Dear friends,
This week’s issue of The Batch is all about medical applications of AI.
Amid the current pandemic, the marriage of AI and medicine is more urgent than ever. My father is a practicing doctor, and I grew up seeing firsthand how the right care can save lives and reunite families. I’ve been privileged to participate in projects that applied deep learning to diagnosing chest X-rays, assisting with mental health, and interpreting electrocardiograms.
Despite significant research progress, there’s still a long way to go. Jumping into AI for medicine now is like jumping into AI for computer vision back in 2012.
For those who are ready to make the leap, deeplearning.ai is proud to introduce the AI for Medicine Specialization. This new series of courses will teach you the machine learning techniques you need to build a wide range of medical applications.
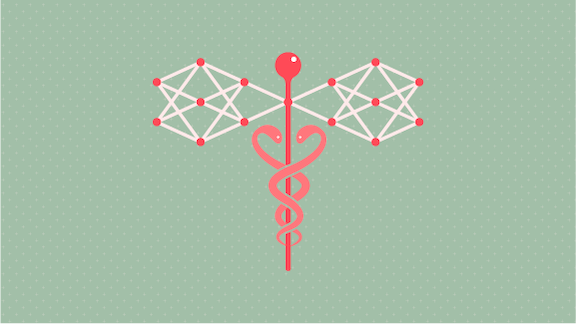
If you’re new to deep learning, start with the Deep Learning Specialization. But if you’ve completed the DLS, or if you have a working knowledge of deep learning and convolutional networks as well as intermediate Python skills, the AI For Medicine Specialization will unlock many opportunities to help solve important problems.
The world needs more AI people working on medicine. I hope you’ll consider being one of them.
Keep learning!
Andrew
AI for Medicine
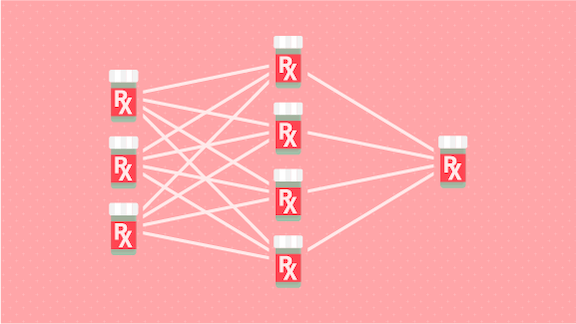
Smarter Care, Healthier Lives
We stand at the threshold of a new era in medicine. We can collect detailed data about individuals continuously throughout their lives. With deep learning, we can correlate background, actions, and outcomes to find paths to optimal health. Some day we may do this globally, so everyone on Earth receives health care appropriately tailored to their unique biology and circumstances. In this special issue of The Batch, we look at how AI is having an impact in medical diagnosis, prognosis, treatment, and data extraction. We hope you’ll join the medical AI revolution and help create a healthier world.
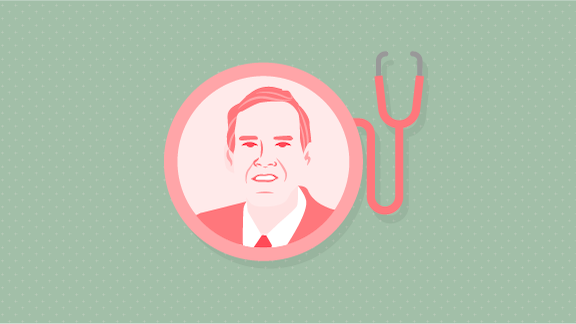
A Visionary Doctor Prescribes AI
Eric Topol is one of the world’s leading advocates for AI in medicine. He believes the technology can not only liberate physicians from the growing burden of clerical work, but also synthesize layers of patient data — behavioral, genomic, microbiomic, and so on — into truly personalized healthcare. A cardiologist and geneticist at Scripps Research Institute in Southern California, he is the author of Deep Medicine: How Artificial Intelligence Can Make Healthcare Human Again. Below he shares his insights into the fusion of AI and medicine and advice for machine learning engineers who want to get involved.
