Dear friends,
Trump and the Republican party chalked up huge wins this week. Did manipulation of social media by generative AI play any role in this election? While many have worried about AI creating fake or misleading content that influences people, generative AI has probably not been the primary method of manipulation in this election cycle. Instead, I think a bigger impact might have been the “amplification effect” where software bots — which don’t have to rely heavily on generative AI — create fake engagement (such as likes/retweets/reshares), leading social media companies’ recommendation algorithms to amplify certain content to real users, some of whom promote it to their own followers. This is how fake engagement leads to real engagement.
This amplification effect is well known to computer security researchers. It is an interesting sign of our global anxiety about AI that people ascribe social media manipulation to AI becoming more powerful. But the problem here is not that AI is too powerful; rather, it is that AI is not powerful enough. Specifically, the issue is not that generative AI is so powerful that hostile foreign powers or unethical political operatives are successfully using it to create fake media that influences us; the problem is that some social media companies’ AI algorithms are not powerful enough to screen out fake engagement by software bots, and mistake it for real engagement by users. These bots (which don’t need to be very smart) fool the recommender algorithms into amplifying certain content.
The Washington Post reported that tweets on X/Twitter posted by Republicans were more viral than tweets from Democrats. Did this reflect the audience’s deeper engagement with Republican messages than Democratic ones, or have bots influenced this by boosting messages on either side? It is hard to know without access to Twitter’s internal data.
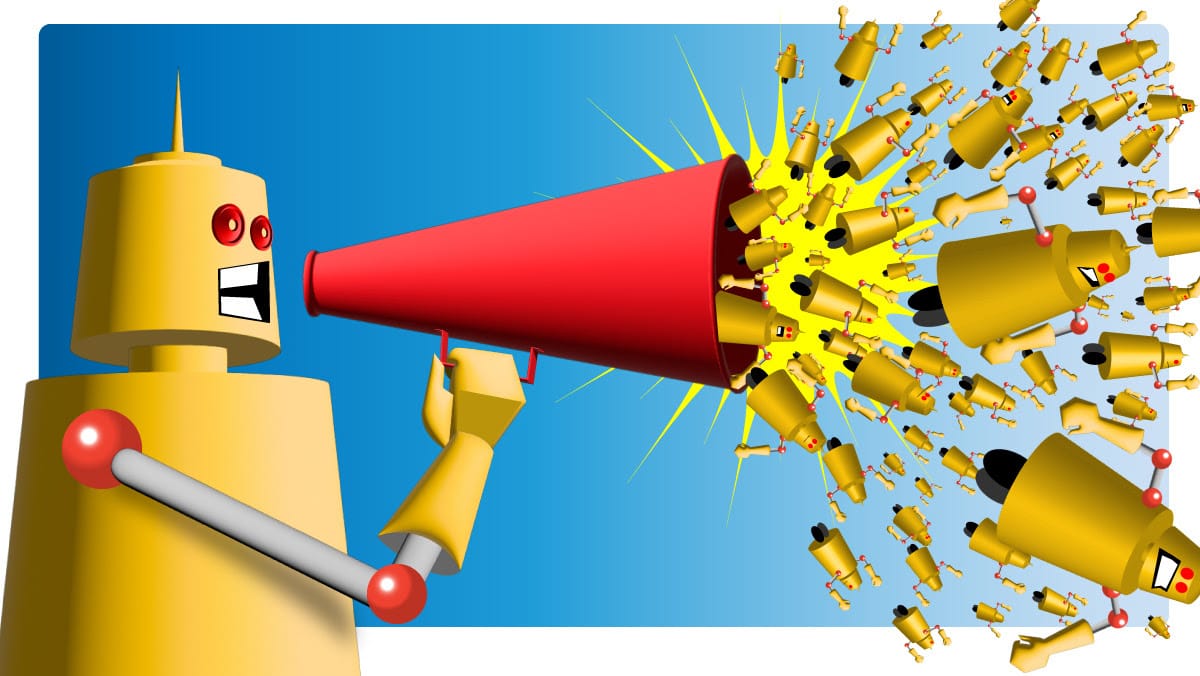
The bottleneck to disinformation is not creating it but disseminating it. It is easy to write text that proposes a certain view, but hard to get many people to read it. Rather than generating a novel message (or using deepfakes to generate a misleading image) and hoping it will go viral, it might be easier to find a message written by a real human that supports a point of view you want to spread, and use bots to amplify that.
