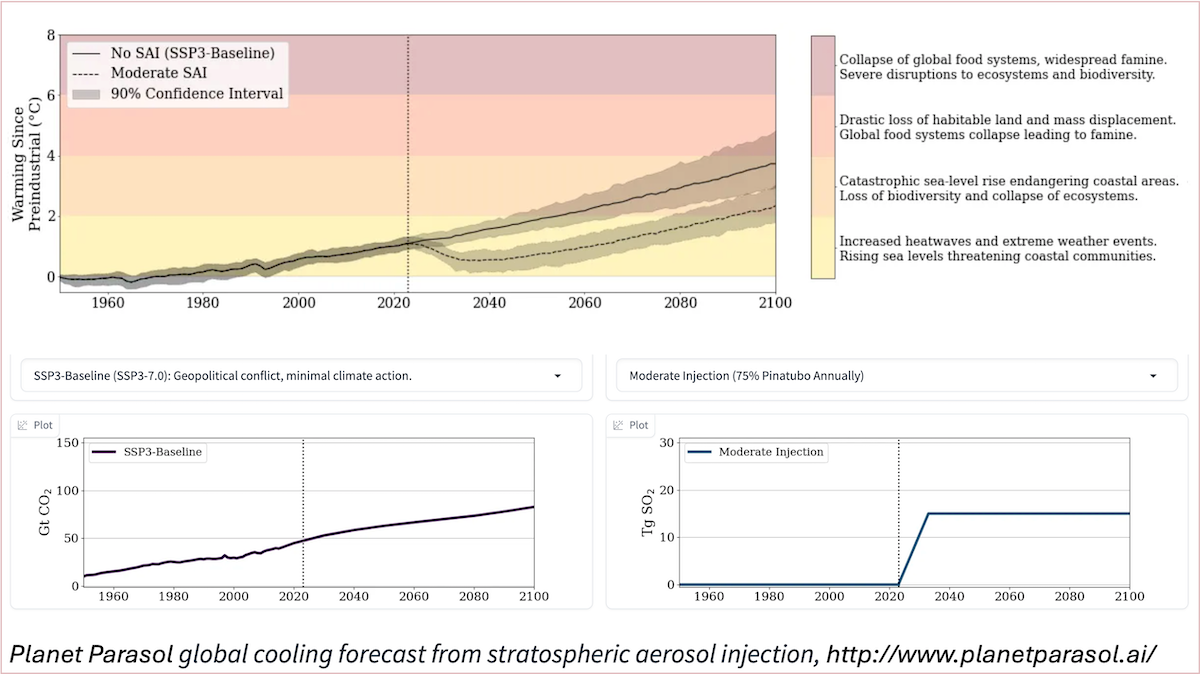
I’m moved by meteorologist John Morales’ emotional account of the havoc caused by Hurricane Milton. The New York Times quoted him as saying, “It claims lives. It also wrecks lives.”
Skyfire AI, a drone company led by CEO Don Mathis that my team AI Fund helped to co-build, was recently on the ground in the aftermath of Helene and Milton, deploying drones to help emergency responders survey remote areas and find survivors. Mathis reports that Skyfire was credited with saving at least 13 lives. On Monday, I also spoke about AI applied to renewable energy with AES’ CEO Andres Gluski and CPO Chris Shelton. You can view our conversation here.
While I’m glad that AI can help mitigate these disasters, it saddens me that so many lives have already been lost due to climate-influenced causes. My mind frequently returns to SAI as one of the few untapped tools in our arsenal that can help. We need to be investing in SAI research now.
I’m grateful to my collaborators on the Planet Parasol emulator (a group that includes many climate scientists) including Jeremy Irvin, Daniele Visioni, Ben Kravitz, Dakota Gruener, Chris Smith, and Duncan Watson-Parris. MIT Technology Review’s James Temple wrote about his experience playing with our emulator and also outlines fair criticisms. Much work remains to be done, and making sure our actions are based on science — a task that AI can help with (witness the recent Chemistry and Physics Nobel Prizes going to innovators in AI!) – will help us make better decisions.
If you’re interested in learning more about SAI, check out this recent panel discussion where I spoke alongside climate scientists Chris Field, David Keith, Douglas MacMartin, and Simone Tilmes about the science and possible roadmaps ahead.