The Batch: Let’s start with the topic on everyone’s mind: Where do you see AI’s greatest potential in addressing the Covid-19 pandemic?
Topol: One thing that’s been overlooked is the ability to develop and validate algorithms for at-home monitoring. We don’t want everyone who has Covid-19 symptoms to go to the hospital. On the other hand, some people who catch Covid-19 have sudden demise, and it’s hard to predict. If we could tell who’s safe to monitor at home, that would be great help in managing this epidemic around the world.
The Batch: You’re concerned with the depersonalization of doctor-patient relationships. How can AI help?
Topol: Four words: the gift of time. Clinicians spend too much of their time being data clerks. There shouldn’t be any need for a screen and a keyboard to see a patient. Entering notes into the medical record should be done by AI.
The Batch: Researchers have had experimental success interpreting medical images. Yet these innovations haven’t had much impact on clinical practice. What’s the holdup?
Topol: The medical community feels threatened that the machines will encroach on their lives. Also, some companies working on things like this have proprietary algorithms and don’t publish their data, so there’s a lack of transparency. They get their FDA clearance based on retrospective studies and use the same data over and over, because there aren’t many large, annotated medical datasets. We need prospective studies based on real-world patients in multiple real-world clinical settings. And we need more randomized trials — there have been only six or seven of those.
The Batch: If you could collect any data you wanted for everyone in the world, what would it be, and for what AI task?
Topol: That’s easy: We need a planetary health system. We’d have multilevel data for every person, and each person would teach the rest of their species about preventing and managing illnesses using nearest neighbor analysis and other tools of AI. It’s possible now, but it requires an international commitment. I wrote about this with my colleague Kai-Fu Lee in an article called “It Takes a Planet.”
The Batch: How can we build a planetary health system that protects data privacy and security?
Topol: The tools are in front of us now. We can use federated and homomorphic computing. No country has to hand their data over. The algorithms can be used at the locale.
The Batch: Much of the AI community is deeply concerned about making sure the technology is used ethically. What should AI practitioners keep in mind in that regard?
Topol: Anything that exacerbates the very significant health inequalities that exist today is not acceptable. Human bias that finds its way into algorithms is a significant ethical concern that needs extensive review and scrutiny. And that’s not all. Algorithms in medicine need to be under constant surveillance because if an algorithm is hacked, it could hurt a lot of people.
The Batch: What advice would you give machine learning engineers who want to make a positive impact in medicine?
Topol: We’re still in the early phase. We need more interdisciplinary or transdisciplinary efforts between clinicians and AI practitioners. We need more large, annotated datasets, or to use self-supervised learning that preempts the need for them. We need to go to a higher validation plane, however we get there. Then we’ll be able to take advantage of this extraordinary opportunity to transform medicine and return the human essence that has been largely lost.
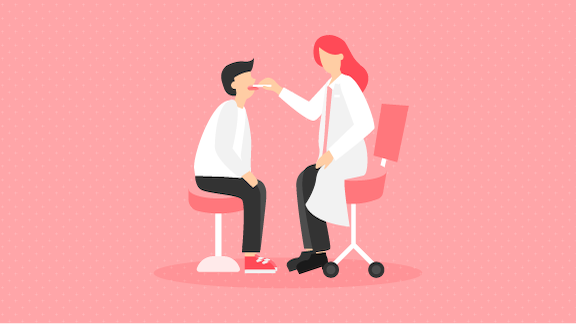
Diagnosis: The Telltale Heart
The wearable revolution is helping doctors figure out what’s troubling your ticker — thanks to deep learning.
The problem: Arrhythmias, a range of conditions in which the heart beats too fast, too slow, or erratically, can cause heart attack or stroke. But they don’t necessarily happen when a doctor is listening.
The solution: Wearable devices from iRhythm constantly monitor a patient’s heartbeat and transmit the measurements to a neural network for analysis.
