Dear friends,
Startups live or die by their ability to execute at speed. For large companies, too, the speed with which an innovation team is able to iterate has a huge impact on its odds of success. Generative AI makes it possible to quickly prototype AI capabilities. AI capabilities that used to take months can sometimes be built in days or hours by simply prompting a large language model. I find this speed exciting and have been thinking about how to help startups and large companies alike go faster.
I’ve been obsessed with speedy execution for a long time. When working on a project, I am loath to take two weeks to do something that I could do in one week. The price of moving at that pace is not that we take one week longer (which might be okay) but that we’re 2x slower (which is not)!
When building an AI-powered product, there are many steps in designing, building, shipping, and scaling the product that are distinct from building the AI capability, and our ability to execute these other steps has not sped up as much as the AI part. But the speed with which we can prototype AI creates significant pressure to speed up these other steps, too. If it took 6 months to collect data, train a supervised learning algorithm, and deploy the model to the cloud, it might be okay to take 2 months to get user feedback. But if it takes a week to build a prototype, waiting 2 months for feedback seems intolerably slow!
I’d like to focus on one key step of building applications: getting user feedback. A core part of the iterative workflow of designing and building a product (popularized by Eric Ries in his book The Lean Startup) is to build a prototype (or MVP, minimum viable product), get user feedback on it, and to use that feedback to drive improvements. The faster you can move through this loop — which may require many iterations — the faster you can design a product that fits the market. This is why AI Fund, a venture studio that I lead, uses many fast, scrappy tactics to get feedback.
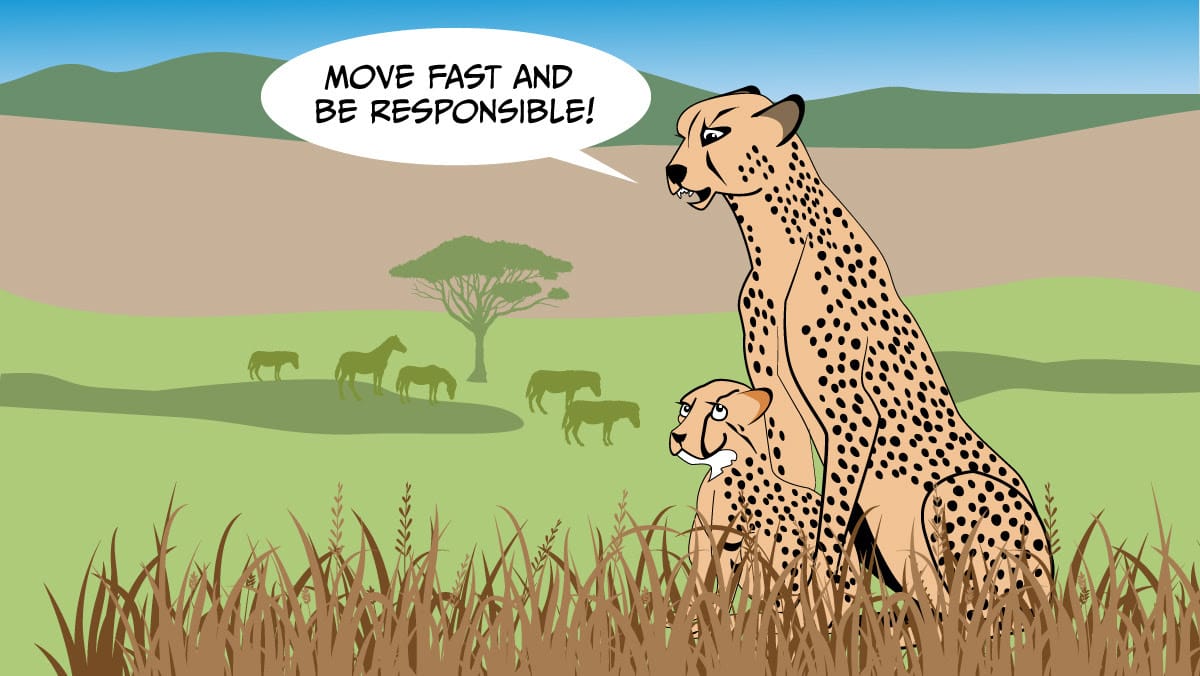
For B2C (business to consumer) offerings, here is a menu of some options for getting customer feedback:
- Ask 3 friends or team members to look at the product and let you know what they think (this might take ~0.5 days).