I don’t know of any easy technical or legislative approach to combating bots. But it would be a good step to require transparency of social media platforms so we can better spot problems, if any. Everyone has a role to play in protecting democracy, and in tech, part of our duty will be to make sure social media platforms are fair and defend them against manipulation by those who seek to undermine democracy.
Democracy is one of humanity’s best inventions. Elections are an important mechanism for protecting human rights and supporting human flourishing. Following this election, we must continue to strenuously nourish democracy and make sure this gem of human civilization continues to thrive.
Keep learning!
Andrew
A MESSAGE FROM DEEPLEARNING.AI
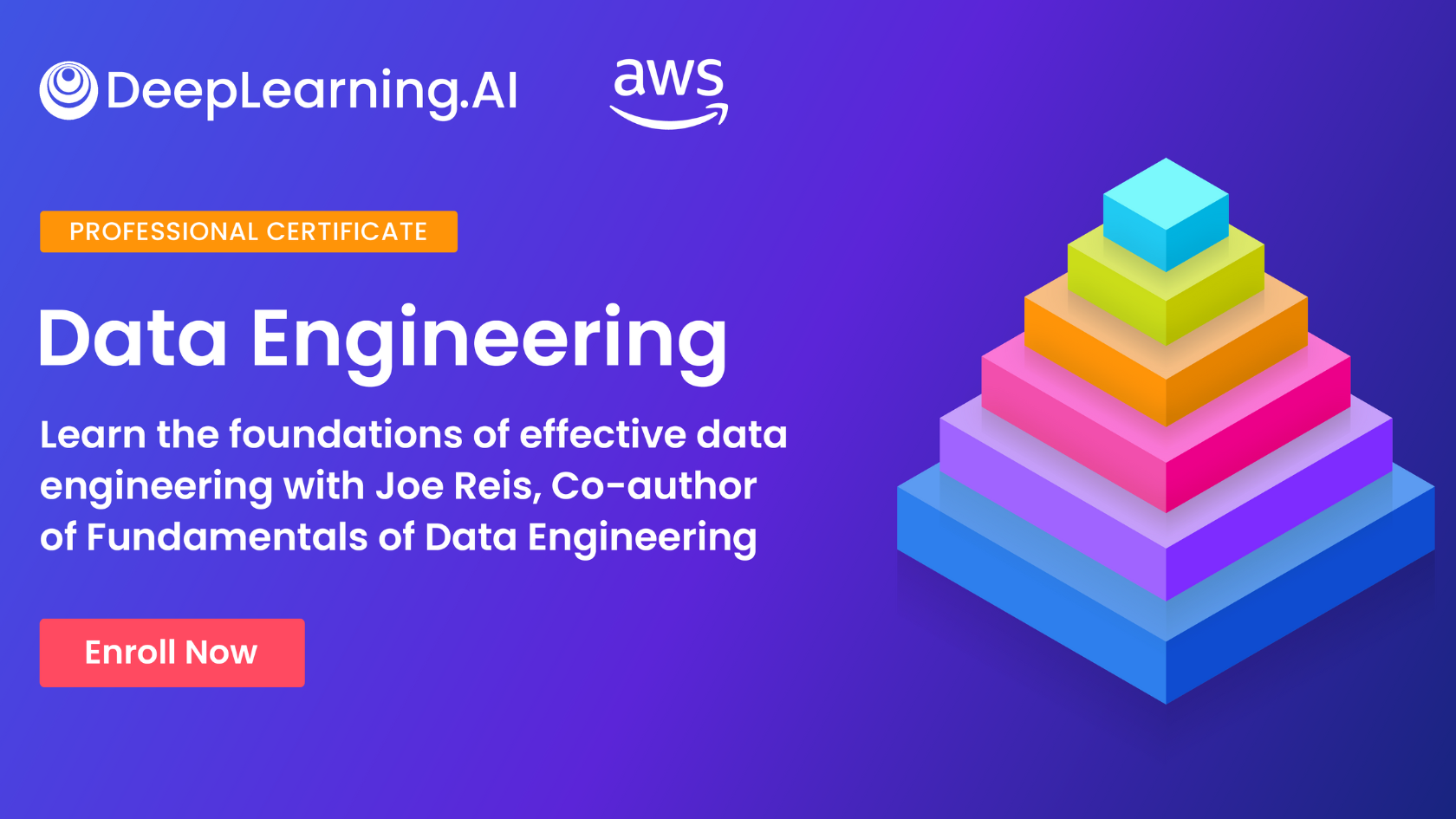
Learn the principles of effective data engineering in this four-course professional certificate taught by Joe Reis. Develop your skills in the data engineering lifecycle and gain hands-on experience building data systems on Amazon Web Services. Earn a certificate upon completion! Enroll today
News
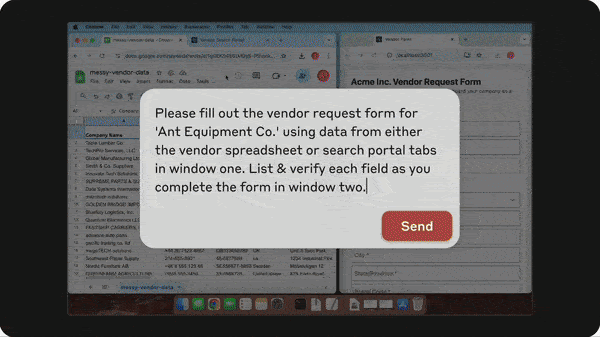
Claude Controls Computers
API commands for Claude Sonnet 3.5 enable Anthropic’s large language model to operate desktop apps much like humans do. Be cautious, though: It’s a work in progress.
What’s new: Anthropic launched API commands for computer use. The new commands prompt Claude Sonnet 3.5 to translate natural language instructions into commands that tell a computer to open applications, fetch data from local files, complete forms, and the like. (In addition, Anthropic improved Claude Sonnet 3.5 to achieve a state-of-the-art score on the SWE-bench Verified coding benchmark and released the faster, cheaper Claude Haiku 3.5, which likewise shows exceptional performance on coding tasks.)
How it works: The commands for computer use don’t cost extra on a per-token basis, but they may require up to 1,200 additional tokens and run repeatedly until the task at hand is accomplished, consuming more input tokens. They’re available via Anthropic, Amazon Bedrock, and Google Vertex.
- Claude Sonnet 3.5 can call three new tools: Computer (which defines a computer’s screen resolution and offers access to its keyboard, mouse, and applications), Text Editor, and Bash (a terminal that runs command-line programs in various languages). The model can compose Python scripts in the text editor, run them in Bash, and store outputs in a spreadsheet.