Keep learning!
Andrew
A MESSAGE FROM DEEPLEARNING.AI
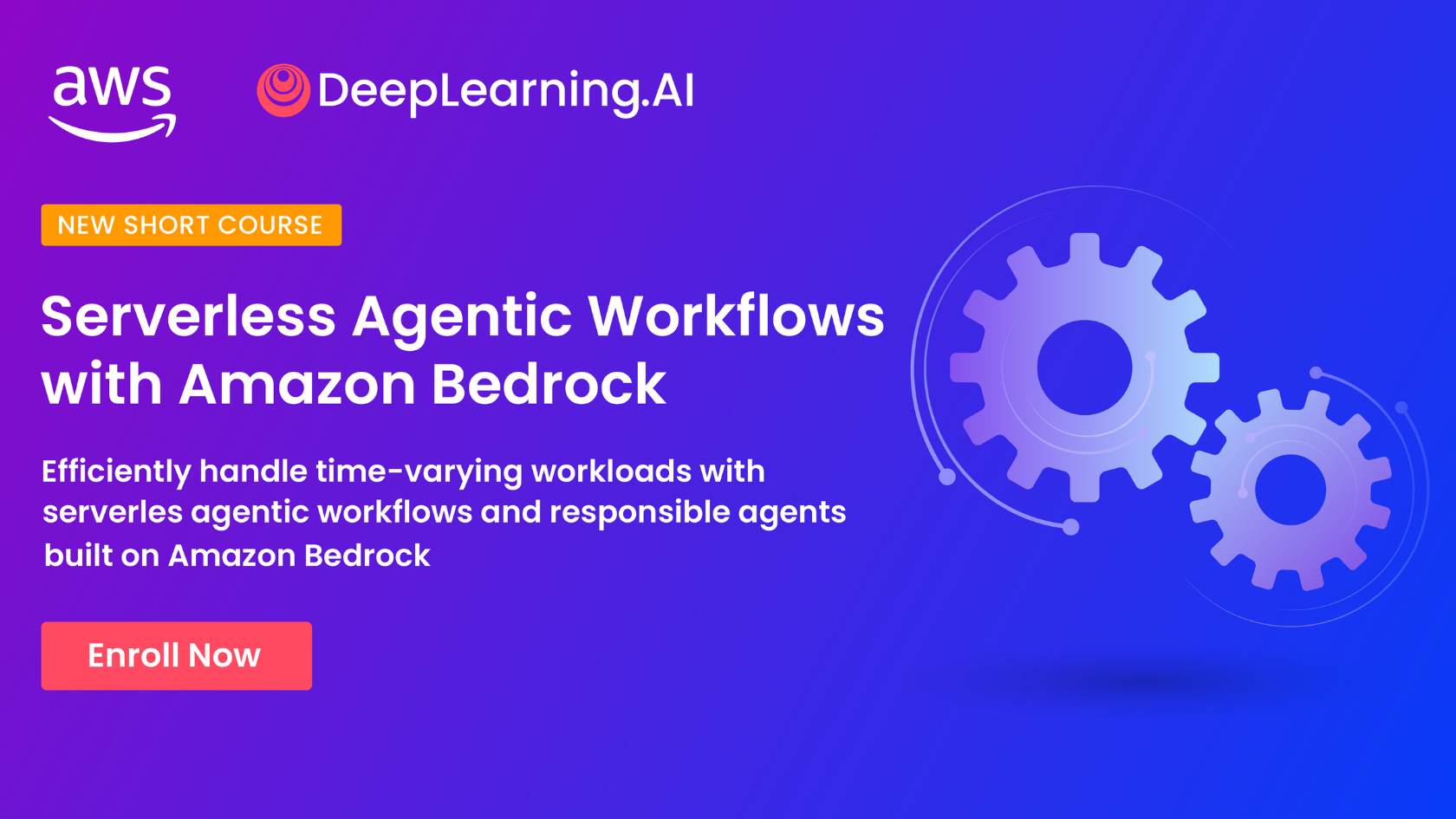
In this course, you’ll learn to build scalable agents without managing infrastructure. Explore agentic workflows, tool integration, and setting up guardrails for secure and responsible operations. Sign up today
News
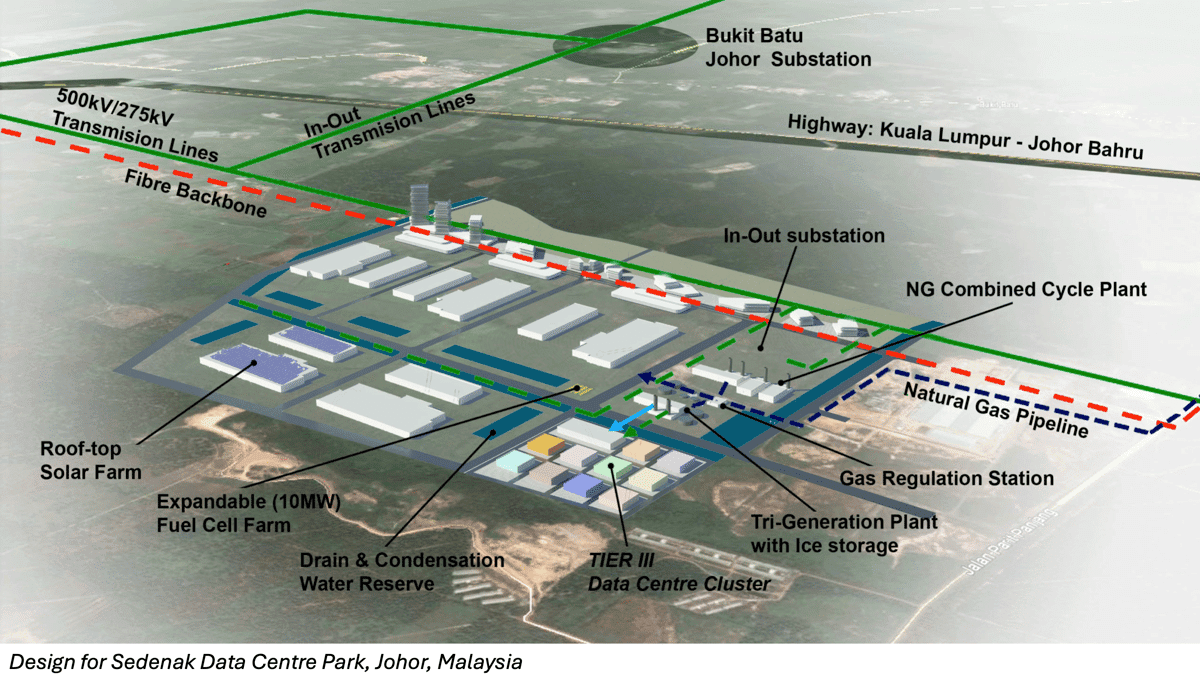
Malaysia’s Data Center Boom
Malaysia’s location, natural resources, and investor-friendly government are perfect for data centers, turning part of the country into an AI-fueled boomtown.
What’s new: Data center construction is flourishing in the southern Malaysian state of Johor, where companies including ByteDance and Microsoft are spending billions of dollars on facilities, The Wall Street Journal reported. These data centers will provide processing power for AI, cloud computing, and telecommunications.
How it works: Data center construction has slowed in established areas like Ireland and Northern Virginia as space and resources have become scarce. All regions face shortages of electrical power, analysts say, and some U.S. locations face public resistance to new projects. Johor has emerged as an attractive alternative.
- Johor has space, energy (mostly coal), water for cooling, and proximity to Singapore, a global communications hub that lacks the land and power to host many new data centers. The Malaysian government and local politicians streamlined the permitting process and advocated for additional infrastructure, such as water desalination plants, to support such projects. Moreover, Malaysia’s strong relationships with both the U.S. and China reduce political risks for companies that operate in the region.
- Data center investments in Johor will reach $3.8 billion this year, according to regional bank Maybank. ByteDance allocated $350 million for data center construction in the region. Microsoft purchased land nearby for $95 million and announced a plan to spend $2.2 billion. Oracle expects to invest $6.5 billion in Malaysia.
- While some tech giants are building their own data centers, independent operators are building facilities to serve companies like Amazon, Alphabet, and Meta.
