Dear friends,
Inflection points in society create opportunities. The rise of online video was an inflection point that enabled scalable online education. The rise of the GPS-enabled smartphones similarly enabled Uber, Lyft, Airbnb, and many other services. Today, the rise of deep learning is transforming many industries.
Covid-19 is both a tragedy and an inflection point.
- Working from home seems to be here to stay. Several of my California-based teams no longer hire exclusively in the state, but anywhere within three hours of our time zone. As more companies do this, it will open up job opportunities while intensifying the need for remote collaboration tools.
- Many parts of society seem to be accepting some Covid tracking tools to improve safety, even if they modestly sacrifice privacy.
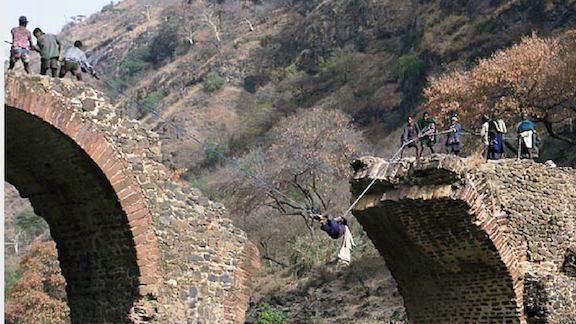
- Industries such as air travel, tourism, and commercial real estate are being decimated and will have to adapt as demand remains suppressed for the foreseeable future.
- Many schools have scrambled to go online. As learners worldwide get used to studying remotely, many won’t want to go back to the old way.
- Untold numbers of workers are unemployed. When we eventually bring unemployment down again, the distribution of jobs will be very different than it is today.
We have powerful AI tools at our disposal, and we can use them to meet this inflection point. Our community can build better collaboration tools, find ways to retrain displaced workers, implement Covid tracking systems that protect civil liberties even as they promote public health, bring decimated brick-and-mortar businesses online, and invent new jobs that can be done from home. The work we do today will lay the foundation for the tomorrow we live in.
How can we navigate these tumultuous changes and help the most vulnerable? My teams will be trying to do our part, and I hope you will too.
Keep learning!
Andrew
Covid-19 Watch
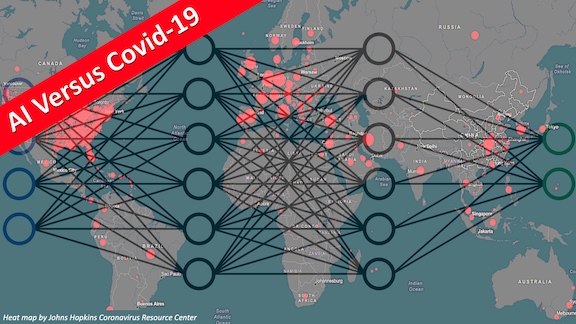
New Machine Learning Resources
Our latest recommended resources for tackling coronavirus: a trove of epidemiological estimates and real-world data for validating models.
- Epidemiological Estimates: Modeling effects of Covid-19 is tricky given all the variables that can influence predictions: rates of transmission, hospitalization, death, and so on. That’s why the MIDAS Network, a scientific collaboration focused on improving modeling of infectious diseases, compiled estimates of such statistics. The list includes a range of epidemiological characteristics in many countries. It could also be incorporated into a meta-analysis of Covid-19 modeling research.
- DREAM Challenge: To help researchers validate their Covid-19 hypotheses, a team at the University of Washington set up a cloud-based framework. Developers can upload models and validate them on anonymized electronic health records from the UW Medical Center. The team poses a starter question: Of patients who saw a doctor and were tested for Covid-19, can we predict who is positive? The best model will be distributed to health systems across the country.
