Dear friends,
Earlier this week, I asked a question on social media: What is the most important problem that the AI community should work on?
Thousands of you responded. The most frequently mentioned themes included:
- Climate change and environmental issues
- Combating misinformation
- Healthcare including Covid-19
- Explainable and ethical AI
Thank you to each person who responded. I have been reading and thinking a lot about your answers. Many of the most pressing problems, such as climate change, aren’t intrinsically AI problems. But AI can play an important role, and I’m encouraged that so many of you want to do good in the world.
Each of us has a role to play. But we rarely succeed alone. That’s why community matters.
To my mind, the defining feature of a community is a shared set of values. The medical community prioritizes patients’ wellbeing. When one doctor meets another, their shared priorities immediately create trust and allow them to work together more effectively, say, consulting on complex cases or building initiatives to help underserved people. The academic community also has a history of collaboration stemming from its shared belief in the value of searching for and disseminating knowledge. So, too, in other fields.
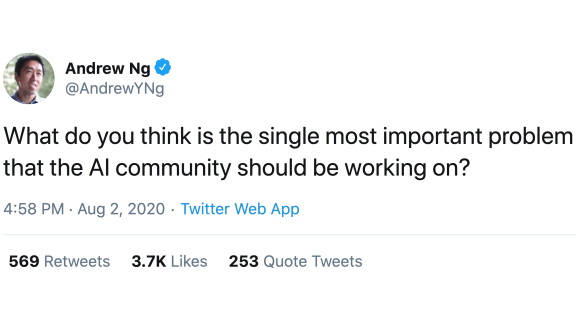
We in the AI community may share many aims, but the first step toward being more effective as a community is to converge on a set of values we can all stand behind. I believe that if we do this, we can tackle much bigger problems with much greater success.
So what, my fellow deep learners, does the AI community stand for? The task of organizing ourselves to tackle big problems together will come later. But first, we need to define the common ground on which we will stand. Many of us hold a strong belief in lifelong learning, sharing information, and working on projects that make society better off. What else? I have ideas of my own, but I would love to hear yours. Please reply to [email protected] or let me know on LinkedIn, Twitter, or Facebook.
None of us can solve even one of these issues single-handedly. But working together, I’m optimistic that we can have a huge impact on all of them.
Keep learning!
Andrew
DeepLearning.ai Exclusive
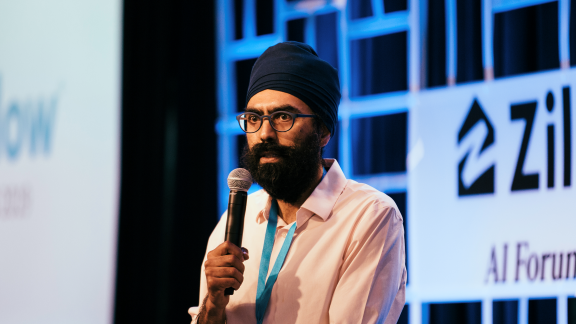
Working AI: Dream Homes Delivered
Jasjeet Thind is bringing the convenience of ecommerce to real estate. In this edition of our Working AI series, Zillow’s VP of AI explains how he’s building an all-in-one pipeline for home sales and offers advice to up-and-coming machine learning engineers. Learn more
News
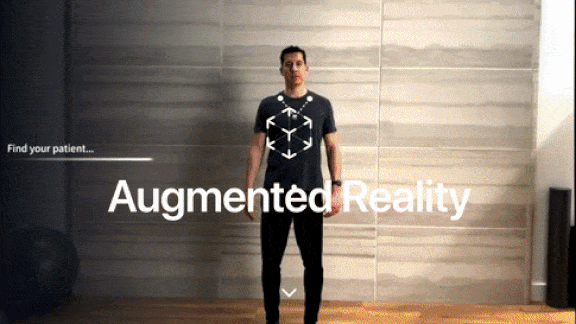
Apple Kicks AI Into High Gear
After years of trailing other tech giants in AI, Apple has a new ambition: to become the industry’s leading purveyor of products powered by machine learning.
What’s new: In an interview with Ars Technica, the company’s AI chief argues that its pro-privacy, on-device approach is the best way to build such applications.
Think different: John Giannandrea, the former head of Google’s AI and search who joined Apple in 2018, outlined the iPhone maker’s effort to infuse the technology into a wide range of products and services.
