Dear friends,
Last Friday, I attended the International Conference on Machine Learning. I spoke at the AI and Climate Change workshop on projects we’re doing to model methane emissions and on wind turbines. John Platt gave an overview of climate issues. Chad Frischmann talked about his remarkable work on Project Drawdown (top ideas for reducing climate change). Claire Monteleoni talked about algorithms for combining multiple climate models. Yoshua Bengio talked about using GANs to synthesize images of floods to help people understand the impact of rising sea levels, and many others dove into specific projects.
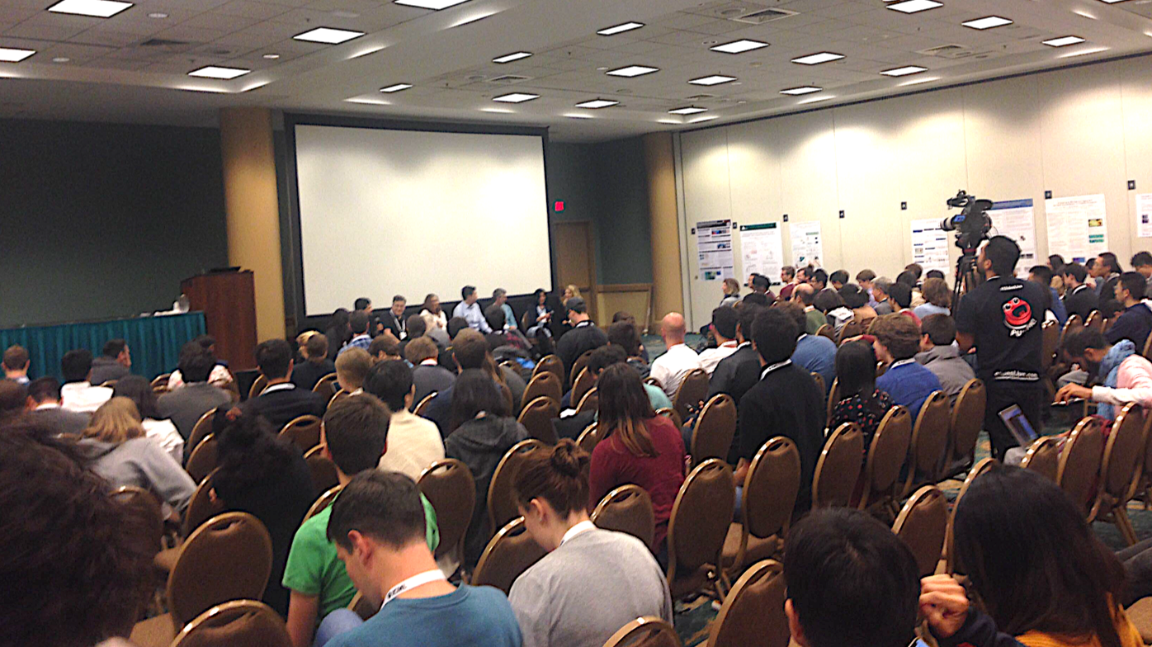
David Rolnick led an effort to compile a list of ways machine learning can impact climate change, resulting in this arXiv paper. (I’m a co-author.) Climate change is one of the most important problems of our time, and we can make a difference!
I spent most of Saturday at the self-supervised learning workshop, where I’m seeing exciting progress in unsupervised learning from images and video. In natural language processing, we’ve already seen how word embeddings can be learned by getting a neural network to predict the next word in a sequence. I saw a lot of papers that built on Aaron van den Oord et al.’s Contrastive Predictive Coding, and multiple authors obtained promising results in learning representations of images from unlabeled data.
Multiple teams are still hitting data and compute scalability issues, but I’m excited about the self-supervised learning research direction and hope more people jump into it.
Keep learning,
Andrew
News
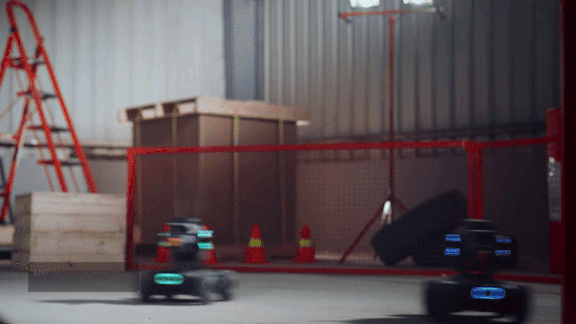
Living Room Wars
Drone maker DJI released a toy robot tank that shoots splattering pellets, skitters on omnidirectional wheels, and learns to navigate the playroom battlefield.
