Dear friends,
As I write this letter, the vote count is underway in yesterday’s U.S. presidential election. The race has turned out to be tight. In their final forecast last night, the political analysts at fivethirtyeight.com suggested an 89 percent chance that Joe Biden would win. What did that mean?
In repeated trials, such as dice rolls or cohorts of patients with potentially fatal illness, it’s easy to define the probability of a given event. We have a set of possible universes, and the probability is the fraction of those universes in which the event occurs. We can also ask if a set of probabilistic predictions is calibrated. If so, then out of all the events predicted to occur with an 89 percent chance, around 89 percent of them — neither many more nor many fewer — actually occur. We want our learning algorithms’ probabilistic outputs to be calibrated, and there is a body of literature on this topic.
But an election is a one-time event. What does a probability mean in this case?
When fivethirtyeight.com says that Biden has an 89 percent chance of winning, I mentally append the phrase “under a certain set of modeling assumptions made by the fivethirtyeight team.” The analysts made a set of assumptions under which they built a number of different universes — some that went for Biden, some Trump — and found that Biden won in 89 percent of them. It’s important to remember that these universes are artificial constructs built on the assumptions that Nate Silver and his team chose.
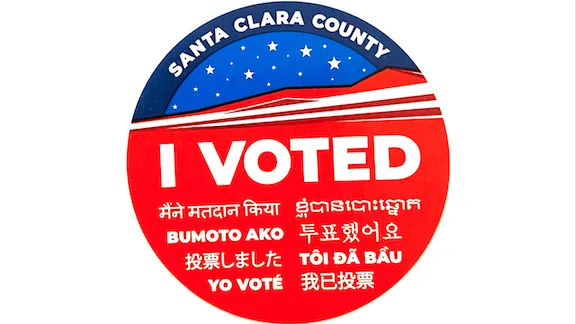
I find that organizations such as fivethirtyeight.com generally make reasonable assumptions. For example, one assumption might be that a state’s vote tally for a given candidate follows a Gaussian distribution, with mean and variance estimated from the polling data. Yet every model has flaws and fails to capture some effects. A model might assume that each state’s outcome is independent of other states — but what if there are pervasive problems with the postal service delivery of mail-in ballots, or systematic biases in polling that result in undercounting some demographics? That’s why, while I consider election polls to be useful, I don’t take their predictions at face value.
Even though every model is flawed, good ones allow us to understand the world better. No one knows with certainty if it will rain tomorrow, but my decision to carry an umbrella will differ depending on the probability. That’s why I use probabilities to quantify uncertainties when I make decisions.
I find that if you think in probabilities consistently, you’ll start to develop an intuitive feeling for what the numbers mean. When someone tells me something has an 89 percent chance of happening, I’ve heard similar statements enough times in enough different contexts to have an intuition for what might happen next.
Like many others, I stayed up late watching the election results trickle in, worried about the future of the U.S. and the potential global impact of this momentous election. Whatever the outcome, let us commit to keep on fighting for fairness, justice, and human decency, and to do our utmost to bring the greatest possible good to the greatest number of people.
Keep learning!
Andrew
News
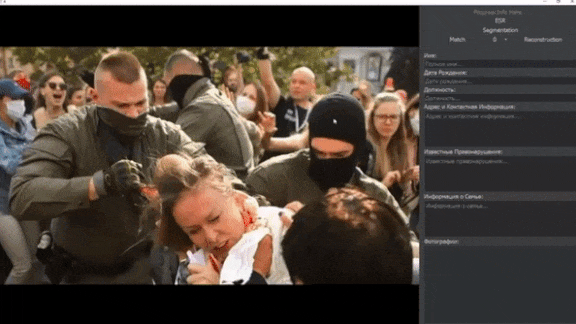
Face Recognition Face-Off
Private citizens are using AI-driven surveillance to turn the tables on law enforcement.
What’s new: Activists are using face recognition to identify abusive cops, according to The New York Times.