- Ask 10 friends or team members to take a look (~2 days).
- Send it to 100 trusted/volunteer alpha testers (~1 week?).
- Send it to 1,000 users to get qualitative or quantitative feedback (~2 weeks?).
- Incorporate it into an existing product to get feedback (1 to 2 months?).
- Roll it out to a large user base of an existing product and do rigorous A/B testing.
As we go down this list, we get (probably) more accurate feedback, but the time needed to get that feedback increases significantly. Also, the tactics at the top of the list create basically no risk, and thus it’s safe to repeatedly call on them, even with preliminary ideas and prototypes. Another advantage of the tactics further up the list is that we get more qualitative feedback (for example, do users seem confused? Are they telling us they really need one additional feature?), which sparks better ideas for how to change our product than an A/B test, which tells us with rigor whether a particular implementation works but is less likely to point us in new directions to try. I recommend using the fast feedback tactics first. As we exhaust the options for learning quickly, we can try the slower tactics.
With these tactics, scrappy startup leaders and innovation-team leaders in large companies can go faster and have a much higher chance of success.
The mantra “move fast and break things” got a bad reputation because, well, it broke things. Unfortunately, some have interpreted this to mean we should not move fast, but I disagree. A better mantra is “move fast and be responsible.” There are many ways to prototype and test quickly without shipping a product that can cause significant harm. In fact, prototyping and testing/auditing quickly before launching to a large audience is a good way to identify and mitigate potential problems.
There are numerous AI opportunities ahead, and our tools are getting better and better to pursue them at speed, which I find exhilarating!
Keep learning!
Andrew
A MESSAGE FROM DEEPLEARNING.AI
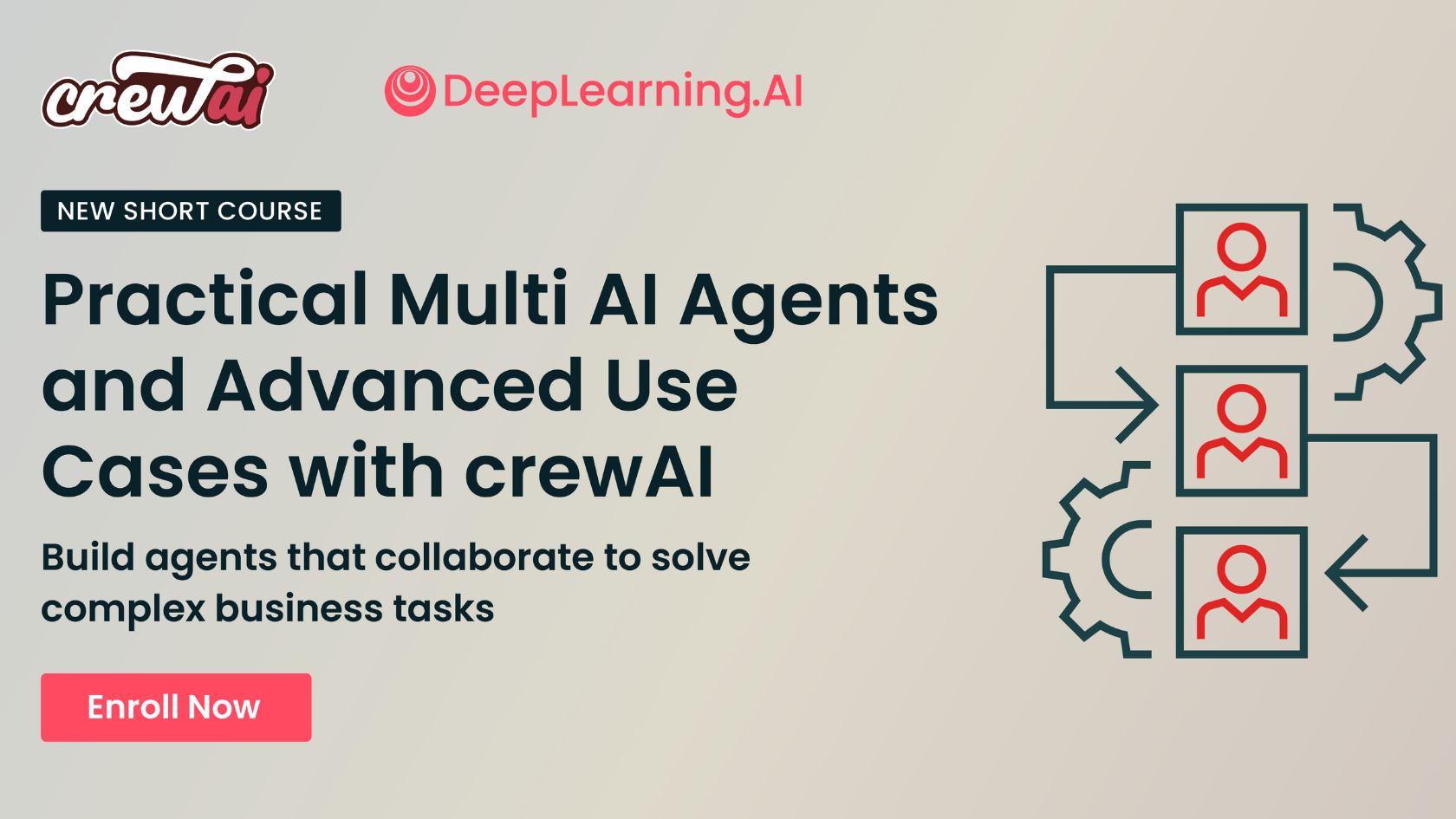
Build advanced, multi-agent systems for project planning, sales pipelines, customer support analysis, and content creation in our new course with crewAI! Gain hands-on skills in performance testing, multi-model setups, and using human feedback to optimize AI agents. Enroll for free
News
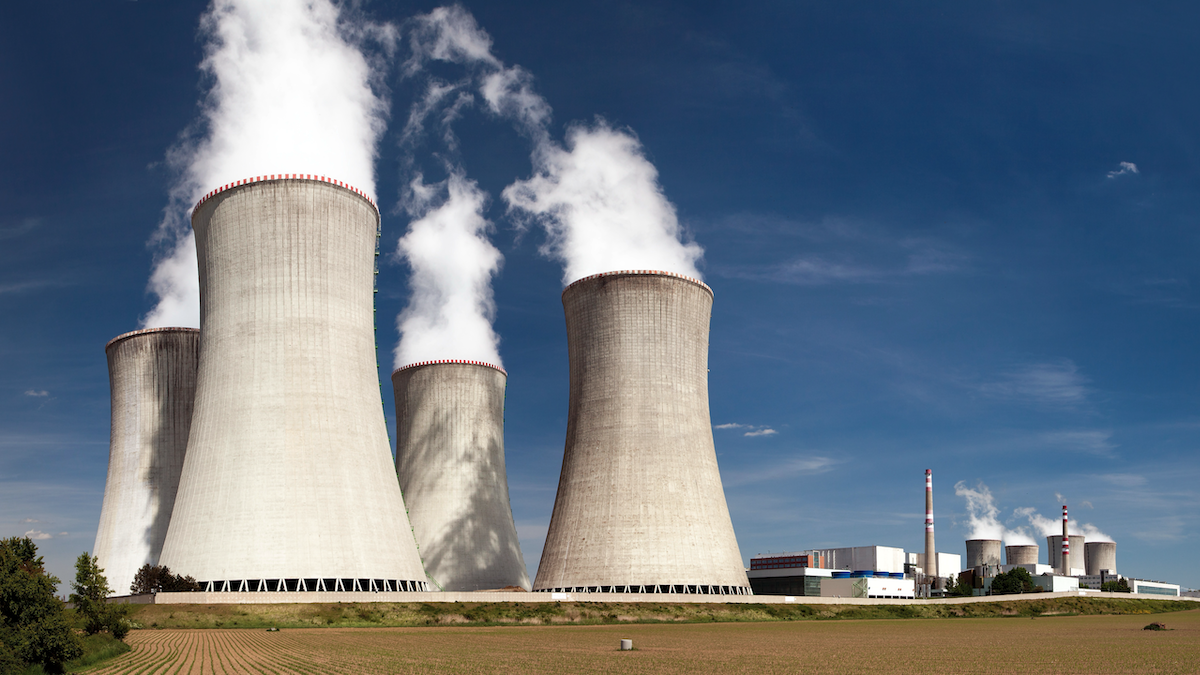
AI Giants Go Nuclear
Major AI companies plan to meet the growing demand with nuclear energy.