Behind the news: The Asia-Pacific region is second to North America in data center construction, according to one recent report, ahead of Europe, South America, and the Middle East and Africa. As Johor builds out its data-center inventory, it will compete with established Asia-Pacific markets in Hong Kong, Mumbai, Seoul, Singapore, Sydney, and Tokyo.
Why it matters: AI is poised to transform virtually every industry, but doing so requires ample processing power. The data-center buildout will help fuel improvements in AI as well as spread the technology to new industries and bring its benefits to people throughout the world. Malaysia’s role as a data center hub is also bound to bring huge economic benefits to the country itself.
We’re thinking: Many data centers have been built near users to reduce latency. But the cost of processing compute-intensive AI workloads is so high relative to the cost of transmitting data that it makes sense to transmit AI-related data long distances for processing. (As Andrew wrote, the gravity of data is decreasing.) We hope the increasing flexibility in siting data centers will enable more nations that aren’t traditional tech hubs to participate in the tech economy and reap significant benefits from doing so.
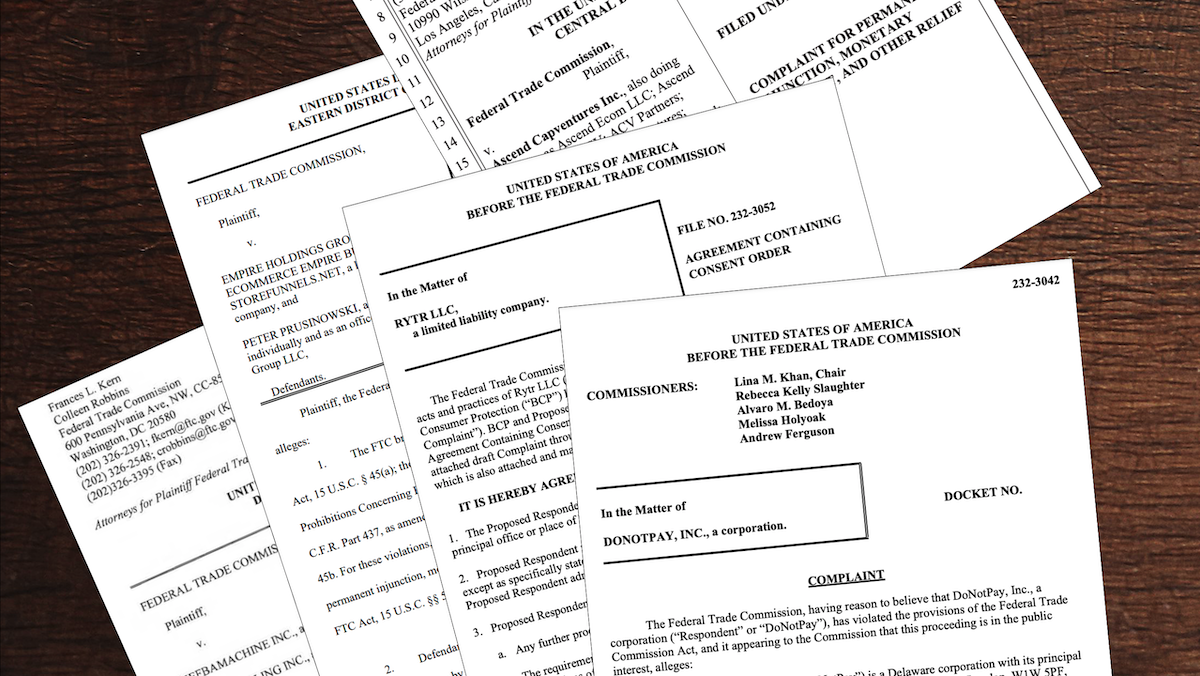
U.S. Cracks Down on AI Apps That Overpromise, Underdeliver
The United States government launched Operation AI Comply, targeting businesses whose uses of AI allegedly misled customers.
What’s new: The Federal Trade Commission (FTC) took action against five businesses for allegedly using or selling AI technology in deceptive ways. Two companies settled with the agency, while three face ongoing lawsuits.