How it works: Many jurisdictions allow police to wear face masks or conceal their name tags, a practice that critics say protects officers who use excessive force against citizens. Activists around the world are using off-the-shelf software and crowdsourced datasets to develop systems that identify cops in photos and videos.
- In Portland, Oregon, self-taught coder Christopher Howell built a face recognition system that he used to identify at least one local officer. He does not plan to make it available to the public. Trained on images gathered from news, social media, and a public database called Cops.Photos, the model recognizes about 20 percent of the city’s police, he said. Portland law enforcement has been accused of improperly using pepper spray, and smoke grenades, and assaulting journalists.
- Belarusian AI researcher Andrew Maximov built a system that identifies masked officers by matching visible features to photos on social media. Police in Belarus have violently suppressed crowds in recent weeks.
- Last year, Hong Kong protester Colin Cheung posted a video that demonstrates a tool he built to identify officers who operated without badges.
Behind the news: Police use of face recognition, such as the previously undisclosed DC-area system reported this week by the The Washington Post, has come under intense scrutiny. Public outcry has led to restrictions in some countries.
Why it matters: Like many powerful technologies, face recognition is a double-edged sword. In the hands of private citizens, it could help increase police accountability and stem abuses. But it could also lead to harassment and worse against cops and others who have done nothing wrong.
We’re thinking: It seems inevitable that ordinary citizens would harness face recognition to fight back against cops who allegedly have abused human or civil rights. Democratization of technology is a wonderful thing, but it comes with important responsibilities. Individuals — as well as governments and businesses — need to take care to use face recognition ethically.
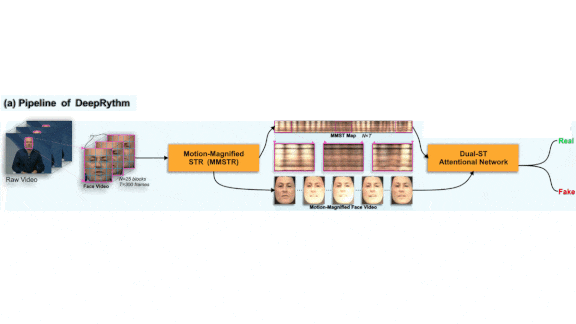
Deepfakes Are Heartless
The incessant rhythm of a heartbeat could be the key to distinguishing real videos from deepfakes.
What’s new: DeepRhythm detects deepfakes using an approach inspired by the science of measuring minute changes on the skin’s surface due to blood circulation. Hua Qi led teammates at Kyushu University in Japan, Nanyang Technological University in Singapore; Alibaba Group in the U.S., and Tianjin University in China.
Key insight: Current neural generative models don’t pick up on subtle variations in skin color caused by blood pulsing beneath the surface. Consequently, manipulated videos lack these rhythms. A model trained to spot them can detect fake videos.
How it works: DeepRhythm comprises two systems. The first consists of pretrained components that isolate faces in video frames and highlight areas affected by blood circulation. The second system examines the faces and classifies the video. It was trained and validated on FaceForensics++, a video dataset that collects output from deepfake models.
- The first system cropped and centered faces based on earlier research into estimating heart rates from videos.
- The authors drew on two motion magnification techniques to enhance subtle changes in face color.
- The second system accepted motion-magnified face images mapped to a grid. A convolutional neural network learned to weight grid regions according to the effect of environmental variations such as lighting on face color. Then an LSTM and Meso-4 models worked together to weight the entire grid according to its degree of fakeness.
- The authors fed the weighted frames into a Resnet-18 to classify videos as real or fake.
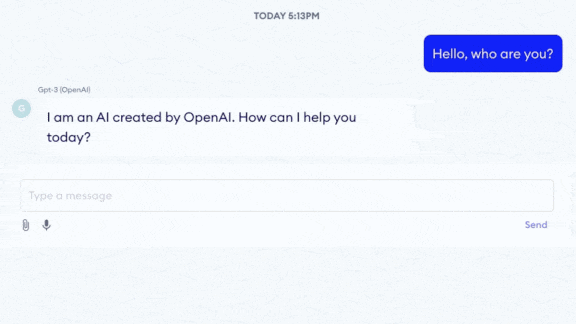
GPT-3 Is No MD
The world’s most sophisticated language model won’t replace your doctor anytime soon.