How it works: The iRhythm Zio AT is an electrocardiogram monitor about the size of a breath-mint box with two wings of peel-and-stick medical tape that fasten onto the skin over a patient’s heart. Electrodes in the monitor track each heartbeat while a separate wireless transmitter sends the data to iRhythm.
- The system collects up to two weeks worth of continuous heartbeat data. If patients feel their heart begin to beat irregularly, they can push a button on the monitor to send a 90-second snippet to iRhythm’s headquarters immediately.
- A neural network analyzes the data. Trained on readings from 53,000 iRhythm Zio wearers, it classifies 12 different patterns: 10 arrhythmias, a normal heartbeat, and a heartbeat distorted by other bodily noises.
- An iRhythm technician reviews the neural network’s analysis and posts it to the patient’s electronic health record for physicians to see.
Status: The United States Food and Drug Administration approved iRhythm’s Zio AT in 2018, and the system is on the market. The company recently partnered with Verily and Apple to develop further products.
Behind the news: A 2019 review of 14 studies that compared AI with human clinicians found that deep learning models were roughly as good as human professionals at diagnosing signs of disease in medical imagery. The authors noted, however, that the studies tend to suffer from poor controls, inconsistent metrics for measuring success, and lack of independent validation. No comparable assessment of non-image AI diagnostics exists, but the fact that Apple is integrating arrhythmia detection into its smartwatch suggests that the field is maturing.
Why it matters: Arrhythmias occur sporadically enough that spotting them requires many days of data. “You’ll never catch one by running an electrocardiogram in the office,” according to Dr. Mauricio Arruda of Cleveland’s University Hospitals Harrington Heart & Vascular Institute. By combining long-term observations with short-turnaround assessment, AI enables cardiologists to intervene with precise, timely, and potentially life-saving treatments.
We’re thinking: Just the thought of AI saving somebody from a stroke makes our hearts skip a beat.
To learn how you can use AI to diagnose illnesses, check out Course 1 of the AI for Medicine Specialization from deeplearning.ai.
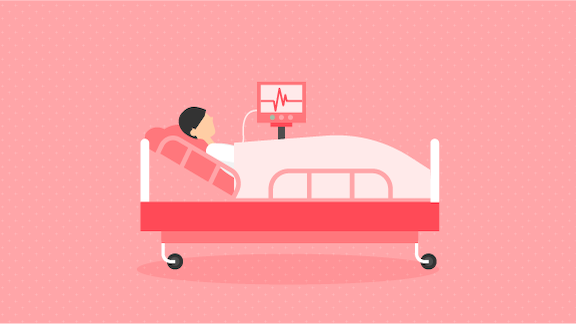
Prognosis: Early Warning for Sepsis
An AI-driven alarm system helps rescue patients before infections become fatal.
The problem: Machine learning can spot patterns in electronic health data indicating where a patient’s condition is headed that may be too subtle for doctors and nurses to catch. Sepsis, for instance, is a response to infection that inflames a patient’s organs, killing some 270,000 Americans each year. The ability to catch it early can save lives.
The solution: Sepsis Watch is a deep learning model that spots signs of sepsis up to five hours before it becomes dangerous. This crucial window allows clinicians to intervene.
How it works: The system integrates vital signs, test results, and medical histories of emergency-room patients, assessing their risk of septic shock on a scale of 0 to 100 percent. If the risk reaches 60 percent, the system alerts nurses in the hospital’s rapid response team. It also publishes an hourly list of each patient’s septic risk score.
- Researchers from Duke University, Harvard, and Google trained the model on a dataset of 50,000 patient records from the Duke hospital system.
- They evaluated the model at Duke and later expanded to two other community hospitals. All three continue to use it.
- The researchers designed Sepsis Watch with input from the hospital’s rapid response nurses. The collaboration, they say, made staff more likely to use the app.