- Apple is putting a marketing push behind augmented reality apps and upgrades to its personal digital assistant Siri. It also touts AI features such as managing its devices’ energy consumption based on user habits and fusing successive photos into a single high-quality image.
- Like Google, Huawei, Qualcomm, and Samsung, Apple designed specialized chips to run AI software on smartphones, tablets, and watches. Its laptops are expected to include the chip later this year.
- Rather than executing tasks in the cloud, a chip subsystem called the Neural Engine processes most machine learning tasks on Apple devices. Processing data on the device helps preserve user privacy and reduces latency, so the software runs closer to real time, according to Giannandrea.
- Despite the company’s pro-privacy stance, it does collect and label some anonymized data, Giannandrea said. It also asks users to donate data with prompts like, “Would you like to make Siri better?”
Buying in: Apple lists dozens of AI job openings, but it has acquired much of its AI technology by buying other companies. It purchased at least 20 machine learning startups — more than any of its rivals — since buying Siri in 2010, according to venture tracker CB Insights.
Why it matters: Apple’s privacy-centric, edge-based approach stands out from much of the industry’s reliance on aggressive data collection and processing in the cloud. The difference could help counteract the longstanding impression that it’s behind other tech giants in AI.
We’re thinking: AI’s voracious appetite for data boosts the accuracy of supervised learning systems, but it poses risks to user privacy. Apple’s effort to avoid collecting and exposing user data is refreshing — and raises the stakes for small data techniques that enable systems to learn effectively with fewer examples.
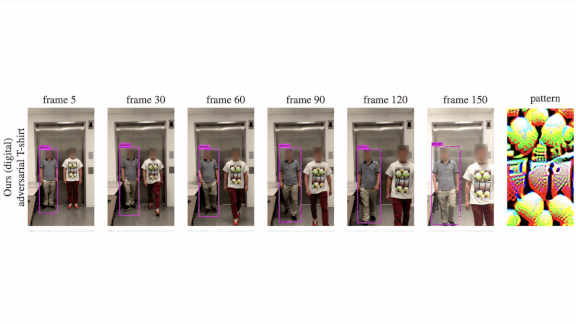
Hidden in Plain Sight
With the rise of AI-driven surveillance, anonymity is in fashion. Researchers are working on clothing that evades face recognition systems.
What’s new: Kaidi Xu and colleagues at Northeastern, MIT-IBM Watson AI Lab, and MIT designed a t-shirt that tricks a variety of object detection models into failing to spot people.
Key insight: Researchers have created images that, when held in front of a camera, can confuse an object detector. But surveillance cameras can view people from a range of distances and angles, and images on clothes warp as the wearer moves. To manage these limitations, the authors tracked a shirt’s deformations in motion. Then they mapped the same deformations onto candidate adversarial images until they found one that evaded the detector.
How it works: Machine learning typically involves training a model to map an image to a label. Generating adversarial images involves choosing a label, holding model weights constant, and finding an input that causes the network to select that label. The researchers devised a design that, when projected onto a t-shirt, caused a variety of object detectors to classify “no label.”
- The researchers printed a checkerboard pattern onto a t-shirt and recorded videos of people wearing the shirt. The checkerboard pattern enabled them to measure the shirt’s deformation in each video frame as the pattern changed with wrinkles, lighting, or scale and angle.
- Armed with these measurements, they used the interpolation technique known as thin plate spline (TPS) to replace the checkerboard in each frame with another image.
- The TPS distortions are differentiable, so backprop can adjust the image to fool the object detector across all frames.
- The adversarial image can be optimized to confuse any object detector or multiple detectors simultaneously. The researchers focused on YOLOv2 and Faster R-CNN, which are commonly deployed in surveillance systems.
Results: The researchers printed an adversarial image onto a shirt and collected videos of it in action. It fooled YOLOv2 in 57 percent of frames, a big improvement over the previous state of the art’s 18 percent.
Yes, but: A detector that classifies even a single frame correctly opens the door to defeating this technique. Practical adversarial wear may require a success rate nearer to 100 percent. If this technique takes off, face detection suppliers are bound to develop countermeasures.
Why it matters: Adversarial images have been added to training data to strengthen image classifiers against attacks. TPS could play a role in similar methods to prevent object detectors from being tricked.
