Dear friends,
At the Artificial Intelligence Action Summit in Paris this week, U.S. Vice President J.D. Vance said, “I’m not here to talk about AI safety. ... I’m here to talk about AI opportunity.” I’m thrilled to see the U.S. government focus on opportunities in AI. Further, while it is important to use AI responsibly and try to stamp out harmful applications, I feel “AI safety” is not the right terminology for addressing this important problem. Language shapes thought, so using the right words is important. I’d rather talk about “responsible AI” than “AI safety.” Let me explain.
First, there are clearly harmful applications of AI, such as non-consensual deepfake porn (which creates sexually explicit images of real people without their consent), the use of AI in misinformation, potentially unsafe medical diagnoses, addictive applications, and so on. We definitely want to stamp these out! There are many ways to apply AI in harmful or irresponsible ways, and we should discourage and prevent such uses.
However, the concept of “AI safety” tries to make AI — as a technology — safe, rather than making safe applications of it. Consider the similar, obviously flawed notion of “laptop safety.” There are great ways to use a laptop and many irresponsible ways, but I don’t consider laptops to be intrinsically either safe or unsafe. It is the application, or usage, that determines if a laptop is safe. Similarly, AI, a general-purpose technology with numerous applications, is neither safe nor unsafe. How someone chooses to use it determines whether it is harmful or beneficial.
Now, safety isn’t always a function only of how something is used. An unsafe airplane is one that, even in the hands of an attentive and skilled pilot, has a large chance of mishap. So we definitely should strive to build safe airplanes (and make sure they are operated responsibly)! The risk factors are associated with the construction of the aircraft rather than merely its application. Similarly, we want safe automobiles, blenders, dialysis machines, food, buildings, power plants, and much more.
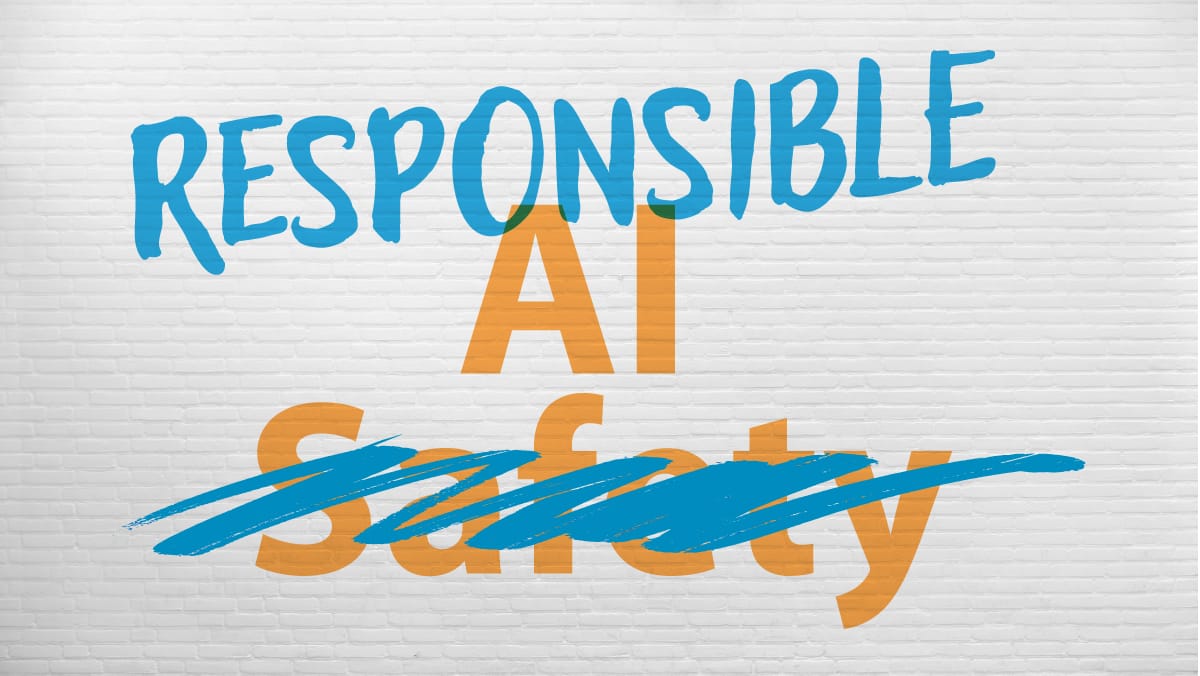
“AI safety” presupposes that AI, the underlying technology, can be unsafe. I find it more useful to think about how applications of AI can be unsafe.