News
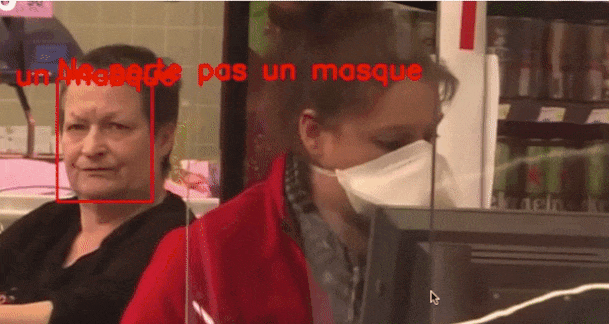
Mask Monitor
Cameras that detect face masks are helping French authorities to evaluate citizens’ adherence to government mandates intended to fight Covid-19.
What’s new: Starting this week, everyone riding public transportation in France is required to wear a face mask. Paris and Cannes are using computer vision to count people who comply.
How it works: Datakalab, a French AI startup, is installing chips in existing CCTV cameras that run an object recognition model. The model is trained to distinguish masked faces from unmasked ones.
- Paris is testing the cameras at the busy Chatelet-Les Halles metro station. Cannes has installed them on buses and in public markets.
- The software counts mask wearers every 15 minutes and transmits aggregate statistics to the authorities. The company says the system is meant to help authorities determine where to step up efforts to promote mask-wearing
- Datakalab provides similar technology for use in retailing. Those systems note customers’ age, gender, how long they stay in certain areas, and whether they’re smiling.
Behind the news: AI is being used widely to monitor compliance with rules designed to combat the spread of Covid-19.
- The Indian state of Punjab is using drones from Skylark Laboratories to enforce social distancing and curfew regulations.
- Hospitality companies have deployed cameras from Wobot Intelligence to ensure that employees are washing their hands for at least 20 seconds.
Yes, but: France’s privacy commission warns that mask detection technology may violate European rules that limit personal data collection. Datakalab counters that its systems neither identify individuals nor store data. In any case, 94 percent of French citizens support wearing masks in public according to a recent poll. (France continues to outlaw burqas and other religious face coverings under a 2011 law.)
Why it matters: As France and other countries begin to lift rules that keep people physically apart, wearing masks is critical to limiting coronavirus transmission.
We’re thinking: Covid-19 surveillance is a double-edged sword: helpful in containing the pandemic but worrisome in other contexts. Governments and businesses must use it appropriately and only while the need persists.
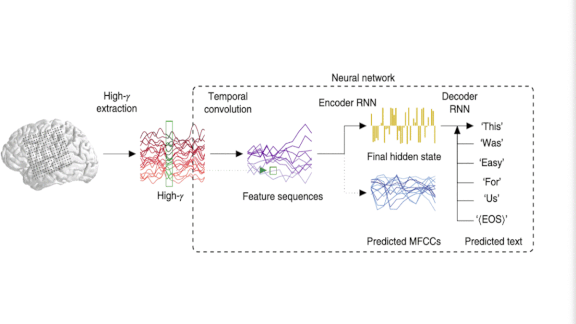
Listening to the Brain
AI has added an unlikely language to its catalog of translation capabilities: brain waves.
What’s new: Joseph Makin led a group from the University of California San Francisco to render a person’s neural signals as English text while the person read a sentence aloud. Sometimes the system produced gibberish. For instance, it translated brain waves representing, “the woman is holding a broom” into “the little is giggling giggling.” But much of its output was very close to the spoken words: “The ladder was used to rescue the cat and the man” came out as “which ladder will be used to rescue the cat and the man.”
Key insight: Brain activity isn’t spoken or readable in the usual sense, but it has structural similarities to language.
How it works: Patients undergoing surgery for epilepsy had electrodes attached to the cortical surface. The researchers captured neural activity while the speaker read a sentence and discarded signals with the lowest strength. A model learned to translate the brain waves into a transcript.
- Brain scans often detect signals at different times relative to when they began. A convolutional filter applied across time captured the signals within a time window to account for mistimings.
- A recurrent neural network learned to extract key features of a sequence of filtered brain activity one time window at a time. After that RNN extracted the features of an entire sequence, a second RNN learned to reconstruct the spoken sentence one word at a time based on the features and the previously predicted word.
- During training, another network predicted features of the sentence’s sound based on the extracted features. This additional task helped the first RNN to extract brainwave features most closely related to the sentence.
