Dear friends,
The rise of AI creates opportunities for new startups that can move humanity forward. In the 1990s, the internet was embraced successfully by incumbent companies including Apple and Microsoft, but it also inspired hugely impactful startups like Amazon, Facebook, and Google. Similarly, AI now is empowering forward-looking incumbent companies — many of them former internet startups — and creating massive opportunities for new startups as well.
I’ve been thinking about what I can do to help members of the DeepLearning.AI community who wish to create a company. At AI Fund (where I am managing general partner), I speak with many entrepreneurs who have either started or are thinking of starting a new company. I’ve noticed a few factors that increase the odds of success:
- Domain knowledge coupled with identification of a problem: Do you deeply understand an industry and a specific pain point? Have you experienced and struggled with solving the problem yourself?
- Initial hypothesis of a solution: Do you have a sense that AI-based automation can lead to a solution? Is it technically feasible and likely to solve the problem in a responsible and value-creating way?
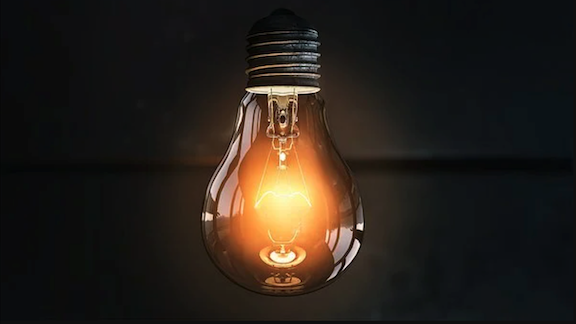
- Large market opportunity: Is there a large number of potential customers who have a similar problem?
- Drive and grit: Startups move forward only because the people involved make it happen. Are you ready to struggle through the hard work, pain, and uncertainty that comes with starting a company?
Many startup founders quietly obsess about startup ideas for years, since it can take a lot of thought and investigation to work out the nuances. (Before I cofounded Coursera, I had spent about five years obsessing over how to deliver effective online education. You can read more about my early experiences in “Origins of the Modern MOOC.”)
Identifying a problem is one of the hardest steps. I didn’t understand this until I saw a lot of examples. So many things compete for attention in today’s world (in both business-to-business and business-to-consumer settings) that unless your offering creates compelling value, it’s hard to get people to pay attention. One test of a problem you’ve identified is: Have a number of people told you they would go to the trouble of exploring possible solutions?
I’d love to hear from those of you who are, or aspire to become, entrepreneurs. My teams at DeepLearning.AI and AI Fund plan to hold a series of entrepreneur-oriented events next year. If the success factors I listed above describe you, and especially if you’re still in the early stages (say, from having identified a problem but not yet decided to start a company to having built a product and being ready to raise capital), please take this short survey and let us know how we can help you in your startup journey.
Keep learning!
Andrew
News
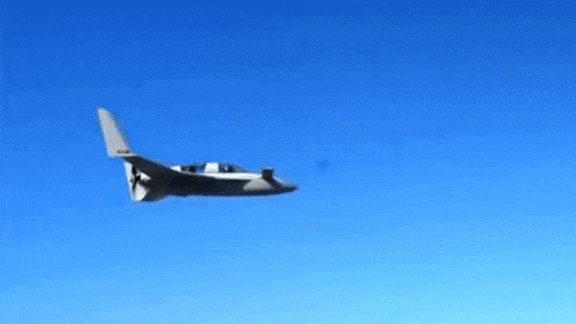
Phantom Menace
A fighter pilot battled a true-to-life virtual enemy in midair.
What’s new: In the skies over southern California, an airman pitted his dogfighting skills against an AI-controlled opponent that was projected onto his augmented-reality visor.
How it works: The trial aimed to test the integration of an autonomous fighter agent developed by EpiSci with high-brightness, low-latency, augmented-reality technology from Red Six Aerospace.
- Red Six CEO Dan Robinson, an alumnus of the UK’s Royal Air Force, piloted a plane of his own design. EpiSci controlled a simulated Chinese J-20 stealth fighter using a combination of deep learning, reinforcement learning, and rules-based modeling.
- EpiSci’s agent previously ran on ground-based hardware in a simulation. The trial confirmed that it ran well on the resources available in the Red Six craft and responded to real-world input from GPS and inertial sensors, Chris Gentile, EpiSci’s VP of tactical autonomous systems, told The Batch.
- The event also confirmed that EpiSci could limit its agent to behaviors useful for training beginners — “It wasn’t kill-at-any-cost,” Gentile said — without compromising its ability to react to its human opponent’s tactics and errors. The U.S. Air Force plans to begin testing the system for pilot training next year.