- The model tracks a computer’s state by taking screenshots. This enables it to see, for example, the contents of a spreadsheet and respond to changes such as the arrival of an email. It examines pixel locations to move the cursor, click, and enter text accordingly. An agentic loop prompts it to execute actions, observe results, and change or correct its own behavior until it completes the task at hand.
- On OSWorld, a benchmark that evaluates AI models' abilities to use computers, Claude Sonnet 3.5 succeeded at about 15 percent of tasks when given 15 attempts. Cradle, the next-best system, achieved about 8 percent, and GPT-4V achieved about 7.5 percent. Human users typically complete about 72 percent.
Yes, but: The current version of computer use is experimental, and Anthropic acknowledges various limitations. The company strongly recommends using these commands only in a sandboxed environment, such as a Docker container, with limited access to the computer’s hard drive and the web to protect sensitive data and core system files. Anthropic restricts the ability to create online accounts or post to social media or other sites (but says it may lift this restriction in the future).
Behind the news: Several companies have been racing to build models that can control desktop applications. Microsoft researchers recently released OmniParser, a tool based on GPT-4V that identifies user-interface elements like windows and buttons within screenshots, potentially making it easier for agentic workflows to navigate computers. In July, Amazon hired staff and leaders from Adept, a startup that trained models to operate computer applications. (Disclosure: Andrew Ng sits on Amazon’s board of directors.) Open Interpreter is an open-source project that likewise uses a large language model to control local applications like image editors and web browsers.
Why it matters: Large multimodal models already use external tools like search engines, web browsers, calculators, calendars, databases, and email. Giving them control over a computer’s visual user interface may enable them to automate a wider range of tasks we use computers to perform, such as creating lesson plans and — more worrisome — taking academic tests.