We’re thinking: Given that software to counter the authors’ technique can be updated faster than clothes manufacturing and distribution, we’re not convinced this approach can scale.
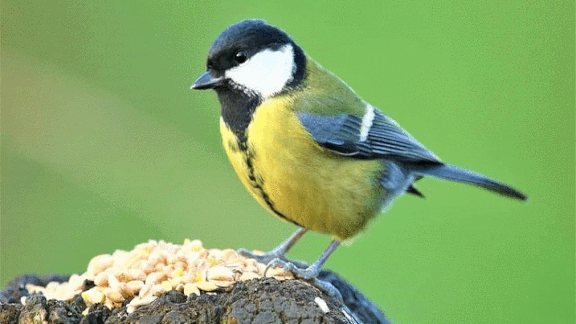
Birdwatching With AI
Neural networks learned to tell one bird from another, enabling scientists to study their behavior in greater detail.
What’s new: Researchers from universities in Europe and Africa trained neural networks to recognize individual birds with up to 90 percent accuracy, as detailed in Methods in Ecology and Evolution.
How it works: Researchers collected data by attaching radio-frequency identification tags to 35 of the African songbirds known as sociable weavers. Then they set up cameras to snap pictures, tagged with each creature’s identity, automatically whenever one entered a feeding area.
- The researchers used the Mask R-CNN instance segmentation network trained on the Coco image dataset, which includes pictures of birds, to locate and crop the birds in each picture.
- They pretrained a VGG19 convolutional neural network on ImageNet and fine-tuned it on 900 images of each bird (plus augmentations) to recognize the individuals based on distinctive patterns on their back and wing feathers.
- The researchers used a similar method to train models to spot individuals of two other species as well.
Behind the news: AI is increasingly useful for identifying individuals of various animal species, including chimpanzees, elephants, and pigs.
Why it matters: The researchers aimed to learn how sociable weavers cooperate to build large, communal nests. Catching, tagging, and observing animals in the wild takes a lot of time and effort. AI that automates the process can free up researchers to focus on extracting insights from the behavioral data they gather.
We’re thinking: Now birds are getting the face recognition tweetment!
A MESSAGE FROM DEEPLEARNING.AI
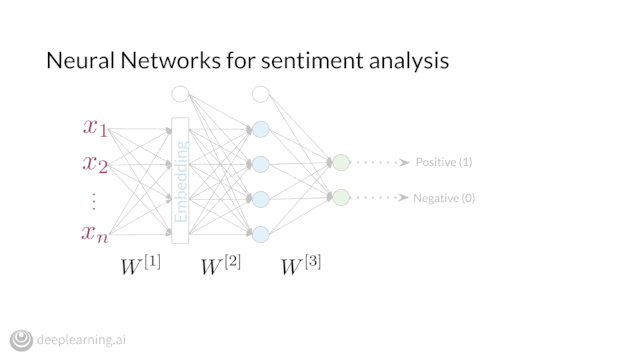
Enroll in Course 3 of our Natural Language Processing Specialization on Coursera to learn in-demand ML skills and cutting-edge NLP techniques.
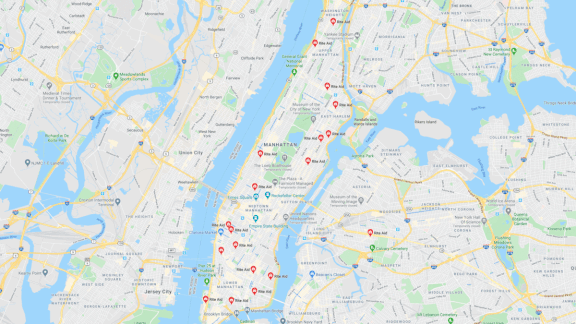
Retail Surveillance Revealed
A major retailer’s AI-powered surveillance program apparently targeted poor people and minorities.
What’s new: Rite-Aid, a U.S.-based pharmacy chain, installed face recognition systems in many of its New York and Los Angeles stores. Most of the locations were in low-income neighborhoods with large Black, Latino, or Asian populations, according to an analysis by Reuters. Rite-Aid terminated the program shortly before the report was published.
Who’s minding the store: The company had installed the systems in 200 locations nationwide, according to internal documents. Reporters spotted them in 33 of the 75 Rite-Aid stores they visited in the two cities.
- Security guards used a smartphone app to photograph customers caught misbehaving or acting suspiciously. The app sent the photos to a face recognition model.