Results: The researchers evaluated their method by word error rate (WER) between true and predicted sentences. Trained on one person reading 50 distinct sentences, the network achieved a 3 percent WER. The network vastly outperformed the previous state of the art, which scored 60 percent WER measured on a different dataset.
Yes, but: The researchers tested their network on a larger vocabulary than previous methods. Still, the vocabulary was small: only around 250 words. Classifying a brain wave as one of 250 words is easier than recognizing it among the 170,000 in the English language.
Why it matters: The ability to find words in brain waves cracks open a sci-fi Pandora’s box. It’s worth emphasizing that the researchers read brain waves associated with speech, not necessarily thought. Yet it’s amazing that the same learning algorithm works for both brain-to-language and language-to-language translations.
We’re thinking: We look forward to upgrading Alexa from voice recognition to mind reading (except for the annoying detail of implanting electrodes in our brains).
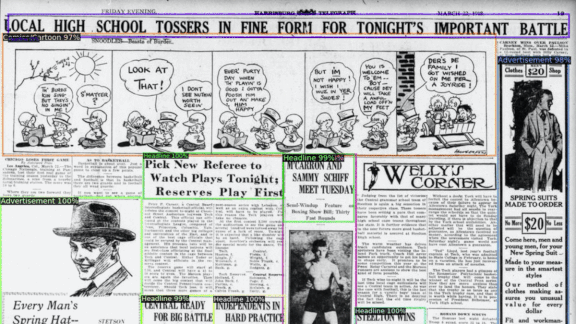
An Archive Unearthed
An algorithm indexed photos, ads, and other images embedded in 170 years of American newspapers.
What’s new: Created by researchers at the University of Washington and U.S. Library of Congress, Newspaper Navigator uses object recognition to organize visual features in 16 million pages of newspapers dating back to 1789. The tool makes it easy to search this archive — and hopefully others before long — for visual elements.
How it works: The researchers fine-tuned Faster R-CNN to flag seven types of visual newspaper content from cartoons to maps.
- They trained the system on Beyond Words, an annotated archive of World War I-era newspapers. The dataset also includes transcriptions of headlines and captions to help calibrate optical character recognition.
- The researchers added labels for headlines and advertisements.
- The system uses optical character recognition to append titles and captions to illustrations and photos. It also produces machine-readable versions of headlines.
Behind the news: A number of researchers are using AI to mine the mountains of information locked in digitized newspapers and other historical sources.
- PageNet recognizes page boundaries in handwritten historical documents.
- Swiss researchers devised dhSegment, a neural network that helps with a range of tasks related to historical images such as analyzing a document’s layout and detecting the ornamental letter illustrations that begin chapters in many old texts.
- The University of Lincoln-Nebraska’s Aida project seeks out poetry in old newspapers.
Why it matters: Newspapers are invaluable resources for historians, journalists, and other researchers. Newspaper Navigator’s creators open-sourced their work so it can be used to search other digital archives.
We’re thinking: Sometimes we have a hard time finding old GIFs from The Batch. Maybe the Library of Congress could give us a hand too?
A MESSAGE FROM DEEPLEARNING.AI
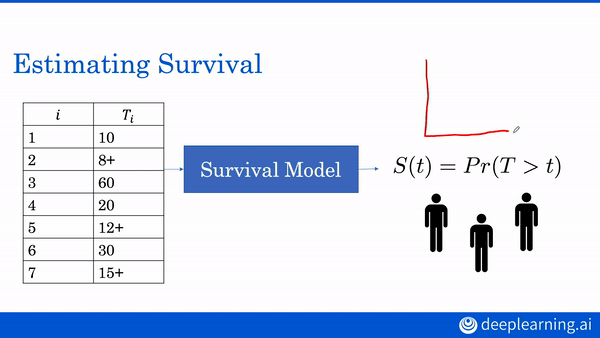
How can you estimate a patient’s future health? Build your own survival model in Course 2 of the AI for Medicine Specialization. Enroll now
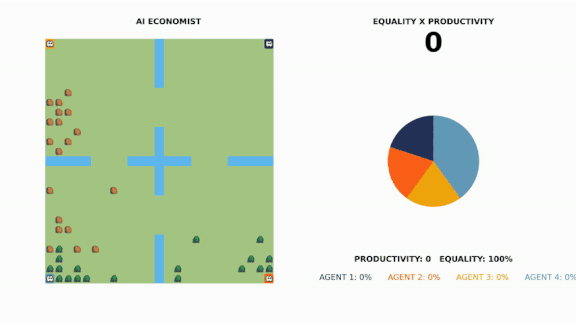
Taxation With Vector Representation
Governments have struggled to find a tax formula that promotes prosperity without creating extremes of wealth and poverty. Can machine learning show the way?