How it works: The FTC filed complaints against the companies based on existing laws and rules against unfair or deceptive commercial practices. The FTC alleges:
- DoNotPay claimed its AI service was a “robot lawyer” that could substitute for human legal expertise. The FTC said the company misled consumers about its system’s ability to handle legal matters and provide successful outcomes. DoNotPay settled the case, paying $193,000 in consumer redress and notifying customers about the limitations of its services.
- Rytr, a writing tool, generated fake reviews of companies. According to the FTC, Rytr offered to create and post fake reviews on major platforms like Google and Trustpilot, which helped it to bring in $3.8 million in revenue from June 2022 to May 2023. Rytr agreed to settle and is barred from offering services that generate consumer reviews or testimonials. The settlement amount was not disclosed.
- Ascend Ecommerce claimed that its “cutting-edge” AI-powered tools would help consumers quickly earn thousands of dollars monthly through online storefronts. The company allegedly charged thousands of dollars for its services, but the promised returns failed to materialize, defrauding customers of at least $25 million. The government temporarily halted the company’s operations and froze its assets.
- Ecommerce Empire Builders promised to help consumers build an “AI-powered Ecommerce Empire” through training programs that cost customers nearly $2,000 each, or readymade online storefronts that cost tens of thousands of dollars. A federal court temporarily halted the scheme.
- FBA Machine said its AI-powered tools could automate the building and management of online stores on platforms like Amazon and Walmart. The company promoted its software with guarantees that customers’ monthly earnings would exceed $100,000. Consumers paid nearly $16 million but didn’t earn the promised profits. A federal court temporarily halted FBA’s operations.
Behind the news: The FTC has a broad mandate to protect consumers, including both deceptive and anticompetitive business practices. In June, it agreed to focus on Microsoft’s investment in OpenAI and Google’s and Amazon’s investments in Anthropic, while the U.S. Department of Justice would examine Nvidia’s dominant market share in chips designed to process AI workloads. The FTC previously brought cases against Rite Aid for misuse of AI-enabled facial recognition, Everalbum for deceptive use of facial recognition, and CRI Genetics, which misled consumers while using AI to conduct DNA tests.
Why it matters: The FTC’s enforcement actions send a message to businesses that aim to take advantage of the latest AI models: making exaggerated claims about AI will bring legal consequences. The complaints point to a set of issues: falsely claiming to use AI to provide a particular service, exaggerating AI’s ability to replace human expertise, generating fake reviews of businesses, promising unrealistic financial returns, and failing to disclose crucial information about AI-based services.
We’re thinking: These particular actions crack down not on AI per se but on companies that allegedly deceived consumers. By taking scams off the market while leaving legitimate businesses to operate freely, they may actually increase customer trust in AI.
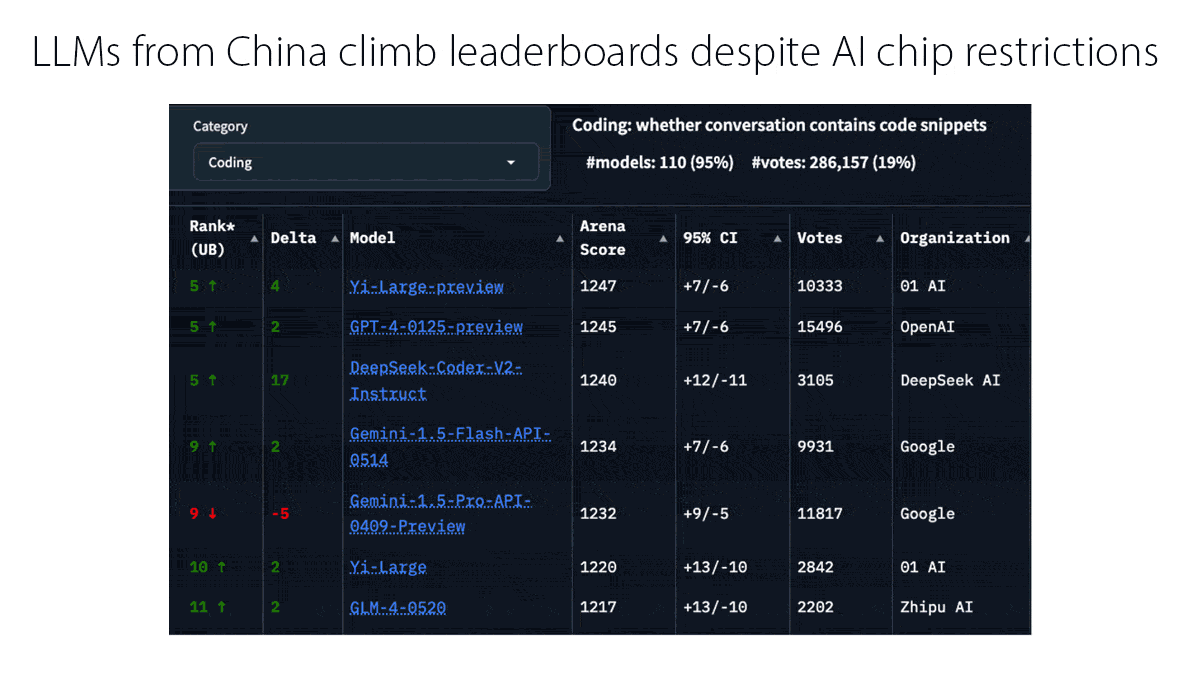
A Year of Contending Forces
A new report documents the interplay of powerful forces that drove AI over the past year: open versus proprietary technology, public versus private financing, innovation versus caution.