What’s new: Robomaster S1 is a self-driving, bullet-firing toy capable of streaming its turret’s-eye view to your smartphone. And it's programmable. You can see it in action here.
How it works: You can control the unit using a smartphone app, or let it find its own way around using its onboard navigation.
- Tactile sensors keep the bot from getting stuck in corners, like an early-model Roomba.
- The wayfinding algorithms take cues from a front-mounted camera.
- The camera also enables the bot to track targets autonomously.
- It uses a laser beam to hunt other tanks, or it can fire soft gel balls.
More than a toy: If you have the programming chops (or the desire to learn), you can use Python or Scratch 3.0 to teach the tank new maneuvers or subroutines for outsmarting opponents.
Behind the news: Shenzhen-based DJI sponsors an annual tournament where teams of university students battle one another using projectile-shooting tanks, quadcopters, and rail-riding guard drones. The new toy is a consumer version of the tank bots featured in this popular competition.
Our take: At $499, Robomaster S1 makes a pricey educational tool. You can buy an Arduino robot tank kit for a fraction of the cost. On the other hand, few Arduino kits come with a projectile-firing turret, and this thing looks like a lot more fun.
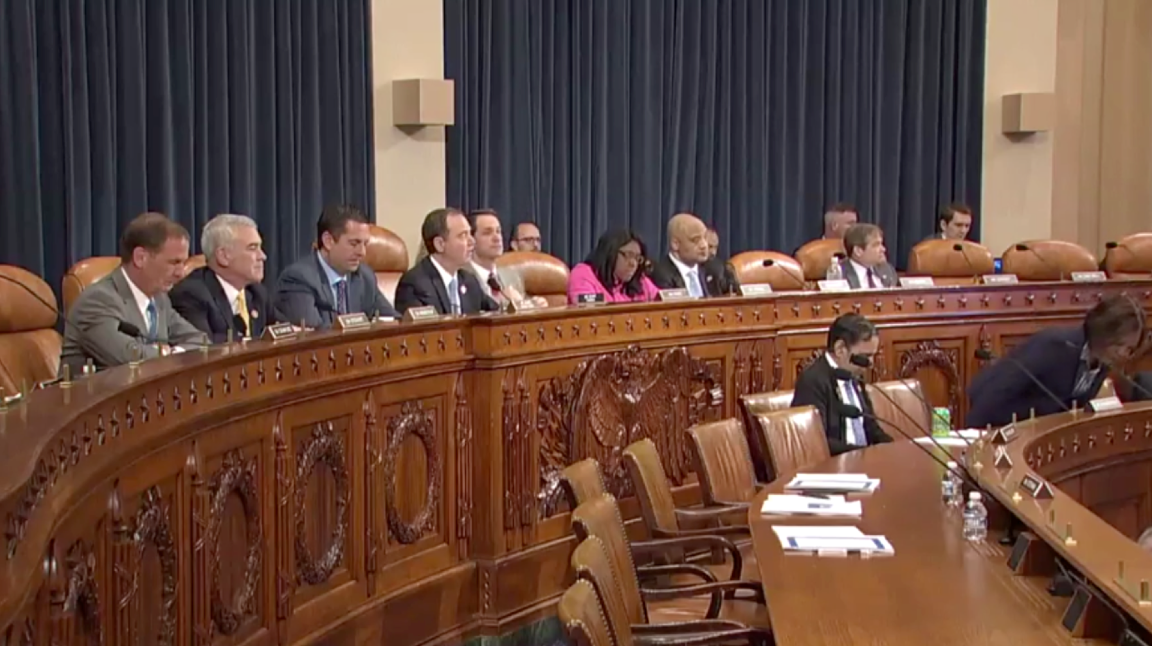
Mr. Deepfake Goes to Washington
U.S. representatives mulled over legal precedents for — and impediments to — regulating the realistic computer-generated videos known as deepfakes.