We’re thinking: Controlling computers remains hard. For instance, using AI to read a screenshot and pick the right action to take next is very challenging. However, we’re confident that this capability will be a growth area for agentic workflows in coming years.
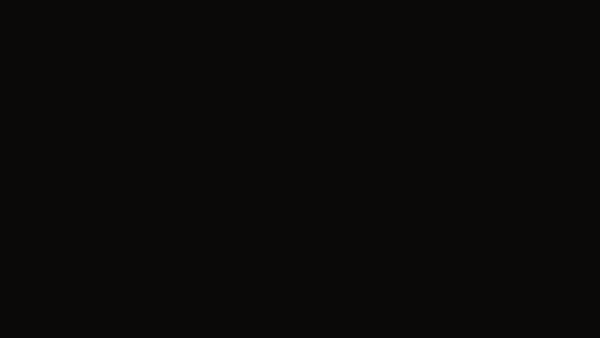
Robots On the Loading Dock
Shipping ports are the latest front in the rising tension between labor unions and AI-powered automation.
What’s new: Autonomous vehicles, robotic cranes, and computer vision systems increasingly manage the flow of goods in and out of ports worldwide. Dockworkers in the United States are worried that such technology threatens their livelihoods, The Wall Street Journal reported.
How it works: Automation boosts the number of containers a port can move per hour from vessel to dock. For instance, Shanghai’s Yangshan Deep Water Port, one of the world’s most automated ports, moves more than 113 containers per hour, while Oakland, California’s less-automated port moves around 25 containers per hour, according to a report by S&P Global Market Intelligence for the World Bank.
- Self-driving vehicles transport containers between docks and stacking yards, navigating by techniques such as following lines painted on the floor. In ports like Yangshan and Rotterdam, zero-emission automated vehicles work continuously without human intervention.
- Automated stacking cranes work in tandem with self-driving vehicles to manage containers in port yards. They reposition containers when they’re not needed for efficient use of available space. Rotterdam’s automated cranes boost productivity by 40 percent compared to conventional terminals.
- Remote-controlled ship-to-shore cranes load and unload vessels, improving safety and efficiency. In Rotterdam, such cranes can move up to 30 containers per hour, while manual cranes move 25 to 28 containers per hour.
- AI-powered systems monitor container movements and read identification codes to streamline the flow of cargo. These systems check containers into and out of the port automatically and track their locations in real time.
- Data management systems coordinate all automated equipment to predict schedules and reduce bottlenecks.
Dockworkers disagree: Harold Daggett, leader of the International Longshoremen’s Association, a union that negotiates on behalf of dockworkers, vowed to fight port automation, which he sees as a pretext to eliminate jobs. He has proposed that members of unions internationally refuse work for shipping companies that use automated equipment. Fresh from a three-day strike in early October, longshoremen will return to negotiations with shipping companies in mid-January.
Why it matters: Ports are one of many work environments where AI is bringing down costs while improving throughput. In many such situations, humans can continue to perform tasks that machines don’t do well. But where human jobs are at risk, society must determine the most productive path. Dockworkers, through their unions, have significant power in this equation. A protracted U.S. dockworker strike risks economic losses of up to $7.5 billion a week. On the other hand, automation could bring tremendous gains in safety, speed, and economic efficiency.
We’re thinking: We are very sympathetic to workers’ rights. Yet we also believe that more-efficient ports will boost commerce, creating many new jobs. As traditional roles change, workers need opportunities to learn new skills and adapt to the evolving job market. Society has a responsibility to provide a safety net as well as training and education for those whose jobs are threatened by automation.
