Dear friends,
My father recently celebrated a milestone: He has completed 146 online courses since 2012. His studies have spanned topics from creative writing to complexity theory.
Ronald Ng is a great example of lifelong learning. For him, learning is not a task or a responsibility. It’s a joy. “The joy of learning helps keep the mind sharp and allows us to appreciate the beauty of the subject matter,” he says. “We need to remain mentally young and have the same sense of wonderment” we had as children.
And he’s not just taking online courses because he has nothing else to do. At age 74, he continues to work as a hematologist and serves as a court-appointed mediator in his spare time.
You never know when learning will show its true value. As a doctor, my father had a patient who suspected he had been poisoned by mercury. The patient’s blood work didn’t show any evidence of this. But my father recalled a course in forensic medicine from Nanyang Technological University, where he had learned that mercury accumulates in hair. He took a hair sample from the patient and found the toxic metal in it. Then he was able to treat the patient appropriately.
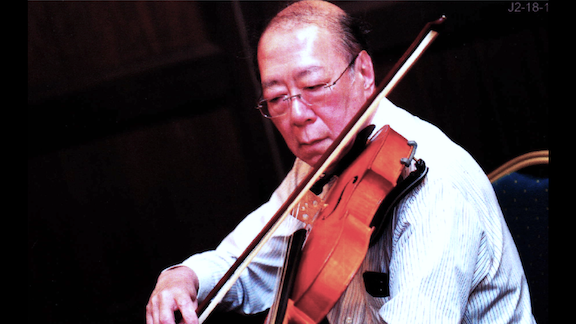
Growing up, I enjoyed having a father who played violin in the Hong Kong Philharmonic and followed the stars through a telescope on the roof of our apartment building. He taught me a lesson he learned as a volunteer in the army, where he discovered a truth that transcends the knowledge he gained studying subjects like military medicine and leadership: “We need very little in life to make us happy, provided we have the frame of mind to enjoy whatever we have.”
You can read an interview with him along with a list of courses he has taken here. I hope his story inspires you to keep learning until you are 74, and well past that, too.
Keep learning!
Andrew
News
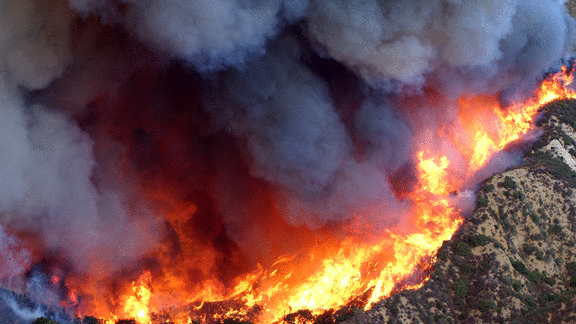
Mapping the Inferno
An AI-powered eye in the sky is helping firefighters control woodland blazes.
What’s new: California used maps drawn by neural networks to fight fires that threatened Yosemite National Park earlier this year, according to Wired. CalFire, the state’s firefighting agency, hopes the technology will help it better track wildfires, which can move quickly and erratically in windswept, mountainous terrain.
How it works: U.S. military drones provide California with aerial imagery that human analysts use to map fire perimeters. But that process can take hours. The Pentagon’s Joint AI Center hired San Francisco startup CrowdAI to build a model that converts flyover videos into wildfire maps in less than 30 minutes. CalFire plans to make the maps available to firefighters through a mobile app.
- The system trained on infrared videos from MQ-9 Reaper drones. Human annotators had labeled and geotagged fires in their frames.
- CrowdAI used a proprietary image segmentation model to outline a fire’s extent, the company’s chief executive Devaki Raj told The Batch.
- Human analysts check the model’s output before passing it along to firefighters.
Behind the news: A number of teams are working on AI systems designed to mitigate the impact of natural disasters.
- AI for Digital Response analyzes text and photos in Twitter to identify damaged infrastructure, calls for aid, and other relief-related topics. The platform has been used to evaluate damage of earthquakes and hurricanes, but it has yet to be used to respond to a crisis in real time.
- Disaster modeling startup One Concern, which uses AI to predict earthquake damage, works with several local U.S. governments and international financial institutions. However, critics have raised concerns about the system’s accuracy in predicting earthquake damage.