What’s new: Data scientists at Salesforce used reinforcement learning to develop a tax policy aimed at optimizing worker productivity and income equality.
How it works: The researchers developed a video game-type simulation in which four reinforcement learning agents worked to earn money while a fifth taxed their income.
- None of the agents had prior knowledge of the game’s economy. The workers were instructed to build wealth by either harvesting resources or building homes.
- Each virtual worker had a different skill level. The lower-skilled workers learned that acquiring and selling wood or stone was the best way for them to make money, while their higher-skilled colleagues gravitated to the more complex, higher-paying task of building houses.
- Each game ran through 10 tax periods. At the end of each period, the tax bot took a portion of each worker’s earnings, then redistributed the money among all the workers. The process was repeated millions of times.
- The researchers also tested the simulation under three human-created tax strategies: A free market approach, the current U.S. tax code, and an academic tax proposal favoring higher income equality.
Results: The system optimized the balance between productivity and inequality more effectively than the human-created strategies. Its policy counterintuitively set high tax rates for the highest and lowest earners and assigned the lowest rates to middle earners.
Yes, but: A model with four workers isn’t nearly complex enough to simulate a real economy, Blake LeBaron, an economist at Brandeis University told MIT Technology Review. The Salesforce team plans to scale up the system to 100 workers.
Why it matters: More than 70 percent of the world’s population live in nations where income inequality is rising, according to the United Nations. Tax policy is a powerful tool for building more prosperous, resilient economies.
We’re thinking: Using AI to discover good social policies? Great idea! Imposing high tax rates on the lowest earners? Not so much.
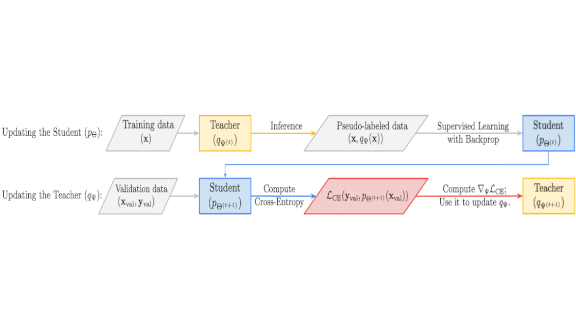
Flexible Teachers, Smarter Students
Human teachers can teach more effectively by adjusting their methods in response to student feedback. It turns out that teacher networks can do the same.
What’s new: Hieu Pham led joint work by Carnegie Mellon and Google Brain that trained teacher models (larger, pretrained networks) to educate student models (smaller networks that learn from the teacher’s predictions) more effectively by observing and adjusting to student performance. The method’s name, Meta Pseudo Labels, refers to meta-learning: in this case, learning from predictions that have been tweaked to optimize their educational value rather than their accuracy. Pseudo labels are teacher classifications, either binary or values between 0 and 1, that a student learns to re-create.
Key insight: A teacher may generate predictions showing that one dog looks more like a wolf than a cat, while another dog falls in between. But its pseudo label “dog” doesn’t capture that difference. For instance, a model considering two images may output [0.8, 0.2, 0.0] and [0.6, 0.2, 0.2] to express its confidence that they depict a dog, wolf, or cat. Both classifications reflect high confidence that the image is a dog, but they contain more nuanced information. Rather than receiving only the highest-confidence classifications, the student will learn better if the teacher adjusts its predictions to exaggerate, say, the dogishness of wolfish dogs. For example, the teacher may change [0.8, 0.2, 0.0] to [0.9, 0.1, 0.0].