Further, the term “responsible AI” emphasizes that it is our responsibility to avoid building applications that are unsafe or harmful and to discourage people from using even beneficial products in harmful ways.
If we shift the terminology for AI risks from “AI safety” to “responsible AI,” we can have more thoughtful conversations about what to do and what not to do.
I believe the 2023 Bletchley AI Safety Summit slowed down European AI development — without making anyone safer — by wasting time considering science-fiction AI fears rather than focusing on opportunities. Last month, at Davos, business and policy leaders also had strong concerns about whether Europe can dig itself out of the current regulatory morass and focus on building with AI. I am hopeful that the Paris meeting, unlike the one at Bletchley, will result in acceleration rather than deceleration.
In a world where AI is becoming pervasive, if we can shift the conversation away from “AI safety” toward responsible [use of] AI, we will speed up AI’s benefits and do a better job of addressing actual problems. That will actually make people safer.
Keep building!
Andrew
A MESSAGE FROM DEEPLEARNING.AI
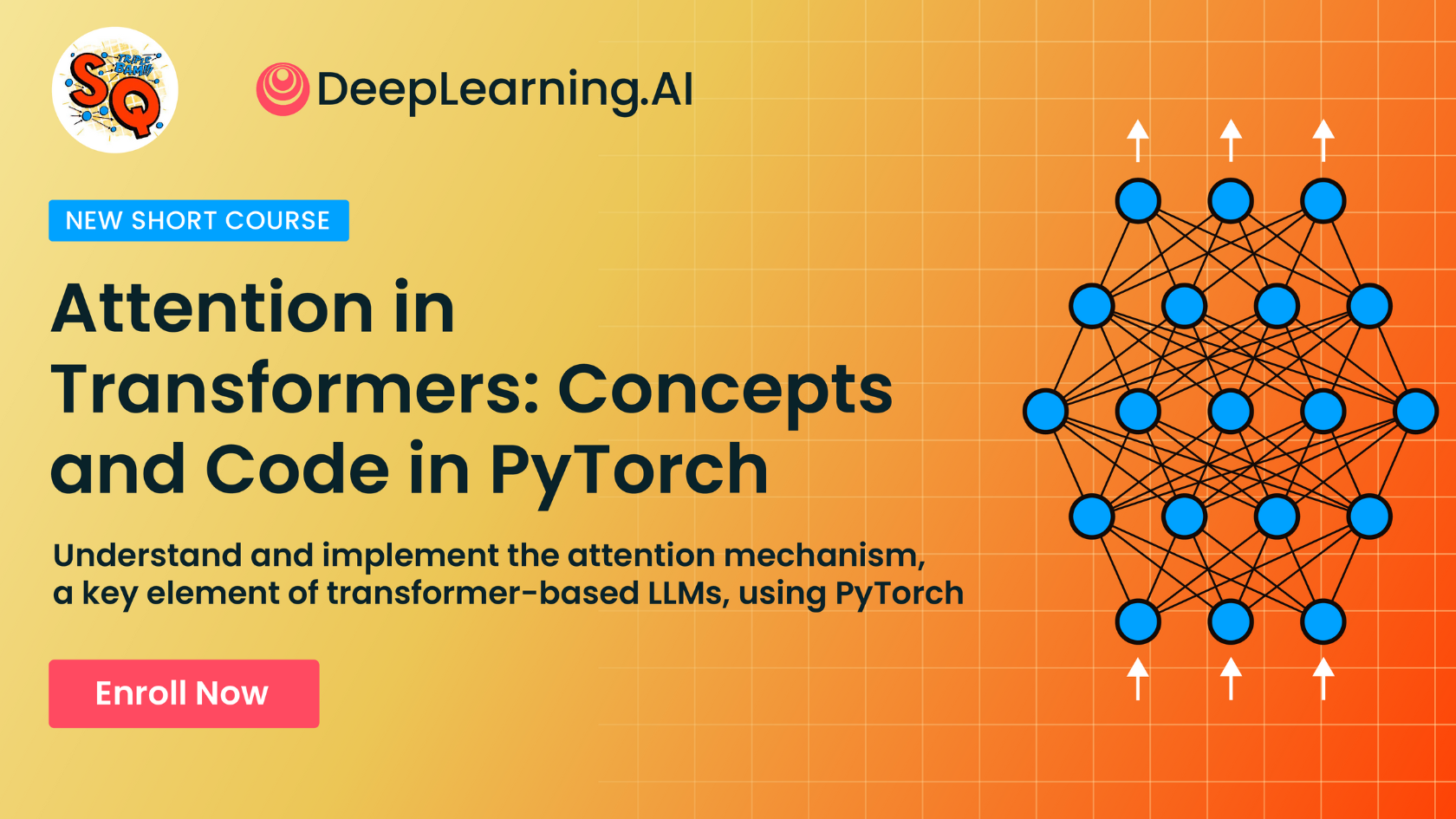
Understand and implement the attention mechanism, a key element in transformer-based LLMs, using PyTorch. In this course, StatQuest’s Josh Starmer explains the core ideas behind attention mechanisms, the algorithm itself, and a step-by-step breakdown of how to implement them in PyTorch. Enroll now
News
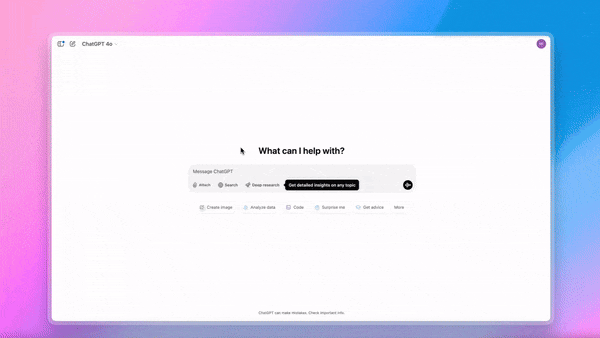
Agents Go Deep
OpenAI introduced a state-of-the-art agent that produces research reports by scouring the web and reasoning over what it finds.