Behind the news: EpiSci honed its agent technology in the U.S. Defense Advanced Research Projects Agency (Darpa) Alpha Dogfight program, in which a pilot on the ground helmed a flight simulator to fight AI-controlled foes. (See our report on the program, “AI Versus Ace.”) Darpa recently awarded the company a grant to develop AI systems for air combat.
Why it matters: Flight simulators don’t replicate all the challenges pilots face in the air — for instance, G-forces — and pitting human pilots against one another in the air is dangerous and expensive. Battling AI-controlled agents in augmented reality could make combat training more effective, safer, and cheaper.
We’re thinking: The ethical boundaries of military AI demand careful navigation. Using machine learning to make training pilots safer may be a reasonable application. Building aircraft that can fight on their own, however, is a different matter. The AI community needs to draw bright red lines to ensure that AI is beneficial and human. To that end, we support the United Nations proposed ban on autonomous weapons.
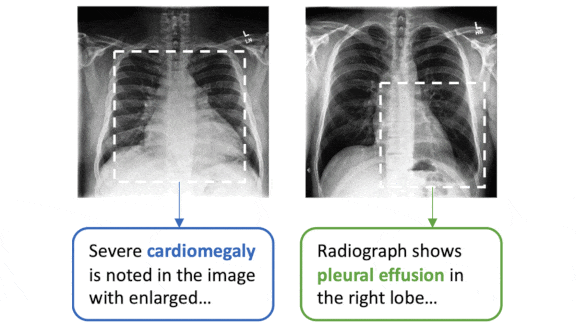
Learning From Words and Pictures
It’s expensive to pay doctors to label medical images, and the relative scarcity of high-quality training examples can make it hard for neural networks to learn features that make for accurate diagnoses. A new method addresses the issue by training a feature extractor on both X-rays and text that accompanies them.
What’s new: Yuhao Zhang and colleagues at Stanford University proposed ConVIRT, a method that uses contrastive learning to learn from unlabeled images paired with corresponding text reports. The effort brought together medical imaging specialist Curt Langlotz and natural language processing luminary Chris Manning (see our Heroes of NLP interview with him here).
Key insight: The text report that accompanies a medical image contains useful information about the image’s contents, and vice-versa. ConVIRT generates features based on similarities between images and corresponding reports, as well as differences between images and unrelated reports.
How it works: The authors built separate pipelines for images and text. The image pipeline consisted of a ResNet-50, followed by a neural network with a single hidden layer (to project the image vectors into a consistent space for comparison with the text vectors}. The text pipeline consisted of BERT followed by a similarly shallow network.
- The researchers used two datasets for pretraining: the MIMIC-CXR database of 217,000 chest X-rays and reports and a Rhode Island Hospital dataset of 48,000 musculoskeletal images with reports.
- They pretrained the models on the image-text pairs using a contrastive loss: The image pipeline learned to produce a vector as similar as possible to the corresponding vector produced by the text pipeline, and different from all the other text vectors. The text pipeline learned in a similar way.
- They extracted the ResNet-50 model and fine-tuned it for four image classification tasks, including the RSNA Pneumonia Detection Challenge of diagnosing pneumonia in chest X-rays.M
Results: In all four tasks, ConVIRT outperformed baseline models including a ResNet-50 pretrained on ImageNet and fine-tuned on RSNA and other datasets, and custom models built to generate the paired text from an image. Fine-tuned on 1 percent of the RSNA dataset, ConVIRT achieved 88.8 AUC (area under the receiver operating characteristic curve, higher is better), compared to the ImageNet model (83.1 AUC) and the best custom image-text model (87.7 AUC). Fine-tuned on 10 percent of RSNA, ConVIRT outperformed those models 91.5 AUC to 87.3 AUC and 89.9 AUC respectively.
Why it matters: Pretraining on paired images and text via contrastive learning could help alleviate the high cost of medical data for deep learning.
We’re thinking: For updates on leading-edge AI for medicine, check out the new AI Health Podcast cohosted by Pranav Rajpurkar, instructor of our AI For Medicine Specialization.