How it works: WideResNet-28-2 and ResNet 50 teachers taught EfficientNet students how to recognize images from CIFAR-10 , SVHN, and ImageNet.
- The student learns from a minibatch of images classified by the teacher. Then the student makes predictions on some of the validation set. The teacher learns to minimize the student’s validation loss. The student learns from the teacher’s prediction distribution, so backpropagation can update the teacher based on student errors. Then the process repeats for the next minibatch.
- It may take many training steps before the teacher learns a better distribution. (As any teacher will tell you, the longer students are confused, the less they learn, and the more the teacher must adjust.) The teacher also learns from a small amount of labeled data in the validation set to prevent mis-teaching the student early in training.
- Training the teacher on the validation set may look like a bad idea, but the student is never directly exposed to the validation set’s labels. The teacher’s additional knowledge helps the student generalize without overfitting the validation set.
Results: Meta Pseudo Labels produced a student with higher ImageNet accuracy (86.9 percent) than a supervised model (84.5 percent). The improvements remained when using a limited number of labels from each dataset, where MPL achieved CIFAR-10 accuracy of 83.7 percent compared with a supervised model’s 82.1, and SVHN accuracy to 91.9 percent compared with 88.2.
Why it matters: Student-teacher training began as a compression technique. But lately Noisy Student and Meta Pseudo Labels are making it a competitive approach to training models that generalize.
We’re thinking: At deeplearning.ai, we aim to keep improving our instruction based on student feedback — but please make your feedback differentiable.
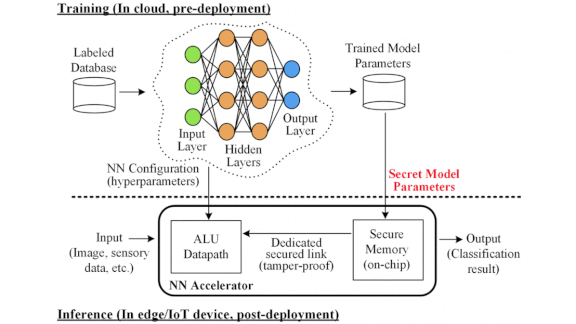
Undercover Networks
Neural networks can spill their secrets to those who know how to ask. A new approach secures them from prying eyes.
What’s new: Security researchers at North Carolina State University and Intel in a paper demonstrate that adversaries can find out a model’s parameter values by measuring its power use. They offer countermeasures that mask those values.
How it works: The authors show that neural networks, especially those designed to run on smart home speakers and other edge devices, are vulnerable to so-called differential power analysis. The researchers deciphered the weights of a binary neural network with three fully connected hidden layers of 1,024 neurons each by monitoring its power consumption over multiple inference operations.
- To thwart such attacks, the researchers adapted a tactic from cryptography called masking. It involves instructing the neural network to randomly split its computations into two streams each time it runs and recombine the streams at the end of the run. In tests, this approach masked the weights.
- The researchers propose another method called hiding, in which the system artificially turns its power consumption up while running sensitive operations. This makes it impossible for adversaries to measure power consumption.
- The researchers say their masking and hiding methods are adaptable to any type of neural network.
Behind the news: Cryptography researchers wrote about differential power analysis and a related technique called simple power analysis as far back as 1998. Both techniques exploit the fact that computer processors use more energy to change a 0 to a 1 (or vice versa) than to maintain either value.
Yes, but: The countermeasures proposed by the researchers throttled the system’s performance, slowing it down by as much as 50 percent. The authors also worry that adversaries could find ways to analyze the two streams, forcing the defenders to split computations further with an even greater impact on performance.
Why it matters: The ability to reverse engineer a neural network’s weights makes it easier to create adversarial examples that fool the network, and to build knockoffs that put security and intellectual property at risk.
We’re thinking: Deep learning opening new use cases — but also new vulnerabilities that will require ongoing research to identify and counter.