Dear friends,
So many people who are just starting out in machine learning are doing amazing work. With online education, open source software, and open publications, it takes less time than ever to go from 0 to 100 in ML.
I spoke with one such person this week, Christine Payne, to learn about her journey. One year ago, she took the Deep Learning Specialization course. Now she's building cutting-edge neural networks at OpenAI. You can watch our conversation in the video linked below.
I’d love to hear from more of you who took our courses and now use AI in your own career. Let us know what you’re building. Send a note to [email protected].
Keep learning,
Andrew
DeepLearning.AI Exclusive
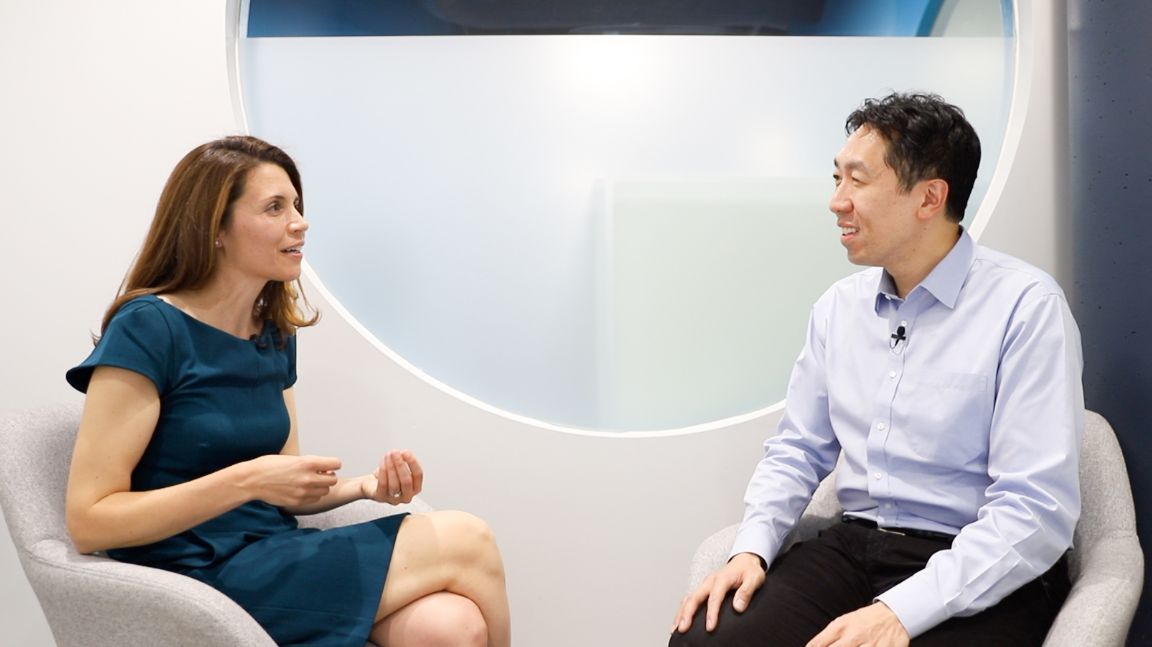
Ones to Watch: Christine Payne
Christine began in physics, moved into medicine, and did a stint as a professional musician. At OpenAI, she led development of MuseNet, a deep learning system that spins melodies and harmonies in a variety of styles. Watch the video
News
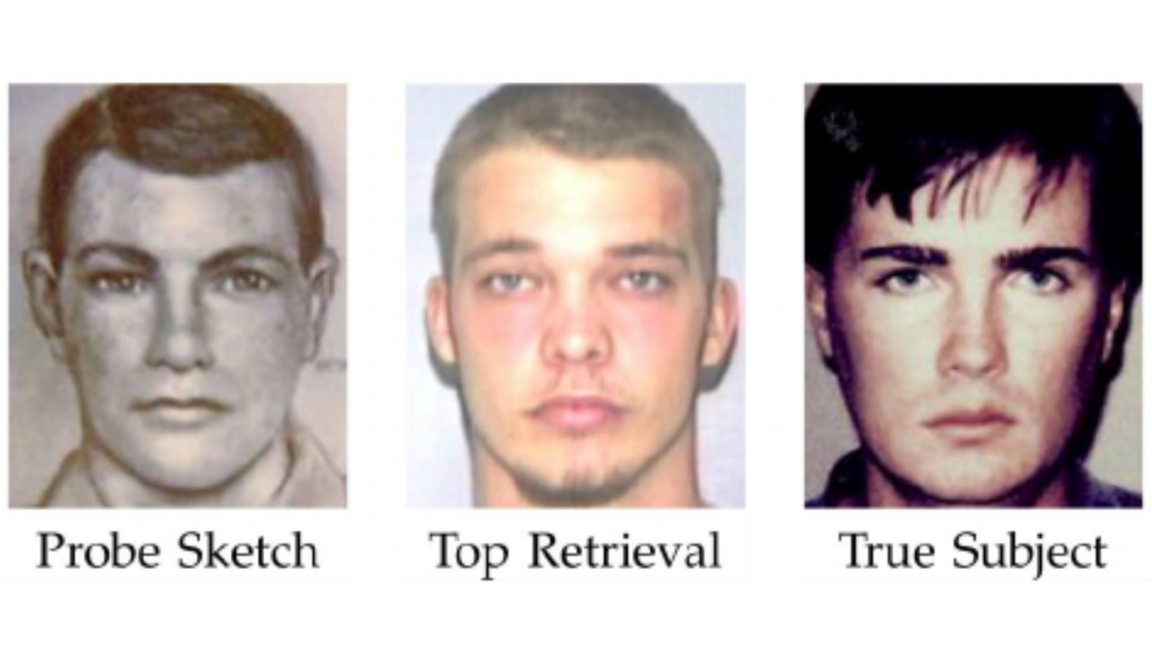
Face Recognition in the Crosshairs
Unfounded worries over malevolent machines awakening into sentience seem to be receding. But fears of face recognition erupted last week — the rumblings of a gathering anti-surveillance movement.
What's new: Recent events cast a harsh light on the technology:
- San Francisco banned use of face recognition systems by municipal agencies including law enforcement. Two other California cities, Oakland and Berkeley, are considering bans. So is Somerville, Massachusetts.
- Investors in Amazon, whose Rekognition system identifies faces as a plug-and-play service, are pressing the company to rein in the technology.