What’s new: Amazon, Google, and Microsoft announced substantial investments in nuclear power projects. Amazon and Google forged partnerships to build a new generation of small reactors, while Microsoft cut a deal to revive a shuttered nuclear plant. (Andrew Ng is a member of Amazon’s board of directors.)
How it works: Nuclear power provides around 18 percent of electricity in the United States and more in France and several other European countries. Its steady generating capacity and zero carbon emissions (after plant construction) make it an attractive way to power AI infrastructure. However, new nuclear plants have been difficult to build in the U.S. since a string of high-profile accidents at Three Mile Island in the U.S. (1979), Chernobyl in Ukraine (1986), and Fukishima in Japan (2011). Since then, pressure to reduce carbon emissions has driven calls to build new plants. In March, President Biden signed legislation that streamlines construction and regulation of nuclear plants.
- Amazon is taking part in a number of nuclear projects. It led a $500 million investment in X-energy, a designer of small modular reactors, an emerging class of lower-cost reactor designs. X-energy’s reactors use advanced fuel that surrounds nuclear particles with carbon and ceramic to resist corrosion, rust, melting, or other dangers of high-temperature reactors. (The International Atomic Energy Agency regards small modular reactors as safer than earlier reactors. The Union of Concerned Scientists expresses doubts.) In addition, Amazon announced a partnership with the utility consortium Energy Northwest to deploy a 320-megawatt X-energy reactor in the state of Washington, which may expand to 960 megawatts. Separately, Amazon agreed with Dominion Energy to build a small modular reactor in Virginia, which would give Amazon’s data centers an additional 300 megawatts.
- Google partnered with Kairos Power to develop small modular reactors. Terms of the deal have not been disclosed. Kairos expects the new plants to begin operation in 2030, with more planned by 2035, providing up to 500 megawatts of electricity. This summer, Kairos broke ground on a demonstration unit in Tennessee, the first small modular reactor project permitted by the U.S. Nuclear Regulatory Commission, which is expected to open in 2027.
- In September, Microsoft signed a 20-year power purchase agreement with Constellation Energy, which intends to restart Unit 1 of Pennsylvania’s Three Mile Island nuclear plant (which was not damaged in the 1979 partial meltdown) by 2028.
Behind the news: The tech industry’s growing interest in nuclear power is driven by surging demand for AI and corporate commitments to reduce carbon emissions. Data centers that train and run AI models consume vast amounts of electricity, and nuclear energy offers a reliable, carbon-free source. Microsoft, Nvidia, and OpenAI have urged the White House to deliver a so-called “energy New Deal” that would allocate hundreds of billions of dollars to subsidize new power plants.
Why it matters: The fact that tech giants are investing directly in nuclear power plants indicates the high stakes of competition in AI. Economists estimate that data centers that process AI, among other workloads, will consume more than 1,000 terawatt-hours of electricity by 2026, more than double the amount they consumed in 2022. Nuclear power could give them bountiful, carbon-free energy for decades to come.
We’re thinking: Fossil fuels like coal do tremendous damage to the environment, while renewables like solar and wind energy can’t fully meet the always-on demands of AI infrastructure. Next-generation reactor designs that improve safety and reduce costs are worth exploring. However, a significant obstacle remains: Few countries have a certifiably safe repository for long-term disposal of highly radioactive spent fuel. U.S. efforts toward this goal are stalled.