- NeurIPS 2020 will host a December workshop to bring together machine learning engineers and first responders.
Why it matters: Wildfires move fast, and maps that are even a few hours out of date can put people and property at risk. As climate change makes wildfires more frequent and more destructive, firefighters need tools that will help them combat blazes quickly and efficiently.
We’re thinking: DeepLearning.AI’s team in California has been experiencing the fallout from forest fires firsthand. We’re eager to see AI play a bigger role in disaster relief.
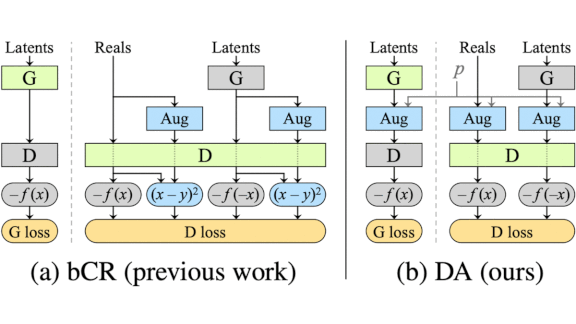
GANs for Smaller Data
Trained on a small dataset, generative adversarial networks (GANs) tend to generate either replicas of the training data or noisy output. A new method spurs them to produce satisfying variations.
What’s new: Tero Karras and colleagues at Nvidia developed Adaptive Discriminator Augmentation (ADA). The process enables GANs to train on small datasets without overfitting, or memorizing the training set, by strategically adding training images that have been augmented via cropping, rotating, color filtering, and so on. The trick is to add augmentations in the right proportion.
Key insight: GANs learn to generate the most common types of training examples. Likewise, when trained on augmented training images, they learn to mimic the most common modifications. The authors dynamically controlled the proportion of 18 different modifications to nudge a GAN toward variety without allowing it to fixate on any particular one.
How it works: The researchers trained a StyleGAN2 on subsets of the Flickr Faces High Quality (FFHQ) dataset.
- As the model trained, ADA tracked the degree to which it was overfitting. Every fourth minibatch, it estimated the proportion of training data classified as real. The higher the proportion, the higher the indication of overfitting.
- If more than 60 percent of the training data was judged realistic, the system increased the probability that modifications would be applied. Below 60 percent, the system lowered the chance of modifications.
- Each modification was applied separately according to the same probability.
Results: Trained on 2,000 images, ADA achieved a 16.71 Fréchet Inception Distance (FID), a measure of the difference between the non-generated input and generated output in which lower is better. This score is less than a quarter that of the StyleGAN2 baseline after training on 2,000 images (78.58 FID). Furthermore, it’s roughly half the StyleGAN2 baseline using 10,000 images (30.74 FID).
Why it matters: Gathering tens of thousands of images to train a GAN is a costly chore, but gathering a few thousand is more manageable. By lightening the cost and work involved in assembling training datasets, ADA could widen the utility of GANs in tasks where data is especially scarce.
We’re thinking: Anybody else want to use this to generate a new generation of Pokémon, or is it just us?
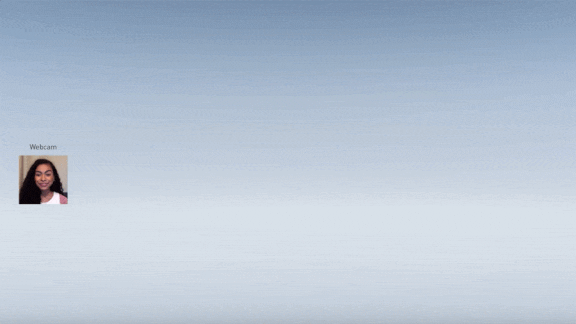
Data Compression By AI
In this work-from-home era, who hasn’t spent a video conference wishing they could read an onscreen document without turning their eyes from the person they’re talking with? Or simply hoping the stream wouldn’t stutter or stall? Deep learning can fill in the missing pieces.
What’s new: Maxine is a media streaming platform from Nvidia. It replaces compression-decompression software with neural networks, using one-tenth the typical H.264 bandwidth. It can also enhance resolution to transmit a sharper picture, alter the video image in useful and creative ways, and deliver additional audio and language services.
