Dear friends,
In the earlier weeks of Covid-19, I didn’t want to contribute noise, so that experts in infectious disease could be heard. But now the situation has worsened. I spoke yesterday with Eric Topol, a cardiologist at Scripps Institute and author of Deep Medicine: How Artificial Intelligence Can Make Healthcare Human Again. He convinced me that it’s urgent for all of us to speak up.
I’m deeply concerned about preventing Covid-19’s spread within healthcare systems. Apart from the widely reported shortages of personal protective equipment, healthcare systems in most countries, including the U.S., are not set up to adequately protect doctors and nurses from infection. We need to prioritize healthcare workers’ safety if we want them to keep taking care of us — and so that the death toll estimates, which are already staggering, don’t become even worse.
Here are some projects that my teams and I have been up to:
- Sourcing best practices from different countries that have more experience with Covid-19 and SARS. We organized this webinar to inform U.S. doctors of South Korea’s best practices.
- Together with several colleagues, compiling such best practices into concrete, practical suggestions like segmenting hospitals according to risk, with appropriate protocols for high-, medium-, and low-risk zones.
- Shipping masks to the U.S. from abroad and donating them to local hospitals. Our first shipment just arrived, and more are on the way.
It’s urgent for all of us to do what we can to flatten the curve. There are many things you can do to help. I hope that each of us will:
- Practice social distancing. Stay at home if you can, and encourage others to do the same.
- Support wearing masks by both healthcare workers and private citizens.
- Make local contributions, from offering to buy groceries for a neighbor to simply voicing your appreciation for the healthcare workers who are treating people nearby.
It’s up to us to respect the quarantine and save lives. Let’s come together as one global community and make it happen. Let me know what you or your friends are doing to help your community by sending email to thebatch@deeplearning.ai.
Stay safe and keep learning!
Andrew
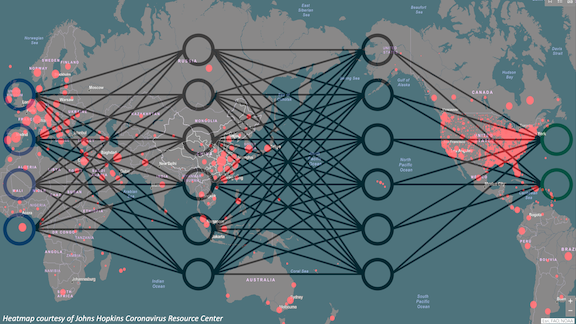
AI Against the Coronavirus
AI could make a life-saving difference in the fight against Covid-19. To assist in the effort, several organizations are contributing open datasets. You can use these resources to analyze trends or launch your own project. You might also want to join efforts like Kaggle’s Covid-19 competitions.
- New York Times Case Data: The New York Times is documenting confirmed Covid-19 cases at the county level. This may be the most granular, comprehensive case dataset available to the public.
- Covid Chest X-Ray Database: Researchers at the University of Montreal offer a database of labeled Covid-19 chest X-ray and CT images. The corpus is updated frequently with data from scientific publications and contributions from the medical community.
- Kinsa Smart Thermometer Weather Map: This map tracks temperature readings from internet-connected thermometers made by Kinsa Health. It provides a fine-grained, albeit noisy, signal of the infection’s prevalence across the U.S.
We’re glad to see so many members of the AI community stepping up to address this crisis. If you want to recommend relevant resources or projects, please let us know at thebatch@deeplearning.ai.
News
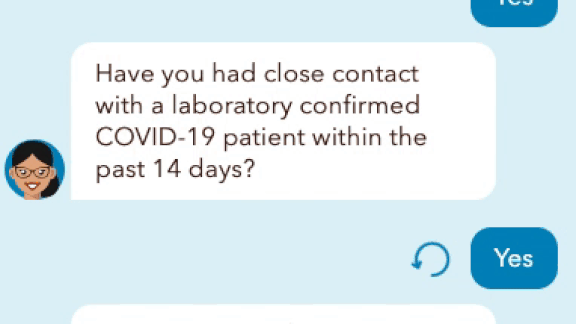
Chatbots Disagree on Covid-19
Chatbots designed to recognize Covid-19 symptoms dispense alarmingly inconsistent recommendations.