What’s new: OpenAI’s deep research responds to users’ requests by generating a detailed report based on hundreds of online sources. The system generates text output, with images and other media expected soon. Currently the agent is available only to subscribers to ChatGPT Pro, but the company plans to roll it out to users of ChatGPT Plus, Team, and Enterprise.
How it works: Deep research is an agent that uses OpenAI’s o3 model, which is not yet publicly available. The model was trained via reinforcement learning to use a browser and Python tools, similar to the way o1 learned to reason from reinforcement learning. OpenAI has not yet released detailed information about how it built the system.
- The system responds best to detailed prompts that specify the desired output (such as the desired information, comparisons, and format), the team said in its announcement video (which features Mark Chen, Josh Tobin, Neel Ajjarapu, and Isa Fulford, co-instructor of our short courses “ChatGPT Prompt Engineering for Developers” and “Building Systems with the ChatGPT API”).
- Before answering, Deep research asks clarifying questions about the task.
- In the process of answering, the system presents a sidebar that summarizes the model’s chain of thought, terms it searched, websites it visited, and so on.
- The system can take as long as 30 minutes to provide output.
Result: On a benchmark of 3,000 multiple-choice and short-answer questions that cover subjects from ecology to rocket science, OpenAI deep research achieved 26.6 percent accuracy. In comparison, DeepSeek-R1 (without web browsing or other tool use) achieved 9.4 percent accuracy and o1 (also without tool use) achieved 9.1 percent accuracy. On GAIA, questions that are designed to be difficult for large language models without access to additional tools, OpenAI deep research achieved 67.36 percent accuracy, exceeding the previous state of the art of 63.64 percent accuracy.