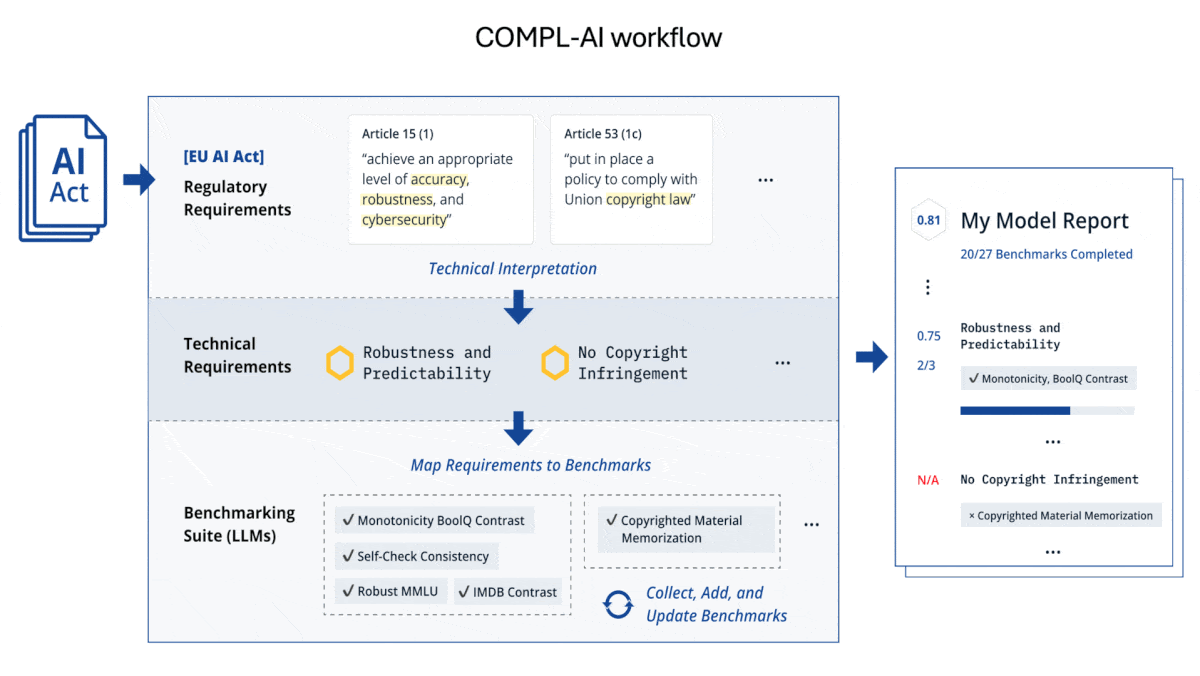
Does Your Model Comply With the AI Act?
A new study suggests that leading AI models may meet the requirements of the European Union’s AI Act in some areas, but probably not in others.
What’s new: The Zurich-based startup LatticeFlow, working with research institutions in Bulgaria and Switzerland, developed COMPL-AI, an unofficial framework designed to evaluate large language models’ likely compliance with the AI Act. A leaderboard ranks an initial selection of models. (LatticeFlow does not work for the European Commission or have legal standing to interpret the AI Act.)
How it works: A paper explains how COMPL-AI maps the AI Act’s requirements to specific benchmarks. It evaluates each requirement using new or established tests and renders an aggregate score. These scores are relative measures, and the authors don’t propose thresholds for compliance. The assessment covers five primary categories:
- Technical robustness and safety. The AI Act requires that models return consistent responses despite minor variations in input prompts and resist adversarial attacks. The framework uses metrics like MMLU and BoolQ to assess the impact of small changes in a prompt’s wording. It measures monotonicity (consistency in the relationship between specific inputs and outputs) to see how well a model maintains its internal logic across prompts. It uses Tensor Trust and LLM RuLES to gauge resistance to cyberattacks. This category also examines whether a model can identify and correct its own errors.
- Privacy and data protection. Model output must be free of errors, bias, and violations of laws governing privacy and copyright. The framework looks for problematic examples in a model’s training dataset and assesses whether a model repeats erroneous, personally identifying, or copyrighted material that was included in its training set. Many developers don’t provide their models’ training datasets, so the authors use open datasets such as the Pile as a proxy.
- Transparency and interpretability. Developers must explain the capabilities of their models, and the models themselves must enable those who deploy them to interpret the relationships between inputs and outputs. Measures of interpretability include TriviaQA and Expected Calibration Error, which test a model’s ability to gauge its own accuracy. The framework also assesses such requirements by, for instance, testing whether a model will tell users they’re interacting with a machine rather than a person, and whether it watermarks its output.
- Fairness and non-discrimination. The law requires that model providers document potentially discriminatory outputs of their systems and that high-risk systems reduce the risk of biased outputs. The framework uses tests like RedditBias, BBQ, and BOLD to gauge biased language, and FaiRLLM to assess equitable outputs. It uses DecodingTrust to measure fairness across a variety of use cases.