- The U.S. House of Representatives scheduled a hearing on the technology’s implications for civil rights.
- The Georgetown Law Center on Privacy & Technology published two reports sharply critical of law-enforcement uses of such technology in several U.S. states, detailing a variety of apparent misuses and abuses.
Backstory: Face recognition is still finding its way into industry — Royal Caribbean reportedly uses it to cut cruise-ship boarding time from 90 to 10 minutes — but it has quietly gained a foothold in law enforcement:
- Authorities in Chicago, Detroit, New York, Orlando, and Washington, DC, have deployed the technology. Los Angeles, West Virginia, Seattle, and Dallas have bought or plan to buy it.
- But police departments are using it in ways that are bound to lead to false identification.
- New York detectives searching for a man they thought looked like actor Woody Harrelson searched for faces that matched not the suspect, but Harrelson, according to the Georgetown Law Center.
- A handful of police departments in the U.S. permit searching for faces that match hand-drawn sketches based on witness descriptions, although such searches “mostly fail,” according to the National Institute of Standards and Technology.
Why it matters: Face recognition has a plethora of commercial uses, and law enforcement has employed it productively in countless cases. However, critics see potential chilling effects on free speech, erosion of privacy, reinforcement of social biases, risk of false arrest, and other troubling consequences. Now is the time to study possible restrictions, before the technology becomes so thoroughly embedded that it can’t be controlled.