What’s new: Given the same symptoms, eight high-profile medical bots responded with divergent, often conflicting advice, according to STAT News.
Conflicting information: Reporters Casey Ross and Erin Brodwin discussed Covid-19 symptoms such as coughing, fever, and shortness of breath with conversational systems offered by government agencies, hospitals, and tech companies.
- The CDC’s Coronavirus Self-Checker told the reporters that they had at least one Covid-19 symptom and recommended they isolate themselves and contact a healthcare provider within 24 hours. A tool from Providence St. Joseph Health told the reporters they might have Covid-19 and suggested they call a physician or 911.
- Buoy Health, a web-based medical service, suggested the symptoms might be a common cold and didn’t recommend special precautions. Google sister company Verily determined that the reporters’ complaints did not warrant further testing.
- In a similar test of popular smart-speakers platforms, Recode found that voice assistants from Amazon, Apple, and Google often answered questions about Covid-19 with information that was overly general, outdated, or lacking context.
Behind the news: A study from Stanford University suggests that symptom checkers built for Covid-19 are flawed partly because the disease’s early signs are similar to those of the common cold or garden-variety influenza. A 2015 study that found that online symptom checkers for a range of conditions often reach faulty conclusions.
Yes, but: Screening tools don’t need to be perfect to add a lot of value. They are statistical tools intended to prioritize quickly and inexpensively which cases should be escalated for deeper examination.
Why it matters: The significant disagreement among these tools means there’s a lot of room for improvement, and bad advice is clearly dangerous in a situation like this. Still, AI-based screening could play a helpful role in this pandemic, especially considering how many countries are short on test kits. It could ease the burden on hospital staff and testing centers, which risk becoming overwhelmed as the pandemic spreads.
We’re thinking: Human doctors’ recommendations aren’t always consistent.
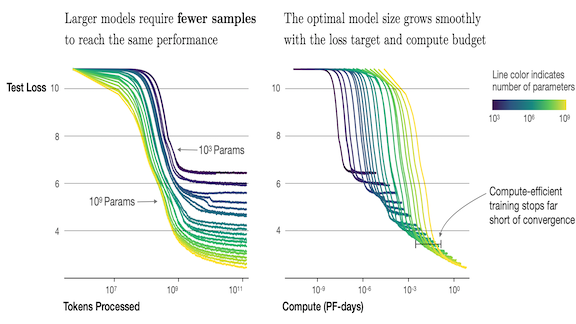
Optimize Your Training Parameters
Last week we reported on a formula to determine model width and dataset size for optimal performance. A new paper contributes equations that optimize some training parameters.
What’s new: Jared Kaplan and Sam McCandlish led researchers at Johns Hopkins and OpenAI to derive equations that describe the effects of parameter count, training corpus size, batch size, and training time on language model performance, plus their own ways to find the best model and dataset sizes.
Key insight: The researchers devised a set of equations that approximate the effects of different combinations of two variables. It’s easier to reason about 2D graphs than to visualize an n-dimensional surface.
Findings: Three findings stand out. First, as many researchers have suspected, transformers outperform LSTMs when trained to convergence. Second, where data and compute are limited, it’s more efficient to train a large model in fewer training steps than to train a smaller model to convergence. Third, some researchers have conjectured that exceeding a so-called critical batch size degrades performance. The researchers offer a way to find optimal batch sizes.
How it works: The researchers trained many model shapes and sizes on various subsets of a proprietary dataset of Reddit posts and linked articles. They measured performance of every combination during training to track the impact of design choices on performance.
- They derived a slightly different formula for loss as a function of model and data size than recent MIT research, but they found a similar relationship: Increasing either parameter count or dataset size improves performance to a point, but then the gains level off. They established a similar relationship between model size and number of training steps.