What’s new: The House Intelligence Committee, worried about the potential impact of fake videos on the 2020 election, questioned experts on AI law, policy, and technology. The panel laid to rest the lawmakers' fear that deepfake technology can fake anybody doing anything. But it highlighted just how easy it is to perpetrate digital hoaxes. The committee contemplated whether to prosecute programmers responsible for deepfake code, and whether regulating deepfakes would impinge on the constitutional right to lie (seriously).
Backstory: Efforts to create realistic-looking video using AI date back to the 1990s. Recently, though, deepfakes have become more sophisticated. Days before the Congressional hearing, activists posted a video of Mark Zuckerberg appearing to deliver a monologue, worthy of a James Bond villain, on the power he wields over popular opinion.
Why it matters: Given the disinformation that swirled around the 2016 election, many experts believe that deepfakes pose a threat to democracy. However, regulating them likely would have a chilling effect on free speech, to say nothing of AI innovation.
Legislative agenda: Congress is considering at least two bills targeting deepfakes.
- One would make it a crime to produce malicious digital manipulations.
- The second would require producers to watermark their creations.
A bigger problem: Digital fakery isn’t just about people using neural networks to synthesize video and voices. Last week, security experts spotted a bogus LinkedIn profile purporting to represent a foreign policy specialist, its portrait photo apparently fabricated by a generative adversarial network. Then there are simple tricks like the slo-mo used to make Speaker of the House Nancy Pelosi appear to slur her words. Not to mention the disinformation potential of Photoshop. And the qwerty keyboard.
Our take: After years of effort, social media platforms are still struggling to define what is and isn’t okay to share. Doing this in the context of deepfake technology won't be easy. House Intelligence Committee chair Adam Schiff (D-CA) hinted at tightening existing regulations — like Section 230 of the Communications Decency Act, which protects platforms from legal liability for content posted by users — to hold platforms accountable for what they publish. This could incentivize services like Facebook and YouTube to root out malicious fakery. But it could also restrict legitimate advocacy or satire. For now, consumers of digital media will have to think twice before believing what they see.
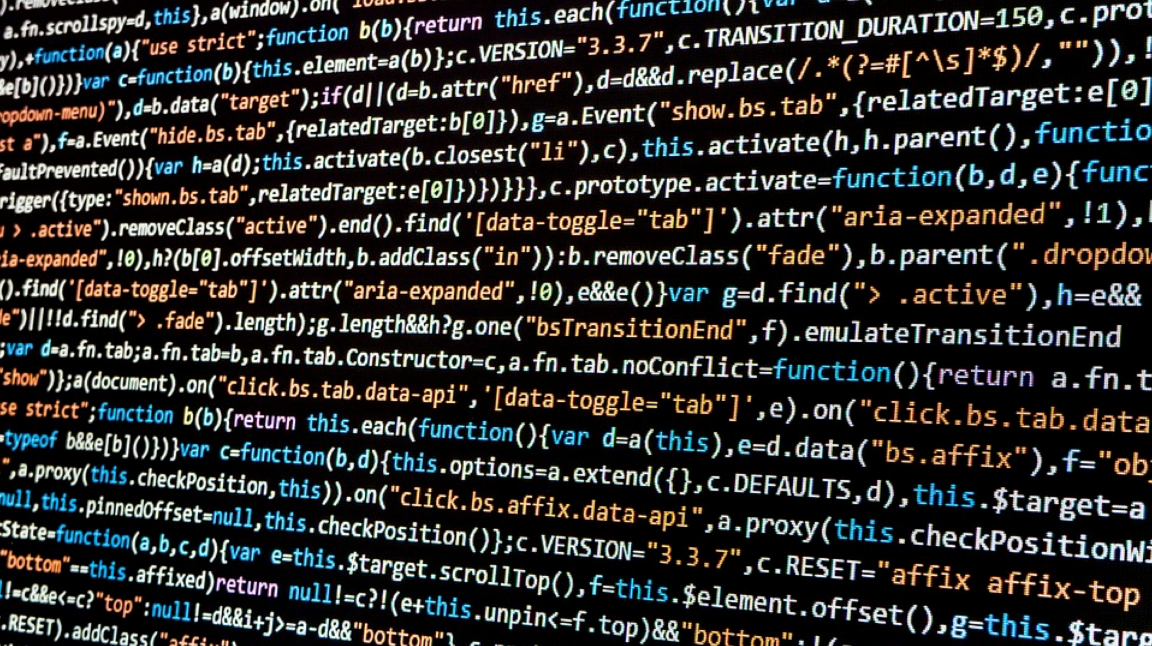
The AI Job Market Shifts
Tech giants have slowed down their hiring for pure AI research. But don’t let that cool your ambitions.