What’s new: Drawn from research papers, news articles, earnings reports, and the like, the seventh annual State of AI Report recaps the highlights of 2024.
Looking back: AI’s rapid advance in 2024 was marked by groundbreaking research, a surge of investment, international regulations, and a shift in safety concerns from hypothetical risks to real-world issues, according to investors Nathan Benaich and Ian Hogarth.
- Top models: Anthropic’s Claude, Google’s Gemini, and Meta’s Llama largely closed the gap with OpenAI’s top multimodal model, GPT-4o, before its successor o1 raised the bar for reasoning. Meanwhile, models built in China such as DeepSeek, Qwen, and Kling challenged the top models despite the United States’ restrictions on exports of the most powerful AI chips. The year saw a proliferation of models small enough to run on local devices, such as Gemini Nano (3.25 billion parameters) and the smaller of Apple’s AFM family (3 billion parameters).
- Research: Model builders settled on mixtures of curated natural and synthetic data for training larger models (Microsoft’s Phi family, Anthropic Claude 3.5 Sonnet, Meta Llama 3.1) and knowledge distillation for training smaller ones (Flux.1, Gemini 1.5 Flash, Mistral-NeMo-Minitron, and numerous others). Meanwhile, researchers established benchmarks to measure new capabilities like video understanding and agentic problem-solving. Another motivation for new benchmarks is to replace older tests in which new models consistently achieve high scores, possibly because the test data had contaminated their training data.
- Finance: Investment boomed. The chip designer Nvidia contributed nearly one-third of the AI industry’s $9 trillion total value, including public and private companies, and the combined value of public AI companies alone exceeded the entire industry’s value last year. The most dramatic single trend in AI finance was the shift by major public companies from acquisitions to acquisition-like transactions, in which tech giants took on talent from top startups, sometimes in exchange for licensing fees, without buying them outright: notably Amazon-Covariant, Google-Character.AI, and Microsoft-Inflection. In venture investment, robotics now accounts for nearly 30 percent of all funding. Standouts included the humanoid startup Figure with a $675 million round at a $2.6 billion valuation and its competitor 1X with a $125 million round.
- Regulation: Regulation of AI remains fragmented globally. The U.S. issued executive orders that mainly relied on new interpretations or implementations of existing laws. Europe’s AI Act sought to balance innovation and caution by declaring that large models pose a special risk and banning applications such as predictive policing, but some observers have deemed it heavy-handed. China focused on enforcement of its more restrictive laws, requiring companies to submit models for government review. Widespread fears that AI would disrupt 2024’s many democratic elections proved unfounded.
- Safety: While anxieties in 2023 focused on abstract threats such as the risk that AI would take over the world, practical concerns came to the fore. Model makers worked to increase transparency, interpretability, and security against external attacks. Actual security incidents occurred on a more personal scale: Bad actors used widely available tools to harass and impersonate private citizens, notably generating fake pornographic images of them, which remains an unsolved problem.