How it works: Maxine is available to video conference providers through major cloud computing vendors. This video illustrates some of the system’s capabilities. Avaya, which plans to implement some features in its Spaces video conferencing app, is the only customer named so far.
- Rather than transmitting a river of pixels, a user’s computer sends periodic keyframes along with locations of facial keypoints around expressive areas like the eyes, nose, and mouth.
- A generative adversarial network (GAN) synthesizes in-between frames, generating areas around the keypoints. In addition, the GAN can adjust a speaker’s face position and gaze or transfer keypoint data into an animated avatar that speaks in the user’s voice while mimicking facial expressions. The GAN is trained to work with faces wearing masks, glasses, hats, and headphones.
- Other models manage audio services such as conversational chatbots and noise filtering, as well as language services such as automatic translation and transcription.
Why it matters: The volume of video data on the internet was growing exponentially before the pandemic hit, and since then, video conferencing has exploded. Neural networks can reclaim much of that bandwidth and boost quality in the bargain, scaling up the resolution of pixelated imagery, removing extraneous sounds, and providing expressive animated avatars and informative synthetic backgrounds.
We’re thinking: AI is working wonders for signal processing in both video and audio domains. Streaming is great, but also look for GANs to revolutionize image editing and video production.
A MESSAGE FROM DEEPLEARNING.AI
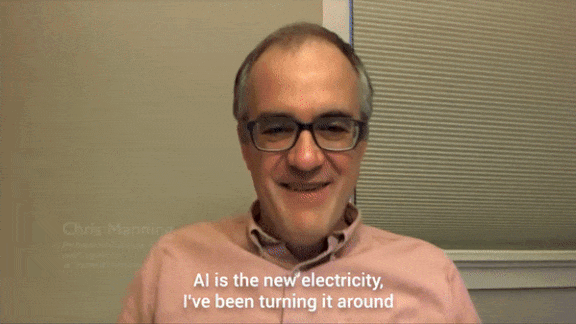
We’re thrilled to present Heroes of NLP, a DeepLearning.AI video series featuring Andrew Ng in conversation with leaders in natural language processing. Get expert advice and perspective from Chris Manning, Kathleen McKeown, Oren Etzioni, and Quoc Le. As Chris Manning says: “There are huge opportunities in industry and academia for people with AI, ML, and NLP skills. You’d be greatly in demand, so this is a great thing to do!” Watch here
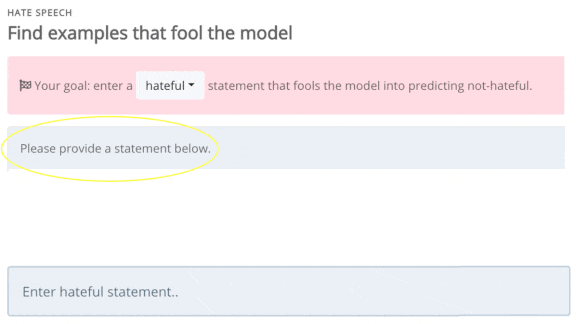
Dynamic Benchmarks
Benchmarks provide a scientific basis for evaluating model performance, but they don’t necessarily map well to human cognitive abilities. Facebook aims to close the gap through a dynamic benchmarking method that keeps humans in the loop.
What’s new: Dynabench is an online platform that invites users to try to fool language models. Entries that prompt an incorrect classification will become fodder for next-generation benchmarks and training sets.
How it works: The platform offers models for question answering, sentiment analysis, hate speech detection, and natural language inference (given two sentences, decide whether the first implies the second). A large team spanning UNC-Chapel Hill, University College London, and Stanford University built the models.
- Users choose a task and enter a tricky example, and the model renders a classification. For instance, we misled the sentiment analyzer into classifying the following restaurant review as positive: “People who say this pizza is delicious are wonderfully deluded.”
- Alternatively, users can validate examples entered by other people. Validation involves reading entries and flagging whether the model’s classifications are true or false.
- The platform adds misclassified examples to a dataset that researchers will use to retrain the model. Then the cycle begins anew, as users try to stump the updated model.