What they’re saying: “People love to always say, ‘Hey, if it's catching bad people, great, who cares,’ until they're on the other end.” — Joshua Crowther, a chief deputy defender in Oregon, quoted by the Washington Post
Smart take: Face recognition, whether it's used in the public or private sphere, has tremendous potential for both good and ill. The issue isn’t bad technology, but misuse. It’s incumbent on AI companies to set bright-line standards for using their products and to build in ready ways of enforcing those standards. And it’s high time for government agencies to hammer out clear policies for using the tech, as well as audit processes that enable the public to evaluate whether those policies are being met.
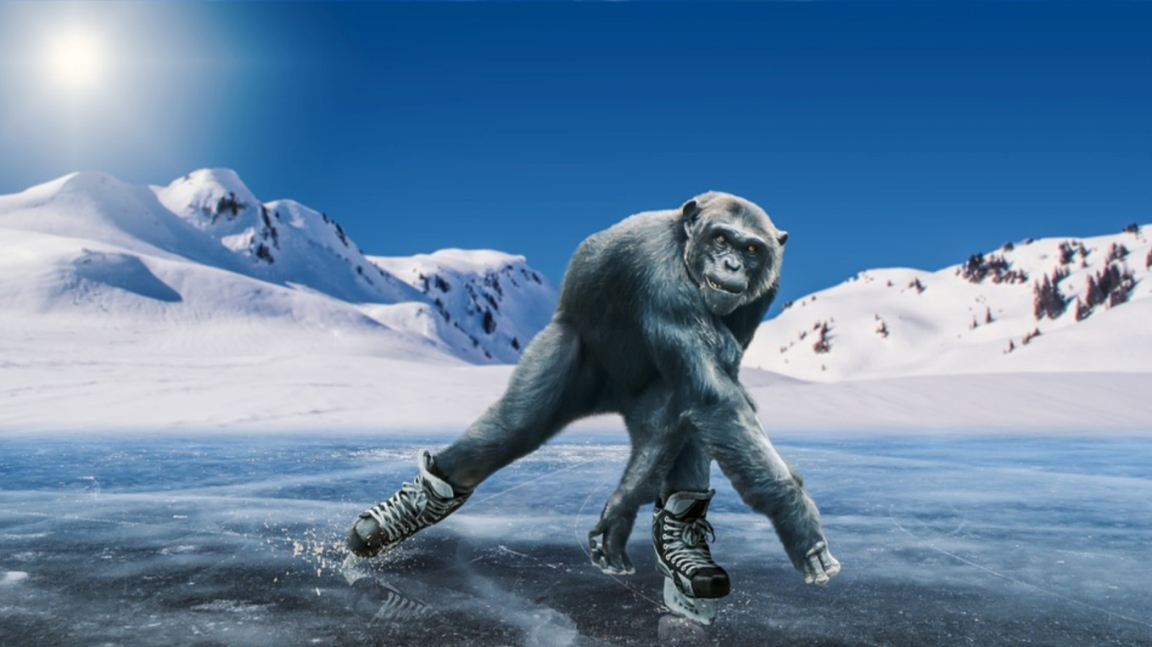
Aping Joe Rogan
The tide of AI-driven fakery rose higher around the Internet’s ankles as the synthesized voice of comedian/podcaster Joe Rogan announced his purported formation of a hockey team made up of chimpanzees.
What happened: Dessa, an AI company based in Toronto, released a recording of its RealTalk technology imitating Rogan. The digital voice, bearing an uncomfortably close resemblance to the real deal, describes the podcaster’s supposed all-simian sports team. Then it tears through tongue twisters before confessing the faux Rogan’s suspicion that he’s living in a simulation.
Behind the news: Researchers have been converging on spoken-word fakes for some time with impressive but generally low-fidelity results. One joker mocked up a classic Eminem rap in the simulated voice of psychologist and culture warrior Jordan Peterson. Dessa employees Hashiam Kadhim, Joe Palermo, and Rayhane Mama cooked up the Rogan demo as an independent project.