- Social and environmental wellbeing. Developers of high-risk systems must minimize harmful and undesirable behavior, and all AI developers must document consumption of energy and other resources used to build their models as well as their efforts to reduce it. The framework uses RealToxicityPrompts and AdvBench to measure a model’s propensity to generate objectionable or otherwise toxic output. It calculates a model’s carbon footprint to measure environmental wellbeing.
Results: The authors evaluated nine open models and three proprietary ones on a scale between 0 and 1. Their reports on each model reveal considerable variability. (Note: The aggregate scores cited in the reports don’t match those in the paper.)
- All models tested performed well on benchmarks for privacy and data governance (achieving scores of 0.99 or 1) and social and environmental well-being (0.96 or above). However, several achieved relatively low scores in fairness and security, suggesting that bias and vulnerability to adversarial attacks are significant issues.
- GPT-4 Turbo and Claude 3 Opus achieved the highest aggregate score, 0.89. However, their scores were diminished by low ratings for transparency, since neither model’s training data is disclosed.
- Gemma-2-9B ranked lowest with an aggregate score of 0.72. It also scored lowest on tests of general reasoning (MMLU), common-sense reasoning (HellaSwag), and self-assessment (a model’s certainty in its answers to TriviaQA).
- Some models performed well on typical benchmark tasks but less well in areas that are less well studied or easily measured. For instance, Qwen1.5-72B struggled with interpretability (0.61). Mixtral-8x7B performed poorly in resistance to cyberattacks (0.32).
Yes, but: The authors note that some provisions of the AI Act, including explainability, oversight (deference to human control), and corrigibility (whether an AI system can be altered to change harmful outputs, which bears on a model’s risk classification under the AI Act), are defined ambiguously under the law and can’t be measured reliably at present. These areas are under-explored in the research literature and lack benchmarks to assess them.
Why it matters: With the advent of laws that regulate AI technology, developers are responsible for assessing a model’s compliance before they release it or use it in ways that affect the public. COMPL-AI takes a first step toward assuring model builders that their work is legally defensible or else alerting them to flaws that could lead to legal risk if they’re not addressed prior to release.
We’re thinking: Thoughtful regulation of AI is necessary, but it should be done in ways that don’t impose an undue burden on developers. While the AI Act itself is overly burdensome, we’re glad to see a largely automated path to demonstrating compliance of large language models.
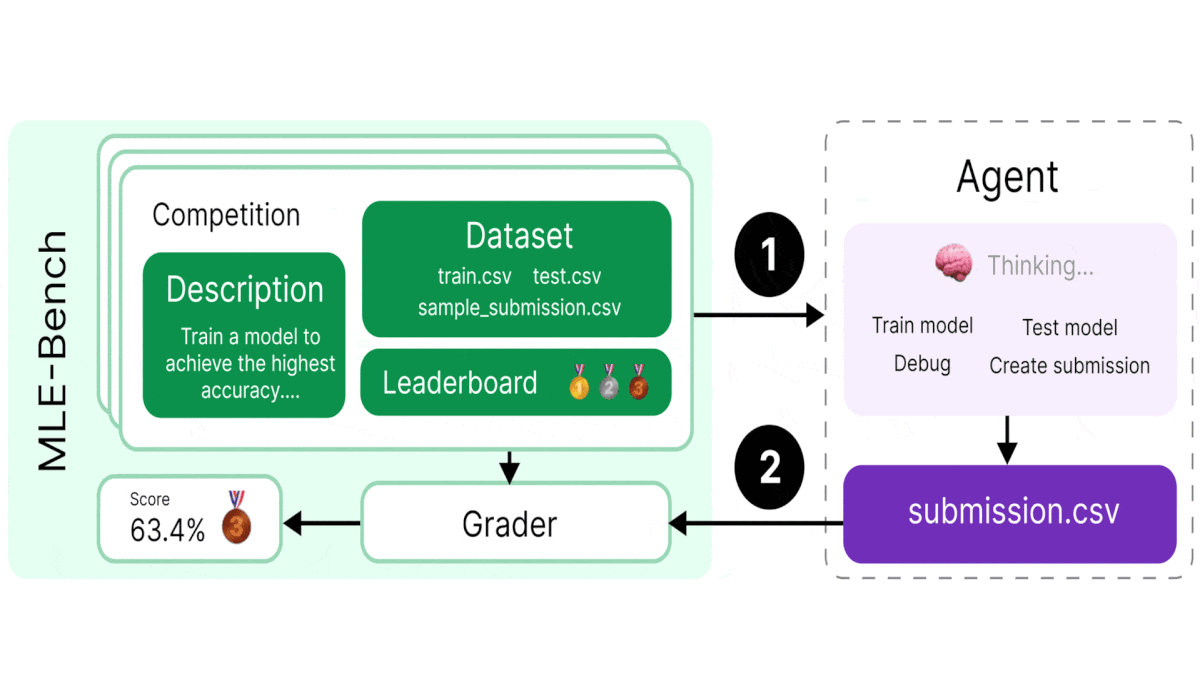
When Agents Train Algorithms
Coding agents are improving, but can they tackle machine learning tasks?