What’s new: Journalist Kevin McLaughlin at The Information spoke with six industry insiders – including Facebook’s Yann LeCun — about hiring trends. His sources said their companies no longer are building huge research teams. Instead, they’re staffing up AI engineering and product development.
Behind the news: The big AI companies were hiring researchers like there’s no tomorrow. Between 2016 and 2017, for instance, Microsoft’s researcher head count jumped from 5,000 to 8,000. Apparently, those efforts were successful enough that Microsoft and its peers have curbed their appetite for boundaries-pushing AI.
The next wave: Corporate AI research may sound like its a victim of its own success, but that’s not the whole story. An insider at Google told McLaughlin that the lower hiring rates for AI researchers need to be kept in context: Google was binging so hard on AI talent that any slowdown is going to look like cold turkey. The company still has plenty of research going on, it’s just not expanding at the former explosive rate. Instead, it’s shifting AI brainpower from research to product.
What they’re saying: “AI is in the process of maturing from academic and basic research, to niche applications, to wide deployment. As the field matures and as the tools become better, companies are massively increasing their investment in the engineering, development, tooling, and infrastructure related to AI.” — Yann LeCun, Facebook’s chief AI scientist, in The Information.
Our take: The majors may be seeing less need for research, but AI is moving from software into every industry, from medicine to agriculture to manufacturing. There's plenty of need for both researchers and product people, and that need will continue to grow.
A MESSAGE FROM DEEPLEARNING.AI
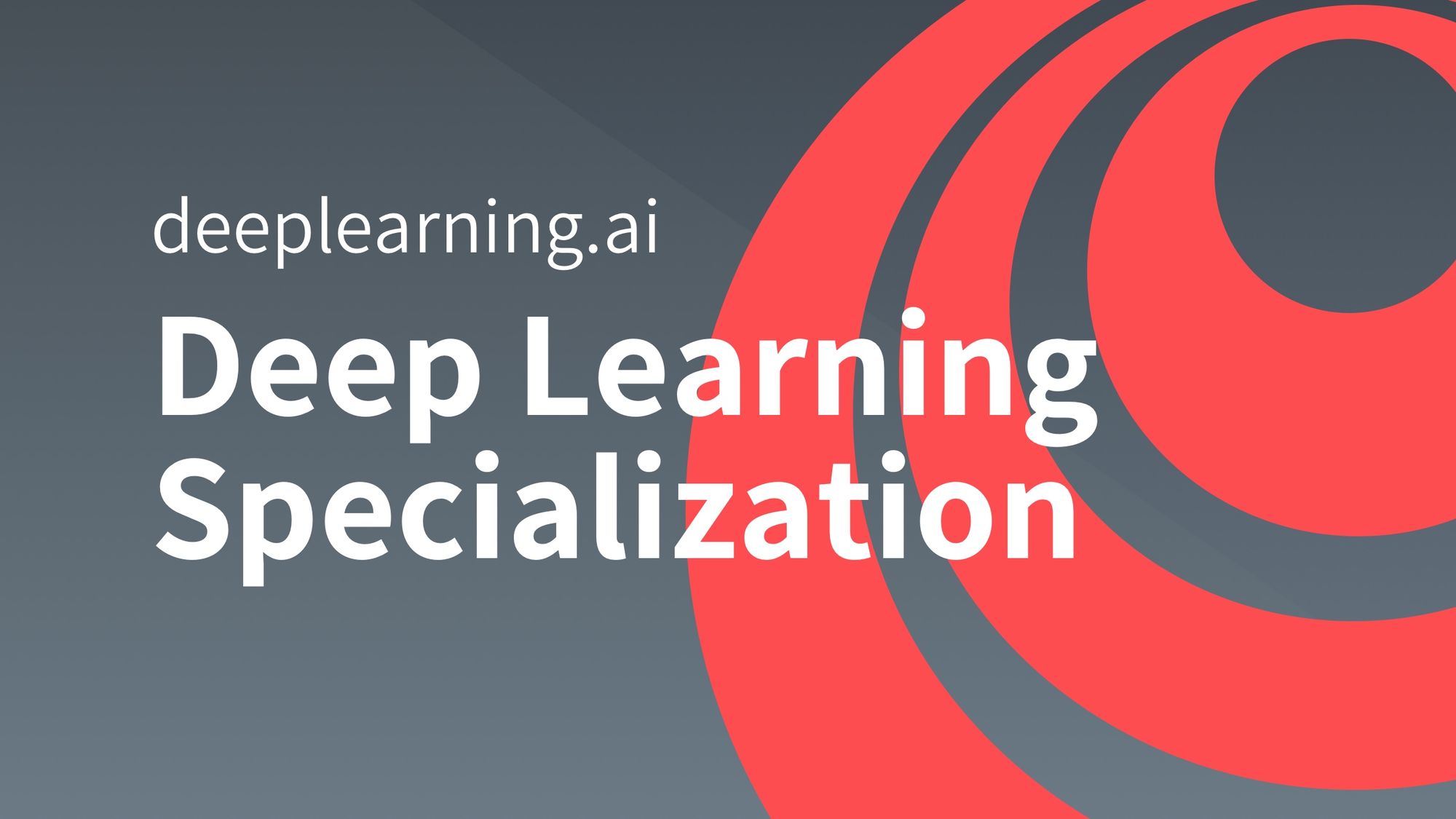
How do you apply deep learning to everything from text and music synthesis to speech recognition? Learn how to build sequence models in the Deep Learning Specialization. Enroll now
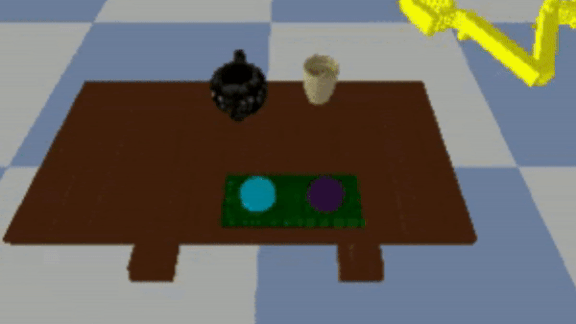
Robots Can Learn From Mistakes
Typical deep reinforcement learning requires millions of training iterations as the network stumbles around in search of the right solution. New research suggests that AI can learn faster by considering its mistakes.