Status: Duke physician and data scientist Mark Sendak and colleagues conducted a clinical trial between November 2018 and July 2019. Sepsis Watch significantly improved sepsis response times, Sendak told The Batch. The team plans to publish the results in the near future. Last July, Duke University licensed the software to Cohere Med, an AI healthcare startup.
Behind the news: Suchi Saria, a machine learning expert at Johns Hopkins University, was a pioneer in the use of reinforcement learning to identify sepsis treatment strategies back in 2018. Duke’s Sendak helped evaluate models for other kinds of clinical decision support in a recent survey in the European Medical Journal. The authors’ picks included early-warning systems for cardiac arrest, surgical complications, pneumonia, and kidney disease.
Why it matters: As little as three hours of warning can give caregivers time to begin tests and medications that dramatically improve a sepsis victim’s odds of survival.
We’re thinking: Building a great model is Step 1. Deployment is Step 2. Collaborating with hospital staff is a sharp way to promote Step 3, utilization.
Learn how to build your own prognostic models in Course 2 of the AI For Medicine Specialization.
A MESSAGE FROM DEEPLEARNING.AI
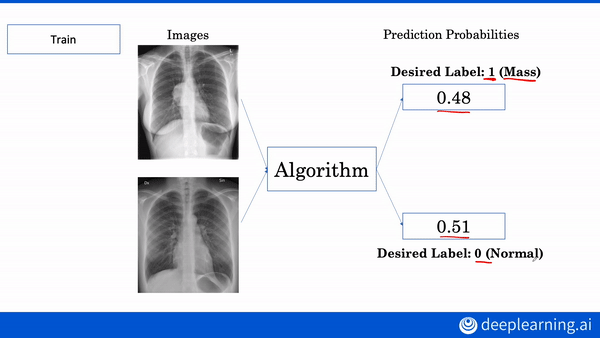
Interested in learning more about AI applications in medicine? Build your own diagnostic and prognostic models in our new AI for Medicine Specialization. Enroll now
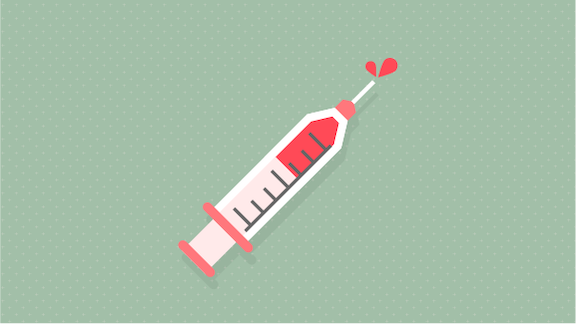
Treatment: The Elusive Molecule
Will deep learning discover new medicines? Startups — and big-pharma partners — are betting on it.
The problem: In theory, there’s a pharmacological cure for just about any ailment. In practice, discovering those therapies takes years and billions of dollars.
The solution: Deep learning, with its ability to discern patterns amid noise, could speed up drug discovery considerably. In a dramatic test, Insilico used an algorithm to sift through petabytes of biochemical data to find potential drugs in 21 days.
How it works: Based in Rockville, Maryland, Insilico used its Generative Tensorial Reinforcement Learning, or GENTRL, to create digital representations of molecules with properties that inhibit an enzyme linked to several types of cancer, atherosclerosis, and fibrosis.
- To make sure the model steered clear of established intellectual property, the researchers fed it a database of 17,000 patented compounds.
- The model produced 30,000 candidates, which the researchers whittled down to 848 using a mix of computational and AI methods.
- They selected 40 at random to examine more closely. They sent six of the most promising to WuXi AppTec, a pharmaceutical contract manufacturer in Shanghai, to synthesize. One of the molecules did indeed inhibit the enzyme in mice.
Status: Insilico’s enzyme inhibitor was only a proof of concept. However, it attracted partnerships with GlaxoSmithKline, Jiangsu Chia Tai Fenghai Pharmaceutical, and Pfizer.