How it works: No one outside the company knows. Dessa worries that the technology could be misused, so it hasn’t yet released the model. It says it will provide details in due course.
Why it matters: Simulations of familiar voices could enable new classes of communication tools and empower people who have lost their own voices to disease or injury. On the other hand, they could give malefactors more effective ways to manipulate people on a grand scale. This fake conversation between President Trump and Bernie Sanders is a playful harbinger of what may lie in store.
Yes, but: Dessa’s Rogan impersonation is remarkably true to life, but the rhythm and intonation give it away. The company offers a simple test to find out whether you can distinguish between real and synthesized Rogan voices.
Our take: Deepfakery has legitimate and exciting applications in entertainment, education, and other fields. Yet there's good reason for concern that it could mislead people and create havoc. Some day, it will be so good that no person or AI can recognize it. Brace yourself.
A MESSAGE FROM DEEPLEARNING.AI
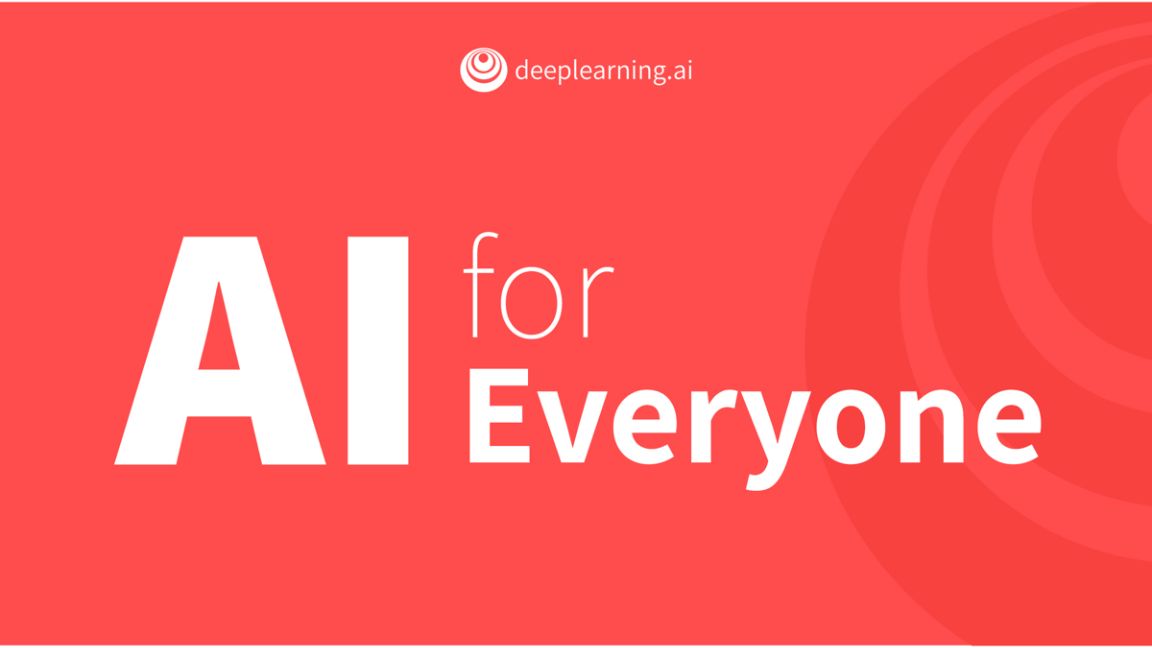
How is a machine learning workflow different from a data science workflow? Walk through examples of each in our nontechnical course, AI For Everyone. Enroll now
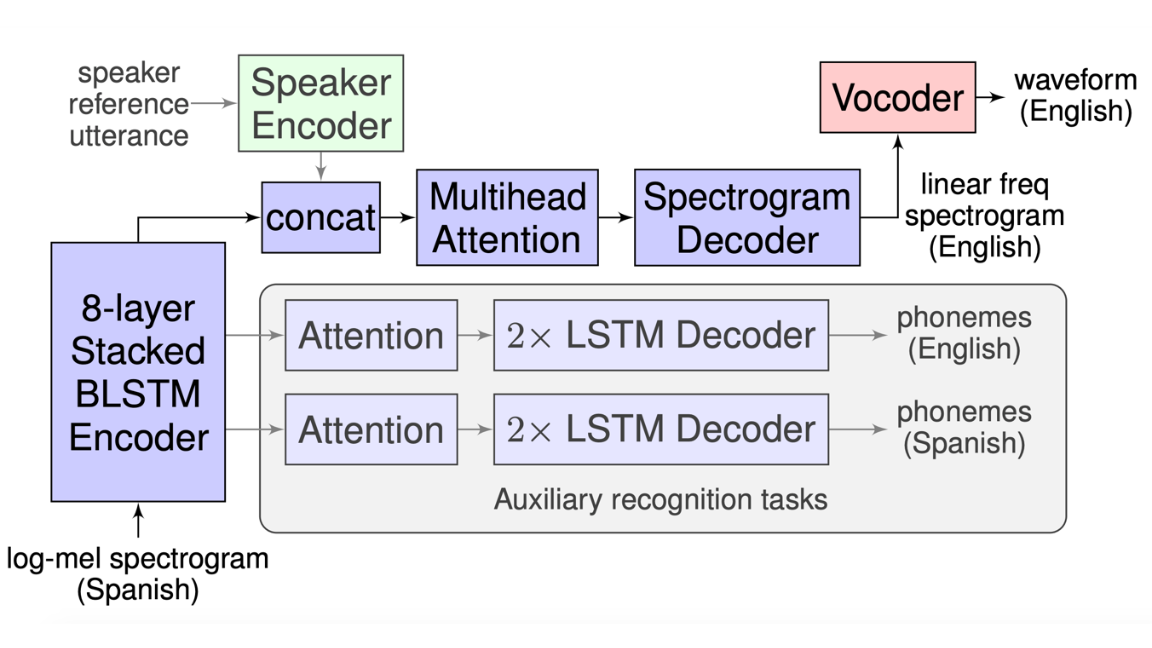
Direct Speech-to-Speech Translation
Systems that translate between spoken languages typically take the intermediate step of translating speech into text. A new approach shows that neural networks can translate speech directly without first representing the words as text.