What’s new: Previous work in reinforcement learning taught machines by having them either experiment until they got it right or imitate human demonstrations. Allan Zhou and his team at Google Brain merge the two approaches in an algorithm they call Watch, Try, Learn.
Key insight: Unlike many RL models, WTL decides what actions to take by combining its own prior performance with human demonstrations as an explicit input. This allows it to correct its behavior quickly and minimize repeated blunders.
How it works: WTL is a training algorithm rather than a specific model. It trains two separate policies, or predictions of what action will maximize its reward, called trial and retrial. The trial policy generates exploration attempts, while the retrial policy decides the best actions. These policies are trained on several tasks.
- WTL starts with a handful of human demonstrations. The trial policy uses them to try to learn directly how to replicate human demonstrations. Trial attempts are recorded alongside the demonstrations to form a large dataset including cases of both successes and failures.
- Then it acts according to the retrial policy. This policy — combining the current state, a human demonstration, and trial attempts at the task — is trained to imitate successful demonstrations and trials.
- During inference, even a few demonstrations can generate a large set of trial explorations that help the retrial policy to generate actions that successfully complete tasks previously unseen in training.
Why it matters: WTL can learn a greater number and variety of robotic object manipulation tasks than previous RL models. Further, previous models are limited to a single task, while WTL learns multiple tasks concurrently and outperforms single-task models in every task. This allows WTL to master new abilities in few-shot settings and with less computation.
Takeaway: Who wants a robot helper that requires thousands of attempts to learn how to empty a dishwasher without breaking dishes? And, once it has learned, can’t do anything else? Zhou et al. raise the prospect that smarter, more flexible robots may be just around the corner — though they’re still bound to break a few dishes.