Behind the news: OpenAI’s deep research follows a similar offering of the same name by Google in December. A number of open source teams have built research agents that work in similar ways. Notable releases include a Hugging Face project that attempted to replicate OpenAI’s work (not including training) in 24 hours (which achieved 55.15 percent accuracy on GAIA) and gpt-researcher, which implemented agentic web search in 2023, long before Google and OpenAI launched their agentic research systems.
Why it matters: Reasoning models like o1 or o3 made a splash not just because they delivered superior results but also because of the impressive reasoning steps the model took to produce the results. Combining that ability with web search and tool use enables large language models to formulate better answers to difficult questions, including those whose answers aren’t in the training data or whose answers change over time.
We’re thinking: Taking as much as 30 minutes of processing to render a response, OpenAI’s deep research clearly illustrates why we need more compute for inference.
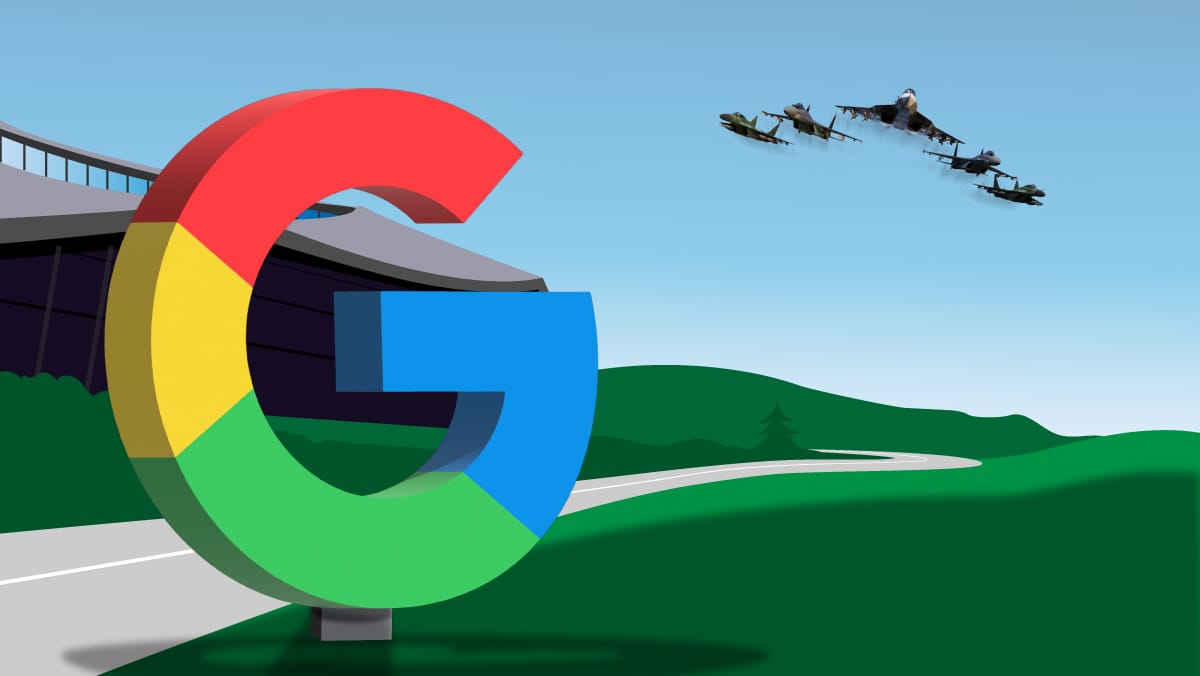
Google Joins AI Peers In Military Work
Google revised its AI principles, reversing previous commitments to avoid work on weapons, surveillance, and other military applications beyond non-lethal uses like communications, logistics, and medicine.
What’s new: Along with releasing its latest Responsible AI Progress Report and an updated AI safety framework, Google removed key restrictions from its AI principles. The new version omits a section in the previous document titled “Applications we will not pursue.” The deleted text pledged to avoid “technologies that cause or are likely to cause overall harm” and, where the technology risks doing harm, to “proceed only where we believe that the benefits substantially outweigh the risks” with “appropriate safety constraints.”
How it works: Google’s AI principles no longer prohibit specific applications but promote developing the technology to improve scientific inquiry, national security, and the economy.