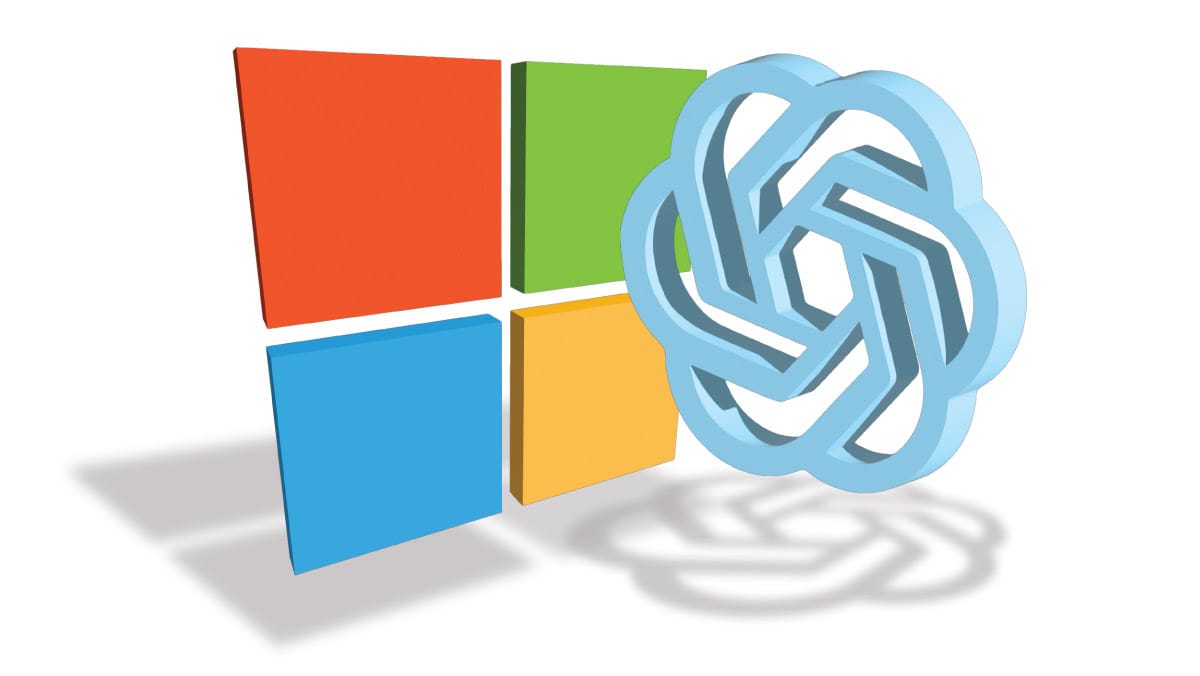
AI Bromance Turns Turbulent
Once hailed by OpenAI chief Sam Altman as the “best bromance in tech,” the partnership between Microsoft and OpenAI is facing challenges as both companies seek greater independence.
What’s new: Sources inside Microsoft and OpenAI revealed that both companies are working to reduce their reliance on the other, according to The New York Times. Their collaboration, which brought both companies great rewards, is now complicated by demands for resources, friction between leaders, and partnerships with other companies.
How it works: In a series of deals that started in 2019, Microsoft invested a total of $13 billion in OpenAI, giving the startup access to Microsoft’s processing infrastructure and Microsoft special access to OpenAI’s models (which it integrated into its own applications), a large cut of its revenue, and potential equity. Microsoft built a 10,000-GPU system on Azure for training OpenAI models. But OpenAI sought to renegotiate its agreements, while Microsoft continued to develop its own AI capabilities.
- Last year, OpenAI CEO Sam Altman negotiated for further investment from Microsoft. But Microsoft reconsidered its commitment after OpenAI briefly ousted Altman in November. The tech giant’s hesitation strained relations as OpenAI continued to seek more funding and computing power.
- In April, Microsoft hired former Inflection AI CEO Mustafa Suleyman to head up its AI efforts. Suleyman’s aggressive leadership, including his frustration over what he perceived as OpenAI’s slow progress delivering new technologies, raised tensions between the parties.
- Microsoft engineers reportedly downloaded critical OpenAI software without following protocols the two companies had agreed upon, further straining the relationship.
- In June, Microsoft agreed to an exception in the partnership that allowed OpenAI to cut a $10 billion deal with Oracle for additional computing power. More recently, it cut the price it charged the startup for cloud computing.
- Under the original agreement, Microsoft would lose access to OpenAI’s technologies if the startup were to develop artificial general intelligence (AGI). This clause was intended to prevent commercial exploitation or abuse of emergent AI capabilities. However, it allows OpenAI’s board of directors to declare that the company has achieved AGI, which could enable OpenAI to exit the contract or give it leverage in renegotiations.