What’s new: Researchers at Google built a system that performs speech-to-speech language translation based on an end-to-end model. Their approach not only translates, it does so in a rough facsimile of the speaker’s voice. You can listen to examples here.
How it works: Known as Translatotron, the system has three main components: An attentive sequence-to-sequence model takes spectrograms as input and generates spectrograms in a new language. A neural vocoder converts the output spectrograms into audio waveforms. And a pre-trained speaker encoder maintains the character of the speaker’s voice. Translatotron was trained end-to-end on a large corpus of matched spoken phrases in Spanish and English, as well as phoneme transcripts.
Why it matters: The architecture devised by Ye Jia, Ron J. Weiss, and their colleagues offers a number of advantages:
- It retains the speaker’s vocal characteristics in the spoken output.
- It doesn't trip over words that require no translation, such as proper names.
- It delivers faster translations, since it eliminates a decoding step.
- Training end-to-end eliminates errors that can compound in speech-to-text and text-to-speech conversions.
Results: The end-to-end system performs slightly below par translating Spanish to English. But it produces more realistic audio than previous systems and plants a stake in the ground for the end-to-end approach.
The hitch: Training it requires an immense corpus of matched phrases. That may not be so easy to come by, depending on the languages you need.
Takeaway: Automatic speech-to-speech translation is a sci-fi dream come true. Google’s work suggests that such systems could become faster and more accurate before long.