- The equation that evaluates loss for a given parameter count and numbers of training steps revealed a lower boundary on the number of training steps necessary for early stopping to prevent overfitting. As you might expect, the number of training steps before overfitting rises with dataset size.
- Optimal batch size depends on the model’s loss, they found, not parameter count or dataset size. Optimal batch size rises as the loss decreases.
Why it matters: Most machine learning practitioners don’t have the seemingly infinite computational resources that some large companies do. These insights should help them use resources more effectively.
We’re thinking: Natural language processing is notoriously compute-hungry. The ability to balance processing power against performance could not only save money but reduce environmental impacts.
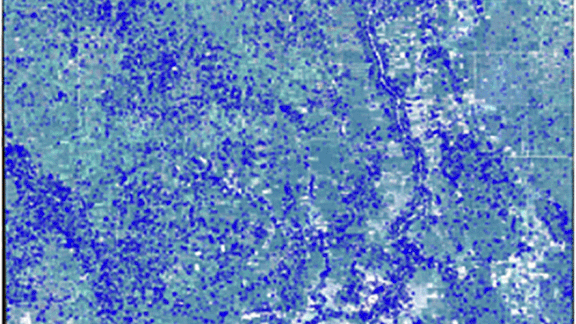
Where Are the Live Bombs?
Unexploded munitions from past wars continue to kill and maim thousands of people every year. Computer vision is helping researchers figure out where these dormant weapons are likely to be.
What’s new: Data scientists at Ohio State University combined computer vision with military records to identify areas in Cambodia where bombs dropped by U.S. planes during its war on neighboring Vietnam remain unexploded.
How it works: The U.S. Air Force kept records of how many bombs it dropped in each air raid, but no one knows how many failed to detonate. The researchers built a tool that counts craters — evidence of bombs that did explode — and then subtracted that number from the total number dropped. The difference enabled them to estimate how many bombs still litter the countryside.
- The researchers hand-labeled 49 craters in satellite imagery, along with 108 other circular objects (such as trees and rice silos) within a 1.5 square kilometer training area. By flipping and rotating the crater images, they increased the training dataset to 1,256 total labeled images.
- The craters can be hard to spot, blurred by decades of erosion and overgrowth and often filled with water. So the researchers developed a two-step model that first identifies potential craters and then culls false positives.
- Step one picked out everything that was circular enough to look vaguely like a crater. It identified 1,229 candidates in the validation area of 9.2 square kilometers (the image with more blue dots in the animation above).
- In step two, the model compared the candidates’ color, shape, size, and texture with craters in the labeled data. Using a decision tree, it correctly identified 152 of 177 verified craters in the area (the image with fewer blue dots).
The results: The researchers used their model to sweep a 100 square kilometer area near the Vietnamese border that had been slammed with 3,205 bombs during the war. Using multiple runs of their model, the researchers found between 1,405 to 1,618 craters, which suggests that up to half of all the bombs dropped in this area are still waiting to be found.
Behind the news: In 1969, U.S. President Richard Nixon secretly ordered the Air Force to begin bombing Cambodia to disrupt Viet Cong supply lines. The campaign left between 50,000 and 150,000 dead. Since then, unexploded bombs and landmines have killed or maimed at least 60,000 people in Cambodia.
Why it matters: Bombs from past wars pose a present danger to people all over the world. But finding them is expensive and labor-intensive. Models that map high concentrations of unexploded ordnance could help organizations working on the problem direct their resources more efficiently.
We’re thinking: It’s heartening to see the technology of the future applied to problems created in the past.