Behind the news: OpenAI’s valuation soared to $157 billion with new funding from Nvidia and other investors following a period of mounting financial pressure. The increased valuation gives OpenAI new power in its relationship with Microsoft. Moreover Microsoft holds no seats on its nonprofit board of directors, which limits its influence over strategic decisions at OpenAI despite its significant financial stake in the startup’s for-profit wing.
Why it matters: The Microsoft-OpenAI partnership has reshaped the AI landscape, and shifts in their partnership have an outsized impact on a wide range of research and product development. Their evolving relationship illustrates the challenge of sustaining a close collaboration amid rapidly changing technology. Microsoft provided vital resources that helped OpenAI scale up, while OpenAI’s models enabled Microsoft to keep rivals off-balance as it reinvented products including Bing, Windows, Office, Azure, and its expanding line of Copilots. However, facing fierce competition, both companies need ample flexibility to innovate and adapt.
We’re thinking: Together and separately, Microsoft and OpenAI have done tremendous work to advance the field from research to applications. We hope they can strike a balance that maintains their partnership and fuels their growth.
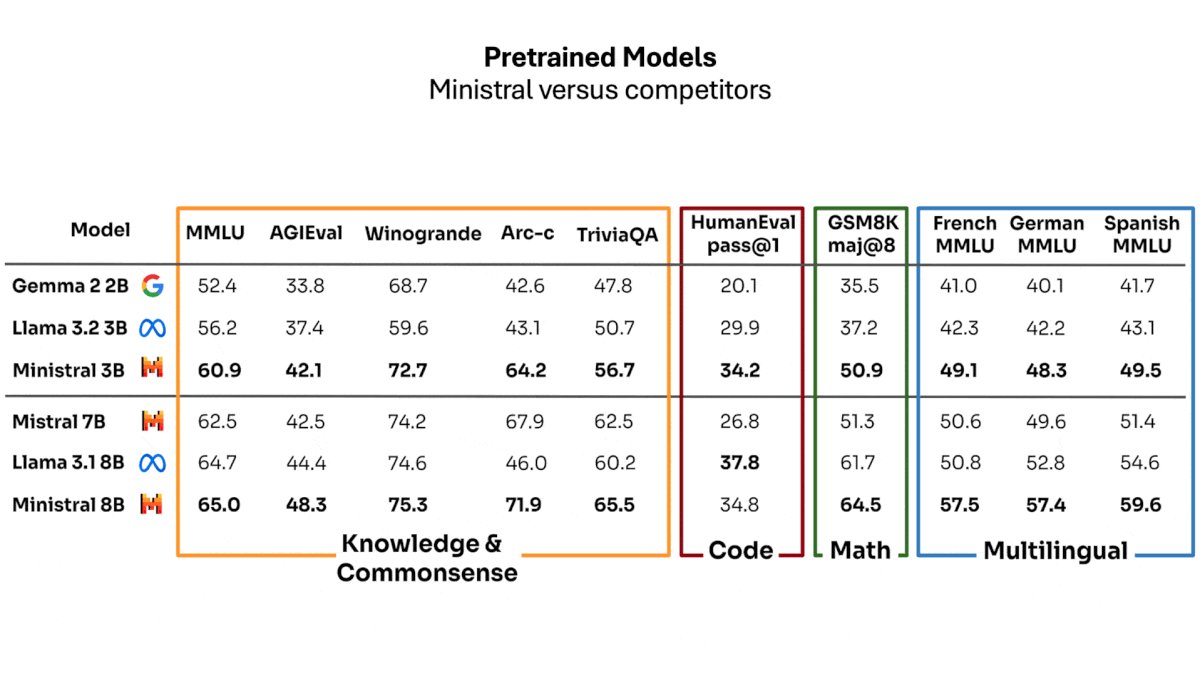
Mistral AI Sharpens the Edge
Mistral AI launched two models that raise the bar for language models with 8 billion or fewer parameters, small enough to run on many edge devices.
What’s new: Ministral 3B and Ministral 8B, which come in base and instruction-tuned versions, outperform Google’s and Meta’s similar-sized models on several measures of knowledge retrieval, common-sense reasoning, and multilingual understanding. Ministral 8B-Instruct is free to download and use for noncommercial purposes, and commercial licenses are negotiable for this model and the others in the family. Accessed via Mistral’s APIs, Ministral 3B costs $0.04 per million tokens of input and output, and Ministral 8B costs $0.10 per million tokens of input and output.