- The revised principles state that AI development should be led by democracies. The company argues that such leadership is needed given growing global competition in AI from countries that are not widely considered liberal democracies.
- The new principles stress “responsible development and deployment” to manage AI’s complexities and risks. They state that AI must be developed with safeguards at every stage, from design and testing to deployment and iteration, and those safeguards must adapt as technology and applications evolve.
- The revised principles also emphasize collaborative progress, stating that Google aims to learn from others and build AI that’s broadly useful across industries and society.
- Google emphasizes the need for “bold innovation,” stating that AI should be developed to assist, empower, and inspire people; drive economic progress; enable scientific breakthroughs; and help address global challenges. Examples include AlphaFold 3, which figures out how biological molecules interact, a key factor in designing chemical processes that affect them.
- The revised principles are buttressed by the 2025 Responsible AI Progress Report. This document outlines the company’s efforts to evaluate risks through measures that align with the NIST AI Risk Management Framework including red teaming, automated assessments, and input from independent experts.
Behind the news: Google’s new stance reverses a commitment it made in 2018 after employees protested its involvement in Project Maven, a Pentagon AI program for drone surveillance, from which Google ultimately withdrew. At the time, Google pledged not to develop AI applications for weapons or surveillance, which set it apart from Amazon and Microsoft. Since then, the company has expanded its work in defense, building on a $1.3 billion contract with Israel. In 2024, Anthropic, Meta, and OpenAI removed their restrictions on military and defense applications, and Anthropic and OpenAI strengthened their ties with defense contractors such as Anduril and Palantir.
Why it matters: Google’s shift in policy comes as AI is playing an increasing role in conflicts in Israel, Ukraine, and elsewhere, and while global geopolitical tensions are on the rise. While Google’s previous position kept it out of military AI development, defense contractors like Anduril, Northrop Grumman, and Palantir — not to mention AI-giant peers — stepped in. The new principles recognize the need for democratic countries to take the lead in developing technology and standards for its use as well as the massive business opportunity in military AI as governments worldwide seek new defense capabilities. Still, no widely accepted global framework governs uses of AI in combat.
We’re thinking: Knowing how and when to employ AI in warfare is one of the most difficult ethical questions of our time. Democratic nations have a right to defend themselves, and those of us who live in democracies have a responsibility to support fellow citizens who would put themselves in harm’s way to protect us. AI is transforming military strategy, and refusing to engage with it doesn’t make the risks go away.
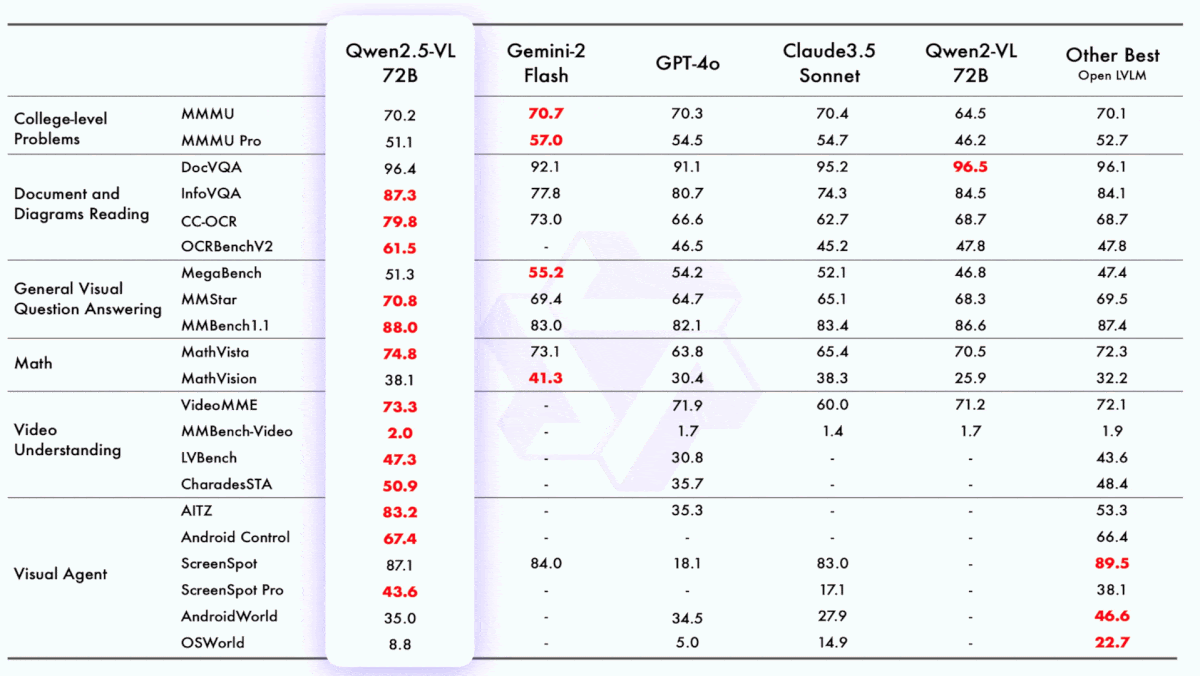
Alibaba’s Answer to DeepSeek