A MESSAGE FROM DEEPLEARNING.AI
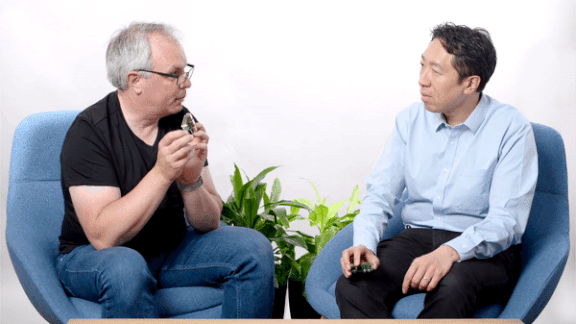
Want to deploy a TensorFlow model in your web browser or on your smartphone? The deeplearning.ai TensorFlow: Data and Deployment Specialization will teach you how. Enroll now
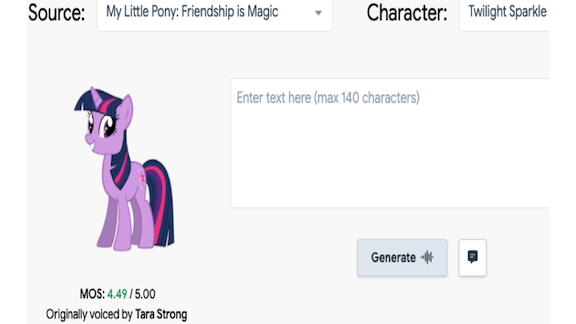
Voice Cloning for the Masses
Are you secretly yearning to have a My Little Pony character voice your next online presentation? A new web app can make your dreams come true.
What’s new: 15.ai translates short text messages into the voices of popular cartoon and video game characters.
How it works: The model’s anonymous developer began the project in 2018 while an undergrad at MIT. In an email to The Batch, the coder declined to disclose details about how the model works but said it was inspired by the 2019 paper that pioneered transfer learning for text-to-speech models.
- 15.ai’s author didn’t disclose how its model differs from the implementation in the paper but said they stumbled on a technique that makes it possible to learn new voices with less than 15 minutes of training data.
- The current roster of voices includes My Little Pony’s Princess Celestia and Team Fortress 2’s Soldier. Characters from the video game Fallout: New Vegas and animated series Steven Universe and Rick and Morty are in the works.
Behind the news: DeepMind made a significant advance in neural audio synthesis in 2016 with WaveNet. That work demonstrated that a neural net trained on multiple examples of similar speech or music could create passable facsimiles. Other teams continue to make advances, for example, in real-time systems.
Why it matters: Voice cloning could be enormously productive. In Hollywood, it could revolutionize the use of virtual actors. In cartoons and audiobooks, it could enable voice actors to participate in many more productions. In online education, kids might pay more attention to lessons delivered by the voices of favorite personalities. And how many YouTube how-to video producers would love to have a synthetic Morgan Freeman narrate their scripts?
Yes, but: Synthesizing a human actor’s voice without consent is arguably unethical and possibly illegal. And this technology will be catnip for deepfakers, who could scrape recordings from social networks to impersonate private individuals.
We’re thinking: Anyone who wants to synthesize Andrew’s voice has more than 15 minutes of deeplearning.ai courseware to train on.
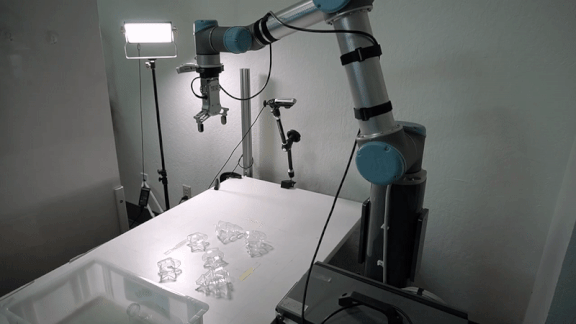
Seeing the See-Through
Glass bottles and crystal bowls bend light in strange ways. Image processing networks often struggle to separate the boundaries of transparent objects from the background that shows through them. A new method sees such items more accurately.
What’s new: Shreeyak Sajjan and researchers at Synthesis.ai, Google, and Columbia University premiered a state-of-the-art model for identifying transparent objects. They call it ClearGrasp, a reference to its intended use in robotics.