How it works: The Ministral family can process 131,072 tokens of input context. The models are built to support function calling natively to interact, for example, with external APIs that fetch real-time weather data or control smart-home devices.
- Ministral 3B is sized for smaller devices like smartphones. In Mistral’s tests, it surpassed Gemma 2 2B and Llama 3.2 3B on MMLU, AGIEval, and TriviaQA (question answering and common-sense reasoning), GSM8K (math), HumanEval (coding), and multilingual tasks in French, German, and Spanish. Independent tests by Artificial Analysis show Ministral 3B behind Llama 3.2 3B on MMLU and MATH.
- In Mistral’s tests, the instruction-tuned Ministral 3B-Instruct outperformed Gemma 2 2B and Llama 3.2 3B across several benchmarks including GSM8K, HumanEval, and three arena-style competitions judged by GPT-4o.
- Ministral 8B targets more powerful devices like laptops and requires 24GB of GPU memory to run on a single GPU. In Mistral’s tests, it outperformed its predecessor Mistral 7B and Meta’s Llama 3.1 8B on most benchmarks reported except HumanEval one-shot, where it was slightly behind Llama 3.1 8B. Independent tests by Artificial Analysis show Ministral 8B behind Llama 3.1 8B and Gemma 2 9B on MMLU and MATH.
- In Mistral’s tests, Ministral 8B-Instruct outperformed its peers on all benchmarks reported except WildBench, on which Gemma 2 9B Instruct achieved a higher score. WildBench tests responses to real-world requests that include digressions, vague language, idiosyncratic requirements, and the like.
Behind the news: Headquartered in France, Mistral AI competes head-to-head in AI with U.S. tech giants. It released its first model, Mistral 7B, a year ago under an Apache open source license. Since then, it has released model weights under a range of licenses while exploring alternative architectures such as mixture-of-experts and mamba. It also offers closed models that are larger and/or built for specialized tasks like code generation and image processing.
Why it matters: Edge devices can play a crucial role in applications that require fast response, high privacy and security, and/or operation in the absence of internet connectivity. This is particularly important for autonomous and smart home devices where uninterrupted, rapid processing is critical. In addition, smaller models like Ministral 8B-Instruct enable developers and hobbyists to run advanced AI on consumer-grade hardware, lowering costs and broadening access to the technology.
We’re thinking: Mistral’s new models underscore the growing relevance of edge computing to AI’s future. They could prove to be affordable and adaptable alternatives to Apple and Google’s built-in models on smartphones and laptops.
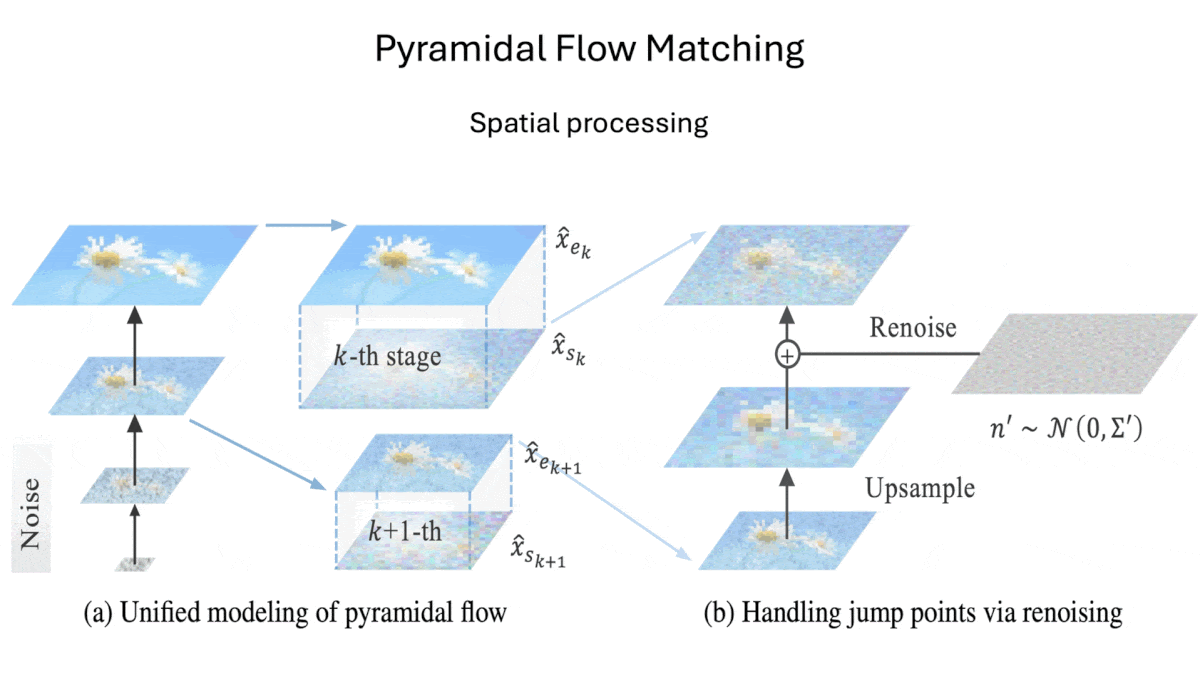
Faster, Cheaper Video Generation
Researchers devised a way to cut the cost of training video generators. They used it to build a competitive open source text-to-video model and promised to release the training code.
What’s new: Yang Jin and colleagues at Peking University, Kuaishou Technology, and Beijing University of Posts and Telecommunications proposed Pyramidal Flow Matching, a method that reduced the amount of processing required to train video generators. They offer the code and a pretrained model that’s free for noncommercial uses and for commercial uses by developers who make less than $1 million in annual revenue.