Looking forward: The authors reviewed predictions they made in last year’s report — among them, regulators would investigate the Microsoft/OpenAI Partnership (accurate), and a model builder would spend over $1 billion on training (not yet) — and forecast key developments in 2025:
- An open source model will outperform OpenAI’s proprietary o1 on reasoning benchmarks.
- European lawmakers, fearing that the AI Act overreaches, will refrain from strict enforcement.
- Generative AI will hit big. A viral app or website built by a noncoder or a video game with interactive generative AI elements will achieve breakout success. An AI-generated research paper will be accepted at a major machine learning conference.
Why it matters: The authors examined AI from the point of view of investors, keen to spot shifts and trends that will play out in significant ways. Their report dives deep into the year’s research findings as well as business deals and political currents, making for a well rounded snapshot of AI at the dawn of a new year.
We’re thinking: The authors are bold enough to make clear predictions and self-critical enough to evaluate their own accuracy one year later. We appreciate their principled approach!
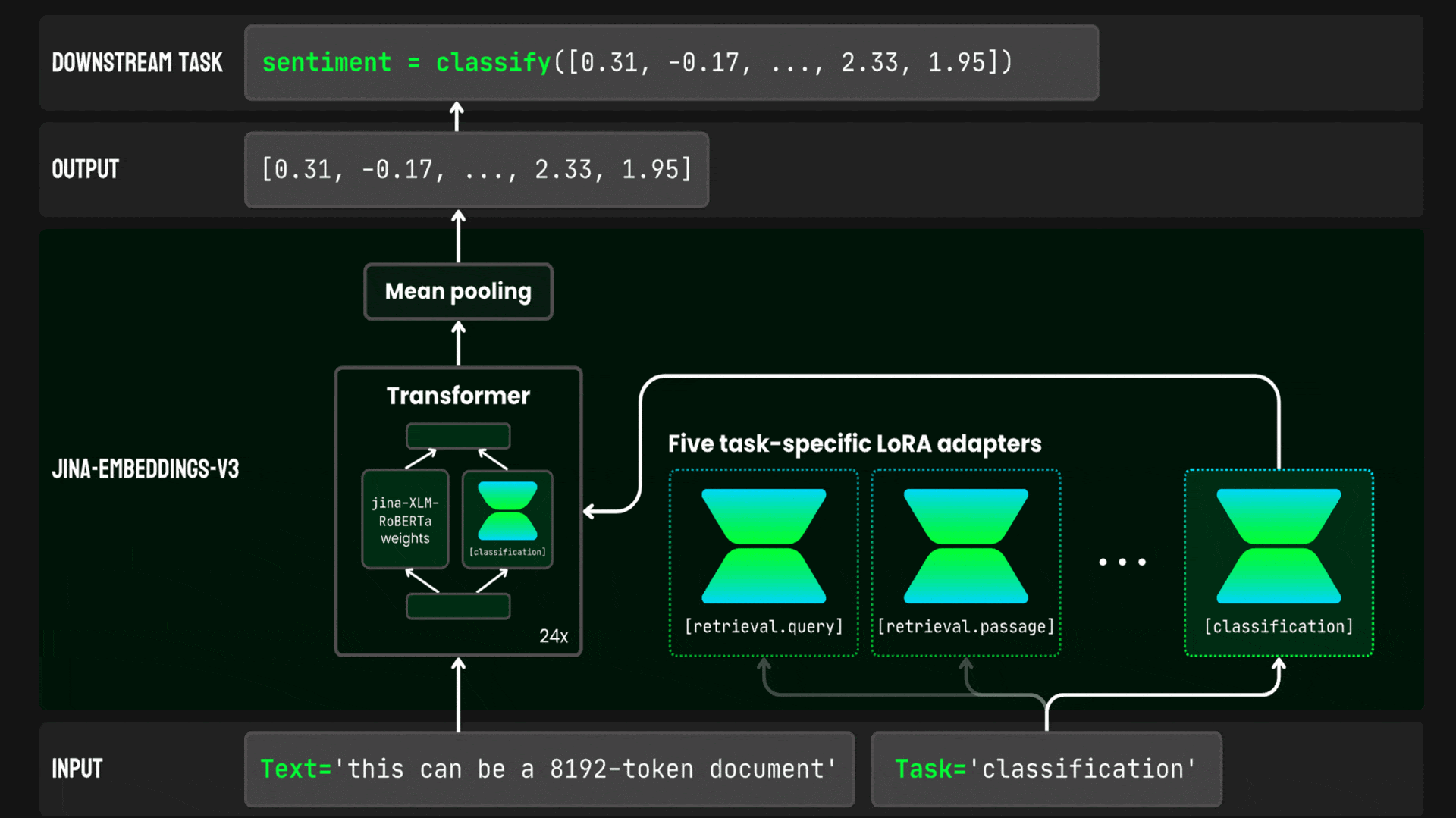
Better Text Embeddings
Text embedding models are often used to retrieve text, cluster text, determine similarity between texts, and generate initial embeddings for text classifiers. A new embedding model comes with adapters that specialize it to each of these use cases.