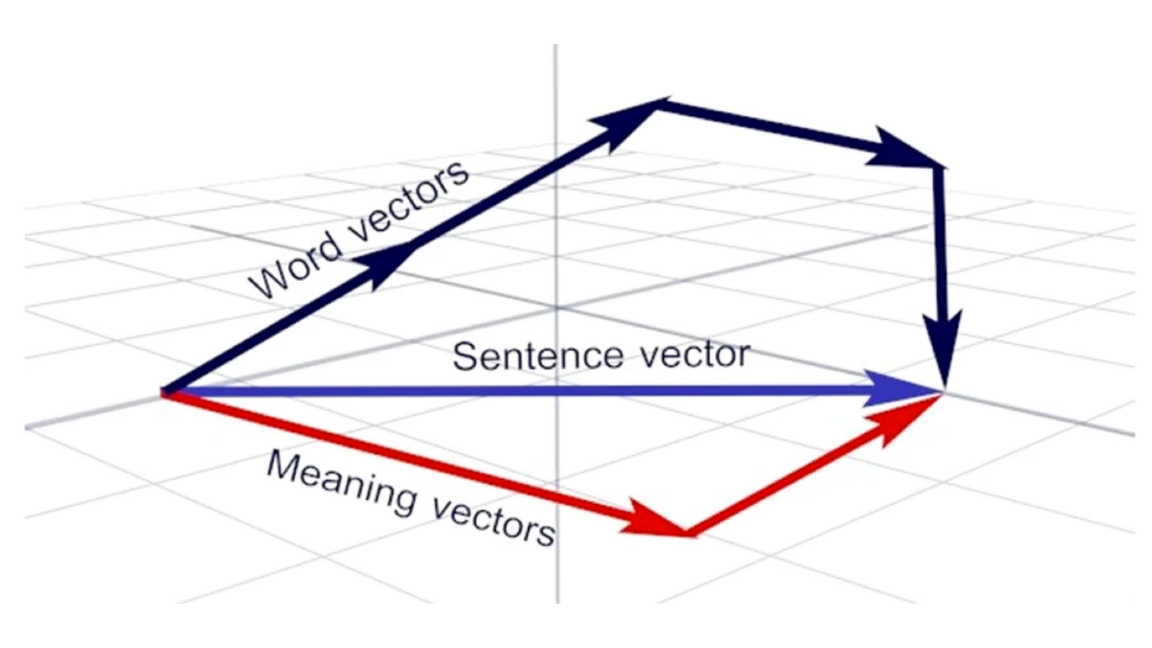
The Sound of Psychosis
Neuroscientists developed a system that, they say, can detect subtle signs of psychosis in conversational speech.
What’s new: Researchers at Emory School of Medicine used machine learning to predict the onset of schizophrenia in a high-risk population with 80 percent accuracy. Their results were published in the journal npj Schizophrenia.
How it works: The researchers trained their neural network on thousands of conversations from Reddit to establish conversational norms and organize word vectors by usage.
- They fed the system transcripts of interviews between young people at high risk of psychosis and their doctors, labeled to indicate speakers who eventually developed schizophrenia.
- Among patients who eventually developed the disease, the researchers found higher rates of two verbal tics: words related to sound (such as loud, hush, and whisper) and use of multiple words with similar meanings.
Why It matters: Schizophrenia is a devastating condition that has no cure, but early detection can help people seek treatment before it becomes overwhelming.
Takeaway: Methods exist to identify warning signs of schizophrenia in patients as young as 17, but only around 30 percent of these people eventually develop the disorder. Machine-learning techniques could help doctors spot the patients who really need help.