Key insight: Faced with a transparent object, RGB-D cameras, which sense color and depth per pixel, can get confused: They take some depth measurements off the object’s surface, others straight through the object. ClearGrasp recognizes such noisy measurements and uses them to predict an object’s shape. Once it knows the object’s shape and how far away one point is, it can infer how far away every point is.
How it works: ClearGrasp incorporates a trio of Deeplabv3+ models with the DRN-D-54 architecture.
- ClearGrasp’s training dataset included 18,000 simulated and 22,000 real images. To make the real images, the researchers photographed transparent objects, yielding depth measurements that encoded distorted light passing through them. Then they painted the objects and photographed them again to obtain accurate depth measurements.
- The first Deeplabv3+ model removes depth measurements associated with transparent objects, retaining data on opaque objects, which presumably is accurate. The second extracts approximate object boundaries. The third generates improved depth measurements.
- ClearGrasp combines the three outputs to get accurate depth measurements of both foreground and background.
Results: Fed real-life data captured by the researchers, ClearGrasp improved the previous state of the art’s root mean squared error of corrected depth measurements from 0.054 to 0.038. A robotic arm using ClearGrasp picked up transparent objects 72 percent of the time, a big step up from 12 percent using unprocessed images.
Why it matters: Machine learning has proven to be adept at noise reduction in various domains. ClearGrasp takes special care to modify only the depth measurements that are distorted.
We’re thinking: ClearGrasp could prevent your robot assistant from having to clean up broken glass all day.
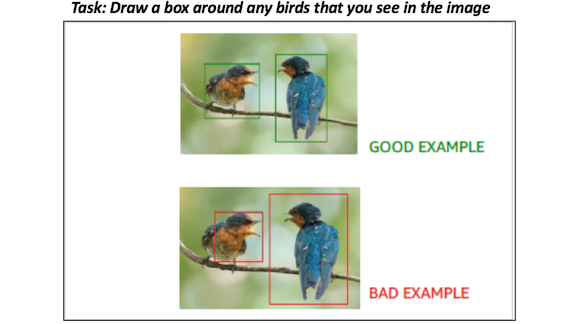
Selling Shovels to Data Miners
When the world is panning for machine learning gold, it pays to help them dig through the data.
What’s new: Machine learning entrepreneurs can make their mark (and their fortune) building services that help other companies develop, deploy, and monitor AI, venture capitalist Rob Toews argues in Forbes.
How it works: Toews points to Scale.AI, a startup that labels data, as one of a new generation of companies capitalizing on the AI industry’s demand for ancillary services. In August, the four-year-old company raised $100 million at a valuation of more than $1 billion. And labeling isn’t the only area of machine learning ripe for entrepreneurship.
- Synthetic data: Applied Intuition, Parallel Domain, and Cognate specialize in making synthetic data for autonomous driving and other applications where real-world training data is often scarce.
- Optimization: Gradio and Alectio help AI developers curate data to improve training efficiency. SigOpt offers a platform that guides companies through model specification from choosing an architecture to determining the number of training epochs.
- End-to-end management: Amazon’s SageMaker offers tools that help manage custom models throughout their lifecycle. Microsoft Azure Machine Learning Studio is geared toward data analysis. Google recently released Cloud AI Platform to get in on the action.
Behind the news: Companies like Adobe and Capital One are spending hundreds of millions on cloud computing. This is driving demand for services that help them handle their cloud resources more efficiently. Among the beneficiaries are companies like Alation, Collibra, and Starburst Data that help catalog, query, and manage machine learning data, writes investor Matt Turck.
Why it matters: Toews believes there are billions of dollars to be made by companies that provide machine learning services. Such services will also nurture new AI applications and accelerate their adoption across a variety of industries.
We’re thinking: These companies aren’t only promising businesses. By taking on tasks like data procurement, model optimization, and lifecycle management, they could free engineers to focus on building products that fulfill deep learning’s potential.