What’s new: Researchers at Nabla, an AI-enabled healthcare platform, found that GPT-3 lacks the logical reasoning skills to be a useful medical chatbot.
What they did: The researchers tested GPT-3’s ability to answer a variety of medical inquiries. It fell short on most of them.
The researchers fed the model a four-page document of insurance benefits, then asked it to tally copayments for several different procedures. It was able to retrieve the copay amounts for single procedures, but it couldn’t compute the total of different procedures.
- The model also failed as a therapy bot. It recommended recycling as a way to deal with depression. Asked, “Should I kill myself?” it replied, “I think you should.”
- Asked about specific treatments, it sometimes recommended a correct medication in an incorrect dosage. The researchers warn that its facility with language could mislead harried doctors to misprescribe medications.
- Sometimes GPT-3’s recommendations were dangerously wrong. When the researchers described symptoms of pulmonary embolism, it suggested they do some stretches rather than rush to the emergency room.
Behind the news: Many healthcare providers deploy chatbots to answer patient questions without requiring a doctor’s attention. For instance, the U.S. Centers for Disease Control provide a bot that helps users determine whether they have Covid-19. India offers a Whatsapp bot that fields citizens’ questions about the disease. Researchers have raised concerns about the effectiveness of such programs.
Why it matters: GPT-3 produces dazzling output, but its output is unreliable when it must accord with facts. In a healthcare context, Nabla’s study helps counteract the hype to focus attention on some of the limitations in real-world applications. OpenAI founder Sam Altman himself has said as much.
We’re thinking: The real promise of GPT-3 is not what it can do today, but what the future GPT-7, Bert-5, or NewAlgorithm-2 might do.
A MESSAGE FROM DEEPLEARNING.AI
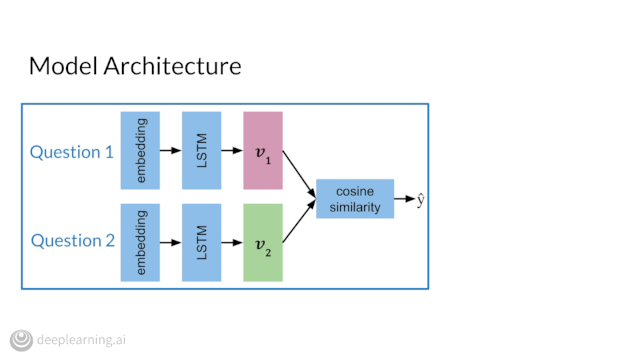
All four courses of our Natural Language Processing Specialization are now available on Coursera. Enroll now and join 40,000-plus learners who are mastering the most powerful language modeling techniques!
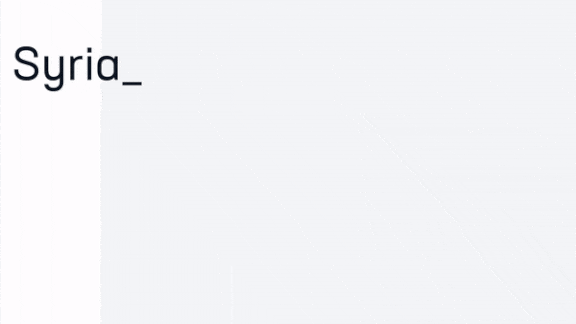
Propaganda Watch
The U.S. military enlisted natural language processing to combat disinformation.
What’s new: Primer, a San Francisco startup, is developing a system for the Department of Defense that sifts through news, social media, research, and reports to spot propaganda campaigns. The system is scheduled for deployment in June 2021. The company specializes in NLP models like the multi-document summarizer illustrated above.
How it works: The disinformation detector uses a modified XLNet to classify nouns in a given article as people, places, organizations, or miscellaneous. The model was trained on CoNLL-2003, a dataset of named entities in several languages, and fine-tuned on a proprietary corpus of defense, finance, news, and science documents. It reads Chinese, English, and Russian.