Behind the news: Drug discovery is an attractive target for AI startups, given the abundance of biochemical data and desperation of pharmaceutical giants to cut costs. But success still seems hit-or-miss. Only one AI-designed drug — made by Exscientia — has progressed to human trials. Verseon has been working on the problem for nearly two decades without creating a marketable product. And, crucially, no one has found a reliable way to accelerate clinical trials, the most expensive and time-consuming part of drug development.
Why it matters: The average successful drug costs $2.5 billion dollars to bring to market, according to a 2016 study. Cutting even a fraction of that cost could allow companies to channel resources towards more and different drugs, potentially providing the public with more cures in less time.
We’re thinking: Finding a molecule that becomes a viable drug is like hunting for a single, specific plankton in the Pacific Ocean. Good thing machine learning engineers relish searching for tiny patterns in massive pools of data.
Use deep learning to estimate treatment effects for individual patients in Course 3 of our AI for Medicine Specialization.
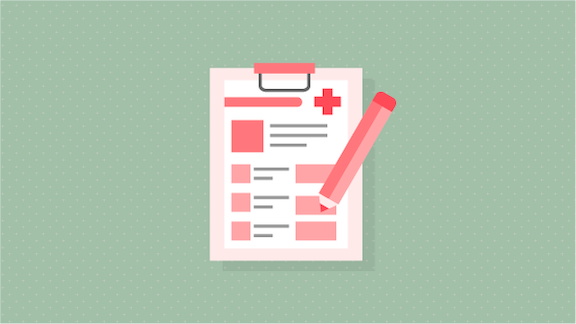
Data: From Patient to Health Record
Doctors are overwhelmed by clerical work. Healthcare-savvy voice assistants are picking up the slack.
The problem: Doctors generate lots of vital information while examining a patient. Properly recorded, it becomes data that informs treatment — but entering it properly is a time-consuming task that drains docs’ attention and finances.
The solution: Voice assistants can serve as clinical stenographers. Suki is one of several apps on the market that transcribe doctors’ observations and instructions and insert them into a patient’s electronic health record.
How it works: Saying “Suki, the patient is running a fever and has fluid in their lungs,” inserts a note in the patient’s record. “Suki, show me the patient’s prescriptions,” retrieves that information. “Suki, I examined the patient,” enters the full description of a normal exam, ready for customization to the particular case. The model also adds diagnostic codes for tests and procedures, which aid in billing.
- Suki uses off-the-shelf voice recognition from Google and other vendors, augmented by the company’s own deep learning models. These models were trained on public datasets of speech plus a proprietary corpus of 250,000 anonymized patient-doctor interactions to capture the nuances of medical jargon. The engineers added background noises and conversation to make the models more robust.
- The engineers built several natural language task models that incorporate custom word embeddings, text classification, and entity recognition. These were trained on a combination of anonymized proprietary patient notes and public repositories of medical and clinical text. They retrain these models periodically using updated data.
- The company cites internal research showing that doctors who use Suki spend 70 percent less time doing clerical work. The system complies with U.S. regulations that protect sensitive personal information.
Status: Suki, which integrates with several popular electronic health records, is deployed in the health network Ascension, Unified Women’s Health Care, and more than 90 small-to-midsize practices. As of July, the software operated in seven specialties including internal medicine, OB-GYN, and pediatrics. The company is working on new features for smarter billing ordering items like prescriptions and tests.
Behind the news: Suki has plenty of competition. Rivals include Saykara, Nuance, M*Modal, and Notable.
Why it matters: Doctors are drowning in paperwork, and voice-assistant technology can help them come up for air. A 2016 study estimates that doctors spend between 37 and 49 percent of their working hours on clerical tasks. All that paperwork contributes to the high level of burnout and depression in the profession, according to a 2019 study.
We’re thinking: If you notice an improvement in your physician’s bedside manner, you might want to thank a robot.
Build a natural language tool to extract data from medical information in Course 3 of the AI for Medicine Specialization from deeplearning.ai.