While Hangzhou’s DeepSeek flexed its muscles, Chinese tech giant Alibaba vied for the spotlight with new open vision-language models.
What’s new: Alibaba announced Qwen2.5-VL, a family of vision-language models (images and text in, text out) in sizes of 3 billion, 7 billion, and 72 billion parameters. The weights for all three models are available for download on Hugging Face, each under a different license: Qwen2.5-VL-3B is free for non-commercial uses, Qwen2.5-VL-7B is free for commercial and noncommercial uses under the Apache 2.0 license, and Qwen2.5-VL-72B is free to developers that have less than 100 million monthly active users. You can try them out for free for a limited time in Alibaba Model Studio, and Qwen2.5-VL-72B is available via the model selector in Qwen Chat.
How it works: Qwen2.5-VL models accept up to 129,024 tokens of input according to the developer reference (other sources provide conflicting numbers) and generate up to 8,192 tokens of output. Alibaba has not released details about how it trained them.
- Qwen2.5-VL comprises a vision encoder and large language model. It can parse videos, images, text, and is capable of computer use (desktop and mobile).
- The vision encoder accepts images of different sizes and represents them with different numbers of tokens depending on the size. For instance, one image might be 8 tokens and another 1125 tokens. This enabled the model to learn about the scale of images and to estimate the coordinates of objects in an image without rescaling.
- To reduce computation incurred by the vision encoder, the team replaced attention (which considers the entire input context) with windowed attention (which limits the input context to a window around a given token) and used full attention only in four layers. The resulting efficiency improves training and inference speeds.
Results: Alibaba reports Qwen2.5-VL-72B’s performance on measures that span image and text problems, parsing documents, understanding videos, and interacting with computer programs. Across 21 benchmarks, it beat Microsoft Gemini 2.0 Flash, OpenAI GPT-4o, Anthropic Claude 3.5 Sonnet, and open competitors on 13 of them (where comparisons are relevant and available).
- For example, on answering math questions about images in MathVista, Qwen2.5-VL-72B achieved 74.8 percent, while the closest competing model (Gemini 2.0 Flash) achieved 73.1 percent.
- In Video-MME, which evaluates a model’s ability to answer questions about videos, Qwen 2.5 VL achieved 73.3 percent. GPT-4o achieved 71.9 percent and InternVL2.5, the next-best open competitor, achieved 72.1 percent.
- Used in an agentic workflow, Qwen2.5-VL-72B outperformed Claude 3.5 Sonnet when controlling Android devices and navigating desktop user interfaces. However, it finished second to other open vision-language models in several tests.
More models: Alibaba also introduced competition for DeepSeek and a family of small models.
- Qwen2.5-Max is a mixture-of-experts model that outperforms GPT-4o and DeepSeek-V3 on graduate-level science questions in GPQA-Diamond and regularly updated benchmarks like Arena-Hard, LiveBench, and LiveCodeBench. However, Qwen2.5-Max performed worse than o1 and DeepSeek-R1.