- An in-store camera texted an alert to security whenever it recognized one of those people entering a Rite-Aid store.
- The retailer used the technology much more often in low-income, non-white neighborhoods. It didn’t install the system in some nearby stores that had similar shoplifting rates but were located in predominantly white neighborhoods.
- Some of the systems were sold by DeepCam, whose parent company is based in China. Civil liberties advocates worry that the company may send data on U.S. citizens overseas.
Behind the news: Several other large retailers in the U.S. have tested face recognition in recent years. Home Depot, Lowe’s, and Menards have been hit with class action lawsuits over the practice.
Why it matters: Face recognition has become a staple of security and law enforcement in the U.S. and elsewhere with very little public debate over limits on its use. The technology poses obvious threats to privacy. Moreover, research shows that it often makes mistakes, especially when it tries to identify people of color.
We’re thinking: Face recognition could be a powerful defense against shoplifting, but much work remains to be done to audit the accuracy, reliability, and fairness of commercial systems and formulate regulations that govern their use.
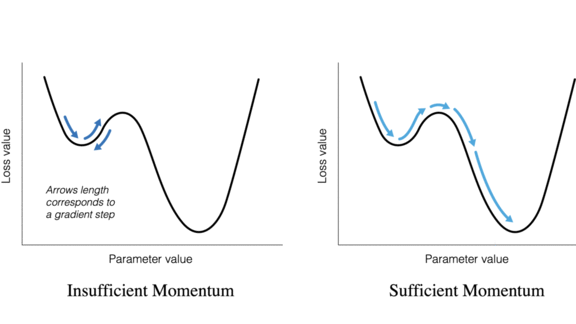
When Optimization is Suboptimal
Bias arises in machine learning when we fit an overly simple function to a more complex problem. A theoretical study shows that gradient descent itself may introduce such bias and render algorithms unable to fit data properly.
What’s new: Suriya Gunasekar led colleagues at Toyota Technical Institute at Chicago, University of Southern California, and Technion-Israeli Institute of Technology to demonstrate that a model’s predictions can be limited by its optimization method regardless of the volume or distribution of training data. In some cases, gradient descent can’t find the optimal solution even with all the data in the world.
Key insight: The researchers considered a model’s ability to learn the optimal solution given a particular optimization method and loss function, as well as sufficient training data. They divided loss functions into two categories inspired by (A) linear regression with more parameters than data samples and (B) logistic regression when data classes are separable. Loss functions in category A have obtainable minima: The optimum sits at the bottom of a valley. Those in category B don’t: The optimum lies at the bottom of an infinitely long downward slope. Considering these categories separately enabled the authors to prove results for a variety of optimization methods.
How it works: The researchers found that optimization hyperparameter values can limit the behavior of certain combinations of loss function and optimization method. A well known example: With enough momentum, gradient descent doesn’t get stuck in local minima, but with too little, it gets trapped.
- Linear regression with quadratic loss defines a convex optimization problem. On the other hand, in logistic regression, with some datasets, certain parameters must approach infinity to realize the optimum. The researchers measured bias in such scenarios by whether the optimizer could approach optimal performance given infinite time.
- In linear models with gradient descent, optimizing losses in category A will always reach the optimal solution. When optimizing losses in category B, how close a model comes to finding an optimal solution depends on how long it trains.
- A natural gradient descent optimizer updates weights by following the gradient and a defined flow (which encourages certain directions, similar to gravity). By extending their results from gradient descent, the researchers showed that natural gradient descent is also biased by the initialization.
- For particular combinations of optimization method and loss function, the researchers were unable to quantify the gap between a solution and the optimum. But they did prove that the bias depends on a model’s learning rate and initialization. In such cases, gradient descent may get stuck in the local minima depending on the starting location.
Results: In theory, gradient descent with loss functions in category A depends on initialization but not hyperparameters. Moreover, momentum depends on both initialization and hyperparameters. For category B, gradient descent always converges on the correct solution given infinite time.
Why it matters: Theory and practice sometimes diverge, but theoretical analyses help simplify practical issues and provide clear guidance when questions arise.
We’re thinking: How to select and tune optimization algorithms is often the realm of tribal knowledge. (We teach some of this in the Deep Learning Specialization.) We’re glad to have higher principles to consult as we debug architectures, datasets, and training methods.