What’s new: Saba Sturua and colleagues at Jina AI released jina-embeddings-v3, a text-embedding system with open weights that can process 8,192 input tokens and output embeddings of 1,024 values. It’s free for noncommercial use and competes with closed weight models from Cohere and OpenAI.
How it works: Jina-embeddings-v3 comprises a transformer (559 million parameters) and five LoRA adapters that plug into the model and adjust its weights for retrieval, clustering, determining similarity, and classification. Two adapters adjust the model for retrieval: one for documents and one for queries.
- The authors started with a pretrained XLM-RoBERTa. They further pretrained it to predict masked words in data from text in 89 languages.
- They add a mean pooling layer to average output vectors into one embedding. They fine-tuned the model, using an unspecified dataset of 1 billion text pairs in various languages, to produce similar embeddings for matching text pairs and dissimilar embeddings for non-matching text pairs.
- They fine-tuned the five adapters on the four tasks. For retrieval, they trained the two adapters to produce similar embeddings of matching queries and documents and dissimilar embeddings for queries and documents that didn’t match. For clustering, the authors fine-tuned the adapter to produce more-similar embeddings of examples from the same class and less-similar embeddings of examples from different classes. Text similarity worked in a related manner: they fine-tuned the adapter to produce more-similar embeddings of similar examples than dissimilar examples. For classification, they fine-tuned the adapter to produce similar embeddings of examples of the same class and different embedding of different classes.
- They modified the loss function during training using matryoshka representation learning. This method encourages the loss function to solve the problem at hand using the first 32, 64, 128, 256, 512, and 768 values of the embedding as effectively as it would if it used all 1,024 values.
Results: The authors compared jina-embeddings-v3 to Cohere’s multilingual embed v3, OpenAI’s text-embedding-3-large, and Microsoft’s open-weights Multilingual-E5-large-instruct. They tested their system on the Massive Text Embedding Benchmark (MTEB) for embedding tasks.
- On English-language tasks, Jina-embeddings-v3 achieved an average score of 65.52 percent, while OpenAI achieved 64.6 percent, Microsoft 64.41 percent, and Cohere 64.01 percent. For example, when they trained logistic classifiers on embeddings produced by the various models, jina-embeddings-v3 performed best as classification, achieving an average accuracy of 82.58 percent, while OpenAI achieved 75.45 percent, Microsoft 77.56 percent, and Cohere 76.01 percent.*
- The team also tested how well smaller versions of the embedding performed on retrieval. Medium sizes reduced performance only slightly. For instance, using all 1,024 values for retrieval, the model achieved 63.35 percent normalized discounted cumulative gain (nDCG), a measure of how well the model ranks the retrieved documents (higher is better). When it used the first 32 values, the model achieved 52.54 percent nDCG; and when it used 128 values, it achieved 61.64 percent nDCG.
Why it matters: Training a set of LoRA adapters is becoming the go-to method for adapting a pretrained model for a variety of tasks. Jina extends the list to computing embeddings for different language tasks and gives developers a further option for generating high-quality embeddings.
We’re thinking: The authors’ results show that using embeddings that are one-eighth the typical size degrades performance by only 2 percent. That tradeoff may be worthwhile if your computational budget is constrained or your task is especially data-intensive.