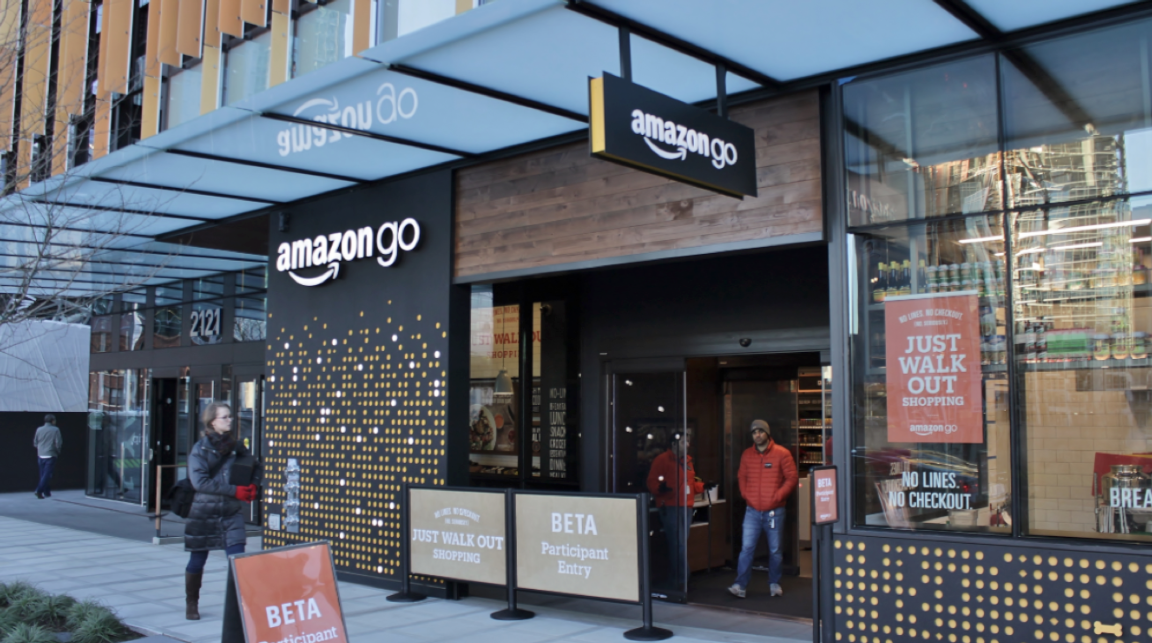
Retailers Spend on Smarter Stores
Retailers are expected to more than triple their outlay for AI over the next few years.
What’s new: A new report from Juniper Research estimates that global retailers will spend $12 billion on AI services by 2023, up from $3.6 billion in 2019. More than 325,000 shopkeepers will adopt AI over that period, mostly in China, the Far East, North America, and West Europe.
Why it’s happening: AI-driven tools are fueling a race among retailers in which early movers will displace competitors, the report says. Three major AI applications are enabling retailers to cut costs, optimize prices, and offer superior service:
- Demand forecasting ($760 million in 2019, $3 billion in 2023) is helping stores stay on top of consumer preferences to optimize inventory and boost sales. Juniper expects the number of retailers using AI-enabled demand forecasting tools to triple by 2023. For instance, Walmart’s pilot store uses computer vision to help employees keep shelves stocked and perishables fresh.
- Smart checkout ($253 million in sales last year, $45 billion in 2023) promises frictionless transactions, but it will take two to three years to settle in. Amazon has opened cashierless stores across Chicago, New York, San Francisco, and Seattle. Walmart's Sam’s Club and 7-11 also are experimenting with this approach.
- Chatbots ($7.3 billion in sales this year, $112 billion in 2023) provide a personalized shopping experience to boost customer retention while cutting costs. For instance, Octane, a bot based on Facebook Messenger, claims to reach 90 percent of customers who have abandoned their online shopping carts, closing sales with 10 percent of them.
To be sure: Sales driven by chatbots likely will come at the expense of other channels, so they may not generate additional revenue. However, they will be more efficient, contributing to return on investment.
Bottom line: Retailers are banking on AI to revolutionize their industry. While they’ve focused for years on ecommerce, AI has the potential to revitalize in-store sales while spurring mobile and other channels.