- Qwen2.5-1M is a family of smaller language models (7 billion and 14 billion parameters) that accept up to 1 million tokens of input context.
Why it matters: Vision-language models are getting more powerful and versatile. Not long ago, it was an impressive feat simply to answer questions about a chart or diagram that mixed graphics with text. Now such models are paired with an agent to control computers and smartphones. Broadly speaking, the Qwen2.5-VL models outperform open and closed competitors and they’re open to varying degrees (though the data is not available), giving developers a range of highly capable choices.
We’re thinking: We’re happy Alibaba released a vision-language model that is broadly permissive with respect to commercial use (although we’d prefer that all sizes were available under a standard open weights license). We hope to see technical reports that illuminate Alibaba’s training and fine-tuning recipes.
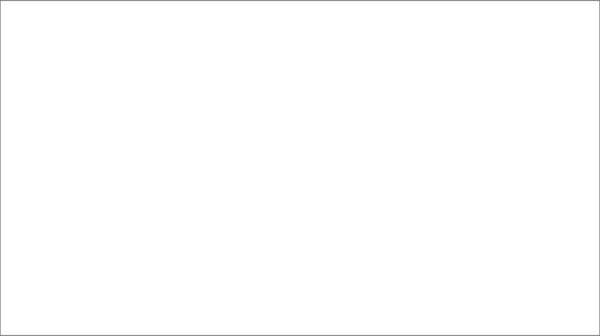
Tree Search for Web Agents
Browsing the web to achieve a specific goal can be challenging for agents based on large language models and even for vision-language models that can process onscreen images of a browser. While some approaches address this difficulty in training the underlying model, the agent architecture can also make a difference.
What’s new: Jing Yu Koh and colleagues at Carnegie Mellon University introduced tree search for language model agents, a method that allows agents to treat web interactions like tree searches. In this way, agents can explore possible chains of actions and avoid repeating mistakes.
Key insight: Some web tasks, for instance finding a price of a particular item, require a chain of intermediate actions: navigating to the right page, scrolling to find the item, matching an image of the item to the image on the page, and so on. If an agent clicks the wrong link during this process, it might lose its way. The ability to evaluate possible actions and remember previous states of web pages can help an agent correct its mistakes and choose a chain of actions that achieves its goal.
How it works: An agent based on GPT-4o attempted 200 tasks using website mockups that mimicked an online retail store, Reddit-like forum, and directory of classified ads. The tasks included ordering an item to be delivered to a given address, finding specific images on the forum, and posting an ad. The authors annotated each web page using the method called Set of Mark, which identifies every visual element capable of interaction with a bounding box and a numerical ID.
- The agent started with a web page and an instruction such as, “Tell me the number of reviews our store received that mention the term ‘not useful.’” It passed an image of the page to the LLM, which predicted five actions that could make progress toward completing the task such as scrolling up or down, hovering over an element, clicking, typing in a text field, or opening a new URL.
- The agent executed the five actions. After each one, the LLM assessed the current state of the page using the previous states as context. The assessment assigned a value between 0 and 1 (meaning the task was complete). The agent kept a list of page states and their values.
- The agent selected the web page state with the highest value after executing the five actions, and repeated the process, making a new set of five predictions based on the highest-value state.
- This process is a search: The agent executed a chain of actions until the value of the new states dropped below the values of other states. If all new states had lower values, the agent backtracked to a previous state with a higher value and asked the LLM for five more actions. The search stopped when the agent had completed the task or explored 20 possible states.
Results: The authors compared two agents, one that followed their search method and another that started at the same page and received the same instruction but took one action per state and never backtracked. The agents attempted 100 shopping tasks, 50 forum tasks, and 50 classified-ads tasks. The one equipped to search successfully completed 26.4 percent of the tasks, while the other agent completed 18.9 percent of the tasks.
Why it matters: Search joins reflection, planning, tool use, and multi-agent collaboration as an emerging agentic design pattern. Following many branching paths of actions enables an agent to determine the most effective set of actions to accomplish a task.
We’re thinking: Agentic design patterns are progressing quickly! In combination with computer use, this sort of search method may enable agents to execute a wide variety of desktop tasks.