Key insight: Models that generate output by starting with noise and removing it over several steps, such as diffusion and flow matching models, typically learn by removing noise from an embedding to which noise was added. Starting with a downsampled (smaller) version of the embedding and then upsampling (enlarging) it gradually throughout the process, hitting the full size near the end, saves processing during training and inference.
How it works: The authors’ system comprises a pretrained SD3 Medium image generator, an image autoencoder, and two pretrained text encoders: T5 and CLIP. They pretrained the autoencoder to reconstruct images and sequences of video frames, and trained SD3 Medium to remove noise from an embedding of eight video frames given both text embeddings and embeddings of previous sequences of frames. The training sets included WebVid-10M, OpenVid-1M, and Open-Sora Plan. The authors modified the typical process of removing noise from image embeddings in two ways: spatially and temporally.
- Spatially: Given an embedding of eight video frames, SD3 Medium starts by removing noise on a heavily downsampled (very small) version of the embedding. After a number of noise-removal steps, the system increases the embedding size and adds further noise. It repeats these steps until SD3 is finished removing noise from the full-size embedding.
- Temporally: When it’s removing noise from an embedding of eight frames, SD3 Medium receives downsampled versions of the previous embeddings it has generated. These embeddings start at the size of the current embedding and get progressively smaller for earlier frames. (They’re progressively smaller because the further they are from the current embedding, the less closely related they are to the current embedding.)
- At inference: Given a prompt, T5 and CLIP produce text embeddings. Given the text embeddings, an embedding of pure noise, and previously denoised embeddings, SD3 Medium removes noise. Given the denoised embeddings from SD3 Medium, the autoencoder’s decoder turns them into a video.
Results: The authors compared their model to other open and closed models using VBench, a suite of benchmarks for comparing the quality of generated video. They also conducted a survey of human preferences. On VBench, their model outperformed other open models but slightly underperformed the best proprietary models, such as Kling. Human evaluators rated their model as superior to Open-Sora 1.2 for esthetics, motion, and adherence to prompts, and better than Kling for esthetics and adherence to prompts (but not motion). Furthermore, running on an Nvidia A100 GPU, their model took 20,700 hours to learn to generate videos up to 241 frames long. Running on a faster Nvidia H100 GPU, Open-Sora 1.2 took 37,800 hours to learn to generate 97 frames.
Why it matters: Video generation is a burgeoning field that consumes enormous amounts of processing. A simple way to reduce processing could help it scale to more users.
We’re thinking: Hollywood is interested in video generation. Studios reportedly are considering using the technology in pre- and post-production. Innovations that make it more compute-efficient will bring it closer to production.