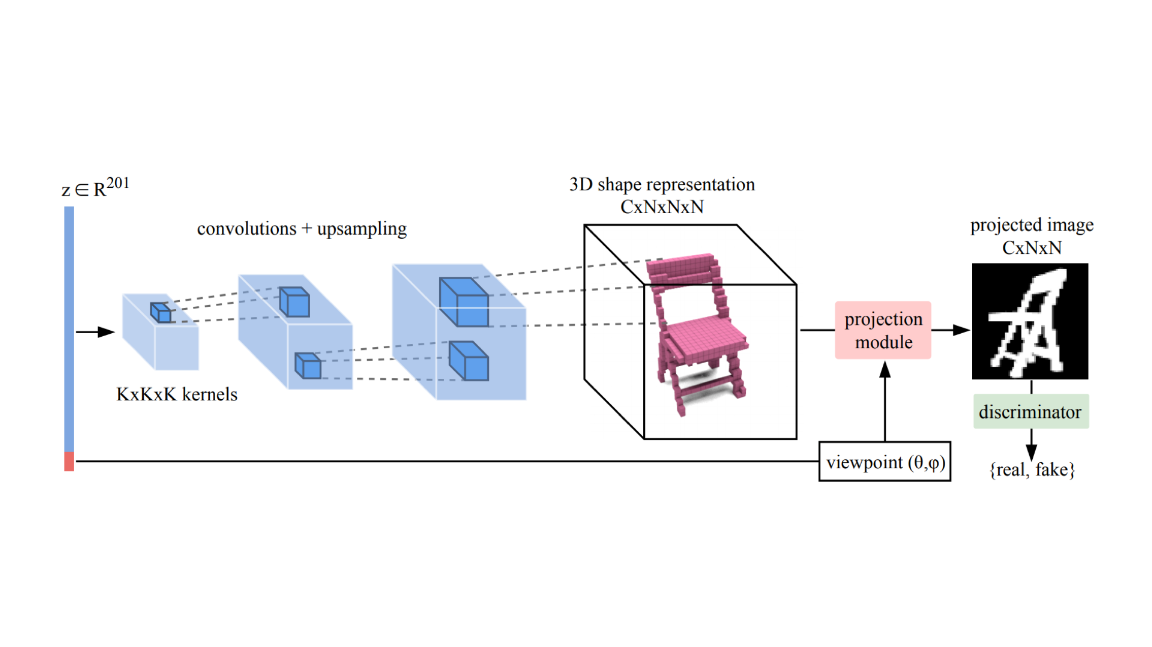
3D Shapes From 2D Pictures
Images produced by generative adversarial networks can be nearly indistinguishable from the real thing. If a picture is worth a thousand words, a GAN-made 3D representation could be worth a million.
What’s new: Gadelha et al. at the University of Massachusetts Amherst developed a neural network, Projective Generative Adversarial Network, that generates 3D models of novel objects from any number of 2D silhouettes.
Key insights: PrGAN’s discriminator relies on 2D images rather than 3D shapes.
- Generating 3D models solely from 2D images requires fewer parameters than earlier approaches like MIT’s 2016 3D-GAN, which is directly trained on hand-built 3D models to produce novel 3D shapers. That means a smaller memory footprint and less training time. And since PrGAN is trained on images rather than 3D models, training data is more readily available.
- Many algorithms that produce 2D views of 3D objects are incompatible with backpropagation, making training on such a system on 2D images impossible. The math behind PrGAN’s projection module allows backprop to be used for training.
How it works: All GANs have a generator that produces new output and a discriminator that learns to classify that output as real or fake. PrGAN has a generator that creates 3D shapes. Its discriminator compares 2D images of those shapes with real-world pictures. A projection component creates the 2D views.
- The generator is a CNN trained to construct 3D shapes from random inputs. The resulting 3D representation is voxelized, or built from small cubes, rather than a polygonal mesh.
- The generator passes a 3D shape to the projection module, which computes a 2D view from a random viewpoint. The real-life 2D silhouettes and generated 2D views are used in training the discriminator. If the discriminator correctly classifies generated 2D views as fake, the generator is adjusted to produce more realistic results.
- PrGAN’s projection algorithm and shape generator can be trained with depth maps, color schemes, and segmented images rather than binary silhouettes. The additional information allows more detailed, diverse shapes.
Why it matters: The graphic design industry is looking toward deep learning to enhance productivity. PrGAN simplifies creation of 3D models from 2D images. This could be a great help to designers and game makers looking to generate 3D representations quickly.
We’re thinking: Deep learning consumes huge amounts of data. PrGAN takes advantage of plentiful 2D images to produce 3D representations, which are far less common. This sort of data-set bootstrapping could be an alternative in situations where training data is scarce.