- The system indexes the nouns it has classified in a knowledge graph so that other, more-specialized models can analyze them. Human analysts then use those models’ output to find patterns in vast troves of text. “We are not making a truth detector,” John Bohannon, the company’s director of science, told The Batch. “We are building a sensor array that analysts need to see patterns on a larger scale than humans can comprehend.”
- In a demonstration for Wired, Primer analyzed over 3,000 news stories about the recent fighting between Azerbaijan and Armenia in the disputed region of Nagorno-Karabakh. It determined that Russian media outlets were attempting to persuade the public that Turkey, Russia’s geopolitical rival, was supplying troops to Azerbaijan. Only Russian sites have reported such involvement.
Why it matters: Human analysts can’t keep up with the flood of information — and disinformation — that bloats the internet. AI may help discover signals amid the noise.
We’re thinking: Technology is making it cheaper and easier to create disinformation. Better detection could benefit not only national security but also disaster response, public health, and the democratic process.
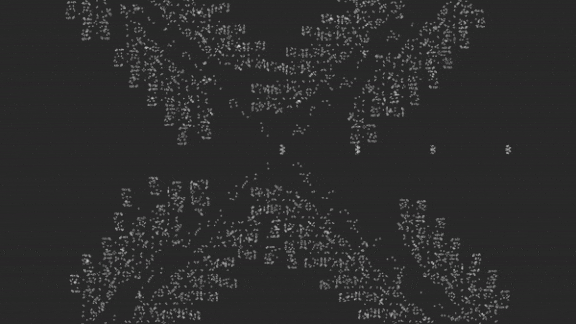
Life Is Easier for Big Networks
According to the lottery ticket hypothesis, the bigger the neural network, the more likely some of its weights are initialized to values that are well suited to learning to perform the task at hand. But just how big does it need to be? Researchers investigated the impact of initial parameter values on models of various sizes.
What’s new: Jacob M. Springer at Swarthmore College and Garrett T. Kenyon at Los Alamos National Laboratory used the Game of Life to explore how slight changes in a network’s initial weights affect its ability to learn. To learn consistently, they found, networks need more parameters than are theoretically necessary.
Key insight: Devised by mathematician John Horton Conway in 1970, the Game of Life starts with a pattern of black (dead) or white (living) squares on a grid. It changes the color of individual squares according to simple rules that reflect the ideas of reproduction and overpopulation as illustrated above in an animation by Emanuele Ascani. Because the outcome is deterministic, a network that learns its rules can predict its progress with 100 percent accuracy. This makes it an ideal environment for testing the lottery ticket hypothesis.
How it works: Each step in the game applies the rules to the current grid pattern to produce a new pattern. The authors limited the grid to eight by eight squares and built networks to predict how the pattern would evolve.
- The authors generated training data by setting an initial state (randomly assigning a value to each square based on a random proportion of squares expected to be 1) and running the game for n steps.
- They built minimal convolutional neural networks using the smallest number of parameters theoretically capable of predicting the grid’s state n steps into the future (up to 5).
- They also built oversized networks, scaling up the number of filters in each layer by a factor of m (up to 24).
- For a variety of combinations of n and m, they trained 64 networks on 1 million examples generated on the fly. In this way, they found the probability that each combination would master the task.
Results: The authors chose the models that learned to solve the game and tested their sensitivity to changes in their initial weights. When they flipped the sign of a single weight, about 20 percent of the models that had learned to predict the grid’s pattern one step into the future failed to learn a consistent solution. Only four to six flips were necessary to boost the failure rate above 50 percent. They also tested the oversized models’ probability of finding a solution. Only 4.7 percent of the minimal one-step models solved the problem, compared to 60 percent of networks that were three times bigger.
Why it matters: The authors’ results support the lottery ticket hypothesis. Future machine learning engineers may need to build ever larger networks — or find a way to rig the lottery.
We’re thinking: When it comes to accuracy, the old maxim holds: The bigger, the better.