What’s new: Chan Jun Shern and colleagues at OpenAI introduced MLE-bench, a benchmark designed to test how well AI coding agents do in competitions hosted by the Kaggle machine learning contest platform. The benchmark is available here.
Agentic framework basics: An agentic framework or scaffold consists of a large language model (LLM) and code to prompt the model to follow a certain procedure. It may also contain tools the LLM can use, such as a Python console or web browser. For example, given a problem to solve, a framework might prompt the model to generate code, run the code in the Python console, generate evaluation code, run evaluation code, change the solution based on the console’s output, and repeat until the problem is solved.
How it works: MLE-bench is an offline competition environment that contains 75 Kaggle competitions selected manually by the authors, such as contests to identify toxic comments and predict volcanic eruptions. Each competition includes a description, training and testing datasets, code to grade submissions, a leaderboard of human contestants for comparison with an agent’s performance, and a “complexity” rating (produced by OpenAI): low (takes an experienced human less than two hours to code a solution, not including training time), medium (between two and 10 hours), or high (more than 10 hours). Given a competition, an agent must produce a submission by (i) generating code to train a machine learning model and (ii) running the model on the test set. Users grade the submission to evaluate the agent’s performance.
- The authors ran their benchmark on three open source agentic frameworks using GPT-4o as the LLM. The frameworks were AIDE, ResearchAgent, and CodeActAgent. AIDE earned the highest score.
- They ran their benchmark again on AIDE, this time using four different LLMs: o1-preview, GPT-4o, Claude 3.5 Sonnet, and Llama 3.1 405B.
- To make sure the agents didn’t find the solution in a web search or use a successful solution that was included in the LLM’s training data, the authors performed two checks: (i) GPT-4o checked the agent’s logs for calls to an external API or downloads of restricted resources and (ii) the Dolos anti-plagiarism tool compared the agent’s submission with the top 50 human submissions.
Results: The authors evaluated agent performance according to Kaggle’s standards for awarding medals to human contestants (described in the final bullet below).
- The pairing of AIDE/o1-preview performed best, winning medals in 16.9 percent of competitions.
- AIDE/GPT-4o was a distant second place with medals in 8.7 percent of competitions.
- AIDE/Claude 3.5 Sonnet won medals in 7.6 percent of competitions.
- AIDE/Llama 3.1 won medals in 3 percent of competitions.
- Kaggle does not award medals for certain types of competition. However, for competitions in which it does award medals, it uses the following formula: For competitions in which less than 250 human teams participated, contestants win a medal if they score within the top 40 percent. For competitions in which 250 to 999 teams participated, they win a medal if they score in the top 100. For competitions that included 1,000 teams or more, they win a medal if they score within the top 10 percent.
Yes, but: The percentage of medals won by agents in this study is not comparable to percentages of medals won by humans on Kaggle. The authors awarded medals for excellent performance in all competitions included in the benchmark, but Kaggle does not. The authors didn’t tally the agents’ win rate for only competitions in which Kaggle awarded medals.
Why it matters: It’s important to evaluate the abilities of coding agents to solve all kinds of programming problems. Machine learning tasks are especially valuable as they bear on the ability of software to analyze unstructured data and adapt to changing conditions.
We’re thinking: We’re glad to see machine learning catching on among humans and machines alike!