- Facebook plans to open the platform to all kinds of tasks, inviting model builders to upload new tasks and interested parties to find ways to reveal their weaknesses.
Yes, but: The new method is plainly experimental. “Will this actually work?” the Dynabench FAQ asks. Answer: “Good question! We won’t know until we try.”
Behind the news: Facebook’s engineers were inspired by earlier efforts to test AI via humans in an adversarial role including Beat the AI, Build It Break It, and Trick Me If You Can.
Why it matters: AI exceeds human performance across a range of standardized benchmarks, and Facebook points out that the time between a benchmark’s debut and a model outdoing the human baseline is getting shorter. Yet the technology clearly falls short of human smarts in many everyday tasks. Benchmarks that better reflect human abilities are bound to drive more rapid progress.
We’re thinking: Social media companies are working to build filters to screen out hateful or misleading speech, but adversaries keep finding ways to get through. A crowdsourcing platform that lets humans contribute deliberately adversarial examples is worth trying.
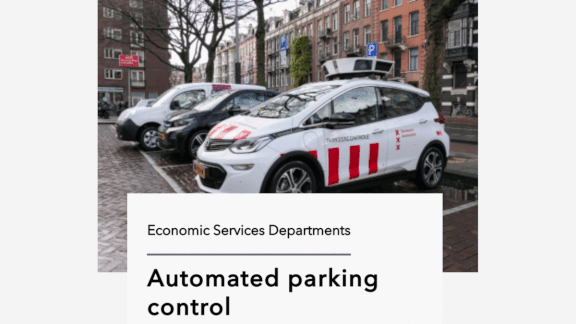
Transparency for Smart Cities
Two European capitals launched public logs of AI systems used by the government.
What’s new: Amsterdam and Helsinki provide online registries that describe the algorithms that govern municipal activities, such as automated parking control and a public health chatbot. The registries are currently in beta testing.
How it works: Entries in the registry describe what each model does and how it was trained, as well as contact information for the official responsible for deploying it.
- Amsterdam defines AI as software that “makes predictions, decisions, or gives advice by using data analysis, statistics, or self-learning.” Helsinki’s registry includes machine learning systems but also simpler programs that the public may consider AI, but experts may not, a spokesperson told The Batch.
- The registries won’t be comprehensive, since city agencies aren’t required to comply. “For now, government agencies are doing it voluntarily for building and retaining trust with their citizens,” Meeri Haataja, CEO of Saidot, the Finnish startup that developed the registers, told The Batch.
- Both cities plan to refine the rules for registration based on user feedback. They also offer ethical statements that describe their priorities for municipal AI.
Why it matters: Budding smart cities will be smarter if everyone has a way of knowing which algorithms are doing what. Documentation is essential when decisions made by automated systems raise questions or when models need to be updated to account for changing circumstances.
We’re thinking: Government investment in AI will be squandered if citizens don’t trust the technology. For instance, Google’s Sidewalk Labs project, which sought to outfit a swath of property in Toronto with sensors, foundered partly on public worries over the handling of the data they would collect. Transparency is crucial for productive public implementation of AI.
A MESSAGE FROM DEEPLEARNING.AI
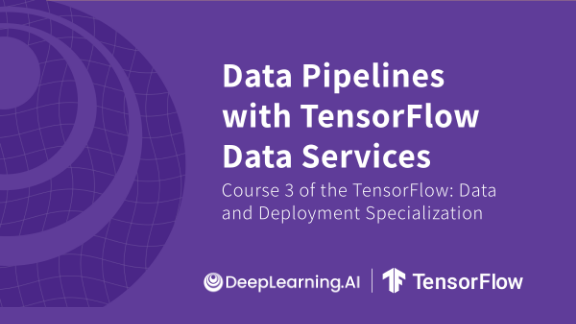
The TensorFlow: Data and Deployment Specialization just got better!
We refreshed “Course 3: Data Pipelines with TensorFlow Data Services” with updated lectures, quizzes, and assignments that reflect recent changes in the TensorFlow API. We also revised Week 4 assignments to ensure that you’re well prepared to apply the principles you’ve learned. Enroll now