A MESSAGE FROM DEEPLEARNING.AI
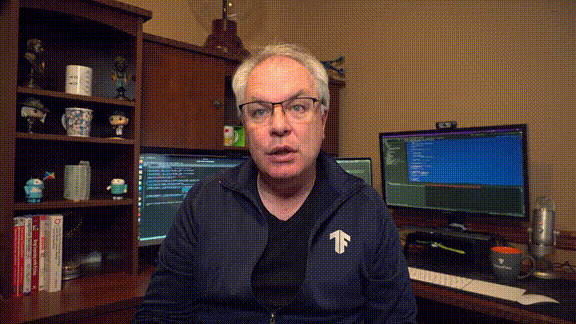
We’re excited to announce that “Advanced Computer Vision with TensorFlow,” Course 3 of the TensorFlow: Advanced Techniques Specialization, will launch on December 9th. Pre-enroll now
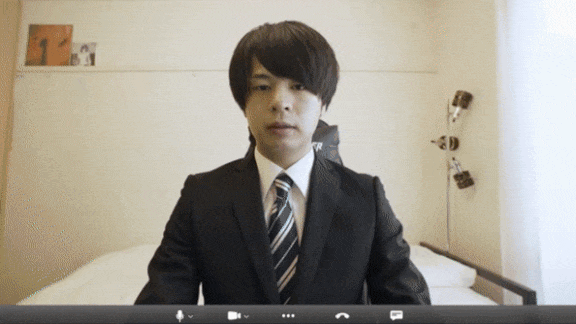
GAN Makes Pajamas Safe For Work
A new camera app uses a generative adversarial network to let users look like they’re dressed for success while they videoconference in their jammies.
What’s new: Xpression is an iPhone app that maps facial expressions onto still images in real time, allowing users to stream live video selfies clothed in digital costumes.
How it works: The app uses three deep learning models, a spokesperson for app maker EmbodyMe told The Batch.
- The first model estimates three-dimensional face shapes and expressions from the source video. The second does the same with the target image, whether it be a work of art, an anime character, or a selfie dressed for success. Then a generative adversarial network (GAN) maps the source frames to the target.
- The software works with video platforms including Zoom, Twitch, Microsoft Teams, and Google Meet. It can also be used to make YouTube videos.
- The app is available to iOS users as a beta version here.
Behind the news: Computer vision networks aren’t the only models helping socially distanced workers stay productive and presentable.
- Otter.ai uses natural language processing to provide real-time captions and translations for Zoom meetings.
- Microsoft Teams’ AI-powered noise suppression feature mutes crinkling snack wrappers, clacking keyboards, and other distracting desktop din.
Why it matters: No more judgement for our rumpled work-from-home looks and untidy bedrooms!
We’re thinking: Apps like these are a lot of fun, and we’re excited to see how they will develop. But they also take us one step further into a world where it is increasingly hard to determine what, and who, is real. Society needs better and more consistent standards for labelling digital fakery.
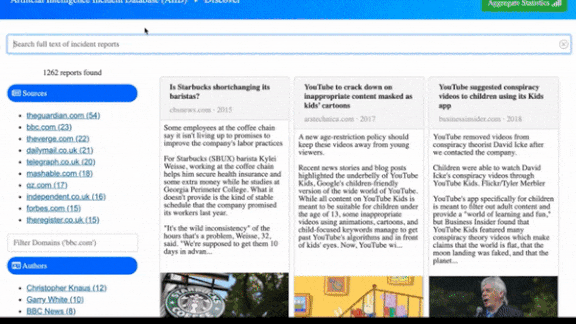
Cataloging AI Gone Wrong
A new database tracks failures of automated systems including machine learning models.
What’s new: The Partnership on AI, a nonprofit consortium of businesses and institutions, launched the AI Incident Database, a searchable collection of reports on the technology’s missteps. Examples include a gender-biased recruiting system, a worrisome recommender algorithm for children, and face recognition that led to wrongful arrests.
How it works: Users can submit descriptions of incidents based on media reports. Editors determine whether to include a given report as a new incident or an addition to a previously reported one.
- The database currently includes 1,174 unique articles covering 77 incidents, project lead Sean McGregor told The Batch.
- Users can query the archive using keywords and narrow searches by story source, author, and submitter.
- The database’s definition of AI includes machine learning as well as symbolic systems and deterministic algorithms, such as the flight control system that contributed to deadly crashes of two Boeing 737 Max aircraft.
Behind the news: Some independent researchers maintain similar lists of AI misfires. Those efforts, however, are not as comprehensive nor as easy to search.
Why it matters: AI failures can cause real harm. To avoid them, we need to learn from past mistakes.
We’re thinking: Incident reports are a well established tool in industries like aviation and cybersecurity. Keeping track of which systems failed, and how and when they did, is just as crucial in AI. The Partnership on AI’s vetting process should help to ensure that incident reports represent genuine problems rather than cherry-picked cases in which AI made a headline-grabbing mistake on a single input example.